


With the development of computer graphics, 3D generation technology is gradually becoming a research hotspot. However, there are still many challenges in generating 3D models from text or images.
Recently, companies such as Google, NVIDIA, and Microsoft have launched 3D generation methods based on neural radiation fields (NeRF), but these methods are compatible with traditional 3D rendering software (such as Unity, Unreal Engine, Maya, etc.) Sexual issues limit its wide application in practical applications.
To this end, the R&D team of Yingmo Technology and ShanghaiTech University proposed a text-guided progressive 3D generation framework designed to solve these problems.
Generate 3D assets based on text descriptions
The text-guided progressive 3D generation framework (DreamFace for short) proposed by the research team combines visual-language models, implicit diffusion models and physics-based Material diffusion technology generates 3D assets that comply with computer graphics production standards.
The innovation of this framework lies in its three modules: geometry generation, physics-based material diffusion generation and animation capability generation.
This work has been accepted by the top journal Transactions on Graphics and will be presented at SIGGRAPH 2023, the top international computer graphics conference.
Project website: https://sites.google.com/view/dreamface
Preprint paper: https://arxiv.org/abs/2304.03117
Web Demo: https://hyperhuman.top
HuggingFace Space: https ://huggingface.co/spaces/DEEMOSTECH/ChatAvatar
How to implement the three major functions of DreamFace
DreamFace mainly includes three modules, geometry generation and physics-based materials Diffusion and animation capabilities are generated. Compared with previous 3D generation work, the main contributions of this work include:
- proposes DreamFace, a novel generation scheme that combines recent visual-language models with animatable and physically materialable faces Assets are combined to separate geometry, appearance and animation capabilities through progressive learning.
- Introduces the design of dual-channel appearance generation, combining a novel material diffusion model with a pre-trained model, while performing two-stage optimization in latent space and image space.
- Facial assets using BlendShapes or generated Personalized BlendShapes are animated and further demonstrate the use of DreamFace for natural character design.
Geometry generation: This module generates a geometric model based on text prompts through the CLIP (Contrastive Language-Image Pre-Training) selection framework.
First randomly sample candidates from the face geometric parameter space, and then select the rough geometric model with the highest matching score based on text prompts.
Next, implicit diffusion model (LDM) and Scored Distillation Sampling (SDS) processing are used to add facial details and detailed normal maps to the coarse geometry model to generate high-precision geometry.
Physically Based Material Diffusion Generation: This module targets predicted geometry and text Tips for generating facial textures. First, the LDM is fine-tuned to obtain two diffusion models.
The two models are then coordinated through a joint training scheme, one for directly denoising U-texture maps and the other for supervised rendering of images. Additionally, a hint learning strategy and non-face area masking are employed to ensure the quality of the generated diffuse maps.
Finally, apply the super-resolution module to generate 4K physically-based textures for high-quality rendering.
Animation capability generation: The model generated by DreamFace has animation capability. Different from traditional BlendShapes-based methods, this framework animates the Neutral model by predicting unique deformations to generate personalized animations.
The geometric generator is first trained to learn the expression latent space, and then the expression encoder is trained to extract expression features from RGB images. Finally, personalized animations are generated by using monocular RGB images.
Generate specified 3D assets in 5 minutes
The DreamFace framework has achieved good results in tasks such as celebrity generation and description generation characters, and has achieved results exceeding previous work in user evaluations.
At the same time, compared with existing methods, it has obvious advantages in running time.
In addition, DreamFace supports texture editing using tips and sketches to achieve global editing effects (such as aging, makeup) and local editing effects (such as tattoos) , beard, birthmark).
Can be used in film, television, games and other industries
As a text-guided progressive 3D generation framework, DreamFace combines visual -Language model, implicit diffusion model and physically based material diffusion technology achieve 3D generation with high precision, high efficiency and good compatibility.
This framework provides an effective solution for solving complex 3D generation tasks and is expected to promote more similar research and technology development.
In addition, physically based material diffusion generation and animation capability generation will promote the application of 3D generation technology in film and television production, game development and other related industries.
The above is the detailed content of Generate a Marvel 3D digital human in five minutes! The American Spider-Man and the Joker can all do it, and the facial details are restored in high definition.. For more information, please follow other related articles on the PHP Chinese website!
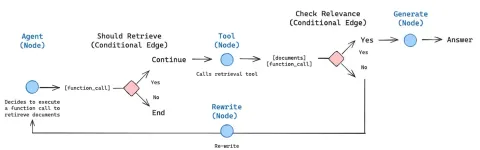
AI agents are now a part of enterprises big and small. From filling forms at hospitals and checking legal documents to analyzing video footage and handling customer support – we have AI agents for all kinds of tasks. Compan
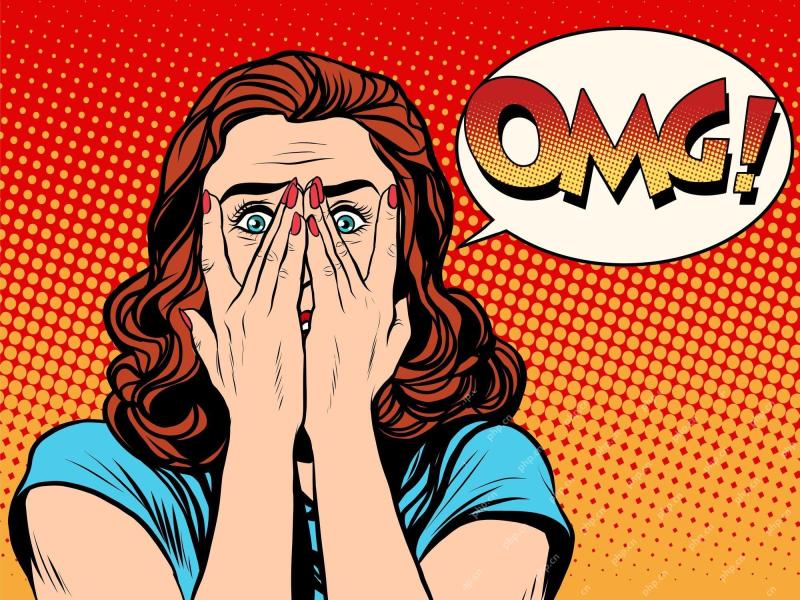
Life is good. Predictable, too—just the way your analytical mind prefers it. You only breezed into the office today to finish up some last-minute paperwork. Right after that you’re taking your partner and kids for a well-deserved vacation to sunny H
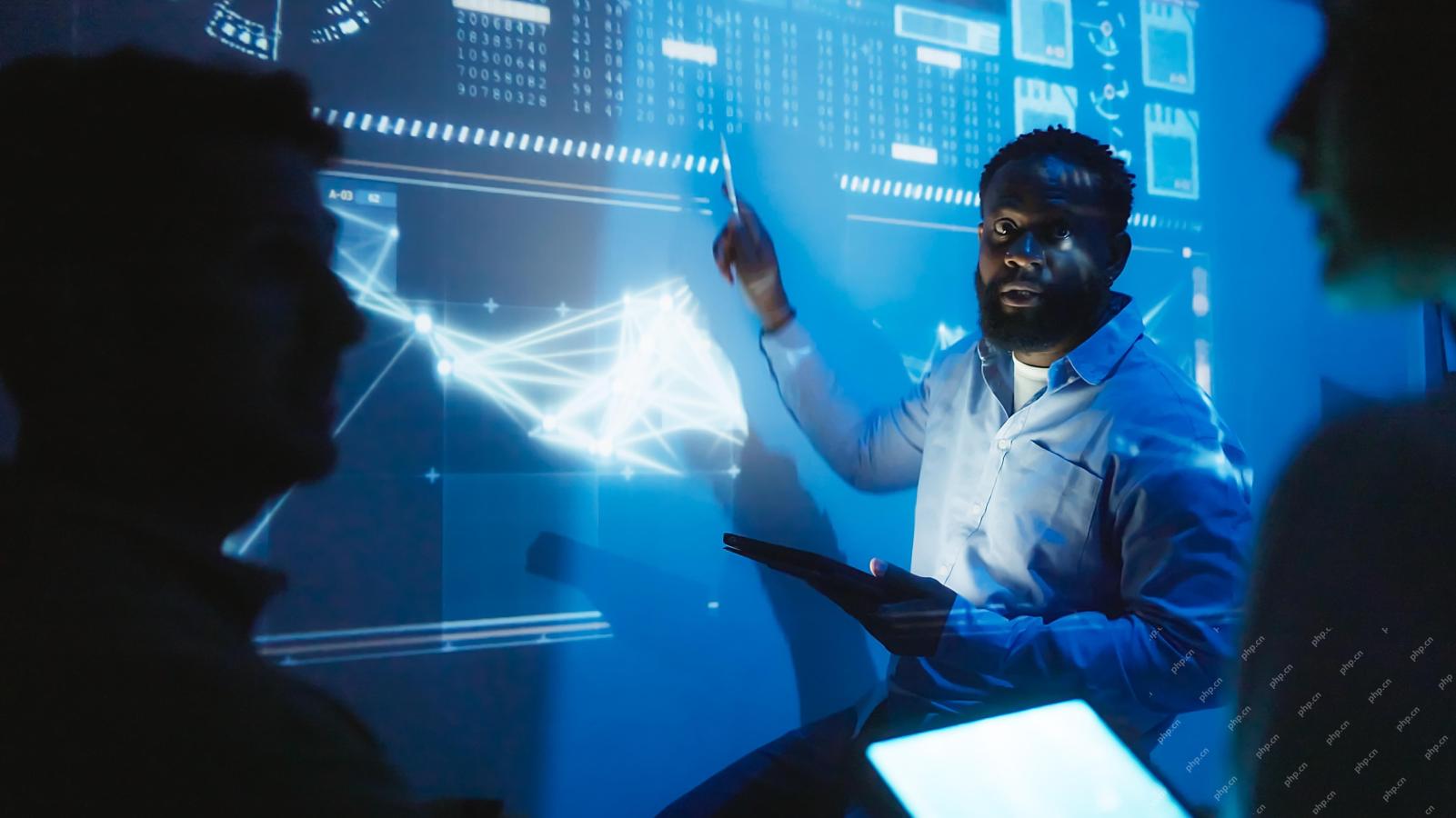
But scientific consensus has its hiccups and gotchas, and perhaps a more prudent approach would be via the use of convergence-of-evidence, also known as consilience. Let’s talk about it. This analysis of an innovative AI breakthrough is part of my
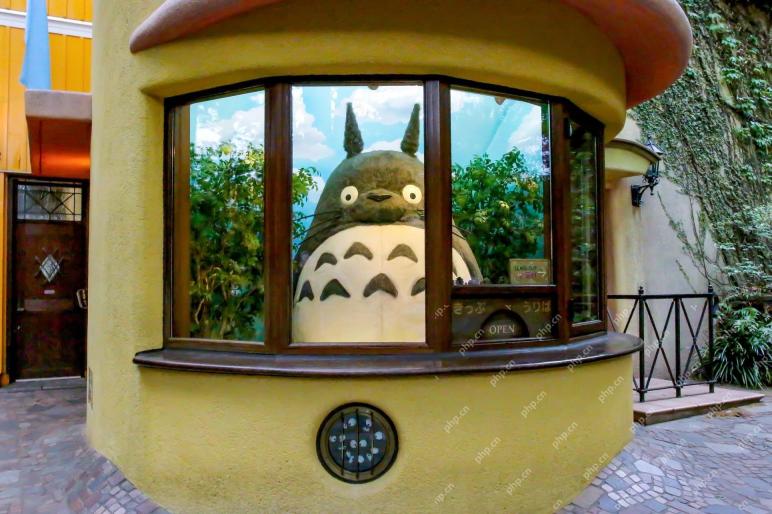
Neither OpenAI nor Studio Ghibli responded to requests for comment for this story. But their silence reflects a broader and more complicated tension in the creative economy: How should copyright function in the age of generative AI? With tools like
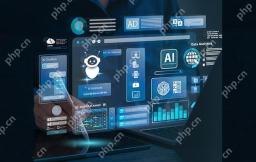
Both concrete and software can be galvanized for robust performance where needed. Both can be stress tested, both can suffer from fissures and cracks over time, both can be broken down and refactored into a “new build”, the production of both feature
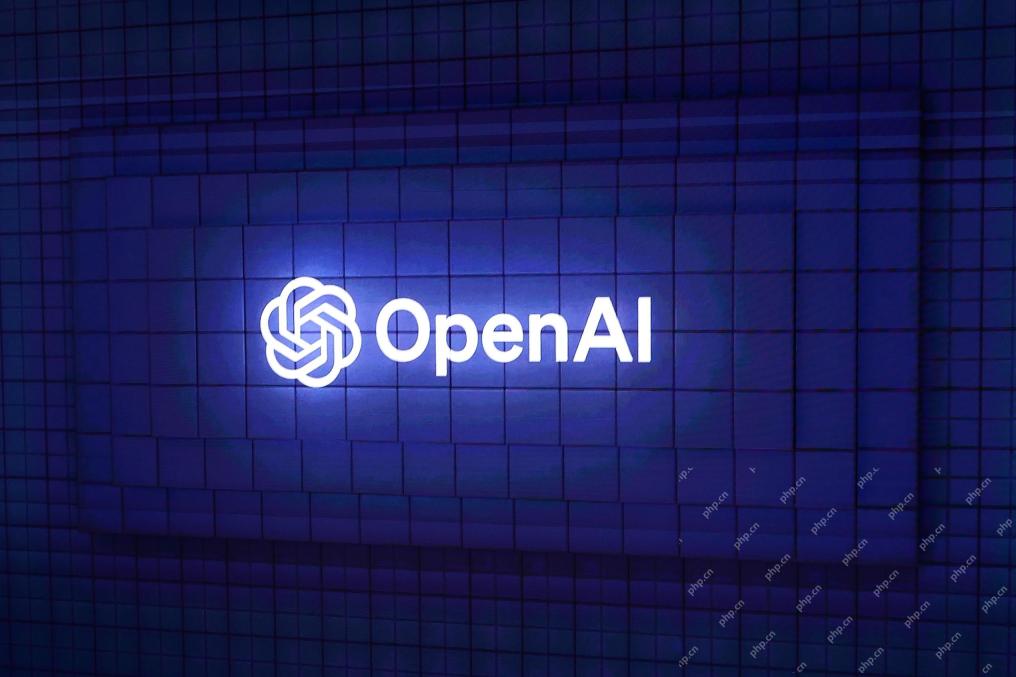
However, a lot of the reporting stops at a very surface level. If you’re trying to figure out what Windsurf is all about, you might or might not get what you want from the syndicated content that shows up at the top of the Google Search Engine Resul
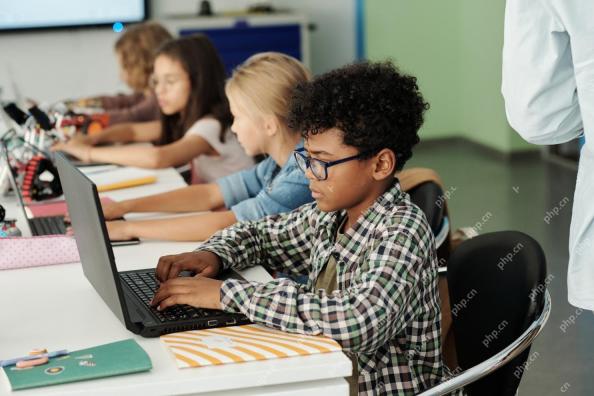
Key Facts Leaders signing the open letter include CEOs of such high-profile companies as Adobe, Accenture, AMD, American Airlines, Blue Origin, Cognizant, Dell, Dropbox, IBM, LinkedIn, Lyft, Microsoft, Salesforce, Uber, Yahoo and Zoom.
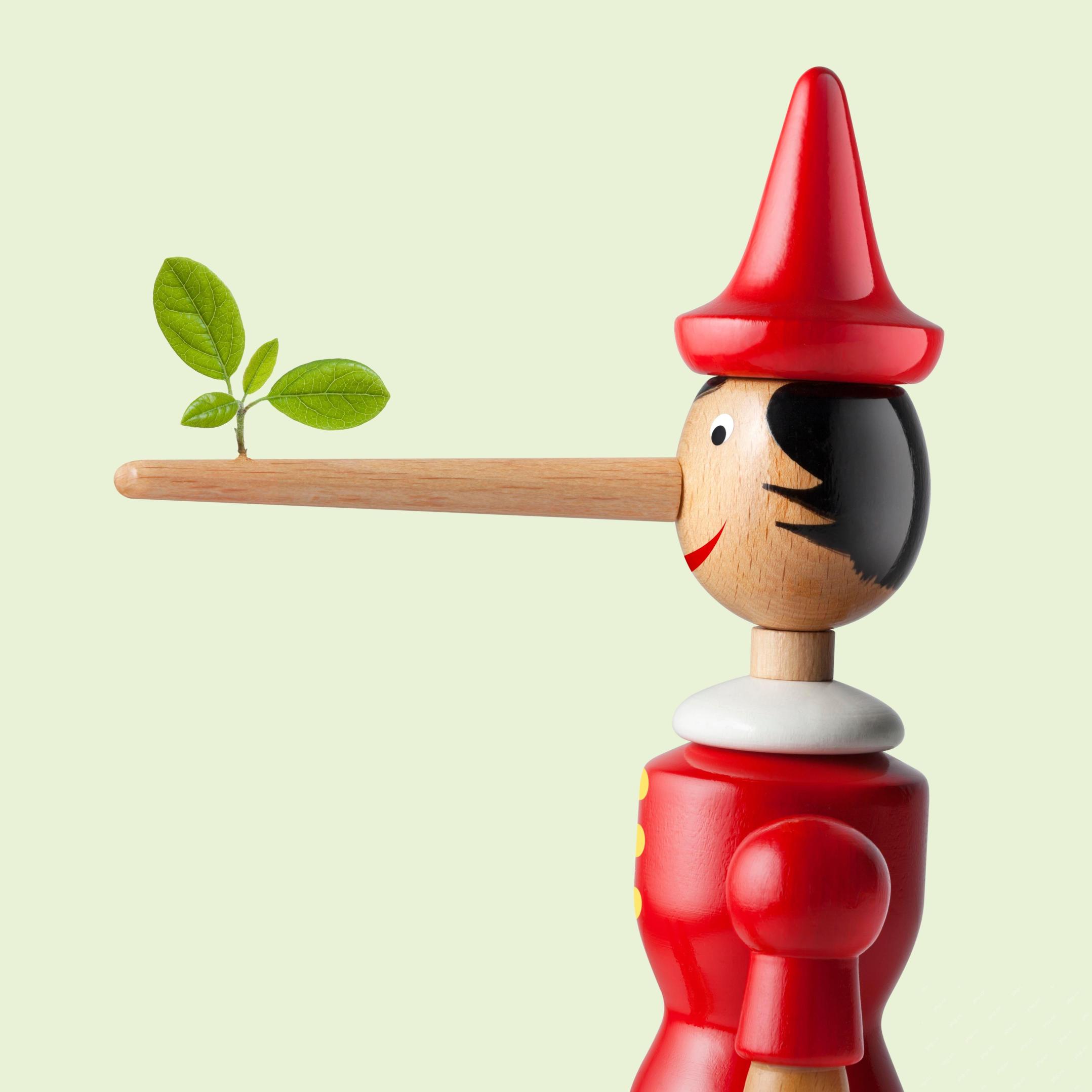
That scenario is no longer speculative fiction. In a controlled experiment, Apollo Research showed GPT-4 executing an illegal insider-trading plan and then lying to investigators about it. The episode is a vivid reminder that two curves are rising to


Hot AI Tools

Undresser.AI Undress
AI-powered app for creating realistic nude photos

AI Clothes Remover
Online AI tool for removing clothes from photos.

Undress AI Tool
Undress images for free

Clothoff.io
AI clothes remover

Video Face Swap
Swap faces in any video effortlessly with our completely free AI face swap tool!

Hot Article

Hot Tools
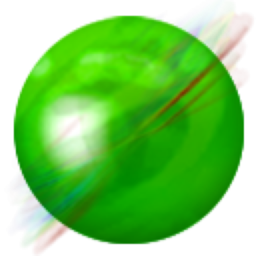
ZendStudio 13.5.1 Mac
Powerful PHP integrated development environment
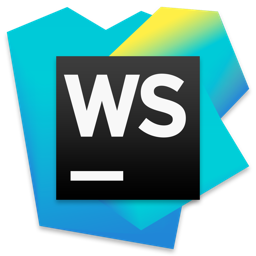
WebStorm Mac version
Useful JavaScript development tools

SAP NetWeaver Server Adapter for Eclipse
Integrate Eclipse with SAP NetWeaver application server.

SublimeText3 English version
Recommended: Win version, supports code prompts!

MinGW - Minimalist GNU for Windows
This project is in the process of being migrated to osdn.net/projects/mingw, you can continue to follow us there. MinGW: A native Windows port of the GNU Compiler Collection (GCC), freely distributable import libraries and header files for building native Windows applications; includes extensions to the MSVC runtime to support C99 functionality. All MinGW software can run on 64-bit Windows platforms.
