


In today's information age, images or visual content have long become the most important carrier of information in daily life. Deep learning models rely on their strong ability to understand visual content and can Various processing and optimization.
However, in the past development and application of visual models, we paid more attention to the optimization of the model itself to improve its speed and effect. On the contrary, for the pre- and post-processing stages of images, little serious thought is given to how to optimize them. Therefore, when the computational efficiency of the model is getting higher and higher, looking back at the pre-processing and post-processing of the image, I did not expect that they have become the bottleneck of the entire image task.
In order to solve such bottlenecks, NVIDIA has joined hands with the ByteDance machine learning team to open source many image preprocessing operator libraries CV-CUDA. They can run efficiently on the GPU, and the operator speed can reach the speed of OpenCV (running on the CPU) About a hundred times. If we use CV-CUDA as the backend to replace OpenCV and TorchVision, the throughput of the entire inference can reach more than 20 times the original. In addition, not only is the speed improved, but also in terms of effect, CV-CUDA has been aligned with OpenCV in terms of calculation accuracy, so training and inference can be seamlessly connected, greatly reducing the workload of engineers.
Taking the image background blur algorithm as an example, CV-CUDA replaces OpenCV as the backend for image pre/post-processing , the throughput of the entire reasoning process can be increased by more than 20 times.
#If you guys want to try a faster and better visual preprocessing library, you can try this open source tool. Open source address: https://github.com/CVCUDA/CV-CUDA
Image pre-/Post-processing has become CV Bottleneck
Many algorithm engineers involved in engineering and products know that although we often only discuss "cutting-edge research" such as model structure and training tasks, we actually need to In order to build a reliable product, you will encounter many engineering problems in the process, but model training is the easiest part.
Image preprocessing is such an engineering problem. We may simply call some APIs to perform geometric transformation, filtering, color transformation, etc. on the image during experiments or training, and we may not particularly care about it. But when we rethink the entire reasoning process, we find that image preprocessing has become a performance bottleneck, especially for visual tasks with complex preprocessing processes.
Such performance bottlenecks are mainly reflected in the CPU. Generally speaking, for conventional image processing processes, we will first perform preprocessing on the CPU, then put it on the GPU to run the model, and finally return to the CPU, and may need to do some post-processing.
Take the image background blur algorithm as an example. In the conventional image processing process, prognosis processing is mainly completed on the CPU, occupying the entire 90% of the workload, it has become the bottleneck for the task.
So for complex scenes such as video applications or 3D image modeling, because the number of image frames or image information is large enough, the preprocessing process is complex enough, and If the latency requirement is low enough, optimizing the pre/post-processing operators is imminent. A better approach, of course, is to replace OpenCV with a faster solution.
Why OpenCV is still not good enough?
In CV, the most widely used image processing library is of course the long-maintained OpenCV. It has a very wide range of image processing operations and can basically meet various visual tasks. pre/post-processing required. However, as the load of image tasks increases, its speed has slowly been unable to keep up, because most of OpenCV's image operations are implemented by the CPU, lacking GPU implementation, or there are some problems with the GPU implementation.
In the research and development experience of NVIDIA and ByteDance algorithm students, they found that there are three major problems in the few operators implemented by GPU in OpenCV:
- The CPU and GPU result accuracy of some operators cannot be aligned;
- The GPU performance of some operators is weaker than the CPU performance;
- There are various CPU operators and various GPU operators at the same time. When the processing process needs to use both at the same time, additional space applications and requirements in memory and video memory are added. Data migration/data copy;
For example, the accuracy of the results of the first question cannot be aligned. NVIDIA and ByteDance algorithm students will find that when we During training, a certain operator of OpenCV uses the CPU, but due to performance issues during the inference phase, the GPU operator corresponding to OpenCV is used instead. Perhaps the accuracy of the CPU and GPU results cannot be aligned, resulting in accuracy anomalies in the entire inference process. When such a problem occurs, you either need to switch back to the CPU implementation, or you need to spend a lot of effort to realign the accuracy, which is a difficult problem to deal with.
Since OpenCV is still not good enough, some readers may ask, what about Torchvision? It will actually face the same problems as OpenCV. In addition, engineers deploying models are more likely to use C to implement the inference process for efficiency. Therefore, they will not be able to use Torchvision and need to turn to a C vision library such as OpenCV. This will bring Another dilemma: aligning the accuracy of Torchvision with OpenCV.
In general, the current pre/post-processing of visual tasks on the CPU has become a bottleneck, but traditional tools such as OpenCV cannot handle it well. Therefore, migrating operations to the GPU, and using CV-CUDA, an efficient image processing operator library implemented entirely based on CUDA, has become a new solution.
Performing pre-processing and post-processing entirely on the GPU will greatly reduce the CPU bottleneck in the image processing part.
GPU Image processing acceleration library: CV-CUDA
As a CUDA-based pre-processor / Post-processing operator library, algorithm engineers may look forward to three things most: fast enough, versatile enough, and easy to use. CV-CUDA, jointly developed by NVIDIA and ByteDance's machine learning team, can exactly meet these three points. It uses the parallel computing power of the GPU to improve operator speed, aligns OpenCV operation results to be versatile enough, and is easy to use with the C/Python interface.
speed of CV-CUDA
CV-CUDA The speed is first reflected in the efficient operator implementation. After all, it is written by NVIDIA. The CUDA parallel computing code must have undergone a lot of optimization. Secondly, it supports batch operations, which can make full use of the computing power of the GPU device. Compared with the serial execution of images on the CPU, batch operations are definitely much faster. Finally, thanks to the GPU architectures such as Volta, Turing, and Ampere that CV-CUDA is adapted to, the performance is highly optimized at the CUDA kernel level of each GPU to achieve the best results. In other words, the better the GPU card you use, the more exaggerated its acceleration capabilities will be.
As shown in the previous background blur throughput acceleration ratio chart, if CV-CUDA is used to replace the pre- and post-processing of OpenCV and TorchVision, the throughput of the entire inference process is increased by more than 20 times. Among them, preprocessing performs operations such as Resize, Padding, and Image2Tensor on the image, and postprocessing performs operations such as Tensor2Mask, Crop, Resize, and Denoise on the prediction results.
On the same compute node (2x Intel Xeon Platinum 8168 CPUs, 1x NVIDIA A100 GPU), Processes 1080p video at 30fps, using the maximum number of parallel streams supported by different CV libraries. The test used 4 processes, each process batchSize is 64. Regarding the performance of a single operator, NVIDIA and ByteDance’s partners have also conducted performance tests. The throughput of many operators on the GPU can reach a hundred times that of the CPU. .
The image size is 480*360, the CPU is Intel(R) Core(TM) i9-7900X, the BatchSize size is 1, and the number of processes is 1
Although many pre/post-processing operators are not simple matrix multiplication and other operations, in order to achieve the above-mentioned efficient performance, CV-CUDA has actually done a lot of optimization at the operator level. For example, a large number of kernel fusion strategies are adopted to reduce the access time of kernel launch and global memory; memory access is optimized to improve data reading and writing efficiency; all operators are processed asynchronously to reduce the time of synchronous waiting, etc.
##CV-CUDA’s universal and flexible
operations The stability of the results is very important for actual projects. For example, the common Resize operation, OpenCV, OpenCV-gpu and Torchvision are implemented in different ways, so from training to deployment, there will be a lot more work to align the results. At the beginning of the design of CV-CUDA, it was considered that many engineers are accustomed to using the CPU version of OpenCV in the current image processing library. Therefore, when designing operators, whether it is function parameters or image processing results, align OpenCV as much as possible CPU version of the operator. Therefore, when migrating from OpenCV to CV-CUDA, only a few changes are needed to obtain consistent computing results, and the model does not need to be retrained.
In addition, CV-CUDA is designed from the operator level, so no matter what the pre/post-processing process of the model is, it can be freely combined and has high flexibility.
The ByteDance machine learning team stated that there are many models trained within the enterprise, and the required preprocessing logic is also diverse, with many customized preprocessing logic requirements. The flexibility of CV-CUDA can ensure that each OP supports the incoming of stream objects and video memory objects (Buffer and Tensor classes, which store video memory pointers internally), so that corresponding GPU resources can be configured more flexibly. When designing and developing each op, we not only take into account versatility, but also provide customized interfaces on demand, covering various needs for image preprocessing.
CV-CUDA’s ease of use
may be a lot Engineers will think, CV-CUDA involves the underlying CUDA operator, so it should be more difficult to use? But this is not the case. Even if it does not rely on higher-level APIs, the bottom layer of CV-CUDA itself will provide structures such as and the Allocator class, so it is not troublesome to adjust it in C. In addition, going to the upper level, CV-CUDA provides data conversion interfaces for PyTorch, OpenCV and Pillow, so engineers can quickly replace and call operators in a familiar way. In addition, because CV-CUDA has both a C interface and a Python interface, it can be used in both training and service deployment scenarios. The Python interface is used to quickly verify model capabilities during training, and the C interface is used for more efficient deployment. predict. CV-CUDA avoids the cumbersome preprocessing result alignment process and improves the efficiency of the overall process.
CV-CUDA C interface for Resize
Practical combat,CV-CUDAHow to use
If we use the Python interface of CV-CUDA during the training process, it will actually be very simple to use. , it only takes a few simple steps to migrate all preprocessing operations originally performed on the CPU to the GPU. Taking image classification as an example, basically we need to decode the image into a tensor in the preprocessing stage and crop it to fit the model input size. After cropping, we need to convert the pixel value into a floating point data type and After normalization, it can be passed to the deep learning model for forward propagation. Below we will use some simple code blocks to experience how CV-CUDA preprocesses images and interacts with Pytorch.
Conventional image recognition pre-processing process, using CV-CUDA will unify the pre-processing process and model calculation Run on GPU.
As follows, after using the torchvision API to load images to the GPU, the Torch Tensor type can be directly converted into the CV-CUDA object nvcvInputTensor through as_tensor, so that the API of the CV-CUDA preprocessing operation can be directly called. Various transformations of images are completed in the GPU.
##The following lines of code will use CV-CUDA to complete the preprocessing process of image recognition in the GPU: crop the image and Normalize the pixels. Among them, resize() converts the image tensor into the input tensor size of the model; convertto() converts the pixel value into a single-precision floating point value; normalize() normalizes the pixel value to make the value range more suitable for the model. train. CV-CUDA The use of various preprocessing operations will not be much different from those in OpenCV or Torchvision. It is just a simple adjustment of the method, and the operation has already been completed on the GPU behind it.
Now with the help of various APIs of CV-CUDA, the preprocessing of the image classification task has been completed. It can efficiently complete parallel computing on GPU and be easily integrated into the modeling process of mainstream deep learning frameworks such as PyTorch. For the rest, you only need to convert the CV-CUDA object nvcvPreprocessedTensor into the Torch Tensor type to feed it to the model. This step is also very simple. The conversion only requires one line of code:
Through this simple example, it is easy to find that CV-CUDA is indeed easily embedded into normal model training logic. If readers want to know more usage details, they can still check the open source address of CV-CUDA mentioned above.
CV-CUDAImprovement of actual business
CV-CUDA has actually gone through actual business improvements test. In visual tasks, especially tasks with relatively complex image pre-processing processes, using the huge computing power of the GPU for pre-processing can effectively improve the efficiency of model training and inference. CV-CUDA is currently used in multiple online and offline scenarios within Douyin Group, such as multi-modal search, image classification, etc. The ByteDance machine learning team stated that the internal use of CV-CUDA can significantly improve the performance of training and inference. For example, in terms of training, ByteDance is a video-related multi-modal task. The pre-processing part includes the decoding of multi-frame videos and a lot of data enhancement, making this part of the logic very complicated. Complex preprocessing logic causes the multi-core performance of the CPU to still not keep up during training. Therefore, CV-CUDA is used to migrate all preprocessing logic on the CPU to the GPU, and the overall training speed is accelerated by 90%. Note that this is an increase in overall training speed, not just in the preprocessing part.
##In Bytedance OCR and video multi-modal tasks, by using CV-CUDA, the overall training speed can be Improved by 1 to 2 times (note: it is an increase in the overall training speed of the model)
The same is true in the inference process. The ByteDance machine learning team stated that in a After using CV-CUDA in the search multi-modal task, the overall online throughput has been improved by more than 2 times compared with using CPU for preprocessing. It is worth noting that the CPU baseline results here have been highly optimized for multi-core, and the preprocessing logic involved in this task is relatively simple, but the acceleration effect is still very obvious after using CV-CUDA. The speed is efficient enough to break the preprocessing bottleneck in visual tasks, and it is also simple and flexible to use. CV-CUDA has proven that it can greatly improve model reasoning and training effects in actual application scenarios, so if Readers' visual tasks are also limited by preprocessing efficiency, so try the latest open source CV-CUDA.
The above is the detailed content of The image preprocessing library CV-CUDA is open sourced, breaking the preprocessing bottleneck and increasing inference throughput by more than 20 times.. For more information, please follow other related articles on the PHP Chinese website!
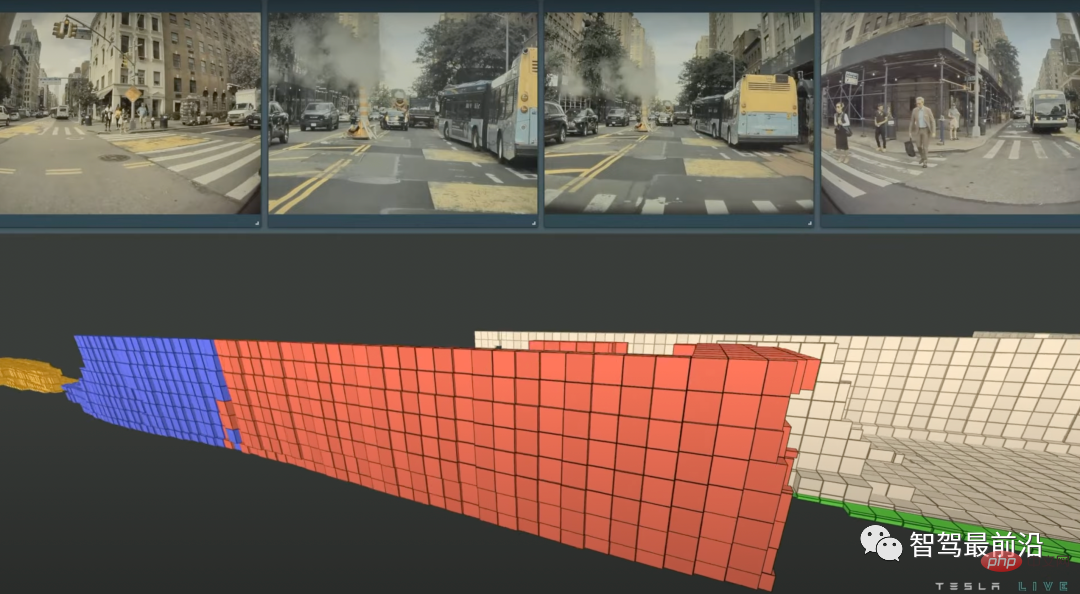
特斯拉是一个典型的AI公司,过去一年训练了75000个神经网络,意味着每8分钟就要出一个新的模型,共有281个模型用到了特斯拉的车上。接下来我们分几个方面来解读特斯拉FSD的算法和模型进展。01 感知 Occupancy Network特斯拉今年在感知方面的一个重点技术是Occupancy Network (占据网络)。研究机器人技术的同学肯定对occupancy grid不会陌生,occupancy表示空间中每个3D体素(voxel)是否被占据,可以是0/1二元表示,也可以是[0, 1]之间的
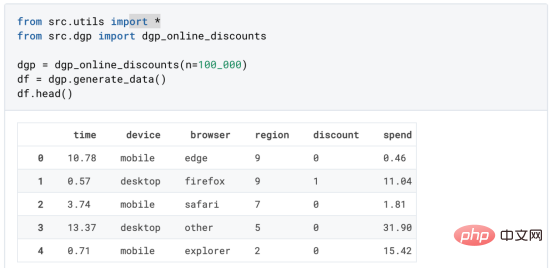
译者 | 朱先忠审校 | 孙淑娟在我之前的博客中,我们已经了解了如何使用因果树来评估政策的异质处理效应。如果你还没有阅读过,我建议你在阅读本文前先读一遍,因为我们在本文中认为你已经了解了此文中的部分与本文相关的内容。为什么是异质处理效应(HTE:heterogenous treatment effects)呢?首先,对异质处理效应的估计允许我们根据它们的预期结果(疾病、公司收入、客户满意度等)选择提供处理(药物、广告、产品等)的用户(患者、用户、客户等)。换句话说,估计HTE有助于我
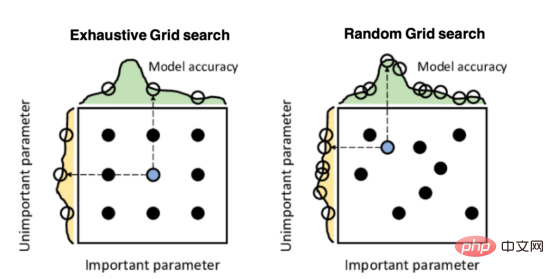
译者 | 朱先忠审校 | 孙淑娟引言模型超参数(或模型设置)的优化可能是训练机器学习算法中最重要的一步,因为它可以找到最小化模型损失函数的最佳参数。这一步对于构建不易过拟合的泛化模型也是必不可少的。优化模型超参数的最著名技术是穷举网格搜索和随机网格搜索。在第一种方法中,搜索空间被定义为跨越每个模型超参数的域的网格。通过在网格的每个点上训练模型来获得最优超参数。尽管网格搜索非常容易实现,但它在计算上变得昂贵,尤其是当要优化的变量数量很大时。另一方面,随机网格搜索是一种更快的优化方法,可以提供更好的
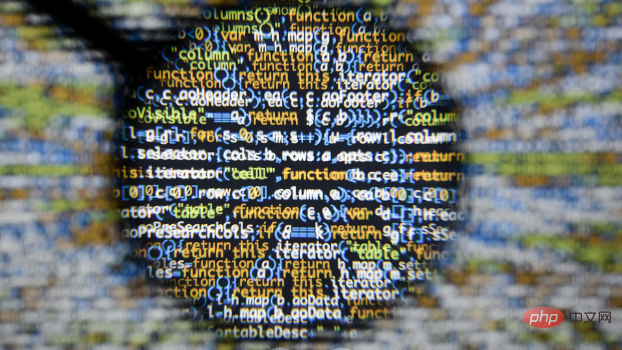
导读:因果推断是数据科学的一个重要分支,在互联网和工业界的产品迭代、算法和激励策略的评估中都扮演者重要的角色,结合数据、实验或者统计计量模型来计算新的改变带来的收益,是决策制定的基础。然而,因果推断并不是一件简单的事情。首先,在日常生活中,人们常常把相关和因果混为一谈。相关往往代表着两个变量具有同时增长或者降低的趋势,但是因果意味着我们想要知道对一个变量施加改变的时候会发生什么样的结果,或者说我们期望得到反事实的结果,如果过去做了不一样的动作,未来是否会发生改变?然而难点在于,反事实的数据往往是
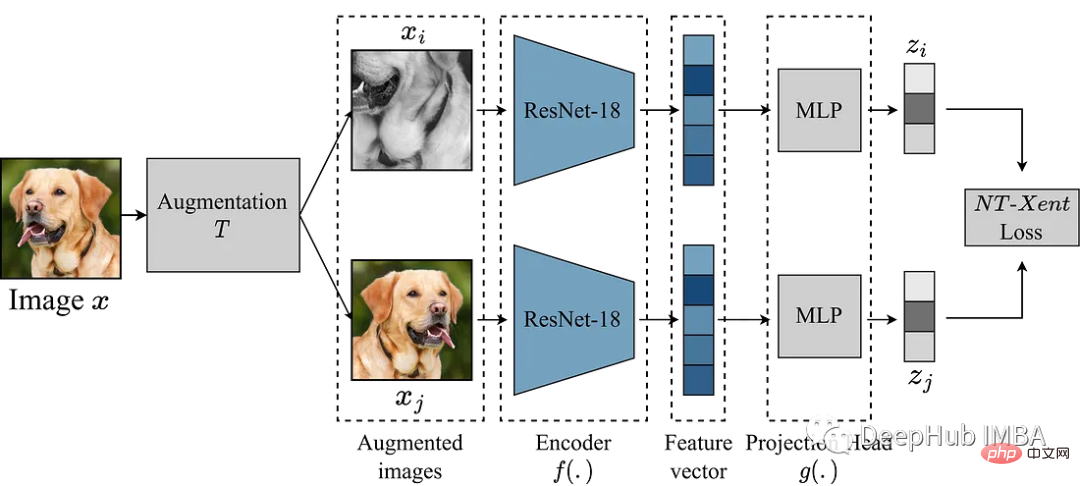
SimCLR(Simple Framework for Contrastive Learning of Representations)是一种学习图像表示的自监督技术。 与传统的监督学习方法不同,SimCLR 不依赖标记数据来学习有用的表示。 它利用对比学习框架来学习一组有用的特征,这些特征可以从未标记的图像中捕获高级语义信息。SimCLR 已被证明在各种图像分类基准上优于最先进的无监督学习方法。 并且它学习到的表示可以很容易地转移到下游任务,例如对象检测、语义分割和小样本学习,只需在较小的标记
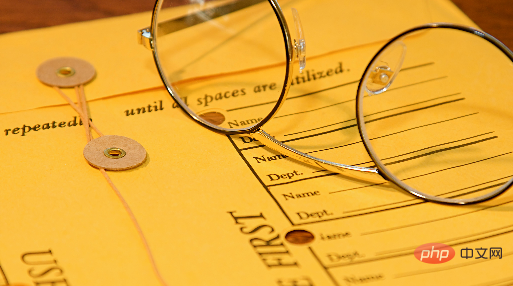
一、盒马供应链介绍1、盒马商业模式盒马是一个技术创新的公司,更是一个消费驱动的公司,回归消费者价值:买的到、买的好、买的方便、买的放心、买的开心。盒马包含盒马鲜生、X 会员店、盒马超云、盒马邻里等多种业务模式,其中最核心的商业模式是线上线下一体化,最快 30 分钟到家的 O2O(即盒马鲜生)模式。2、盒马经营品类介绍盒马精选全球品质商品,追求极致新鲜;结合品类特点和消费者购物体验预期,为不同品类选择最为高效的经营模式。盒马生鲜的销售占比达 60%~70%,是最核心的品类,该品类的特点是用户预期时
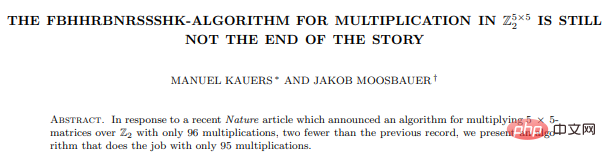
10 月 5 日,AlphaTensor 横空出世,DeepMind 宣布其解决了数学领域 50 年来一个悬而未决的数学算法问题,即矩阵乘法。AlphaTensor 成为首个用于为矩阵乘法等数学问题发现新颖、高效且可证明正确的算法的 AI 系统。论文《Discovering faster matrix multiplication algorithms with reinforcement learning》也登上了 Nature 封面。然而,AlphaTensor 的记录仅保持了一周,便被人类
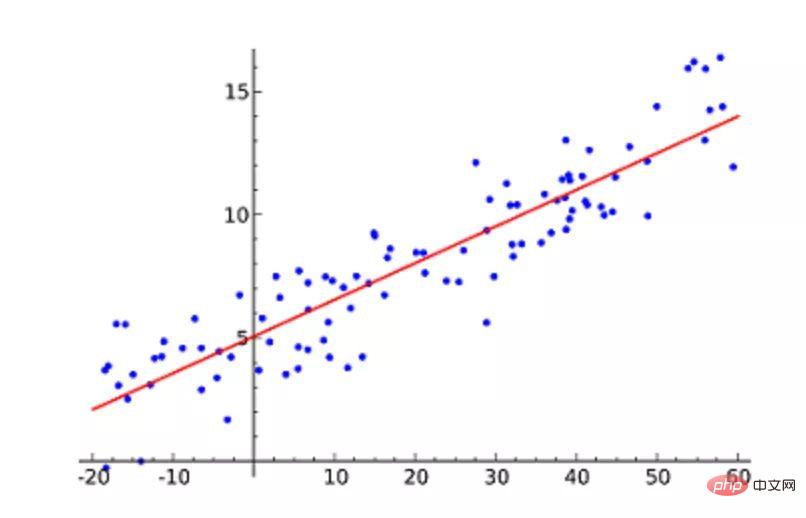
1.线性回归线性回归(Linear Regression)可能是最流行的机器学习算法。线性回归就是要找一条直线,并且让这条直线尽可能地拟合散点图中的数据点。它试图通过将直线方程与该数据拟合来表示自变量(x 值)和数值结果(y 值)。然后就可以用这条线来预测未来的值!这种算法最常用的技术是最小二乘法(Least of squares)。这个方法计算出最佳拟合线,以使得与直线上每个数据点的垂直距离最小。总距离是所有数据点的垂直距离(绿线)的平方和。其思想是通过最小化这个平方误差或距离来拟合模型。例如


Hot AI Tools

Undresser.AI Undress
AI-powered app for creating realistic nude photos

AI Clothes Remover
Online AI tool for removing clothes from photos.

Undress AI Tool
Undress images for free

Clothoff.io
AI clothes remover

AI Hentai Generator
Generate AI Hentai for free.

Hot Article

Hot Tools

mPDF
mPDF is a PHP library that can generate PDF files from UTF-8 encoded HTML. The original author, Ian Back, wrote mPDF to output PDF files "on the fly" from his website and handle different languages. It is slower than original scripts like HTML2FPDF and produces larger files when using Unicode fonts, but supports CSS styles etc. and has a lot of enhancements. Supports almost all languages, including RTL (Arabic and Hebrew) and CJK (Chinese, Japanese and Korean). Supports nested block-level elements (such as P, DIV),

Safe Exam Browser
Safe Exam Browser is a secure browser environment for taking online exams securely. This software turns any computer into a secure workstation. It controls access to any utility and prevents students from using unauthorized resources.

MinGW - Minimalist GNU for Windows
This project is in the process of being migrated to osdn.net/projects/mingw, you can continue to follow us there. MinGW: A native Windows port of the GNU Compiler Collection (GCC), freely distributable import libraries and header files for building native Windows applications; includes extensions to the MSVC runtime to support C99 functionality. All MinGW software can run on 64-bit Windows platforms.

Notepad++7.3.1
Easy-to-use and free code editor

SublimeText3 Linux new version
SublimeText3 Linux latest version
