Network effects determine the success of technologies from phone calls to online shopping platforms, and AI tools like ChatGPT are no exception . The difference, however, is how these network effects work. Data network effects are a new form, like the more familiar direct and indirect network effects, where the value of a technology increases as the number of users it acquires increases.
However, the value we are discussing today is not the number of phone calls or the presence of many buyers and sellers on an e-commerce platform, but rather the feedback that comes from helping it make better predictions. More users means more responses, which further improves prediction accuracy, creating a virtuous cycle. There are three lessons for businesses to consider: 1) Feedback is critical, 2) Gather information regularly and meticulously, and 3) Consider the data that is shared intentionally or unintentionally.
When OpenAI launched ChatGPT late last year, the reaction from industry observers was a mixture of praise and concern. We’ve all heard how the technology is putting computer programmers, teachers, financial traders and analysts, graphic designers and artists alike out of work en masse. Many universities are rushing to revise course plans and related requirements out of concern that AI will stifle the creative abilities of college students. Others say that perhaps the most direct impact is that ChatGPT can reshape or even replace traditional Internet search engines. Search and related advertising generate the vast majority of Google’s revenue, so will chatbots be the end of Google?
ChatGPT is an excellent demonstration of machine learning technology, but is almost unfeasible as a standalone service. In order to leverage its technical strength, OpenAI needs a partner. So we weren't surprised when the company quickly announced a deal with Microsoft. The combination of an AI startup and an established tech company could ultimately pose a credible threat to Google's dominance, raising the stakes in the "AI arms race." It also provides a lesson on the forces that will determine which companies will thrive and which will falter when deploying this technology.
To understand what compelled OpenAI to align with Bing (and why Google might still win), we considered how the technology differs from past developments, such as phone calls or marketplace platforms like Uber or Airbnb. In these examples, network effects—where the value of a product rises as more users—play an important role in determining how these products grow and which companies succeed. Generative AI services like ChatGPT are subject to similar but different types of network effects. In order to choose an AI-friendly strategy, managers and entrepreneurs must understand how this new AI network effect works.
Network effects work differently for AI
The value of artificial intelligence lies in accurate predictions and recommendations. But unlike traditional products and services that rely on converting supplies (such as electricity or human capital) into outputs (such as lighting or tax advice), AI requires massive data sets that must be kept up to date through back-and-forth customer interactions. To remain competitive, AI operators must collect data, analyze the data, provide predictions, and then seek feedback to refine the recommendations. The value of the system depends on the data from the users and increases with the data.
The performance of this technology—the ability to accurately predict and advise—depends on an economic principle called data network effects (some prefer to call it data-driven learning). These are distinct from familiar direct network effects, such as a phone call becoming more valuable as your user base grows because you can call more people. They are also different from indirect network effects, which describe how more buyers invite more sellers to join the platform, and vice versa—when more sellers are present, shopping on an e-commerce platform or on Airbnb Booking a room becomes more attractive.
Data network effects are a new form: Like the more familiar effect, the more users, the more valuable the technology becomes. But here, value does not come from the number of peers, nor from the presence of many buyers and sellers. Rather, these impacts stem from the nature of the technology: AI improves through reinforcement learning, prediction, and feedback. As intelligence increases, systems can make better predictions, enhance their usefulness, attract new users and retain existing users. More users means more responses, which further improves prediction accuracy, creating a virtuous cycle.
Take Google Maps as an example, which uses AI to recommend the fastest route to your destination. This ability depends on predicting true traffic patterns in alternative paths, which is achieved by leveraging data from many users. Here, data users are also suppliers, and the more people use Google Maps, the more historical and concurrent data it accumulates. With a wealth of data, Google can compare countless predictions with actual results: Did you arrive at the time the app predicted? To refine the predictions, the app also needs your impressions: How good was the description? As objective facts and subjective comments accumulate, network effects come into play. These effects improve predictions and increase the value of the application to users and to Google.
Once we understand how network effects drive artificial intelligence, we can imagine the new strategies this technology will require.
OpenAI and Microsoft
Let’s start with the marriage between OpenAI and Microsoft. When we beta-tested ChatGPT, we were impressed by its creative, human-like responses, but also realized it had its bottlenecks: It relies on massive amounts of data last collected in 2021, so don't ask about recent events or even weather. Worse, it lacks a robust feedback loop mechanism.
However, through a link with Microsoft, OpenAI found a way to test its predictions. Bing users' questions - and how they rate the answers - are critical to updating and improving ChatGPT. The next step, we imagine, is for Microsoft to feed the massive cloud of user data it maintains into the algorithm. While ChatGPT can digest countless Excel sheets, PowerPoint presentations, Word documents, and LinkedIn resumes, it will do a better job at recreating those files to the delight or horror of those in the office.
There are at least three broad lessons here.
First of all, feedback is crucial. The value of AI increases with continued user response. To stay smart, the algorithm needs a data stream of current user selections and past recommendation ratings. Without feedback, even the best engineering algorithms won't stay smart for long. As OpenAI realizes, even the most complex models need to be linked to constantly flowing data sources. AI entrepreneurs should be well aware of this.
Second, executives shouldcollect informationregularly and meticulously to maximize the impact. They should go through typical financial and operating records. Useful data is everywhere, both inside and outside the enterprise. They may come from interactions with buyers, suppliers, and colleagues. For example, retailers can track what consumers view, what they put into their shopping carts, and what they ultimately pay for. Cumulatively, these tiny details can dramatically improve an AI system’s predictions. Even uncommon data, including data over which a business has no control, may be worth collecting. Weather data helps Google Maps predict traffic conditions. Tracking the keywords recruiters use to search for resumes can help LinkedIn provide candidates with tips for success.
Finally, Everyone should consider the data they share either intentionally or unintentionally. Facts and feedback are critical to building better predictions, but the value of your data may be captured by others, and executives should consider which AI can benefit from the data they share (or allow access to). Sometimes, they should limit sharing. For example, when Uber drivers use the app Waze to navigate, they help Google estimate the frequency and length of ride-hailing trips. Such data could be invaluable as Google considers operating self-driving taxis.
Additionally, when brands like Adidas sell products on Amazon, the retail giant can estimate demand for different brands (such as compared to Nike) and categories, as well as buyer sensitivity to price. . The results could feed into competitors or benefit Amazon's private-label products. To combat this, executives can avoid third-party platforms or intermediaries. They can negotiate data access, and they can work to maintain direct contact with their customers. Sometimes, the best solution may be to have data owners bind and share in a data exchange, as banks have done when establishing ways to share credit data.
When you consider AI network effects, we can better understand the future of the technology. You can also see how these effects, like other network effects, make the rich even richer. The dynamics behind AI mean that first movers may be richly rewarded, while followers, no matter how fast, may be left behind. It also means that when one has access to AI algorithms and data streams, the advantages accrue over time and cannot be easily surpassed. For executives, entrepreneurs, policymakers, and everyone else, the best and worst of artificial intelligence are yet to come.
The above is the detailed content of How network effects can make artificial intelligence smarter. For more information, please follow other related articles on the PHP Chinese website!
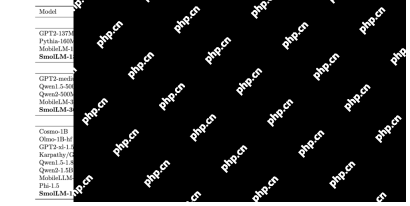
Harness the Power of On-Device AI: Building a Personal Chatbot CLI In the recent past, the concept of a personal AI assistant seemed like science fiction. Imagine Alex, a tech enthusiast, dreaming of a smart, local AI companion—one that doesn't rely
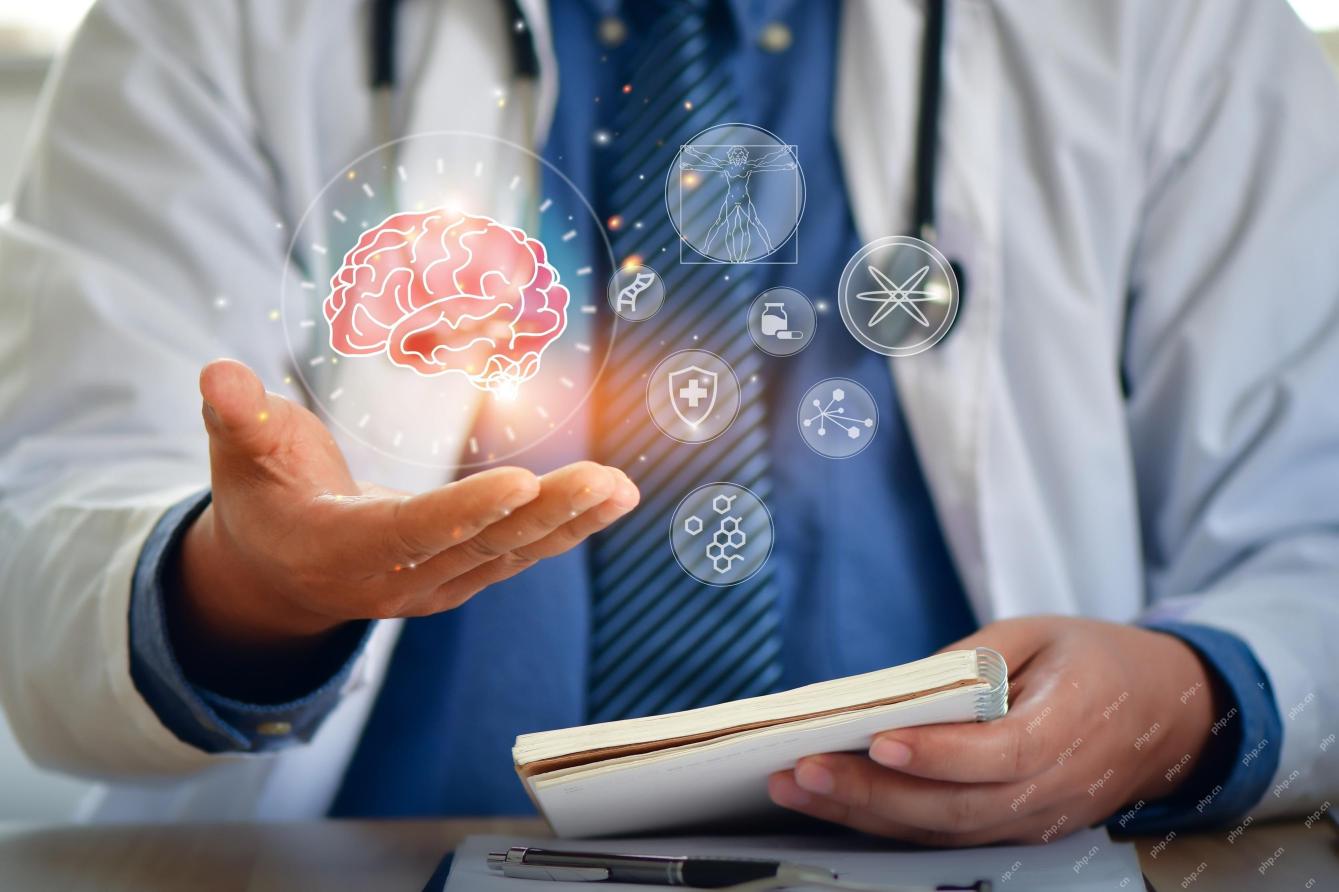
Their inaugural launch of AI4MH took place on April 15, 2025, and luminary Dr. Tom Insel, M.D., famed psychiatrist and neuroscientist, served as the kick-off speaker. Dr. Insel is renowned for his outstanding work in mental health research and techno
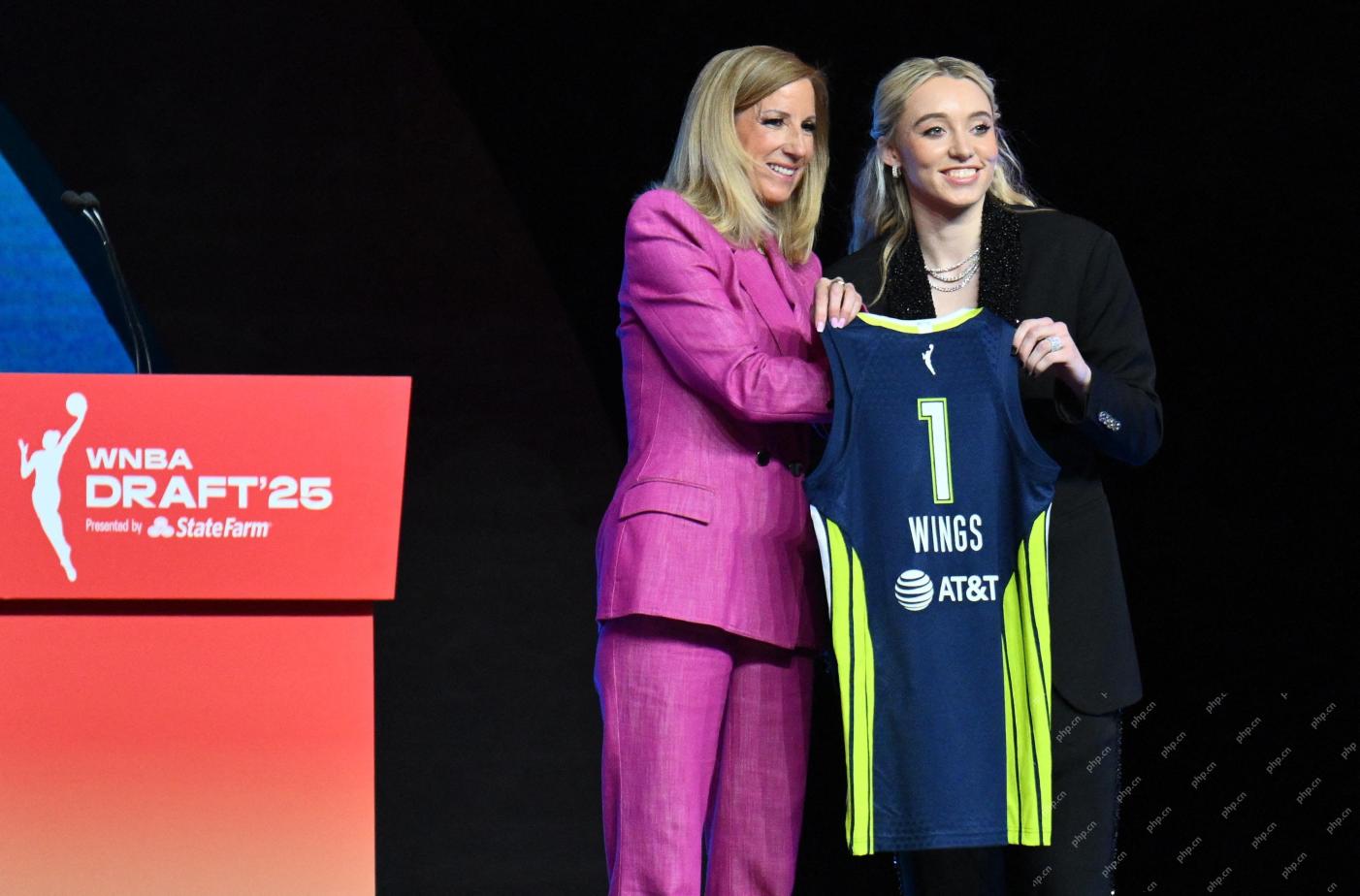
"We want to ensure that the WNBA remains a space where everyone, players, fans and corporate partners, feel safe, valued and empowered," Engelbert stated, addressing what has become one of women's sports' most damaging challenges. The anno
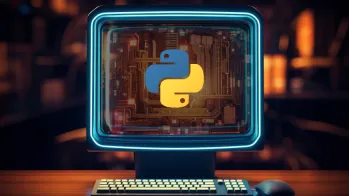
Introduction Python excels as a programming language, particularly in data science and generative AI. Efficient data manipulation (storage, management, and access) is crucial when dealing with large datasets. We've previously covered numbers and st
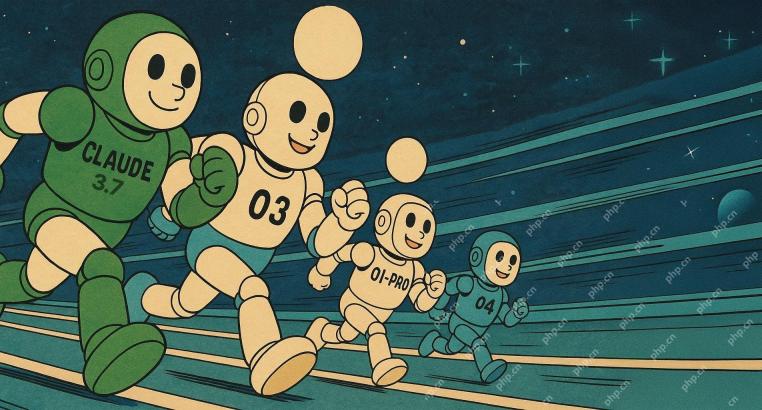
Before diving in, an important caveat: AI performance is non-deterministic and highly use-case specific. In simpler terms, Your Mileage May Vary. Don't take this (or any other) article as the final word—instead, test these models on your own scenario
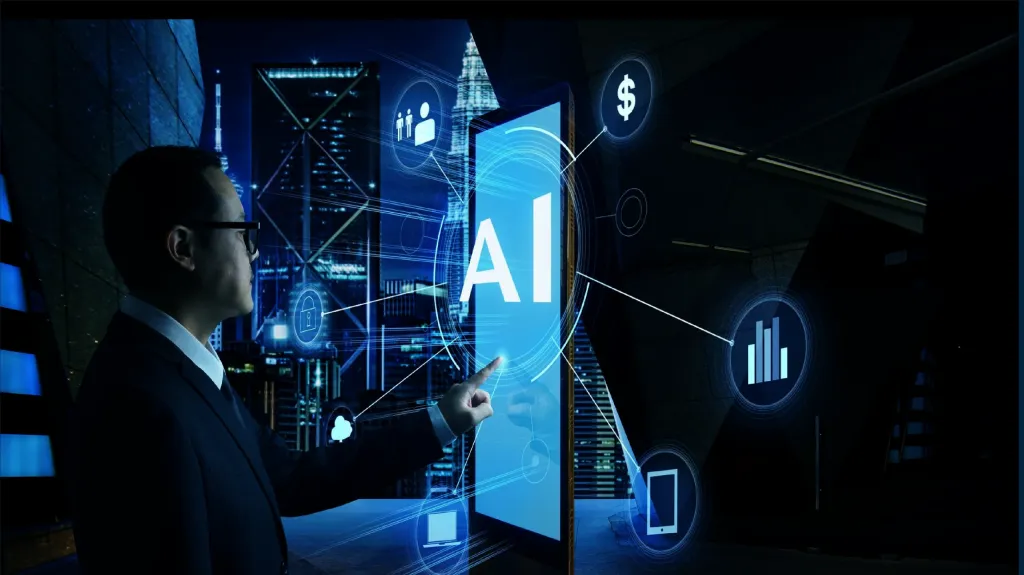
Building a Standout AI/ML Portfolio: A Guide for Beginners and Professionals Creating a compelling portfolio is crucial for securing roles in artificial intelligence (AI) and machine learning (ML). This guide provides advice for building a portfolio
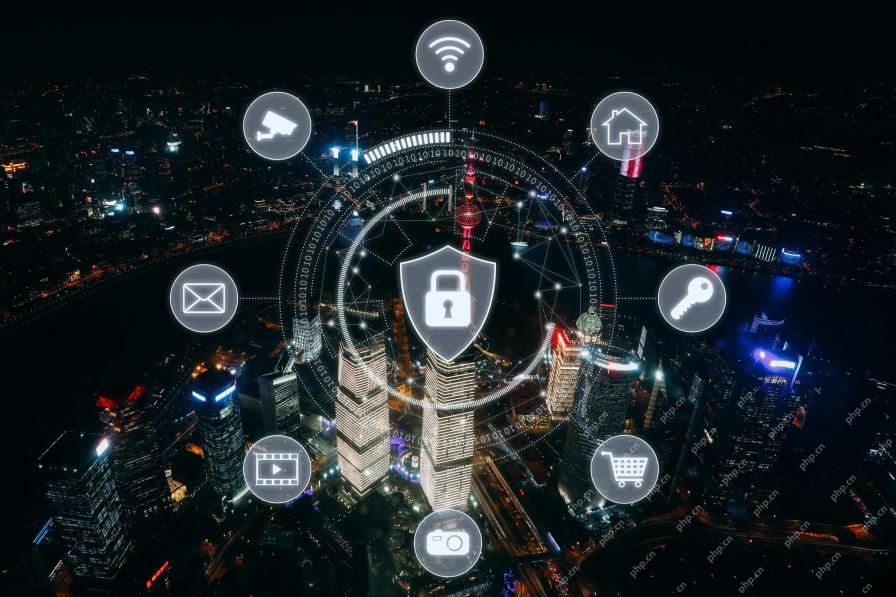
The result? Burnout, inefficiency, and a widening gap between detection and action. None of this should come as a shock to anyone who works in cybersecurity. The promise of agentic AI has emerged as a potential turning point, though. This new class
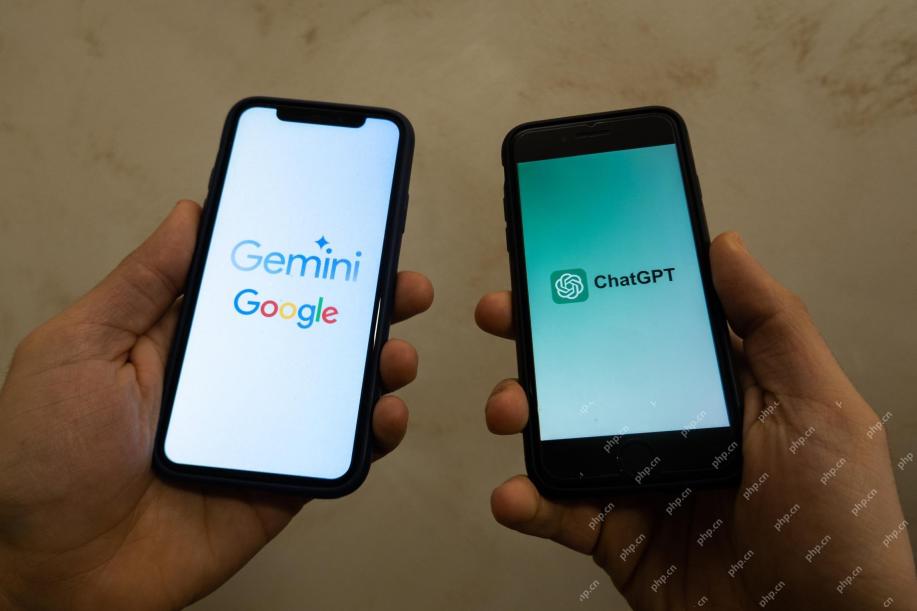
Immediate Impact versus Long-Term Partnership? Two weeks ago OpenAI stepped forward with a powerful short-term offer, granting U.S. and Canadian college students free access to ChatGPT Plus through the end of May 2025. This tool includes GPT‑4o, an a


Hot AI Tools

Undresser.AI Undress
AI-powered app for creating realistic nude photos

AI Clothes Remover
Online AI tool for removing clothes from photos.

Undress AI Tool
Undress images for free

Clothoff.io
AI clothes remover

AI Hentai Generator
Generate AI Hentai for free.

Hot Article

Hot Tools

Notepad++7.3.1
Easy-to-use and free code editor

SecLists
SecLists is the ultimate security tester's companion. It is a collection of various types of lists that are frequently used during security assessments, all in one place. SecLists helps make security testing more efficient and productive by conveniently providing all the lists a security tester might need. List types include usernames, passwords, URLs, fuzzing payloads, sensitive data patterns, web shells, and more. The tester can simply pull this repository onto a new test machine and he will have access to every type of list he needs.

PhpStorm Mac version
The latest (2018.2.1) professional PHP integrated development tool

Atom editor mac version download
The most popular open source editor
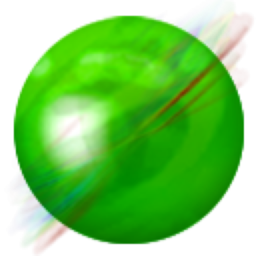
ZendStudio 13.5.1 Mac
Powerful PHP integrated development environment