AI bias is a serious problem that can have a variety of consequences for individuals.
As artificial intelligence advances, questions and ethical dilemmas surrounding data science solutions begin to surface. Because humans have removed themselves from the decision-making process, they want to ensure that the judgments made by these algorithms are neither biased nor discriminatory. Artificial intelligence must be supervised at all times. We cannot say that this possible bias is caused by artificial intelligence, as it is a digital system based on predictive analytics that can process large amounts of data. The problem starts much earlier, with unsupervised data being "fed" into the system.
Throughout history, humans have always had prejudices and discrimination. Our actions don't appear to be changing anytime soon. Biases are found in systems and algorithms that, unlike humans, appear immune to the problem.
What is artificial intelligence bias?
AI bias occurs in data-related fields when the way data is obtained results in samples that do not correctly represent interest groups. This suggests that people from certain races, creeds, colors and genders are underrepresented in data samples. This may lead the system to make discriminating conclusions. It also raises questions about what data science consulting is and why it’s important.
Bias in AI does not mean that the AI system is created to intentionally favor a specific group of people. The goal of artificial intelligence is to enable individuals to express their desires through examples rather than instructions. So, if AI is biased, it can only be because the data is biased! Artificial intelligence decision-making is an idealized process that operates in the real world, and it cannot hide human flaws. Incorporating guided learning is also beneficial.
Why does it happen?
The problem of artificial intelligence bias arises because the data may contain human choices based on preconceptions, which are conducive to drawing good algorithmic conclusions. There are several real-life examples of AI bias. Racial people and famous drag queens were discriminated against by Google's hate speech detection system. For 10 years, Amazon's human resources algorithms have primarily fed data on male employees, resulting in female candidates being more likely to be rated as qualified for jobs at Amazon.
Facial recognition algorithms have a higher error rate when analyzing the faces of minorities, especially minority women, according to data scientists at the Massachusetts Institute of Technology (MIT). This may be because the algorithm was primarily fed white male faces during training.
Because Amazon’s algorithms are trained on data from its 112 million Prime users in the U.S., as well as tens of millions of additional individuals who frequent the site and frequently use its other merchandise, the company can predict Consumer purchasing behavior. Google's advertising business is based on predictive algorithms fed by data from the billions of internet searches it conducts every day and the 2.5 billion Android smartphones on the market. These Internet giants have established huge data monopolies and have nearly insurmountable advantages in the field of artificial intelligence.
How can synthetic data help address AI bias?
In an ideal society, no one would be biased and everyone would have equal opportunities, regardless of skin color, gender, religion or Sexual orientation. However, it exists in the real world, and those who are different from the majority in certain areas have a harder time finding jobs and obtaining education, making them underrepresented in many statistics. Depending on the goals of the AI system, this could lead to erroneous inferences that such people are less skilled, less likely to be included in these data sets, and less suitable to achieve good scores.
On the other hand, AI data could be a big step in the direction of unbiased AI. Here are some concepts to consider:
Look at real-world data and see where the bias is. The data is then synthesized using real-world data and observable biases. If you want to create an ideal virtual data generator, you need to include a definition of fairness that attempts to transform biased data into data that might be considered fair.
AI-generated data may fill in the gaps in a data set that don’t vary much or aren’t large enough to form an unbiased data set. Even with a large sample size, it is possible that some people were excluded or underrepresented compared to others. This problem must be solved using synthetic data.
Data mining can be more expensive than generating unbiased data. Actual data collection requires measurements, interviews, large samples, and in any case a lot of effort. Data generated by AI is cheap and requires only the use of data science and machine learning algorithms.
Over the past few years, executives at many for-profit synthetic data companies, as well as MitreCorp., the founder of Synthea, have noticed a surge in interest in their services. However, as algorithms are used more widely to make life-changing decisions, they are being found to exacerbate racism, sexism, and harmful biases in other high-impact areas, including facial recognition, crime prediction, and health care decision-making. Researchers say training algorithms on algorithmically generated data increases the likelihood that AI systems will perpetuate harmful biases in many situations.
The above is the detailed content of How to avoid AI bias issues with synthetic data generators. For more information, please follow other related articles on the PHP Chinese website!
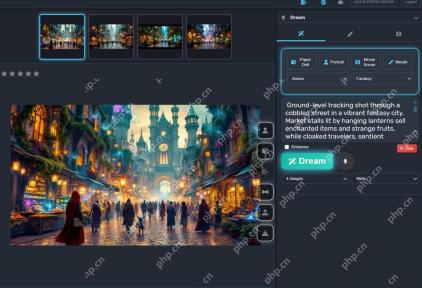
Upheaval Games: Revolutionizing Game Development with AI Agents Upheaval, a game development studio comprised of veterans from industry giants like Blizzard and Obsidian, is poised to revolutionize game creation with its innovative AI-powered platfor
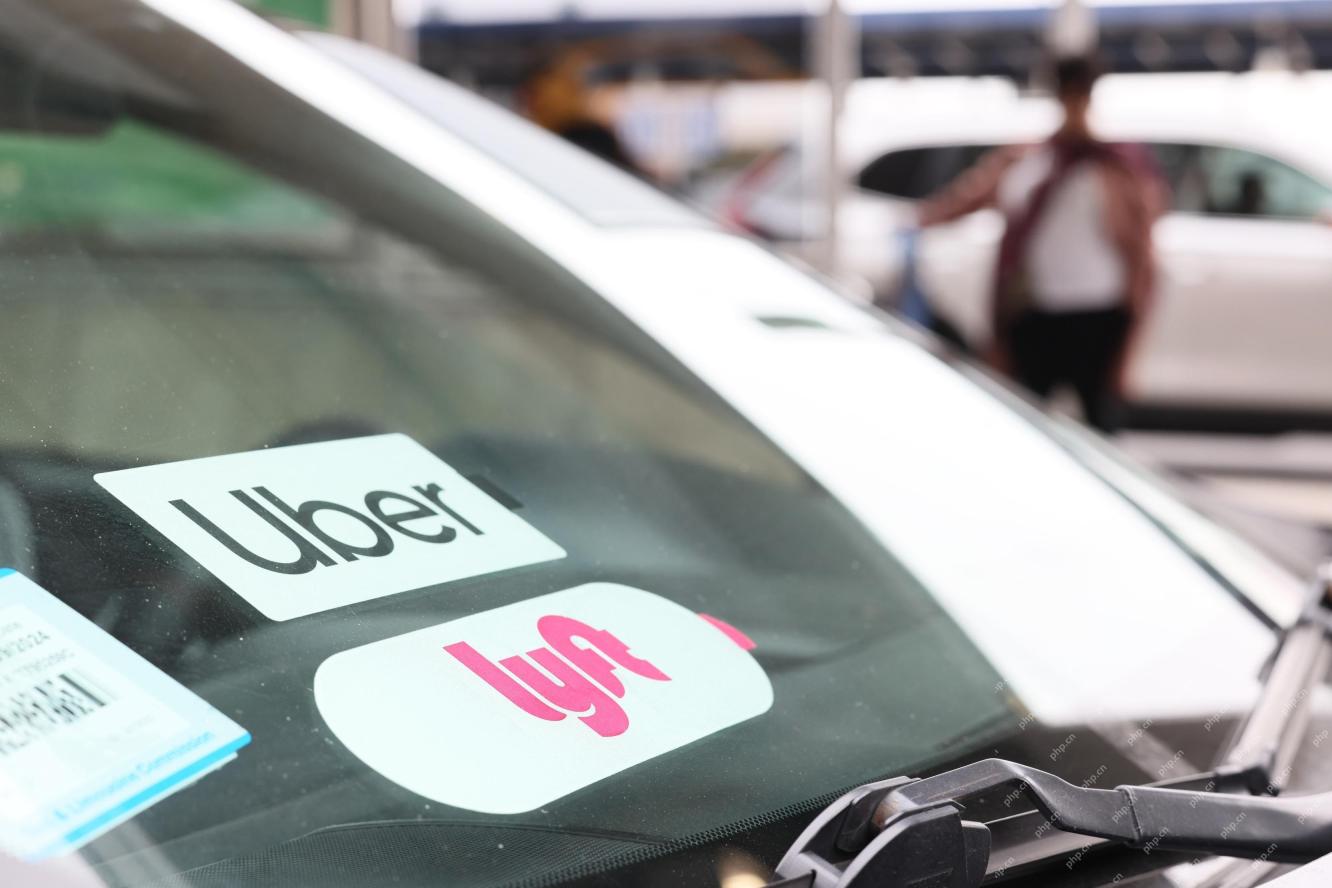
Uber's RoboTaxi Strategy: A Ride-Hail Ecosystem for Autonomous Vehicles At the recent Curbivore conference, Uber's Richard Willder unveiled their strategy to become the ride-hail platform for robotaxi providers. Leveraging their dominant position in
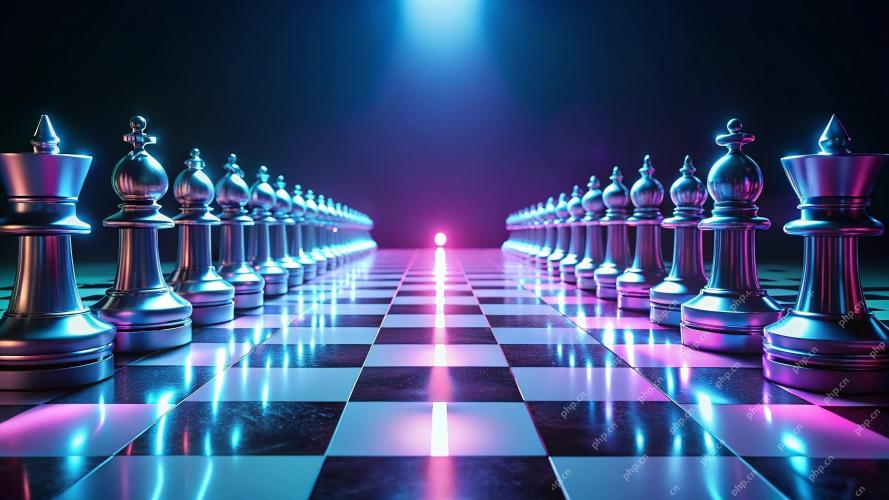
Video games are proving to be invaluable testing grounds for cutting-edge AI research, particularly in the development of autonomous agents and real-world robots, even potentially contributing to the quest for Artificial General Intelligence (AGI). A
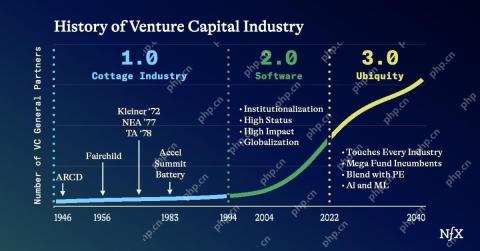
The impact of the evolving venture capital landscape is evident in the media, financial reports, and everyday conversations. However, the specific consequences for investors, startups, and funds are often overlooked. Venture Capital 3.0: A Paradigm
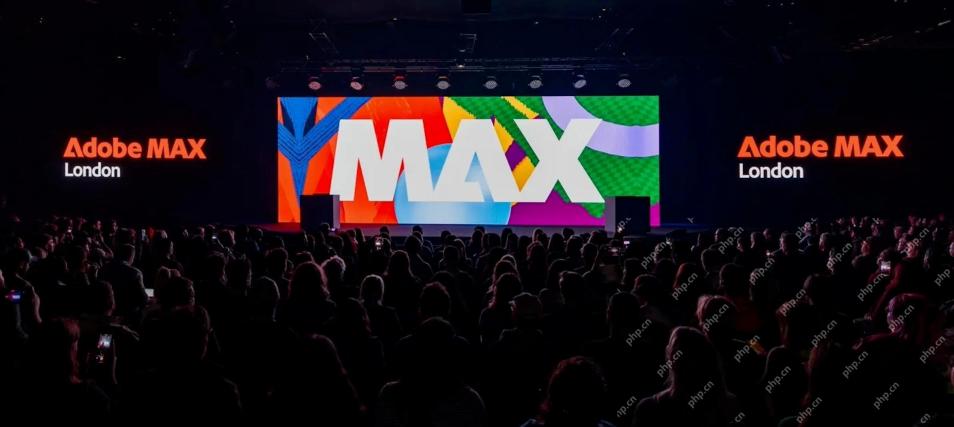
Adobe MAX London 2025 delivered significant updates to Creative Cloud and Firefly, reflecting a strategic shift towards accessibility and generative AI. This analysis incorporates insights from pre-event briefings with Adobe leadership. (Note: Adob
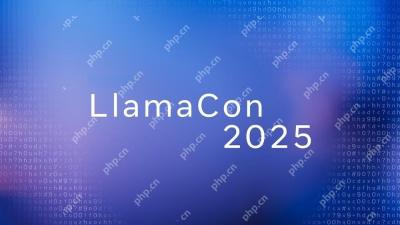
Meta's LlamaCon announcements showcase a comprehensive AI strategy designed to compete directly with closed AI systems like OpenAI's, while simultaneously creating new revenue streams for its open-source models. This multifaceted approach targets bo
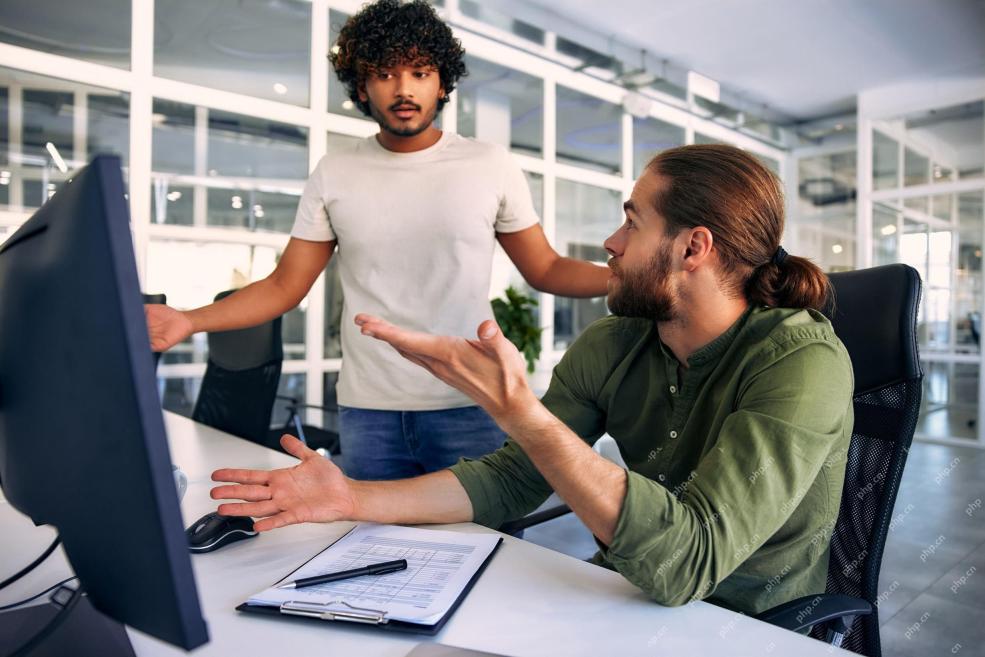
There are serious differences in the field of artificial intelligence on this conclusion. Some insist that it is time to expose the "emperor's new clothes", while others strongly oppose the idea that artificial intelligence is just ordinary technology. Let's discuss it. An analysis of this innovative AI breakthrough is part of my ongoing Forbes column that covers the latest advancements in the field of AI, including identifying and explaining a variety of influential AI complexities (click here to view the link). Artificial intelligence as a common technology First, some basic knowledge is needed to lay the foundation for this important discussion. There is currently a large amount of research dedicated to further developing artificial intelligence. The overall goal is to achieve artificial general intelligence (AGI) and even possible artificial super intelligence (AS)
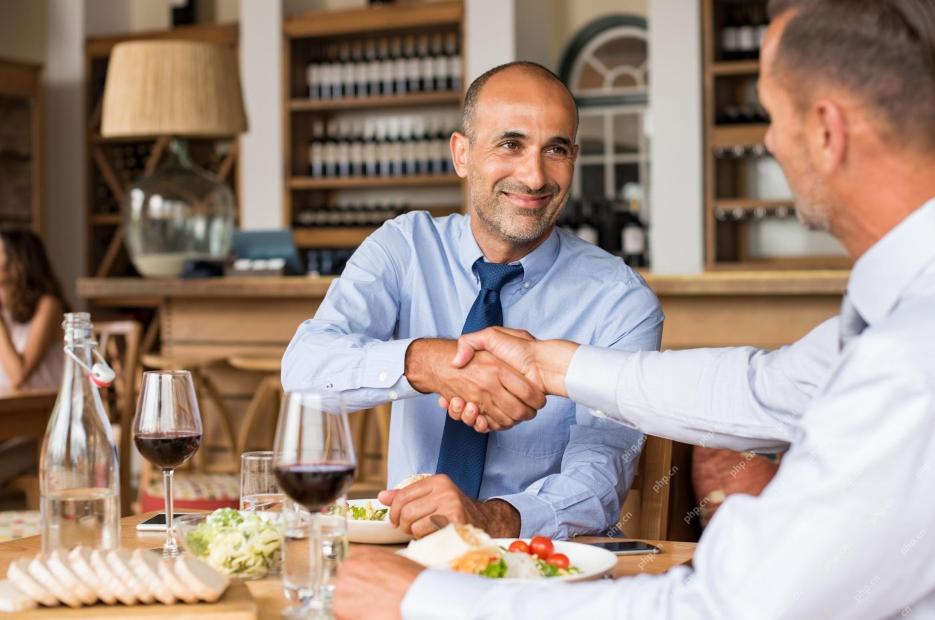
The effectiveness of a company's AI model is now a key performance indicator. Since the AI boom, generative AI has been used for everything from composing birthday invitations to writing software code. This has led to a proliferation of language mod


Hot AI Tools

Undresser.AI Undress
AI-powered app for creating realistic nude photos

AI Clothes Remover
Online AI tool for removing clothes from photos.

Undress AI Tool
Undress images for free

Clothoff.io
AI clothes remover

Video Face Swap
Swap faces in any video effortlessly with our completely free AI face swap tool!

Hot Article

Hot Tools
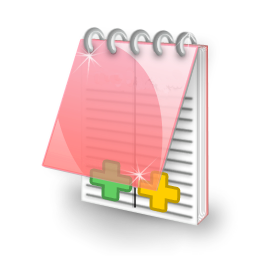
EditPlus Chinese cracked version
Small size, syntax highlighting, does not support code prompt function

SecLists
SecLists is the ultimate security tester's companion. It is a collection of various types of lists that are frequently used during security assessments, all in one place. SecLists helps make security testing more efficient and productive by conveniently providing all the lists a security tester might need. List types include usernames, passwords, URLs, fuzzing payloads, sensitive data patterns, web shells, and more. The tester can simply pull this repository onto a new test machine and he will have access to every type of list he needs.

MinGW - Minimalist GNU for Windows
This project is in the process of being migrated to osdn.net/projects/mingw, you can continue to follow us there. MinGW: A native Windows port of the GNU Compiler Collection (GCC), freely distributable import libraries and header files for building native Windows applications; includes extensions to the MSVC runtime to support C99 functionality. All MinGW software can run on 64-bit Windows platforms.
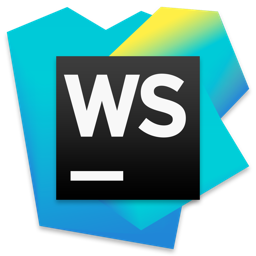
WebStorm Mac version
Useful JavaScript development tools
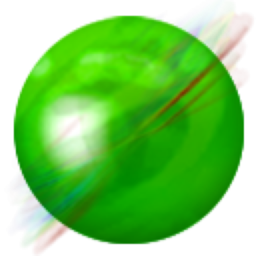
ZendStudio 13.5.1 Mac
Powerful PHP integrated development environment
