


Recently, Jürgen Schmidhuber, the father of LSTM, had a disagreement with LeCun again!
In fact, students who were a little familiar with this grumpy old man before knew that there had been unpleasantness between the maverick Jürgen Schmidhuber and several big names in the machine learning community.
Especially when "those three people" won the Turing Award together, but Schmidhuber did not, the old man became even more angry...
After all, Schmidhuber has always believed that these current ML leaders, such as Bengio, Hinton, LeCun, including the father of "GAN" Goodfellow and others, many of their so-called "pioneering achievements" were first proposed by him. came out, and these people didn't mention him at all in the paper.
To this end, Schmidhuber once wrote a special article to criticize the review article "Deep Learning" published by Bengio, Hinton, and LeCun in Nature in 2015.
Mainly talking about the results in this article, which things were mentioned first by him, and which things were mentioned first by other seniors. Anyway, it was not the three authors who mentioned it first.
Why are they arguing again?
Going back to the cause of this incident, it was actually a tweet sent by LeCun in September.
The content is a response to Professor David Chalmers' question: "What is the most important intellectual breakthrough (new idea) in AI in the past ten years?"
On October 4th, Schmidhuber wrote an article on his blog angrily: Most of these five "best ideas" came from my laboratory, and they were proposed in a long time. Far earlier than the "10 years" time point.
In the article, Schmidhuber listed six pieces of evidence in detail to support his argument.
But probably because too few people saw it, Schmidhuber tweeted again on November 22 to stir up this "cold rice" again Read it again.
However, compared to the last time, which was quite a heated argument, LeCun didn’t even pay attention to it this time...
The father of LSTM presents "six major evidences"
1. "Self-supervised learning" that automatically generates labels through neural networks (NN):It goes back at least to my work in 1990-91.
(I) Self-supervised object generation in a recurrent neural network (RNN) via predictive coding to learn to compress data sequences at multiple time scales and levels of abstraction.
Here, an "automaton" RNN learns the pre-task of "predicting the next input" and sends unexpected observations in the incoming data stream as targets to the "analyzer" block machine" RNN, which learns higher-level regularities and subsequently refines its acquired predictive knowledge back into an automaton through appropriate training objectives.
This greatly facilitates the downstream deep learning task of sequence classification that was previously unsolvable.
(II) Self-supervised annotation generation via GAN-type intrinsic motivation, where a world model NN learns to predict adversarial, annotation generation The behavioral consequences of the experimentally invented controller NN.
In addition, the term "self-supervision" has already appeared in the title of the paper I published in 1990.
But, this word was also used in an earlier (1978) paper...
2. "ResNets": is actually the Highway Nets I proposed early on. But LeCun thinks that the intelligence of ResNets is "not deep", which makes me very sad.
Before I proposed Highway Nets, feedforward networks only had a few dozen layers (20-30 layers) at most, and Highway Nets was the first truly deep feedforward neural network, with Hundreds of layers.
In the 1990s, my LSTM brought essentially infinite depth to supervised recursive NNs. In the 2000s, LSTM-inspired Highway Nets brought depth to feedforward NNs.
As a result, LSTM has become the most cited NN in the 20th century, and Highway Nets (ResNet) is the most cited NN in the 21st century.
It can be said that they represent the essence of deep learning, and deep learning is about the depth of NN.
3. "Gating->Attention->Dynamic Connected Graph": It can be traced back to at least my Fast Weight Programmers and Key from 1991-93 -Value Memory Networks (the "Key-Value" is called "FROM-TO").
In 1993, I introduced the term "attention" as we use it today.
However, it is worth noting that the first multiplication gate in NN can be traced back to Ivakhnenko & Lapa’s deep learning machine in 1965.
4. "Differentiable memory": It can also be traced back to my Fast Weight Programmers or Key-Value Memory Networks in 1991.
Separate storage and control like in traditional computers, but in an end-to-end differential, adaptive, fully neural way (rather than in a hybrid way).
5. "Replacement equivariant module, such as multi-head self-attention->Transformer": I published it in 1991 Transformer with linearized self-attention. The corresponding term "internal spotlights of attention" dates back to 1993.
6. "GAN is the best machine learning concept in the past 10 years"
You mentioned The principle of this GAN (2014) was actually proposed by me in 1990 in the name of artificial intelligence curiosity.
The last time was a few months ago
In fact, this is no longer the relationship between Schmidhuber and LeCun There was a dispute for the first time this year.
In June and July, the two had a back-and-forth quarrel about an outlook report on the "Future Direction of Autonomous Machine Intelligence" published by LeCun.
On June 27, Yann LeCun published the paper "A Path Towards Autonomous Machine Intelligence" that he had been saving for several years, calling it "a work that points to the future development direction of AI."
This paper systematically talks about the issue of "how machines can learn like animals and humans" and is more than 60 pages long.
LeCun said that this article is not only his thoughts on the general direction of AI development in the next 5-10 years, but also what he plans to research in the next few years, and hopes to inspire the AI community. More people come to study together.
Schmidhuber learned about the news about ten days in advance, got the paper, and immediately wrote an article to refute it.
According to Schmidhuber’s own blog post, this is what happened at the time:
On June 14, 2022, a science media released news that LeCun would release a report on June 27, and sent me a draft of the report (it was still in the confidentiality period at the time), and I was asked to comment.
I wrote a review telling them that this was basically a replica of our previous work, which was not mentioned in LeCun's article.
However, my comments fell on deaf ears.
In fact, long before his article was published, we had proposed LeCun’s so-called “main original contribution” in this article. Most of the content mainly includes:
(1) "Cognitive architecture, in which all modules are separable and many modules are trainable" (we proposed it in 1990 ).
(2) "Predicting hierarchical structures of world models, learning representations at multiple abstraction levels and multiple time scales" (we proposed in 1991).
(3) "A self-supervised learning paradigm that produces representations that are simultaneously informative and predictable" (Our model has been used in reinforcement learning and world building since 1997 Modeled)
(4) Predictive model "for hierarchical planning under uncertainty", including gradient-based neural subgoal generator (1990), abstract concepts Spatial reasoning (1997), neural networks that “learn to act primarily through observation” (2015), and learning to think (2015) were all proposed by us first.
On July 14th, Yann LeCun responded, saying that discussions must be constructive. He said this:
I don’t want to get into a situation. In this useless debate about "who invented a certain concept", you don't want to delve into the 160 references listed in your response article. I think a more constructive approach would be to identify 4 publications that you think may contain ideas and methods from the 4 contributions I listed.
As I said at the beginning of the paper, there are many concepts that have been around for a long time and neither you nor I are the inventors of them: for example, the concept of fine-tunable world models , which can be traced back to early optimization control work.
Training the World Model Using neural networks to learn system recognition of world models, this idea dates back to the late 1980s, by Michael Jordan, Bernie Widrow, Robinson & Fallside, Kumpathi Narendra, Paul Werbos The work being done precedes your work.
In my opinion, this straw man answer seems to be LeCun changing the subject and avoiding the issue of taking credit for others in his so-called "main original contribution".
I replied on July 14th:
Regarding what you said about "something neither you nor I invented": your paper claims , using neural networks for system identification can be traced back to the early 1990s. However, in your previous response you seemed to agree with me that the first papers on this appeared in the 1980s.
As for your "main original contribution", they actually used the results of my early work.
(1) Regarding the "cognitive architecture in which all modules are differentiable and many modules are trainable" you proposed, "through intrinsic motivation Driving behavior":
# I proposed a differentiable architecture for online learning and planning in 1990. This was the first control with "intrinsic motivation" It is used to improve the world model, which is both generative and adversarial; the 2014 GAN cited in your article is a derivative version of this model.
(2) About your proposed "hierarchical structure of predictive world models that learn representations at multiple abstraction levels and time scales":
This was made possible by my 1991 Neural History Compressor. It uses predictive coding to learn hierarchical internal representations of long sequence data in a self-supervised manner, greatly facilitating downstream learning. Using my 1991 neural network refinement procedure, these representations can be collapsed into a single recurrent neural network (RNN).
(3) About your "self-supervised learning paradigm in control, producing representations that are both informative and predictable":
This point was made in the system I proposed to build in 1997. Rather than predicting all the details of future inputs, it can ask arbitrary abstract questions and give computable answers in what you call a "representation space." In this system, two learning models named "left brain" and "right brain" select opponents with maximum rewards to engage in zero-sum games, and occasionally bet on the results of such computational experiments.
(4) Regarding your hierarchical planning predictive differentiable model that can be used under uncertainty, your article says this:
"One unanswered question is how the configurator learns to decompose a complex task into a series of sub-goals that can be completed by the agent alone. I will leave this question to the future Investigation."
Don’t talk about the future. In fact, I published this article more than 30 years ago:
a The controller neural network is responsible for obtaining additional command inputs in the form of (start, target). An estimator neural network is responsible for learning to predict the expected cost from start to goal. A subgoal generator based on a fine-tunable recurrent neural network sees this (start, goal) input and learns a sequence of minimal-cost intermediate subgoals via gradient descent using an estimator neural network.
# (5) You also emphasized the neural network that “learns behavior mainly through observation”. We actually solved this problem very early, in this article in 2015, which discussed the general problem of reinforcement learning (RL) in partially observable environments.
World model M may be good at predicting some things but uncertain about others. Controller C maximizes its objective function by learning to query through a self-invented sequence of questions (activation patterns) and interpret answers (more activation patterns).
C can benefit from learning to extract any kind of algorithmic information from M, such as for hierarchical planning and reasoning, leveraging passive observations encoded in M, etc.
The above is the detailed content of The father of LSTM once again challenged LeCun: Your five points of 'innovation' were all copied from me! But unfortunately, 'I can't read it back'. For more information, please follow other related articles on the PHP Chinese website!
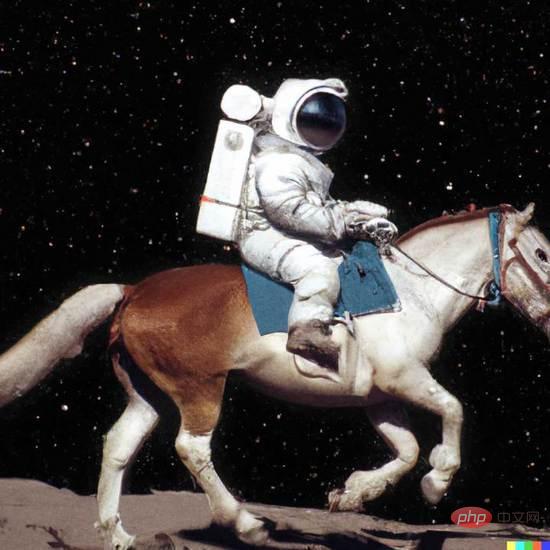
1 前言在发布DALL·E的15个月后,OpenAI在今年春天带了续作DALL·E 2,以其更加惊艳的效果和丰富的可玩性迅速占领了各大AI社区的头条。近年来,随着生成对抗网络(GAN)、变分自编码器(VAE)、扩散模型(Diffusion models)的出现,深度学习已向世人展现其强大的图像生成能力;加上GPT-3、BERT等NLP模型的成功,人类正逐步打破文本和图像的信息界限。在DALL·E 2中,只需输入简单的文本(prompt),它就可以生成多张1024*1024的高清图像。这些图像甚至
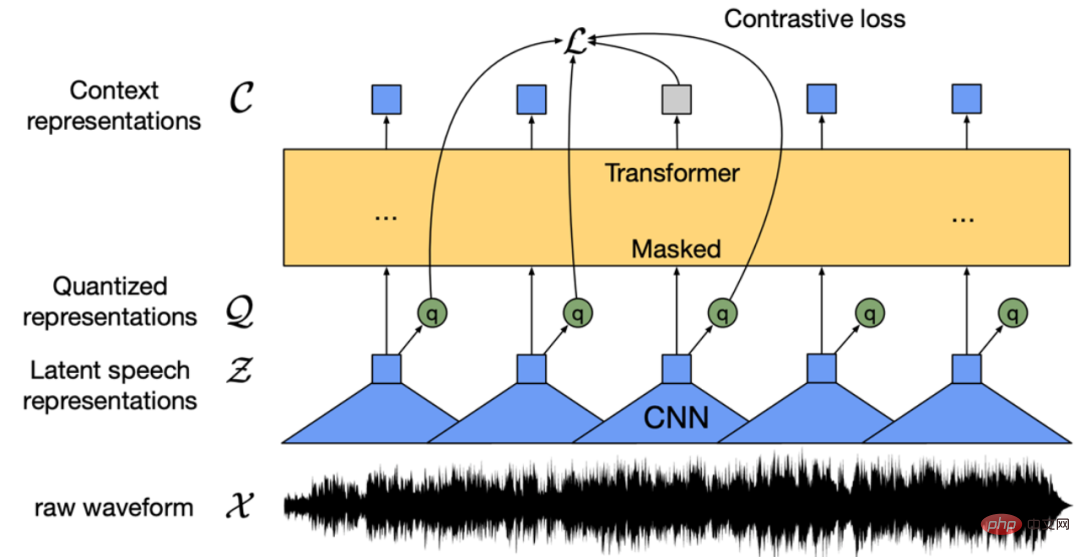
Wav2vec 2.0 [1],HuBERT [2] 和 WavLM [3] 等语音预训练模型,通过在多达上万小时的无标注语音数据(如 Libri-light )上的自监督学习,显著提升了自动语音识别(Automatic Speech Recognition, ASR),语音合成(Text-to-speech, TTS)和语音转换(Voice Conversation,VC)等语音下游任务的性能。然而这些模型都没有公开的中文版本,不便于应用在中文语音研究场景。 WenetSpeech [4] 是
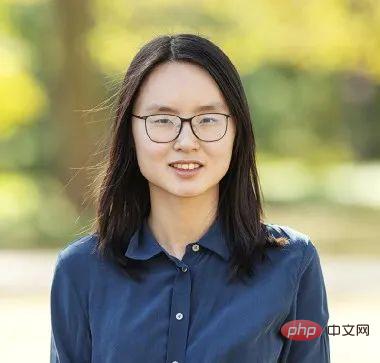
“Making large models smaller”这是很多语言模型研究人员的学术追求,针对大模型昂贵的环境和训练成本,陈丹琦在智源大会青源学术年会上做了题为“Making large models smaller”的特邀报告。报告中重点提及了基于记忆增强的TRIME算法和基于粗细粒度联合剪枝和逐层蒸馏的CofiPruning算法。前者能够在不改变模型结构的基础上兼顾语言模型困惑度和检索速度方面的优势;而后者可以在保证下游任务准确度的同时实现更快的处理速度,具有更小的模型结构。陈丹琦 普
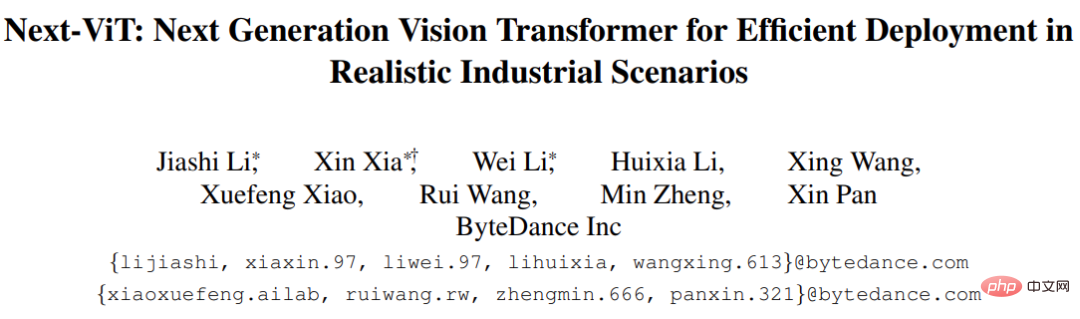
由于复杂的注意力机制和模型设计,大多数现有的视觉 Transformer(ViT)在现实的工业部署场景中不能像卷积神经网络(CNN)那样高效地执行。这就带来了一个问题:视觉神经网络能否像 CNN 一样快速推断并像 ViT 一样强大?近期一些工作试图设计 CNN-Transformer 混合架构来解决这个问题,但这些工作的整体性能远不能令人满意。基于此,来自字节跳动的研究者提出了一种能在现实工业场景中有效部署的下一代视觉 Transformer——Next-ViT。从延迟 / 准确性权衡的角度看,
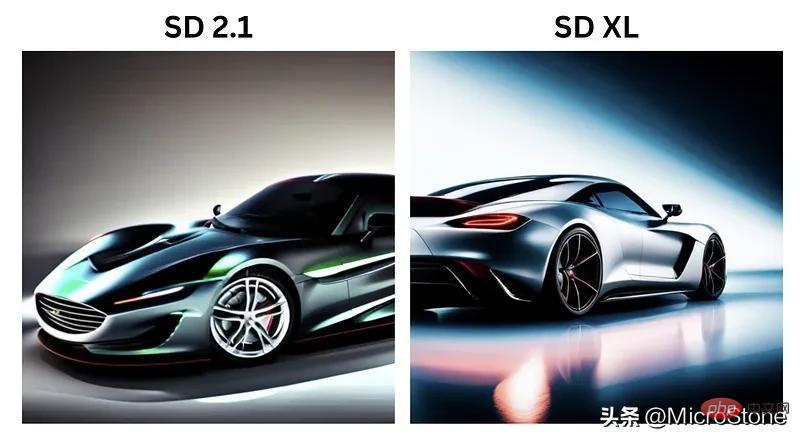
3月27号,Stability AI的创始人兼首席执行官Emad Mostaque在一条推文中宣布,Stable Diffusion XL 现已可用于公开测试。以下是一些事项:“XL”不是这个新的AI模型的官方名称。一旦发布稳定性AI公司的官方公告,名称将会更改。与先前版本相比,图像质量有所提高与先前版本相比,图像生成速度大大加快。示例图像让我们看看新旧AI模型在结果上的差异。Prompt: Luxury sports car with aerodynamic curves, shot in a
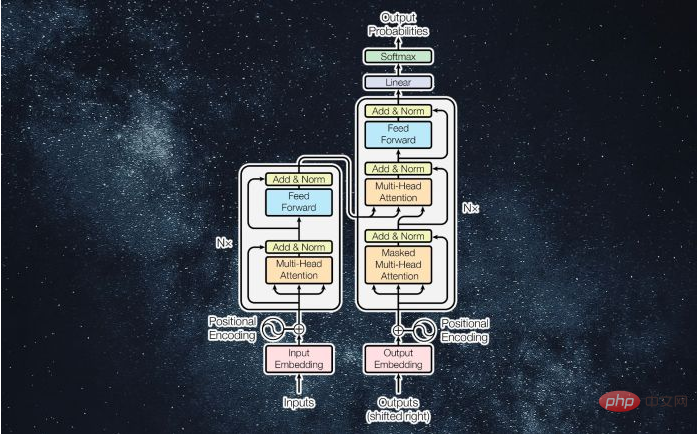
译者 | 李睿审校 | 孙淑娟近年来, Transformer 机器学习模型已经成为深度学习和深度神经网络技术进步的主要亮点之一。它主要用于自然语言处理中的高级应用。谷歌正在使用它来增强其搜索引擎结果。OpenAI 使用 Transformer 创建了著名的 GPT-2和 GPT-3模型。自从2017年首次亮相以来,Transformer 架构不断发展并扩展到多种不同的变体,从语言任务扩展到其他领域。它们已被用于时间序列预测。它们是 DeepMind 的蛋白质结构预测模型 AlphaFold
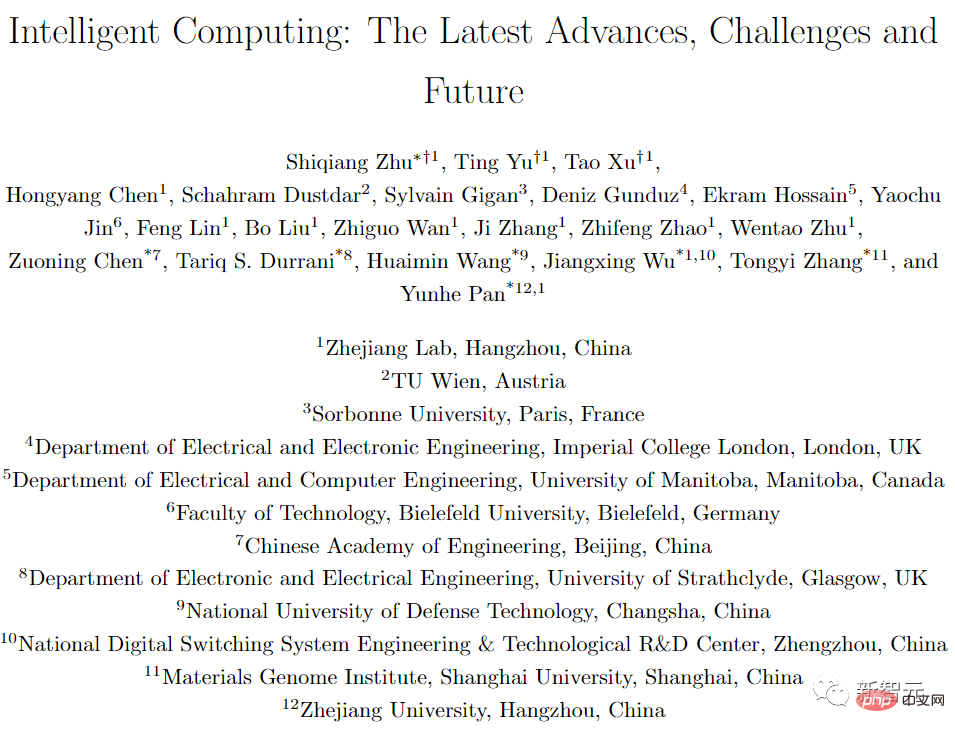
人工智能就是一个「拼财力」的行业,如果没有高性能计算设备,别说开发基础模型,就连微调模型都做不到。但如果只靠拼硬件,单靠当前计算性能的发展速度,迟早有一天无法满足日益膨胀的需求,所以还需要配套的软件来协调统筹计算能力,这时候就需要用到「智能计算」技术。最近,来自之江实验室、中国工程院、国防科技大学、浙江大学等多达十二个国内外研究机构共同发表了一篇论文,首次对智能计算领域进行了全面的调研,涵盖了理论基础、智能与计算的技术融合、重要应用、挑战和未来前景。论文链接:https://spj.scien
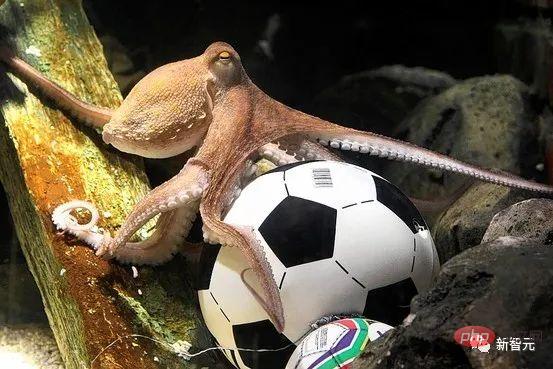
说起2010年南非世界杯的最大网红,一定非「章鱼保罗」莫属!这只位于德国海洋生物中心的神奇章鱼,不仅成功预测了德国队全部七场比赛的结果,还顺利地选出了最终的总冠军西班牙队。不幸的是,保罗已经永远地离开了我们,但它的「遗产」却在人们预测足球比赛结果的尝试中持续存在。在艾伦图灵研究所(The Alan Turing Institute),随着2022年卡塔尔世界杯的持续进行,三位研究员Nick Barlow、Jack Roberts和Ryan Chan决定用一种AI算法预测今年的冠军归属。预测模型图


Hot AI Tools

Undresser.AI Undress
AI-powered app for creating realistic nude photos

AI Clothes Remover
Online AI tool for removing clothes from photos.

Undress AI Tool
Undress images for free

Clothoff.io
AI clothes remover

AI Hentai Generator
Generate AI Hentai for free.

Hot Article

Hot Tools

Zend Studio 13.0.1
Powerful PHP integrated development environment

MantisBT
Mantis is an easy-to-deploy web-based defect tracking tool designed to aid in product defect tracking. It requires PHP, MySQL and a web server. Check out our demo and hosting services.
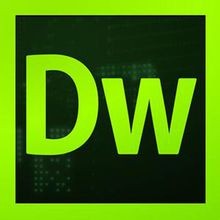
Dreamweaver CS6
Visual web development tools

Safe Exam Browser
Safe Exam Browser is a secure browser environment for taking online exams securely. This software turns any computer into a secure workstation. It controls access to any utility and prevents students from using unauthorized resources.

PhpStorm Mac version
The latest (2018.2.1) professional PHP integrated development tool