Andrew Brosnan, principal analyst at research firm Omdia, predicts that although the healthcare industry is initially slow to embrace artificial intelligence, healthcare and pharmaceutical companies will increase it rapidly in the next few years. Adoption of artificial intelligence, medical image analysis and drug discovery will be the most popular use cases.
Healthcare spending on AI software is expected to grow 40% in 2023, from $4.4 billion in 2022 to nearly $6.2 billion in the new year, according to Omdia forecasts.
"Healthcare will grow faster than most other industries, and according to our forecasts, we expect healthcare to rank second behind consumers in AI spending in 2027," Omdia Artificial Intelligence and Brosnan of the Intelligent Automation practice said.
Healthcare AI adoption will catch up with other industries
Brosnan said healthcare companies have historically been conservative in adopting new technologies because of risks to patient care and privacy, security and regulatory issues. Very high.
Healthcare lags behind other industries in artificial intelligence adoption. According to a 2022 OM Dia survey, while 25% of all industries have expanded AI deployment across multiple business units or functions, only 19% have done so in healthcare.
But this is changing rapidly. Artificial intelligence has proven effective in health care, which has fueled growth in use, he said. For example, artificial intelligence has been used during the epidemic to help health care providers with COVID-19 diagnosis, patient prognosis, and to help researchers understand changes in the spike protein.
“The use of AI during the pandemic and in proof-of-concept projects has increased confidence in the value AI can provide in healthcare,” said Brosnan.
In fact, 96% of healthcare organizations surveyed by Omdia in 2022 said they are confident or very confident that artificial intelligence will lead to positive outcomes, and 67% of respondents said that artificial intelligence will increase The ability to value has increased over the past year.
This will translate into significant investment in artificial intelligence. Spending on AI software will grow at a compound annual growth rate (CAGR) of 29% and reach $13.8 billion in spending in 2027, tied for the fastest-growing sector, according to Omdia.
Top Five Healthcare IT Use Cases
Medical image analysis is the most popular use case of artificial intelligence. With annual growth of 26%, it will retain the largest share of spending, reaching $2.6 billion in AI software spending in 2027.
Meanwhile, Omdia forecasts show that drug discovery will be the fastest-growing use case by 2027, with AI spending reaching $2 billion and a CAGR of 33%.
Other top use cases are virtual assistants such as online chatbots and intelligent document processing, both of which have a CAGR of 27%. AI spending for virtual assistants is expected to reach nearly $1.7 billion in 2027, while intelligent document processing (such as claims processing) is expected to reach $1 billion.
Medical advice – through tools such as clinical decision support – completes the top five use cases with a CAGR of 28% and $900 million in AI spending in 2027.
Innovating Drug Discovery
Brosnan said that artificial intelligence has the potential to speed up the drug discovery and development process and reduce its cost. In 2023, the pharmaceutical industry will continue to advance drug discovery through artificial intelligence.
The traditional drug discovery and development process currently takes approximately $1 billion and 10 years to bring a new drug to market. This involves synthesizing more than 5,000 molecules to advance a candidate into clinical trials, he said.
But with artificial intelligence, drugmakers can reduce the number of molecules they have to physically make by doing production "on the computer," meaning they can do it virtually, he said.
This reduced the number of molecules they had to physically synthesize to 250, which saved money and shortened time to market, Brosnan said. The pipeline of AI-first drug candidates is very strong, with 18 candidates entering clinical trials in 2022. In 2020, that number was zero.
"Early-stage drug discovery takes months or even years," he said.
Emerging technologies could better train healthcare AI models
Federated learning, or group learning, is an emerging technology that will allow healthcare providers to safely use it with patients, Brosnan said. data to better train AI models and will gain greater traction in 2023.
To reduce bias, it is important to train AI models against large data sets. But to do that, many healthcare organizations want to share data so they can build a more comprehensive dataset to train AI models.
Traditionally, they had to move data to a central repository. However, with federated or group learning, the data does not have to move. Instead, he said, the AI model goes to each individual health care facility and is trained on the data. In this way, healthcare providers can maintain the security and governance of their data.
“With federated or swarm learning, the data does not have to leave the source institution, but the AI model moves to the data,” Brosnan said. Federated learning uses a centralized orchestrator, while swarm learning is more distributed and does not use a centralized orchestrator.
This technology is currently undergoing a proof of concept. In 2021, major pharmaceutical company Sanofi invested $180 million in a healthcare-focused federated learning company.
"This is an emerging technology and we will see the rise of it in 2023 and 2024," he said.
The above is the detailed content of Artificial Intelligence Trends in Healthcare in 2023. For more information, please follow other related articles on the PHP Chinese website!
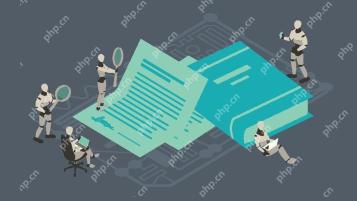
The legal tech revolution is gaining momentum, pushing legal professionals to actively embrace AI solutions. Passive resistance is no longer a viable option for those aiming to stay competitive. Why is Technology Adoption Crucial? Legal professional
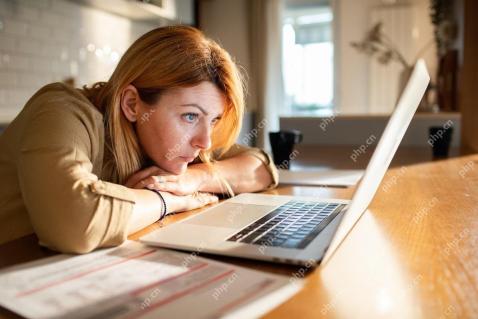
Many assume interactions with AI are anonymous, a stark contrast to human communication. However, AI actively profiles users during every chat. Every prompt, every word, is analyzed and categorized. Let's explore this critical aspect of the AI revo
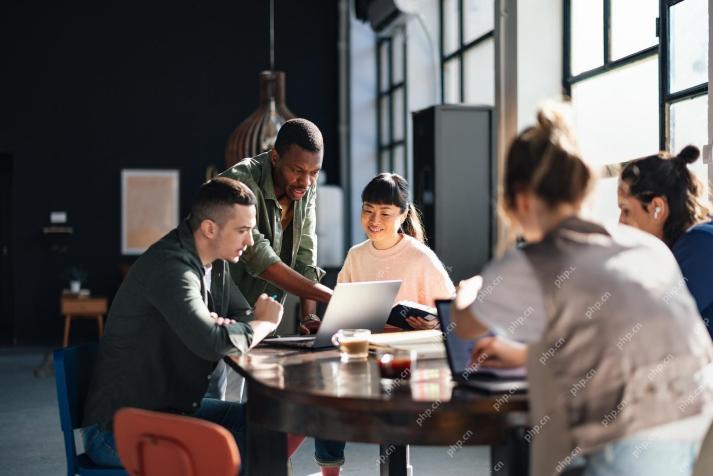
A successful artificial intelligence strategy cannot be separated from strong corporate culture support. As Peter Drucker said, business operations depend on people, and so does the success of artificial intelligence. For organizations that actively embrace artificial intelligence, building a corporate culture that adapts to AI is crucial, and it even determines the success or failure of AI strategies. West Monroe recently released a practical guide to building a thriving AI-friendly corporate culture, and here are some key points: 1. Clarify the success model of AI: First of all, we must have a clear vision of how AI can empower business. An ideal AI operation culture can achieve a natural integration of work processes between humans and AI systems. AI is good at certain tasks, while humans are good at creativity and judgment
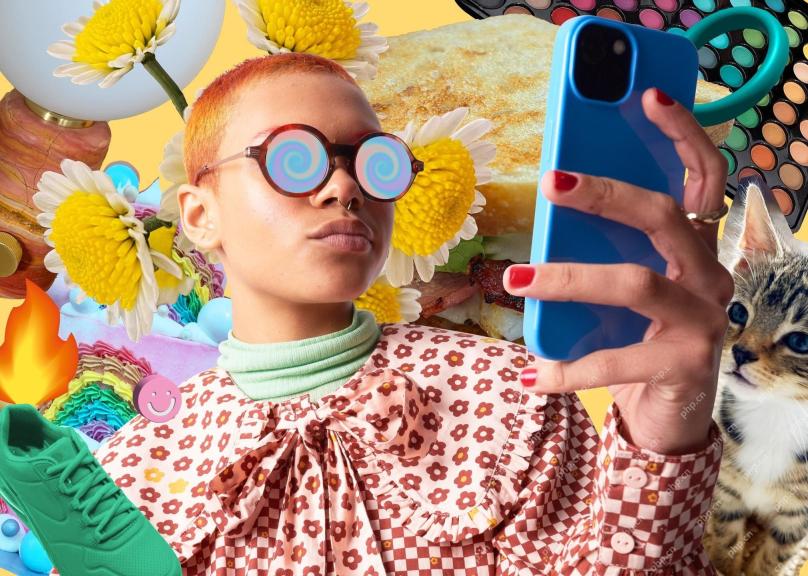
Meta upgrades AI assistant application, and the era of wearable AI is coming! The app, designed to compete with ChatGPT, offers standard AI features such as text, voice interaction, image generation and web search, but has now added geolocation capabilities for the first time. This means that Meta AI knows where you are and what you are viewing when answering your question. It uses your interests, location, profile and activity information to provide the latest situational information that was not possible before. The app also supports real-time translation, which completely changed the AI experience on Ray-Ban glasses and greatly improved its usefulness. The imposition of tariffs on foreign films is a naked exercise of power over the media and culture. If implemented, this will accelerate toward AI and virtual production
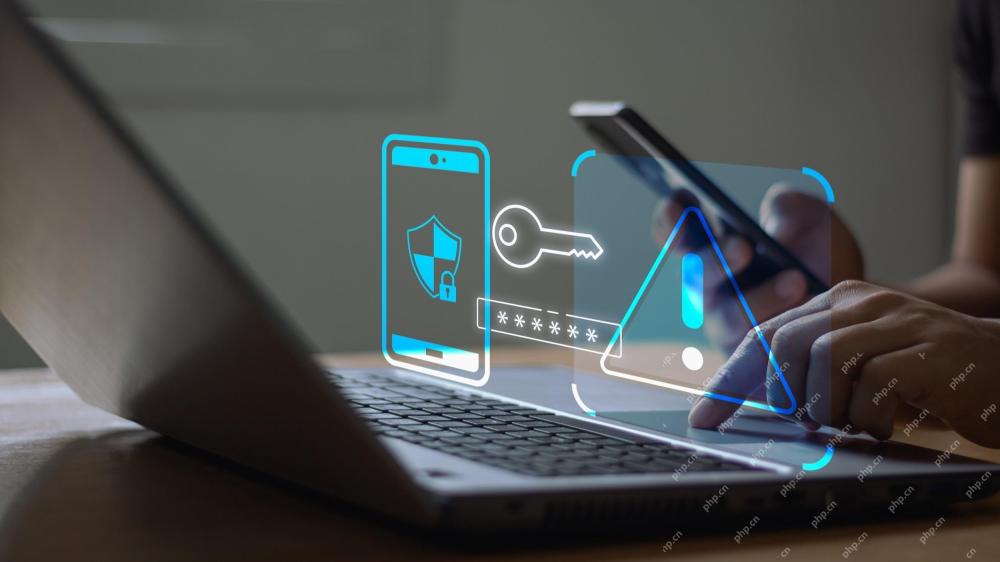
Artificial intelligence is revolutionizing the field of cybercrime, which forces us to learn new defensive skills. Cyber criminals are increasingly using powerful artificial intelligence technologies such as deep forgery and intelligent cyberattacks to fraud and destruction at an unprecedented scale. It is reported that 87% of global businesses have been targeted for AI cybercrime over the past year. So, how can we avoid becoming victims of this wave of smart crimes? Let’s explore how to identify risks and take protective measures at the individual and organizational level. How cybercriminals use artificial intelligence As technology advances, criminals are constantly looking for new ways to attack individuals, businesses and governments. The widespread use of artificial intelligence may be the latest aspect, but its potential harm is unprecedented. In particular, artificial intelligence
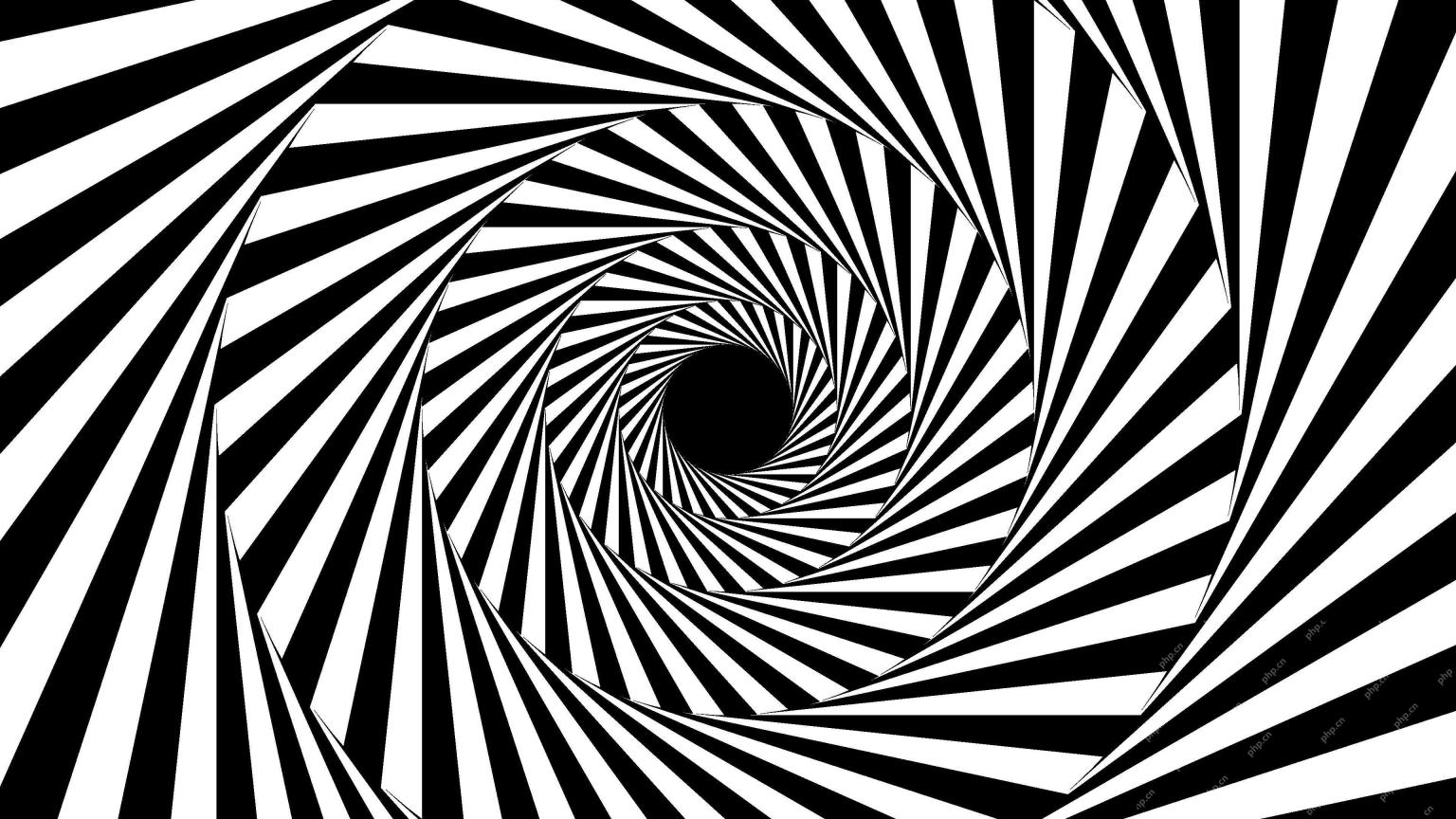
The intricate relationship between artificial intelligence (AI) and human intelligence (NI) is best understood as a feedback loop. Humans create AI, training it on data generated by human activity to enhance or replicate human capabilities. This AI
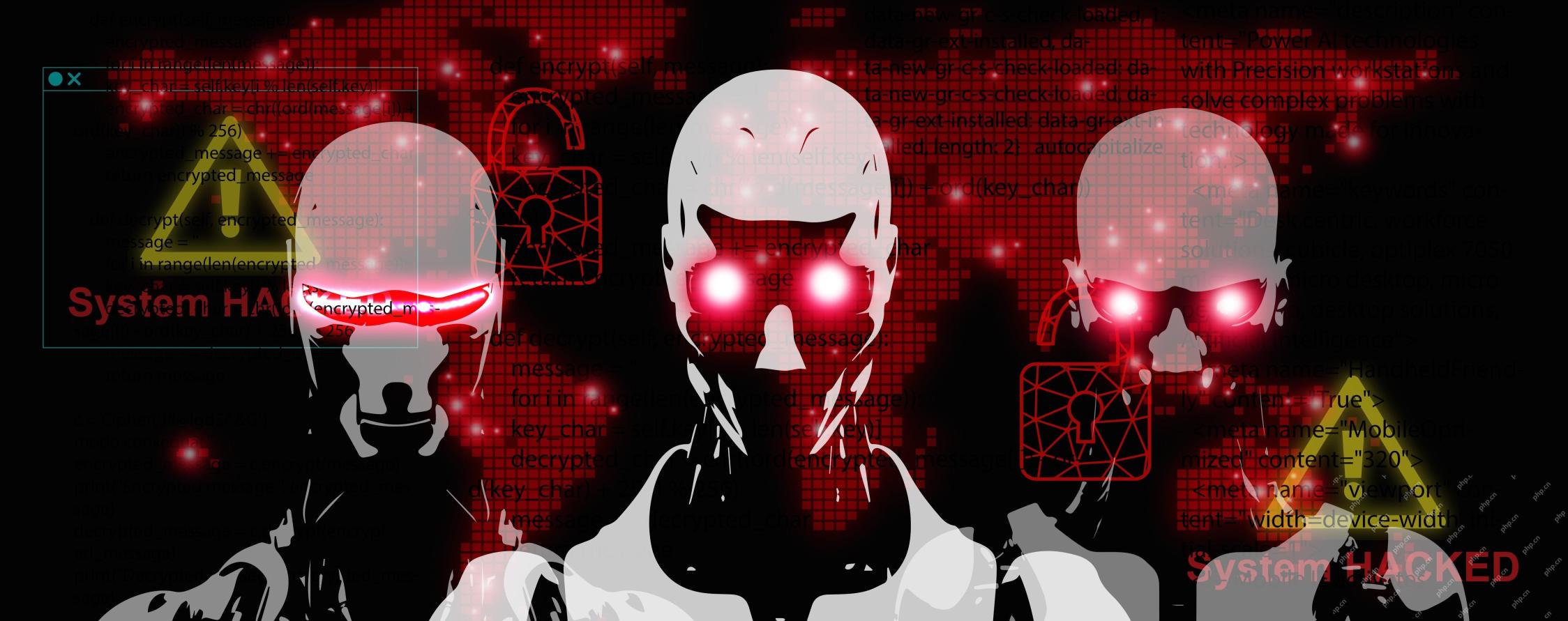
Anthropic's recent statement, highlighting the lack of understanding surrounding cutting-edge AI models, has sparked a heated debate among experts. Is this opacity a genuine technological crisis, or simply a temporary hurdle on the path to more soph
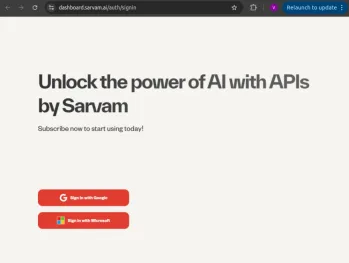
India is a diverse country with a rich tapestry of languages, making seamless communication across regions a persistent challenge. However, Sarvam’s Bulbul-V2 is helping to bridge this gap with its advanced text-to-speech (TTS) t


Hot AI Tools

Undresser.AI Undress
AI-powered app for creating realistic nude photos

AI Clothes Remover
Online AI tool for removing clothes from photos.

Undress AI Tool
Undress images for free

Clothoff.io
AI clothes remover

Video Face Swap
Swap faces in any video effortlessly with our completely free AI face swap tool!

Hot Article

Hot Tools

Safe Exam Browser
Safe Exam Browser is a secure browser environment for taking online exams securely. This software turns any computer into a secure workstation. It controls access to any utility and prevents students from using unauthorized resources.

SublimeText3 Linux new version
SublimeText3 Linux latest version

DVWA
Damn Vulnerable Web App (DVWA) is a PHP/MySQL web application that is very vulnerable. Its main goals are to be an aid for security professionals to test their skills and tools in a legal environment, to help web developers better understand the process of securing web applications, and to help teachers/students teach/learn in a classroom environment Web application security. The goal of DVWA is to practice some of the most common web vulnerabilities through a simple and straightforward interface, with varying degrees of difficulty. Please note that this software

SublimeText3 English version
Recommended: Win version, supports code prompts!
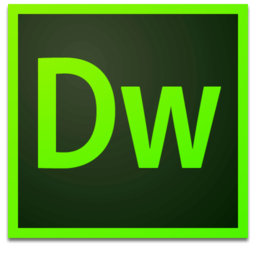
Dreamweaver Mac version
Visual web development tools
