Three major challenges of artificial intelligence voice technology
Artificial intelligence practitioners often encounter three common obstacles when it comes to speech-to-speech technology.
The prospect of artificial intelligence (AI) being able to generate human-like data has been talked about for decades. However, data scientists have tackled this problem with limited success. Precisely identifying effective strategies for creating such systems poses challenges ranging from technical to ethical and everything in between. However, generative AI has emerged as a bright spot to watch.
At its most basic, generative AI enables machines to generate content from speech to writing to art using elements such as audio files, text and images. Technology investment firm Sequoia Capita said: "Generative AI will not only become faster and cheaper, but in some cases will be better than artificial intelligence created by humans."
Especially based on generative Recent advances in machine learning technology for speech have made huge strides, but we still have a long way to go. In fact, voice compression appears in apps that people rely on heavily, like Zoom and Teams, which are still based on technology from the 1980s and 1990s. While speech has unlimited potential for speech technology, it is critical to assess the challenges and shortcomings that stand in the way of generative AI development.
Here are three common obstacles that AI practitioners face when it comes to speech-to-speech technology.
1. Sound Quality
Arguably the most important part of the best dialogue is that it is understandable. In the case of speech-to-speech technology, the goal is to sound like a human. For example, Siri and Alexa's robotic intonations are machine-like and not always clear. This is difficult to achieve with artificial intelligence for several reasons, but the nuances of human language play a big role.
Merabian's Law can help explain this. Human conversation can be divided into three parts: 55% facial expressions, 38% tone of voice, and only 7% text. Machine understanding relies on words or content to operate. Only recent advances in natural language processing (NLP) have made it possible to train AI models based on mood, emotion, timbre, and other important (but not necessarily spoken) aspects of language. It's even more challenging if you're only dealing with audio, not vision, because not more than half of the understanding comes from facial expressions.
2. Latency
Comprehensive AI analysis may take time, but in voice-to-voice communications, real-time is the only time that matters. Speech conversion must occur immediately when speaking. It also has to be accurate, which as you can imagine is no easy task for a machine.
The necessity of real-time varies by industry. For example, a content creator doing podcasts might be more concerned with sound quality than real-time voice conversion. But in an industry like customer service, time is of the essence. If call center agents use voice-assisted AI to respond to callers, they may make some sacrifices in quality. Still, time is of the essence in delivering a positive experience.
3. Scale
For speech-to-speech technology to reach its potential, it must support a variety of accents, languages, and dialects and be available to everyone—not just specific ones region or market. This requires mastering the specific application of the technology and doing a lot of tuning and training in order to scale effectively.
Emerging technology solutions are not one-size-fits-all; for a given solution, all users will need thousands of architectures to support this AI infrastructure. Users should also expect consistent testing of models. This is not new: all the classic challenges of machine learning also apply to the field of generative AI.
So how do people start to solve these problems so they start to realize the value of speech to speech technology? Fortunately, when you break it down step by step, it's less scary. First, you must master the problem. Earlier I gave the example of a call center and a content creator. Make sure you think about the use cases and desired outcomes and go from there.
Second, make sure your organization has the right architecture and algorithms. But before that happens, make sure your business has the right data. Data quality is important, especially when considering something as sensitive as human language and speech. Finally, if your application requires real-time speech conversion, make sure that feature is supported. Ultimately, no one wants to talk to a robot.
While ethical concerns about generating AI deepfakes, consent, and appropriate disclosure are now emerging, it is important to first understand and address the fundamental issues. Voice-to-speech technology has the potential to revolutionize the way we understand each other, creating opportunities for innovation that brings people together. But in order to achieve this goal, major challenges must first be faced. ?
The above is the detailed content of Three major challenges of artificial intelligence voice technology. For more information, please follow other related articles on the PHP Chinese website!
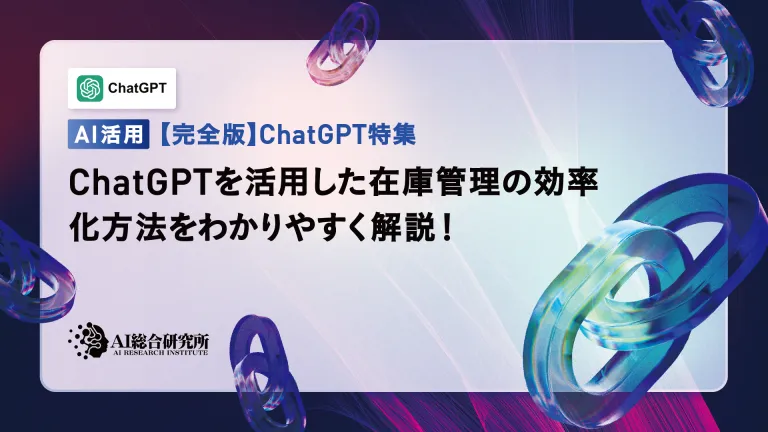
Easy to implement even for small and medium-sized businesses! Smart inventory management with ChatGPT and Excel Inventory management is the lifeblood of your business. Overstocking and out-of-stock items have a serious impact on cash flow and customer satisfaction. However, the current situation is that introducing a full-scale inventory management system is high in terms of cost. What you'd like to focus on is the combination of ChatGPT and Excel. In this article, we will explain step by step how to streamline inventory management using this simple method. Automate tasks such as data analysis, demand forecasting, and reporting to dramatically improve operational efficiency. moreover,
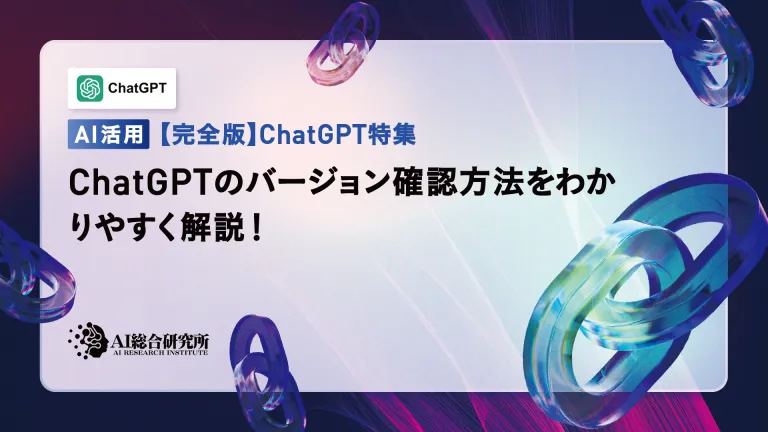
Use AI wisely by choosing a ChatGPT version! A thorough explanation of the latest information and how to check ChatGPT is an ever-evolving AI tool, but its features and performance vary greatly depending on the version. In this article, we will explain in an easy-to-understand manner the features of each version of ChatGPT, how to check the latest version, and the differences between the free version and the paid version. Choose the best version and make the most of your AI potential. Click here for more information about OpenAI's latest AI agent, OpenAI Deep Research ⬇️ [ChatGPT] OpenAI D
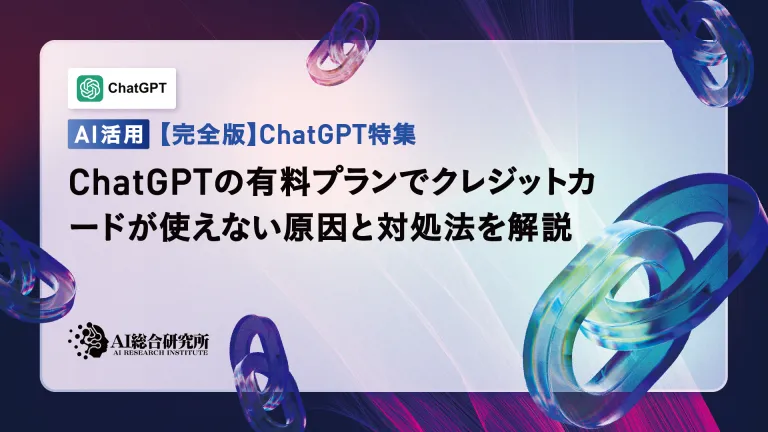
Troubleshooting Guide for Credit Card Payment with ChatGPT Paid Subscriptions Credit card payments may be problematic when using ChatGPT paid subscription. This article will discuss the reasons for credit card rejection and the corresponding solutions, from problems solved by users themselves to the situation where they need to contact a credit card company, and provide detailed guides to help you successfully use ChatGPT paid subscription. OpenAI's latest AI agent, please click ⬇️ for details of "OpenAI Deep Research" 【ChatGPT】Detailed explanation of OpenAI Deep Research: How to use and charging standards Table of contents Causes of failure in ChatGPT credit card payment Reason 1: Incorrect input of credit card information Original
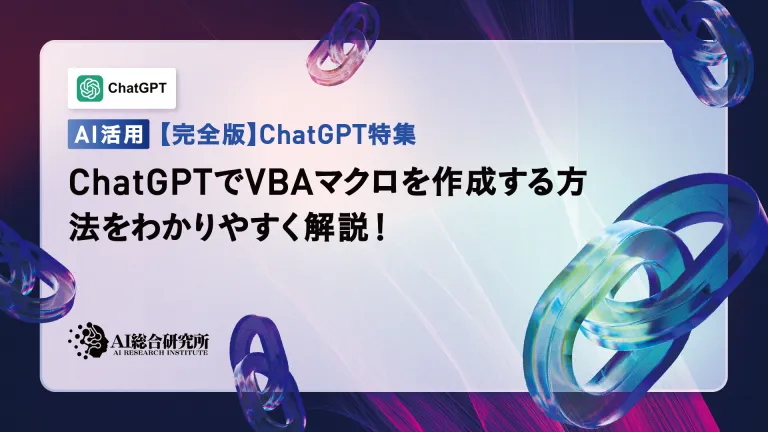
For beginners and those interested in business automation, writing VBA scripts, an extension to Microsoft Office, may find it difficult. However, ChatGPT makes it easy to streamline and automate business processes. This article explains in an easy-to-understand manner how to develop VBA scripts using ChatGPT. We will introduce in detail specific examples, from the basics of VBA to script implementation using ChatGPT integration, testing and debugging, and benefits and points to note. With the aim of improving programming skills and improving business efficiency,
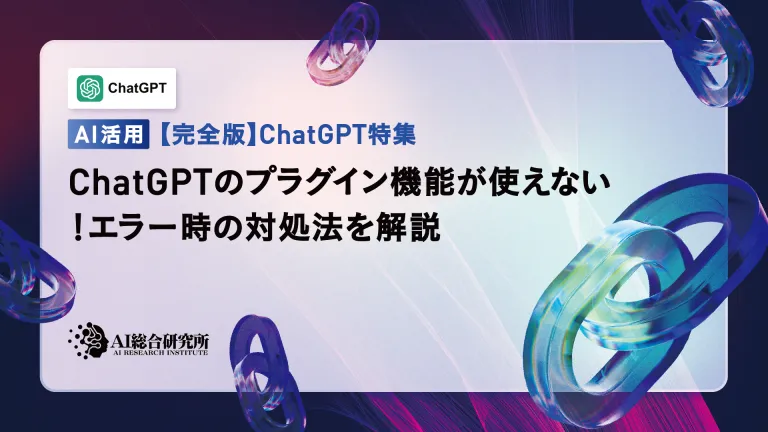
ChatGPT plugin cannot be used? This guide will help you solve your problem! Have you ever encountered a situation where the ChatGPT plugin is unavailable or suddenly fails? The ChatGPT plugin is a powerful tool to enhance the user experience, but sometimes it can fail. This article will analyze in detail the reasons why the ChatGPT plug-in cannot work properly and provide corresponding solutions. From user setup checks to server troubleshooting, we cover a variety of troubleshooting solutions to help you efficiently use plug-ins to complete daily tasks. OpenAI Deep Research, the latest AI agent released by OpenAI. For details, please click ⬇️ [ChatGPT] OpenAI Deep Research Detailed explanation:
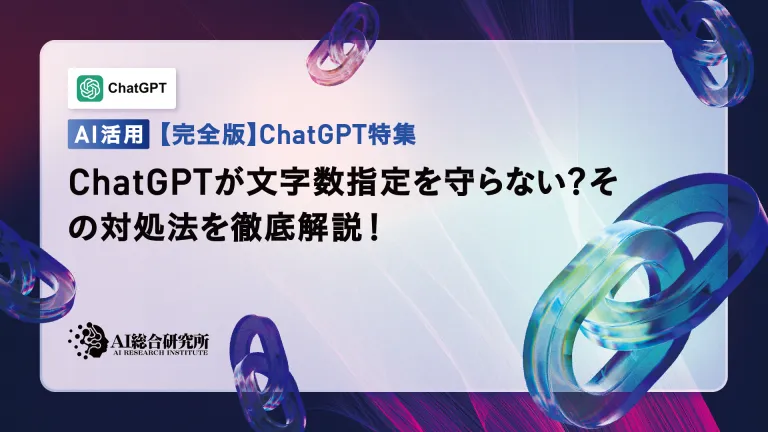
When writing a sentence using ChatGPT, there are times when you want to specify the number of characters. However, it is difficult to accurately predict the length of sentences generated by AI, and it is not easy to match the specified number of characters. In this article, we will explain how to create a sentence with the number of characters in ChatGPT. We will introduce effective prompt writing, techniques for getting answers that suit your purpose, and teach you tips for dealing with character limits. In addition, we will explain why ChatGPT is not good at specifying the number of characters and how it works, as well as points to be careful about and countermeasures. This article
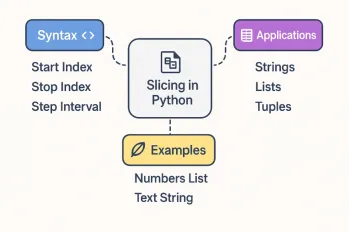
For every Python programmer, whether in the domain of data science and machine learning or software development, Python slicing operations are one of the most efficient, versatile, and powerful operations. Python slicing syntax a
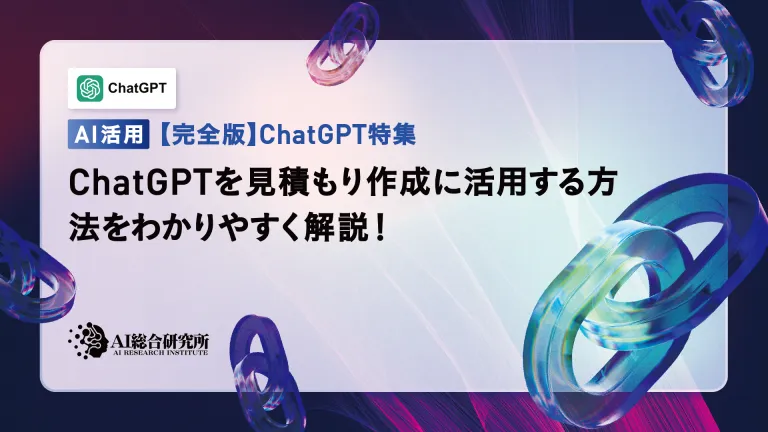
The evolution of AI technology has accelerated business efficiency. What's particularly attracting attention is the creation of estimates using AI. OpenAI's AI assistant, ChatGPT, contributes to improving the estimate creation process and improving accuracy. This article explains how to create a quote using ChatGPT. We will introduce efficiency improvements through collaboration with Excel VBA, specific examples of application to system development projects, benefits of AI implementation, and future prospects. Learn how to improve operational efficiency and productivity with ChatGPT. Op


Hot AI Tools

Undresser.AI Undress
AI-powered app for creating realistic nude photos

AI Clothes Remover
Online AI tool for removing clothes from photos.

Undress AI Tool
Undress images for free

Clothoff.io
AI clothes remover

Video Face Swap
Swap faces in any video effortlessly with our completely free AI face swap tool!

Hot Article

Hot Tools

mPDF
mPDF is a PHP library that can generate PDF files from UTF-8 encoded HTML. The original author, Ian Back, wrote mPDF to output PDF files "on the fly" from his website and handle different languages. It is slower than original scripts like HTML2FPDF and produces larger files when using Unicode fonts, but supports CSS styles etc. and has a lot of enhancements. Supports almost all languages, including RTL (Arabic and Hebrew) and CJK (Chinese, Japanese and Korean). Supports nested block-level elements (such as P, DIV),

SublimeText3 Chinese version
Chinese version, very easy to use
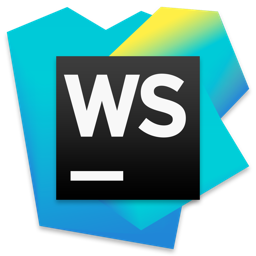
WebStorm Mac version
Useful JavaScript development tools

Zend Studio 13.0.1
Powerful PHP integrated development environment
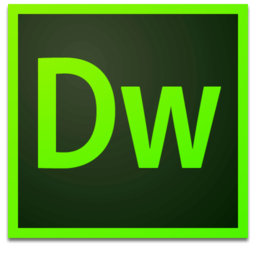
Dreamweaver Mac version
Visual web development tools
