AT&T explores collective practices in data science and automation
I believe everyone has realized that if an organization wants to promote its own transformation through data and technology, then relying on technical experts alone is not enough to achieve the goal. Other “amateurs,” or non-professionals, must also be involved in application development, data analysis, and automated workflows. Many companies have made their own attempts in this kind of exploration, and AT&T is obviously better at exploring the capabilities of all employees and leveraging collective efforts to deal with data science and automation issues.
This idea comes from a cruel fact - no organization has enough professional data scientists and automation experts, and it is unable to complete all the analysis and AI work required for successful transformation. AT&T chief data officer Andy Markus said the telecom giant is working to embed AI and automation into the core of its business. After spinning off WarnerMedia in early 2022, AT&T no longer has entertainment business attributes and has become a pure telecommunications company, with abundant data and talents capable of extracting information insights. Maybe collective exploration isn't right for every company, but it's certainly a good fit for AT&T. In addition to hundreds of professional data scientists and automation experts, AT&T also has many more ordinary developers on staff.
Popularization of Data Science
AT&T has always had a data-centric corporate culture, and the company has been working hard to promote employees to use machine learning models to conduct data analysis. Mark Austin, director of data science, AI and automation at AT&T, said in an interview that the company’s goal is to support all aspects of the machine learning pipeline, including finding suitable data, obtaining data, engineering the data to build the required functions, creating models, Deploy models to production, monitor model performance over time, and effectively implement model management. Like most companies, the first three items account for 80% of a developer's time, and AT&T is working hard to reduce that percentage.
To this end, AT&T has cooperated with H2O.ai, an AI cloud platform service provider, to establish a feature "store" for commonly used source data and derived data, thereby reducing the need for extremely time-consuming data sorting. Data science experts and amateurs alike rave about its effectiveness. Less than a year after the feature store was launched, more than 26,000 features have been opened to professional and amateur data users. In addition, data science models are often matched with Pinnacle projects, an internal competition crowdsourcing scheme similar to Kaggle. Austin explained that this competition mechanism can often improve model performance by nearly 30% on average. The process not only involves a series of crowdsourced autoML solutions, but also incorporates innovative algorithms and integrations from hundreds of AT&T data scientists and engineers.
Popular Popularization of Automation
AT&T began to promote business automation through robotic process automation technology as early as 2015. Since then, the company has put more than 3,000 robots into production environments. After the first year of experimentation, AT&T established its first Automation Center of Excellence (ACoE), which currently employs 20 full-time employees and multiple contractors. But the company’s automation explorations would never have scaled quickly without the active participation of rank-and-file employees. Austin followed up on each project carefully and was surprised to find that up to 92% of RPA implementations occurred outside the center of excellence.
Over time, process automation has created tremendous value for AT&T. Austin said that the flexible combination of robot implementation solutions saves about 17 million minutes of manual operations every year, generates hundreds of millions of dollars in average annual revenue, and achieves a return on investment ratio of up to 20 times.
Analysis and AI are also projects that Austin is responsible for, and he is also eager to look forward to the opportunity to integrate machine learning and AI functions into robotic process automation. His team has built multiple RPA implementations covering natural language processing, optical character recognition (OCR), and machine learning-based decision making. There are many companies that like to talk about "intelligent automation", but AT&T is one of the few companies that can actually achieve this goal.
Develop employee technical capabilities
AT&T has realized that the collective exploration of data science and automation development is inseparable from necessary technical capabilities and resource support. In data science, the company has developed an extensive technical infrastructure to help professional and amateur participants join the automated machine learning research team. It contains the following features:
• Use up to seven different autoML tools simultaneously and compete among them to see which option creates the highest quality model;
• Some autoML tools can directly participate in the production deployment of selected models;
• Various types of reusable data sets can be analyzed through machine learning;
• Provide an intuitive semantic search tool, such as returning all "churn-related features";
• Provide machine learning and The operational tool "Watchtower for MLOps" not only monitors data and AI (traditionally known as MLOps), but also tracks the entire set of activities in the entire business pipeline (applications, API calls, etc.).
In terms of technical automation, AT&T uses Microsoft Power Automate as the tool benchmark, which greatly lowers the threshold for national participation. This tool can be integrated with Microsoft Office tools, PowerBI and even Azure machine learning models. AT&T also maintains a "Bot Marketplace" robot market, where ordinary employees can choose developed automation solutions and obtain configuration guidance from the Automation Center of Excellence when necessary. Every month, approximately 75 new reusable automation components are added to the market.
Cultivate human capabilities
AT&T is also actively promoting community building and paying attention to the human foundation for collective exploration of data science and automation. In terms of data science, the company has established an "AI Popular Forum" to organize online demonstrations every week, share solutions to specific problems, and announce developed functions to participants. The event attracts about 200 employees each week, most of whom are not professional data scientists and many of whom don’t even know how to code. AT&T has also compiled a set of online training materials covering all aspects of data science, with a total of 575 courses to choose from, and provides official certification for various vendor tools.
The construction of the automation community mainly involves providing 40 hours of training courses for employees who want to participate. In addition, the Automation Center of Excellence hosts an annual "Automation Summit" for internal teams to showcase and share their automation projects.
Currently, some enterprises and professional AI developers are still skeptical about the role of ordinary participants in model building and automation solutions. However, AT&T has proven with actual actions that as long as it provides ordinary employees with the right tools and resources, it will be able to establish feasibility and create economic value. Facing a tight data science and automation labor market, AT&T is proving that a well-planned onboarding strategy can create replacement talent to fill the gap.
The above is the detailed content of AT&T explores collective practices in data science and automation. For more information, please follow other related articles on the PHP Chinese website!
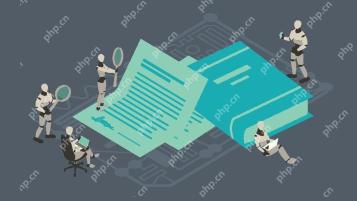
The legal tech revolution is gaining momentum, pushing legal professionals to actively embrace AI solutions. Passive resistance is no longer a viable option for those aiming to stay competitive. Why is Technology Adoption Crucial? Legal professional
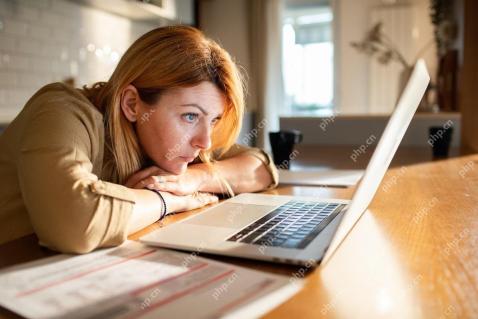
Many assume interactions with AI are anonymous, a stark contrast to human communication. However, AI actively profiles users during every chat. Every prompt, every word, is analyzed and categorized. Let's explore this critical aspect of the AI revo
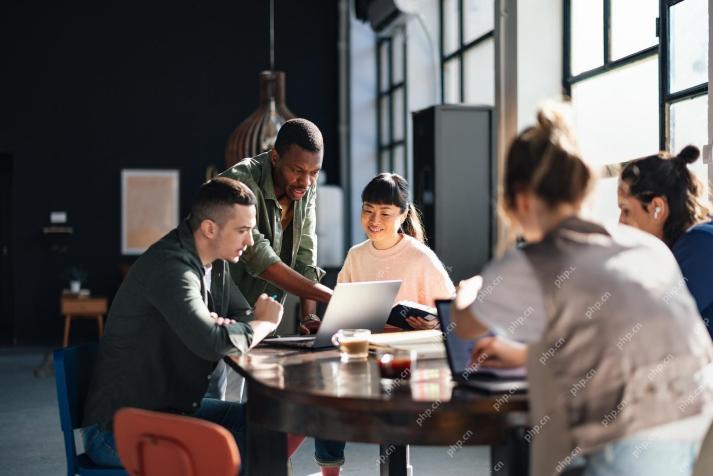
A successful artificial intelligence strategy cannot be separated from strong corporate culture support. As Peter Drucker said, business operations depend on people, and so does the success of artificial intelligence. For organizations that actively embrace artificial intelligence, building a corporate culture that adapts to AI is crucial, and it even determines the success or failure of AI strategies. West Monroe recently released a practical guide to building a thriving AI-friendly corporate culture, and here are some key points: 1. Clarify the success model of AI: First of all, we must have a clear vision of how AI can empower business. An ideal AI operation culture can achieve a natural integration of work processes between humans and AI systems. AI is good at certain tasks, while humans are good at creativity and judgment
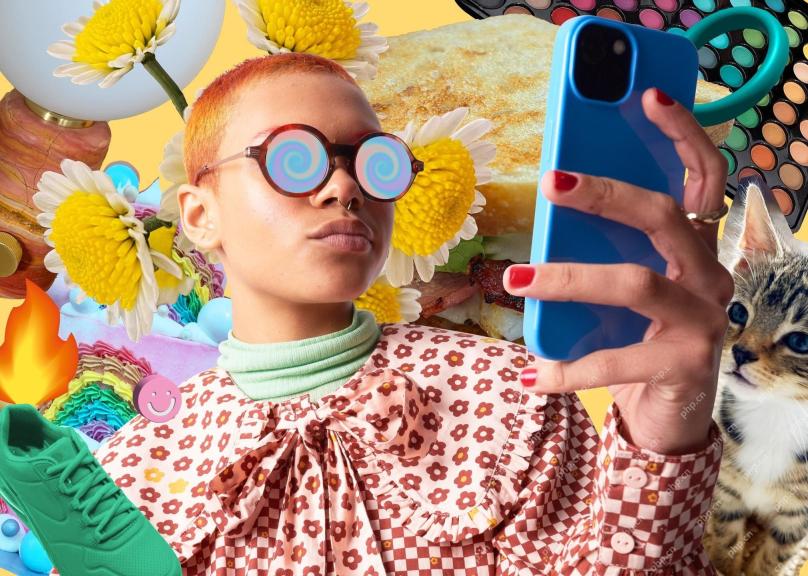
Meta upgrades AI assistant application, and the era of wearable AI is coming! The app, designed to compete with ChatGPT, offers standard AI features such as text, voice interaction, image generation and web search, but has now added geolocation capabilities for the first time. This means that Meta AI knows where you are and what you are viewing when answering your question. It uses your interests, location, profile and activity information to provide the latest situational information that was not possible before. The app also supports real-time translation, which completely changed the AI experience on Ray-Ban glasses and greatly improved its usefulness. The imposition of tariffs on foreign films is a naked exercise of power over the media and culture. If implemented, this will accelerate toward AI and virtual production
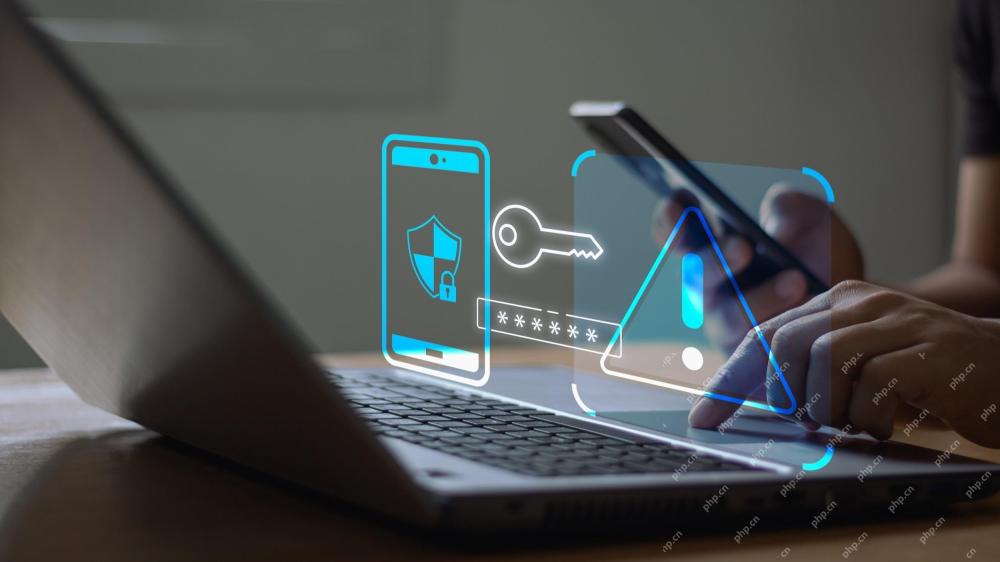
Artificial intelligence is revolutionizing the field of cybercrime, which forces us to learn new defensive skills. Cyber criminals are increasingly using powerful artificial intelligence technologies such as deep forgery and intelligent cyberattacks to fraud and destruction at an unprecedented scale. It is reported that 87% of global businesses have been targeted for AI cybercrime over the past year. So, how can we avoid becoming victims of this wave of smart crimes? Let’s explore how to identify risks and take protective measures at the individual and organizational level. How cybercriminals use artificial intelligence As technology advances, criminals are constantly looking for new ways to attack individuals, businesses and governments. The widespread use of artificial intelligence may be the latest aspect, but its potential harm is unprecedented. In particular, artificial intelligence
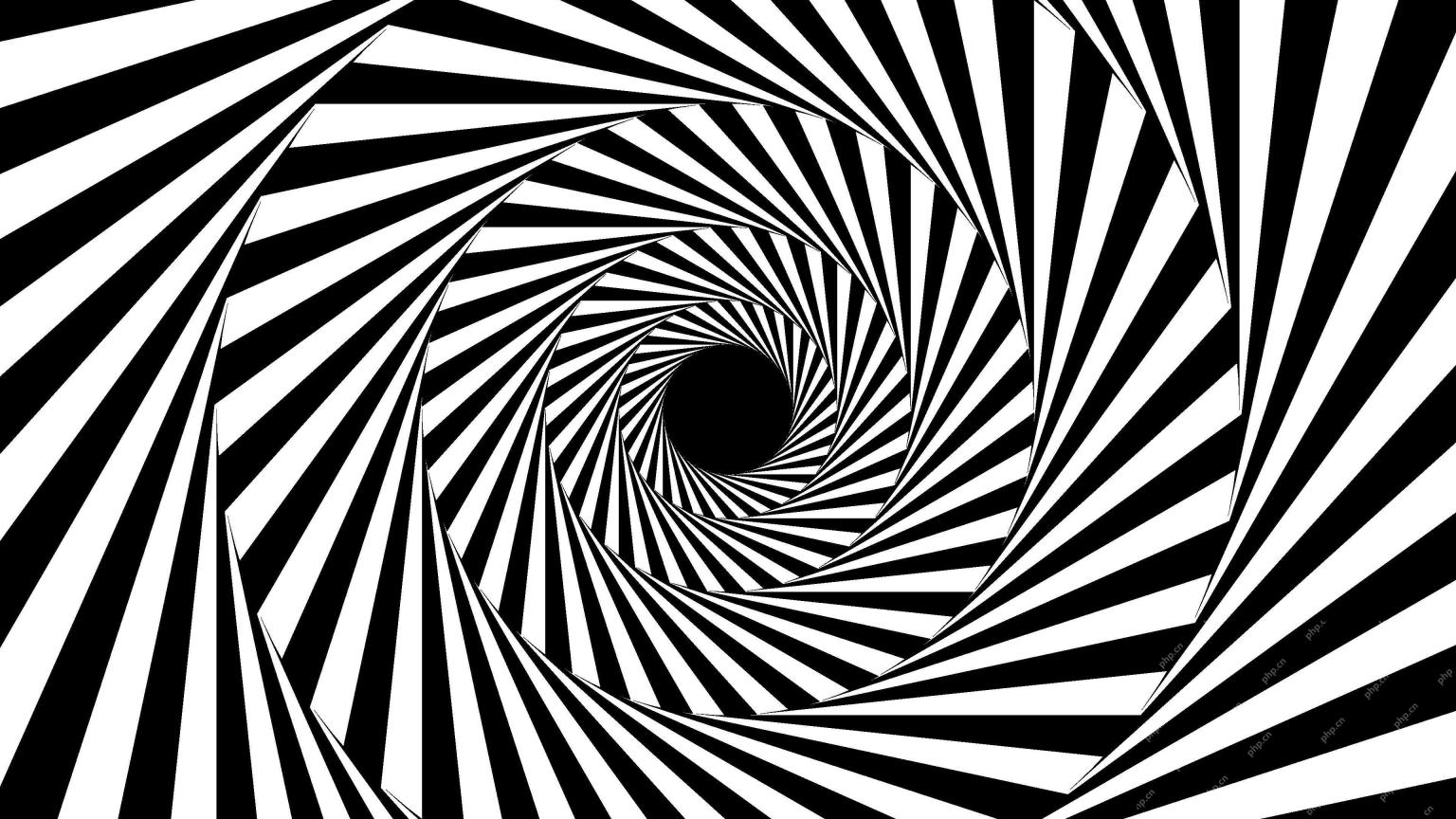
The intricate relationship between artificial intelligence (AI) and human intelligence (NI) is best understood as a feedback loop. Humans create AI, training it on data generated by human activity to enhance or replicate human capabilities. This AI
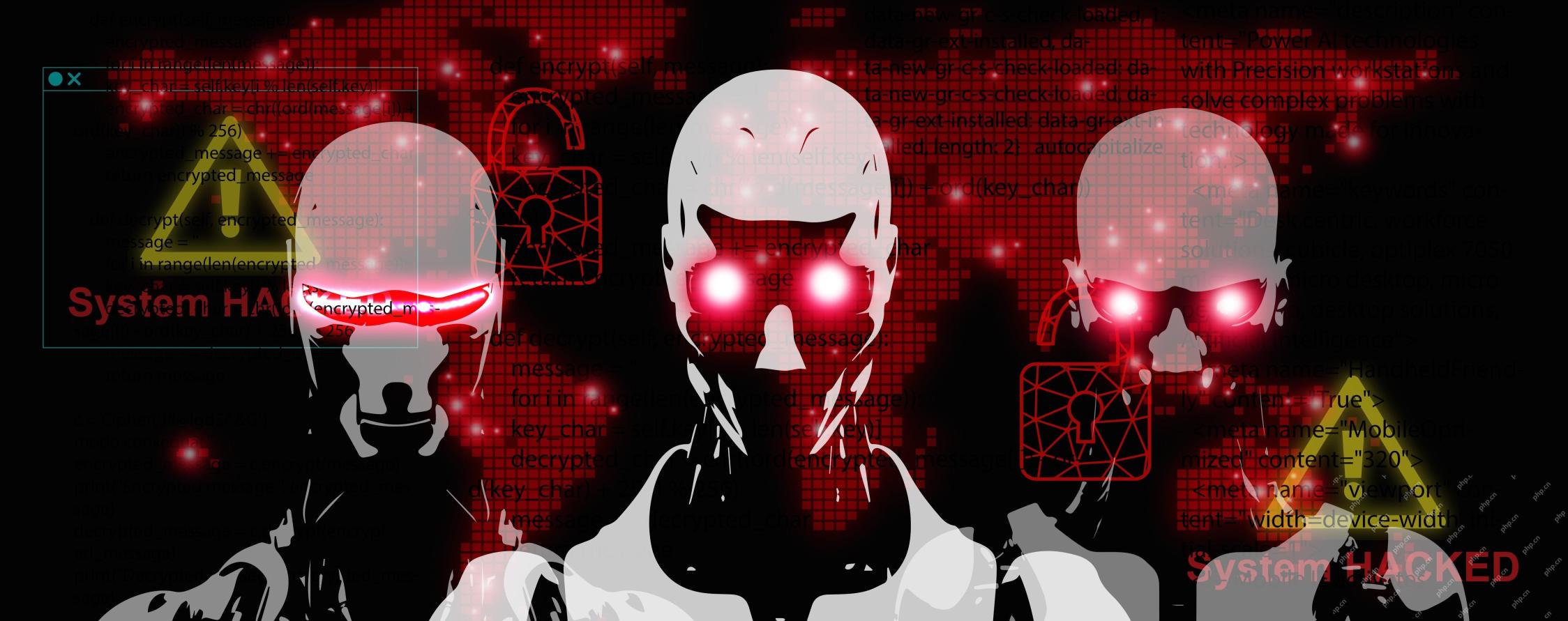
Anthropic's recent statement, highlighting the lack of understanding surrounding cutting-edge AI models, has sparked a heated debate among experts. Is this opacity a genuine technological crisis, or simply a temporary hurdle on the path to more soph
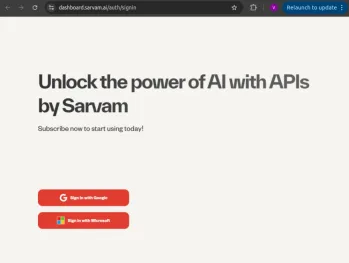
India is a diverse country with a rich tapestry of languages, making seamless communication across regions a persistent challenge. However, Sarvam’s Bulbul-V2 is helping to bridge this gap with its advanced text-to-speech (TTS) t


Hot AI Tools

Undresser.AI Undress
AI-powered app for creating realistic nude photos

AI Clothes Remover
Online AI tool for removing clothes from photos.

Undress AI Tool
Undress images for free

Clothoff.io
AI clothes remover

Video Face Swap
Swap faces in any video effortlessly with our completely free AI face swap tool!

Hot Article

Hot Tools

Safe Exam Browser
Safe Exam Browser is a secure browser environment for taking online exams securely. This software turns any computer into a secure workstation. It controls access to any utility and prevents students from using unauthorized resources.

SublimeText3 Linux new version
SublimeText3 Linux latest version

DVWA
Damn Vulnerable Web App (DVWA) is a PHP/MySQL web application that is very vulnerable. Its main goals are to be an aid for security professionals to test their skills and tools in a legal environment, to help web developers better understand the process of securing web applications, and to help teachers/students teach/learn in a classroom environment Web application security. The goal of DVWA is to practice some of the most common web vulnerabilities through a simple and straightforward interface, with varying degrees of difficulty. Please note that this software

SublimeText3 English version
Recommended: Win version, supports code prompts!
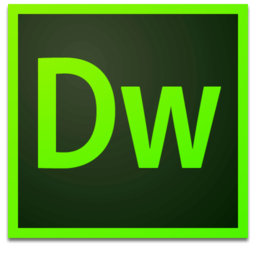
Dreamweaver Mac version
Visual web development tools
