


In the past two years, the Hazy Research Laboratory at Stanford University has been engaged in an important task: Increasing sequence length .
They have a view: longer sequences will usher in a new era of basic machine learning models - models that can learn from longer contexts and multiple media sources , complex demonstrations, etc. to learn.Currently, this research has made new progress. Tri Dao and Dan Fu from the Hazy Research laboratory led the research and promotion of the FlashAttention algorithm. They proved that a sequence length of 32k is possible and will be widely used in the current basic model era (OpenAI, Microsoft, NVIDIA and other companies models are using the FlashAttention algorithm).
- ##Paper address: https://arxiv.org/abs/2205.14135
- Code address: https://github.com/HazyResearch/flash-attention
- As the relevant information of GPT4 points out, it Allows nearly 50 pages of text as context, and implements tokenization/patching like Deepmind Gato uses images as context.
In this article, the author introduces new methods for increasing sequence length at a high level and provides a "bridge" to a new set of primitives.
In the Hazy Research lab, this work started with Hippo, then S4, H3, and now Hyena. These models have the potential to handle context lengths in the millions or even billions. FlashAttention speeds up attention and reduces its memory footprint — without any approximation. “Since we released FlashAttention 6 months ago, we’re excited to see many organizations and research labs adopting FlashAttention to accelerate their training and inference,” the blog post reads.
FlashAttention is a method that reorders attention calculations and leverages classic techniques (tiling, recalculation) to speed up and reduce memory usage from quadratic to linear in sequence length algorithm. For each attention head, to reduce memory reads/writes, FlashAttention uses classic tiling techniques to load query, key and value blocks from the GPU HBM (its main memory) to SRAM (its fast cache), computing attention and write the output back to HBM. This reduction in memory reads/writes results in significant speedups (2-4x) in most cases.
Long Range Arena Benchmark and S4
Researchers at Google launched the Long Range Arena (LRA) benchmark in 2020 to evaluate how well different models handle long-range dependencies. LRA is capable of testing a range of tasks covering many different data types and modalities, such as text, images and mathematical expressions, with sequence lengths up to 16K (Path-X: Classification of images unfolded into pixels without any spatial generalization bias). There has been a lot of great work on scaling Transformers to longer sequences, but a lot of it seems to sacrifice accuracy (as shown in the image below). Note the Path-X column: all Transformer methods and their variants perform even worse than random guessing.
#Now let us get to know the S4, which was developed by Albert Gu. Inspired by the LRA benchmark results, Albert Gu wanted to find out how to better model long-range dependencies. Based on long-term research on the relationship between orthogonal polynomials and recursive models and convolutional models, he launched S4—— A new sequence model based on structured state space models (SSMs).
The key point is that the time complexity of SSM when extending a sequence of length N to 2N is , unlike attention The mechanism also grows at a square level! S4 successfully models long-range dependencies in LRA and becomes the first model to achieve above-average performance on Path-X (now achieving 96.4% accuracy!). Since the release of S4, many researchers have developed and innovated on this basis, with new models such as the S5 model of Scott Linderman's team, Ankit Gupta's DSS (and the subsequent S4D of Hazy Research laboratory), Hasani and Lechner's Liquid-S4, etc. Model.
In addition, when Hazy Research released FlashAttention, it was already possible to increase the sequence length of Transformer. They also found that Transformer also achieved superior performance on Path-X (63%) simply by increasing the sequence length to 16K.
Shortcomings in modeling
But there is a gap in the quality of S4 in language modeling as high as 5% perplexity (for context, this is 125M models and 6.7B gap between models). To close this gap, researchers have studied synthetic languages such as associative recall to determine what properties a language should possess. The final design was H3 (Hungry Hungry Hippos): a new layer that stacks two SSMs and multiplies their outputs with a multiplication gate.
Using H3, researchers at Hazy Research replaced almost all attention layers in the GPT-style Transformer and were able to achieve high performance in perplexity and Comparable to transformer in terms of downstream evaluation.
##Since the H3 layer is built on SSM, its computational complexity is also high in terms of sequence length. Growing at the rate of . The two attention layers make the complexity of the entire model still
##. This issue will be discussed in detail later.
Of course, Hazy Research is not the only one considering this direction: GSS also found that SSM with gating can work well with attention in language modeling (which inspired H3), Meta released The Mega model, which also combines SSM and attention, the BiGS model replaces the attention in the BERT-style model, and RWKV has been working on a fully looped approach.
New progress: Hyena
Based on a series of previous work, researchers at Hazy Research were inspired to develop a new architecture: Hyena. They tried to get rid of the last two attention layers in H3 and obtain a model that grows almost linearly for longer sequence lengths. It turns out that two simple ideas are the key to finding the answer:
- #Each SSM can be viewed as a convolutional filter with the same length as the input sequence. Therefore, the SSM can be replaced with a convolution of size equal to the input sequence to obtain a more powerful model with the same computational effort. Specifically, the convolutional filter is implicitly parameterized via another small neural network, drawing on powerful methods from the neural field literature and work on CKConv/FlexConv. In addition, the convolution can be calculated in O (NlogN) time, where N is the sequence length, achieving nearly linear scaling; the gating behavior in
- #H3 can be summarized as: H3 takes three projections of the input and iteratively convolves and applies gating. In Hyena, simply adding more projections and more gates helps generalize to more expressive architectures and close the gap with attention.
Hyena proposed a completely near-linear temporal convolution model for the first time, which can match Transformer in perplexity and downstream tasks, and has achieved good results in experiments. result. And small and medium-sized models were trained on a subset of PILE, and their performance was comparable to Transformer:
With some optimizations (more on that below), the Hyena model is slightly slower than a Transformer of the same size at a sequence length of 2K, but is faster at longer sequence lengths.
The next thing that still needs to be considered is to what extent can these models be generalized? Is it possible to scale them to the full size of PILE (400B tokens)? What would happen if you combined the best of H3 and Hyena’s ideas, and how far could it go?
FFT or a more basic approach?
A common basic operation in all these models is FFT, which is an efficient way to calculate convolution and only takes O (NlogN) time. However, FFT is poorly supported on modern hardware where the dominant architecture is dedicated matrix multiplication units and GEMMs (e.g. tensor cores on NVIDIA GPUs).
The efficiency gap can be closed by rewriting the FFT as a series of matrix multiplication operations. Members of the research team achieved this goal by using butterfly matrices to explore sparse training. Recently, Hazy Research researchers have leveraged this connection to build fast convolution algorithms such as FlashConv and FlashButterfly, by using butterfly decomposition to transform FFT calculations into a series of matrix multiplication operations.
Additionally, deeper connections can be made by drawing on previous work: including letting these matrices be learned, which also takes the same time but adds additional parameters. Researchers have begun to explore this connection on some small data sets and have achieved initial results. We can clearly see what this connection can lead to (e.g. how to make it suitable for language models):
This extension deserves a deeper exploration: what kind of transformation does this extension learn, and what does it allow you to do? What happens when you apply it to language modeling?
These are exciting directions, and what will follow will be longer and longer sequences and new architectures that will allow us to further explore this new area. We need to pay special attention to applications that can benefit from long sequence models, such as high-resolution imaging, new data formats, language models that can read entire books, etc. Imagine giving an entire book to a language model to read and having it summarize the storyline, or having a code generation model generate new code based on the code you wrote. There are so many possible scenarios, and they are all very exciting.
The above is the detailed content of Want to move half of 'A Dream of Red Mansions' into the ChatGPT input box? Let's solve this problem first. For more information, please follow other related articles on the PHP Chinese website!
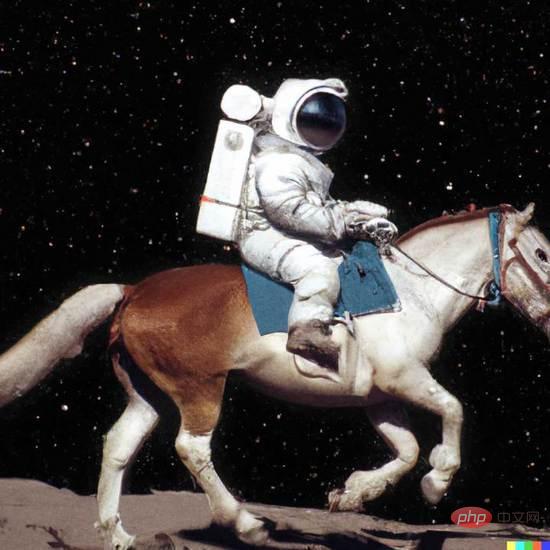
1 前言在发布DALL·E的15个月后,OpenAI在今年春天带了续作DALL·E 2,以其更加惊艳的效果和丰富的可玩性迅速占领了各大AI社区的头条。近年来,随着生成对抗网络(GAN)、变分自编码器(VAE)、扩散模型(Diffusion models)的出现,深度学习已向世人展现其强大的图像生成能力;加上GPT-3、BERT等NLP模型的成功,人类正逐步打破文本和图像的信息界限。在DALL·E 2中,只需输入简单的文本(prompt),它就可以生成多张1024*1024的高清图像。这些图像甚至
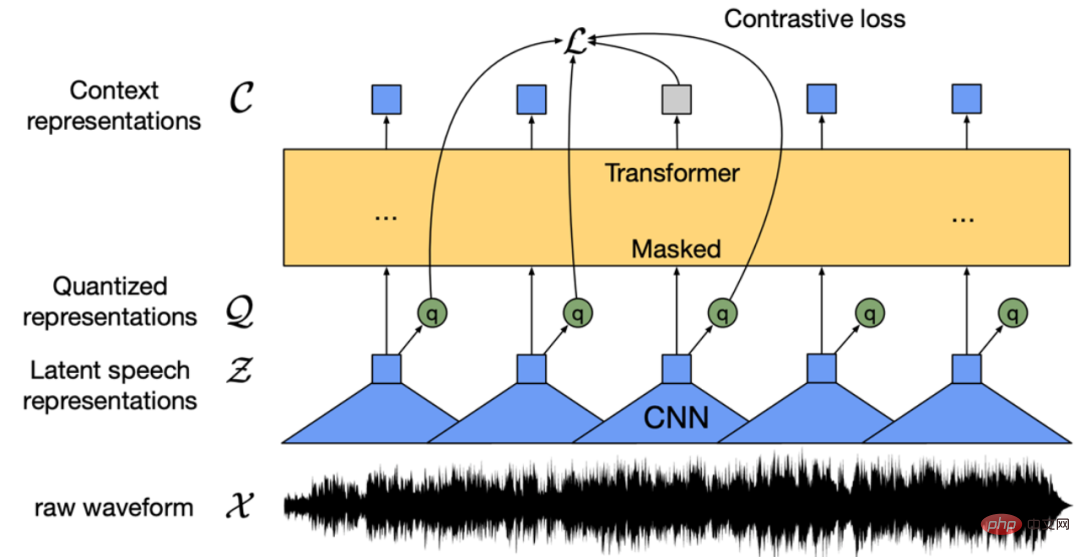
Wav2vec 2.0 [1],HuBERT [2] 和 WavLM [3] 等语音预训练模型,通过在多达上万小时的无标注语音数据(如 Libri-light )上的自监督学习,显著提升了自动语音识别(Automatic Speech Recognition, ASR),语音合成(Text-to-speech, TTS)和语音转换(Voice Conversation,VC)等语音下游任务的性能。然而这些模型都没有公开的中文版本,不便于应用在中文语音研究场景。 WenetSpeech [4] 是
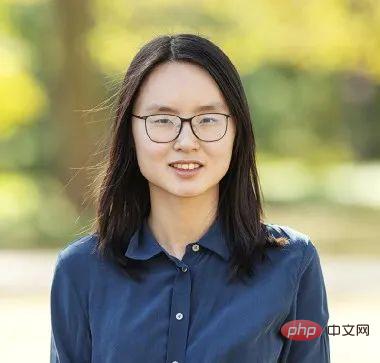
“Making large models smaller”这是很多语言模型研究人员的学术追求,针对大模型昂贵的环境和训练成本,陈丹琦在智源大会青源学术年会上做了题为“Making large models smaller”的特邀报告。报告中重点提及了基于记忆增强的TRIME算法和基于粗细粒度联合剪枝和逐层蒸馏的CofiPruning算法。前者能够在不改变模型结构的基础上兼顾语言模型困惑度和检索速度方面的优势;而后者可以在保证下游任务准确度的同时实现更快的处理速度,具有更小的模型结构。陈丹琦 普
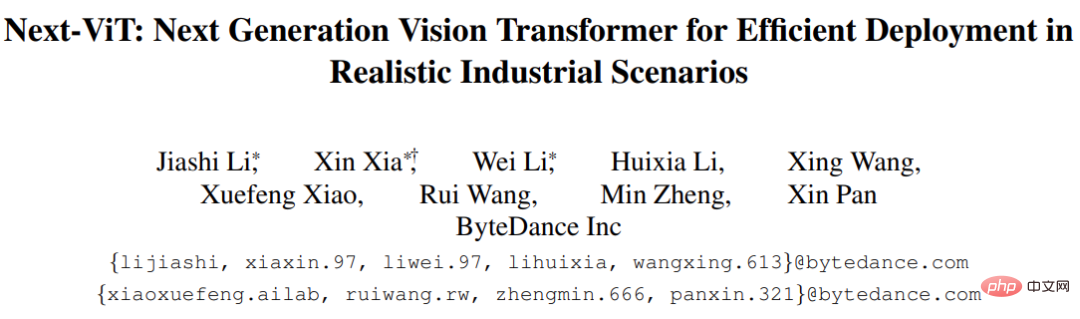
由于复杂的注意力机制和模型设计,大多数现有的视觉 Transformer(ViT)在现实的工业部署场景中不能像卷积神经网络(CNN)那样高效地执行。这就带来了一个问题:视觉神经网络能否像 CNN 一样快速推断并像 ViT 一样强大?近期一些工作试图设计 CNN-Transformer 混合架构来解决这个问题,但这些工作的整体性能远不能令人满意。基于此,来自字节跳动的研究者提出了一种能在现实工业场景中有效部署的下一代视觉 Transformer——Next-ViT。从延迟 / 准确性权衡的角度看,
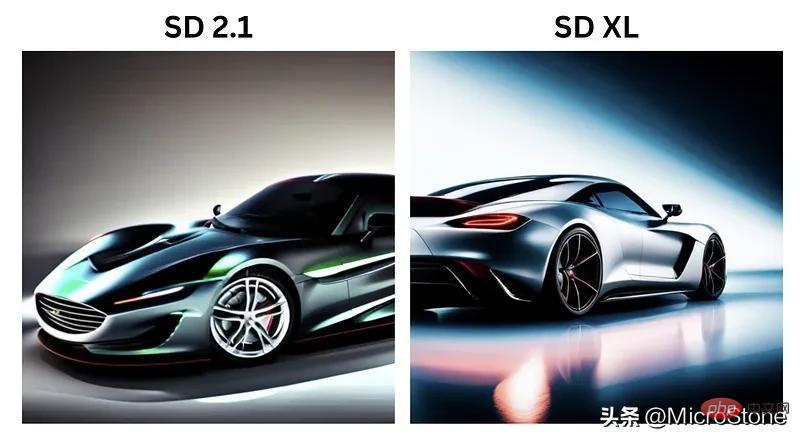
3月27号,Stability AI的创始人兼首席执行官Emad Mostaque在一条推文中宣布,Stable Diffusion XL 现已可用于公开测试。以下是一些事项:“XL”不是这个新的AI模型的官方名称。一旦发布稳定性AI公司的官方公告,名称将会更改。与先前版本相比,图像质量有所提高与先前版本相比,图像生成速度大大加快。示例图像让我们看看新旧AI模型在结果上的差异。Prompt: Luxury sports car with aerodynamic curves, shot in a
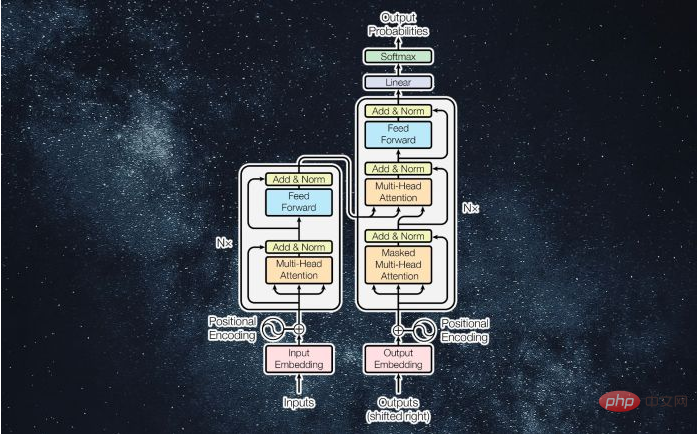
译者 | 李睿审校 | 孙淑娟近年来, Transformer 机器学习模型已经成为深度学习和深度神经网络技术进步的主要亮点之一。它主要用于自然语言处理中的高级应用。谷歌正在使用它来增强其搜索引擎结果。OpenAI 使用 Transformer 创建了著名的 GPT-2和 GPT-3模型。自从2017年首次亮相以来,Transformer 架构不断发展并扩展到多种不同的变体,从语言任务扩展到其他领域。它们已被用于时间序列预测。它们是 DeepMind 的蛋白质结构预测模型 AlphaFold
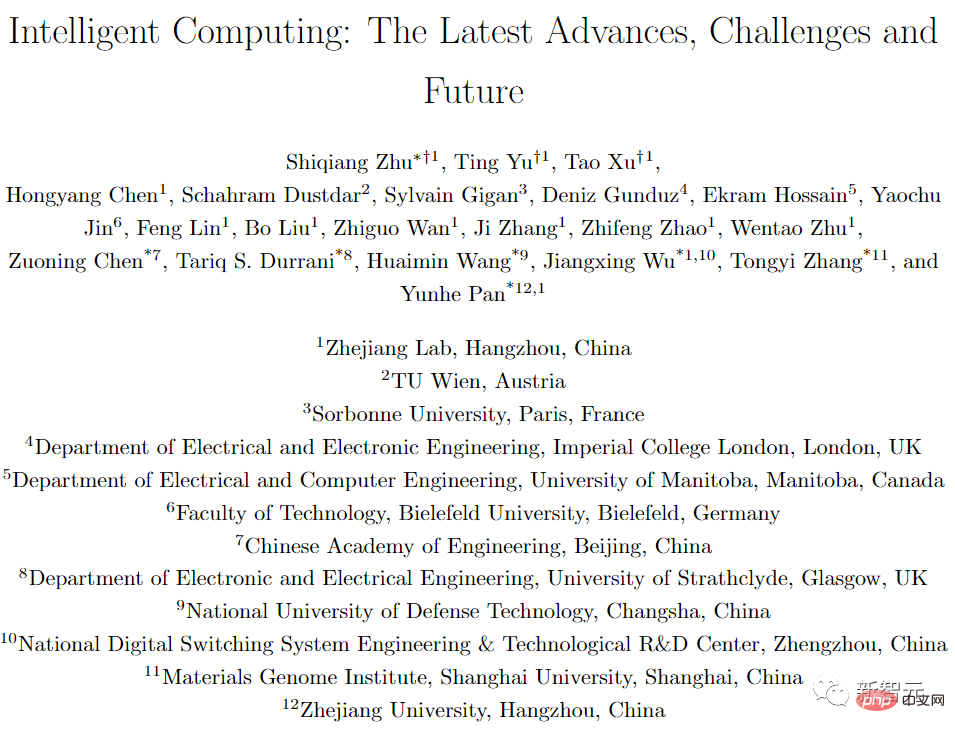
人工智能就是一个「拼财力」的行业,如果没有高性能计算设备,别说开发基础模型,就连微调模型都做不到。但如果只靠拼硬件,单靠当前计算性能的发展速度,迟早有一天无法满足日益膨胀的需求,所以还需要配套的软件来协调统筹计算能力,这时候就需要用到「智能计算」技术。最近,来自之江实验室、中国工程院、国防科技大学、浙江大学等多达十二个国内外研究机构共同发表了一篇论文,首次对智能计算领域进行了全面的调研,涵盖了理论基础、智能与计算的技术融合、重要应用、挑战和未来前景。论文链接:https://spj.scien
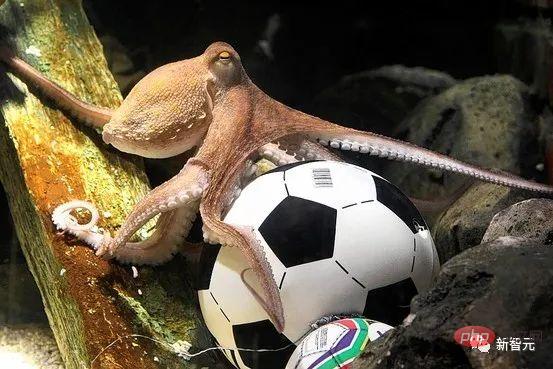
说起2010年南非世界杯的最大网红,一定非「章鱼保罗」莫属!这只位于德国海洋生物中心的神奇章鱼,不仅成功预测了德国队全部七场比赛的结果,还顺利地选出了最终的总冠军西班牙队。不幸的是,保罗已经永远地离开了我们,但它的「遗产」却在人们预测足球比赛结果的尝试中持续存在。在艾伦图灵研究所(The Alan Turing Institute),随着2022年卡塔尔世界杯的持续进行,三位研究员Nick Barlow、Jack Roberts和Ryan Chan决定用一种AI算法预测今年的冠军归属。预测模型图


Hot AI Tools

Undresser.AI Undress
AI-powered app for creating realistic nude photos

AI Clothes Remover
Online AI tool for removing clothes from photos.

Undress AI Tool
Undress images for free

Clothoff.io
AI clothes remover

AI Hentai Generator
Generate AI Hentai for free.

Hot Article

Hot Tools
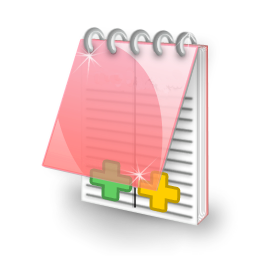
EditPlus Chinese cracked version
Small size, syntax highlighting, does not support code prompt function
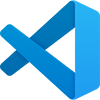
VSCode Windows 64-bit Download
A free and powerful IDE editor launched by Microsoft
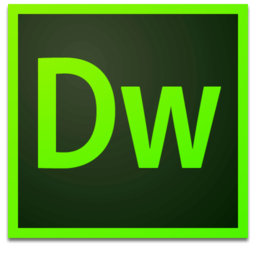
Dreamweaver Mac version
Visual web development tools

MinGW - Minimalist GNU for Windows
This project is in the process of being migrated to osdn.net/projects/mingw, you can continue to follow us there. MinGW: A native Windows port of the GNU Compiler Collection (GCC), freely distributable import libraries and header files for building native Windows applications; includes extensions to the MSVC runtime to support C99 functionality. All MinGW software can run on 64-bit Windows platforms.

PhpStorm Mac version
The latest (2018.2.1) professional PHP integrated development tool