1. Problem background
1. Introduction to bilateral market experiment
A two-sided market, that is, a platform, includes two participants, producers and consumers, and both parties promote each other. For example, Kuaishou has a video producer and a video consumer, and the two identities may overlap to a certain extent.
#Bilateral experiment is an experimental method that combines groups on the producer and consumer sides.
Bilateral experiments have the following advantages:
(1) The impact of new strategies on two aspects can be detected at the same time, such as product DAU and uploaded works Number of people changes. Bilateral platforms often have cross-side network effects. The more readers there are, the more active the authors will be, and the more active the authors will be, the more readers will follow.
#(2) Can detect effect overflow and transfer.
(3) Help us better understand the mechanism of action. The AB experiment itself cannot tell us the relationship between the cause and the result, but can only tell us what has been done. What impact will things draw and how the data changes. However, the mechanism of action between the production end and the consumer end requires more complex experimental designs and more experimental indicators to clearly understand these issues.
2. Examples of bilateral experiments
# #This uses an example of live beauty to help everyone further understand the bilateral experiment.
Assume that the beauty effect is added to the live broadcast scene. Looking sideways from the table, the experimental audience groups in the two rows control whether the audience can see the difference before and after the live beauty treatment. The columns in the table represent the actual impact of whether the anchor has beauty or not. Combining the above two aspects, the beautification function will be enabled for the video if and only when the anchor of the experimental group compares with the audience of the experimental group. In fact, the other three groups cannot see the beauty function. But there is a difference between BC not seeing beauty and D not seeing beauty. AD distinction is a common scenario in regular AB experiments. This scene uses a bilateral design to observe whether there is overflow on the audience side.
As for the anchor beauty, there is no beautification function. If there is no audience overflow, the BD data should be consistent, but in fact, if there are differences in the data BD, if The anchor does not have a beauty function. If the audience sees the beauty function on other anchors, the actual effect will have a positive or negative impact. In the same way, overflow on the anchor side can also be done through this kind of bilateral experiment to better understand the mechanism of the experiment and whether there is overflow on both sides of the experiment.
2. Challenges of incentive strategies
# #In the supply-side-consumer-side ecosystem, business duration requires policy traffic support. This is the incentive strategy, which mainly includes the following three scenarios:
(1) The operation introduces high-quality authors, but the data performance of the authors on the platform is not sure;
(2) Some businesses need to mine specific types of authors to Some macro-control traffic support will be given to strengthen traffic distribution;
#(3) Under the platform’s will scenario, it is considered that the platform will develop in a certain direction. Change the traffic distribution method to strengthen the supply of certain corresponding content. In the above scenarios, it is often not a method of online learning, but a macro-control of platform traffic from a human perspective. For those that focus on the relatively long term, it is necessary to observe the learning effect (promoting production, etc.), and methods such as time slice rotation are not tried. For example, the following scenario: provide traffic support to authors with a type of directional traffic to study whether the interaction and production of such traffic can last long in a long-term scenario.
The first is crowding on the author side: most of these experiments, the total exposure of the platform The number is limited, and under the scenario of platform support, the exposure of authors in the experimental group increases, while the exposure of the unsupported control group decreases. If the author's cold start exposure increases more than the reader's cold start exposure, it proves that there is crowding.
According to the above figure, based on the relationship between the experimental group and the control group and the relative baseline diff of each group's exposure, it can be seen that as the experiment begins, the author's boost will eventually pass The recommendation system not only passes it to user group B but also to user group A, and the exposure diffs of author B, user B, author B, and user A are basically consistent. Traditional experiments have been devoted to correcting the traffic situation distorted by this strategy.
SUTVA Assume that individual i is only assigned to the experimental group with itself during the experiment Or it is related to the control group and has nothing to do with which group the other nodes are in under the experimental system, regardless of whether the other nodes are in a cooperative or competitive relationship. SUTVA is the most basic assumption for obtaining effective conclusions in AB experiments.
#The actual bilateral network violates the SUTVA assumption.
##In the short video scenario, if each recording strategy is regarded as a Sorting Algorithm. Different incentive strategies represent different ranking results of short videos. RC in the above figure represents the control group, RT_25% is the algorithm sorting combination when the experimental group traffic is 25%, and RT represents the experimental group's experimental push of 100% algorithm sorting combination. BCDE is the experimental target user type, that is, the selected incentive author works. And D means that when the experimental inference is 25%, it falls exactly in the experimental group. Suppose that through the recommendation weighting method, D is ranked directly to the front position. If the strategy increases to 100%, BCDE will be weighted. In this case, the ranking of D works will decrease. This scenario is the crowding of the experimental group and the reason for the crowding.
3. Optional solutions
1. Option 1: Gradually expand
The sorting gap of the experimental group will gradually approach as the data proportion of the experimental group expands, and the squeeze-out effect will decrease as the traffic of the control group decreases.
#[First Mover Advantage] During the experiment, it was found that in the scenario of traffic support, with equal support intensity, supporting the author first will always maintain the traffic advantage. The logic of earlier support and accelerated excavation process is consistent.
Experimental details of phased expansion: The above figure shows the phased expansion, and the ordinate is the difference in powder growth data relative to the base group. At the beginning of the experiment, 20% of the experimental group only supported experimental group 1, and the data indicators of experimental group 1 began to rise; when the experiment increased to 60%, experimental groups 123 began to support, and the experimental indicators of the other two groups also began to rise, but there was still no Exceeded experimental group 1; later changed the experimental group to 124, and found that 4 also began to improve, but 4 still could not surpass experimental group 3. The following conclusions can be drawn from this: Gradual expansion is useful. The indicator will increase according to the expansion. It is impossible to confirm whether the increase will become smaller as the traffic expands. The current experimental results can be concluded that the data performance of the experimental group that received traffic support first will be better than that of the experimental group that received traffic support later. 2. Plan 2: Divide the small world
As shown in the above figure, the experimental group and the control group are completely isolated. Readers in the experimental group can only see the works of the experimental group, and readers in the control group can only see the works of the control group. This avoids a squeeze between author and reader.
A similar approach is to treat the traffic distribution between authors and readers as a network diagram. This network diagram is not connected everywhere, and some readers only like to read some parts. Based on this kind of work, the experimental group and the control group can be segmented based on such a network diagram. The above approach is consistent with the method of dividing small worlds and has better practical results, but at the same time it also has greater computational costs.
The main problems in dividing the small world are:
(1) The algorithm recommendation system requires certain A cold start can only be made if the size of the system is of the order of magnitude. When the sharding pool must be small, it will affect the actual personalized distribution space. Different businesses and different platforms have different requirements for the finest granularity of the segmentation structure under the premise of retaining the flexible effect of recommendations. In most cases, diminishing marginal effects are recommended.
# (2) Clear traffic isolation will have certain restrictions on the number of experiments and inspection methods for samples. For parallel experiment scenarios, isolated users need to be constantly reorganized and re-split.
Correction from the analysis method rather than the experimental design method:
- according to Perform correction analysis on the actual network effect;
- make some linear assumptions and other conditional assumptions based on the experimental results.
The reasons for using experimental correction:
First of all, the assumptions in the actual analysis correction method are difficult to verify, and for large differences In experiments, the spillover and crowding out of network effects vary, and it is difficult to summarize the rules in a short period of time, and it is impossible to obtain a general method. In fact, our solution hopes to solve a large class of problems.
4. Build a comprehensive plan
## Solution construction based on ranking fusion - essentially we hope to ensure that the ranking of experimental group RT_a% and the actual ranking of experimental group RT_100% can maintain consistent results.
Implementation method: First, use two sets of RT/RC sorting algorithms to sort at the same time, and record the corresponding order of works; divide the authors into experimental groups and control groups, For the experimental group, the readers are shown the sorting and fusion order of the two algorithms.
Regard RC as an online sorting solution currently unsupported by all authors, in RT Elevate the rights of all knowledge authors. To fuse the sorting results of RC and RT, first place the authors (T1T2) corresponding to the RT of the experimental group in the corresponding sorting position of the final group, and keep the authors of the control group in an order unrelated to the original experiment. To be conservative, during the period of low traffic, it is recommended that except for experimental works, other works should be filled in the original order. If the experiment has been extrapolated, the RT results will be used in full.
What if the experimental group and the control group compete for the same position?
According to the above experimental design, if the works of the experimental group and the control group compete for the same position, the simplest way is to select randomly. The probability of this happening is very low.
If the experimental group and the control group both have a% of the total traffic, assuming a=2,
Assuming that 10 works are promoted at a time, the probability that the top 10 works from both the experimental group and the control group will appear is calculated as shown above, which is about 3.3%. If the two algorithms are completely independent, the probability of conflict in the same top 10 positions is lower.
Often improvements are gradual, with RC and RT highly correlated and less conflicting. At the same time, the probability of conflicts can also be estimated in advance through offline testing.
The main indicator evaluations of the above bilateral experiments can be divided into the following three categories:
- Author-side indicators: number of works, number of production authors, directly from the author Side-check;
- Report viewing volume indicators: CTR, EVTR, the increase in the exposure of the author's work = the increase in the number of reader views;
- Reader-side indicators: reader-side unilateral experimental verification.
##The solution may have some other problems:
First of all, there will be problems with any plan. The strong spillover effects of two-sided markets make it difficult to solve all problems with one solution.
The main issues in current experimental design include the following aspects:
(1 ) First of all, there is a certain cost from the engineering side to retaining two sets of sorting. If policy incentives are provided, it will be better promoted. From an algorithmic point of view, it is not easy to keep the two sets without fusion;
(2) Secondly, from the perspective of algorithm data isolation, part of the improvement comes from the data itself. There are major changes in the model itself, and as a result, the logic of the sorting algorithm no longer holds.
#(3) Third, the calculation assumes a=2%. Can the value of a be increased if more traffic is used to test small effects? Randomly select proportional mixing to make the possibility of larger traffic conflicts less likely. Finally, bilateral issues will be resolved unilaterally. Whether they can be resolved bilaterally will be explored in the future.
The above is the detailed content of Complex experimental design issues in Kuaishou's two-sided market. For more information, please follow other related articles on the PHP Chinese website!
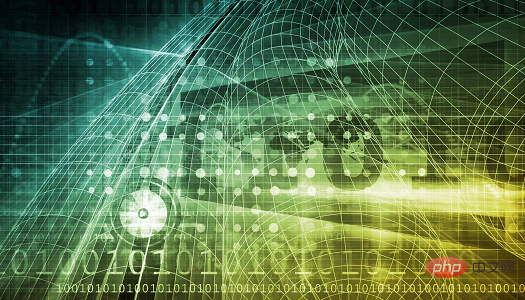
一、问题背景1、双边市场实验介绍双边市场,即平台,包含生产者与消费者两方参与者,双方相互促进。比如快手有视频的生产者,视频的消费者,两种身份可能存在一定程度重合。双边实验是在生产者和消费者端组合分组的实验方式。双边实验具有以下优点:(1)可以同时检测新策略对两方面的影响,例如产品DAU和上传作品人数变化。双边平台往往有跨边网络效应,读者越多,作者越活跃,作者越活跃,读者也会跟着增加。(2)可以检测效果溢出和转移。(3)帮助我们更好得理解作用的机制,AB实验本身不能告诉我们原因和结果之间的关系,只
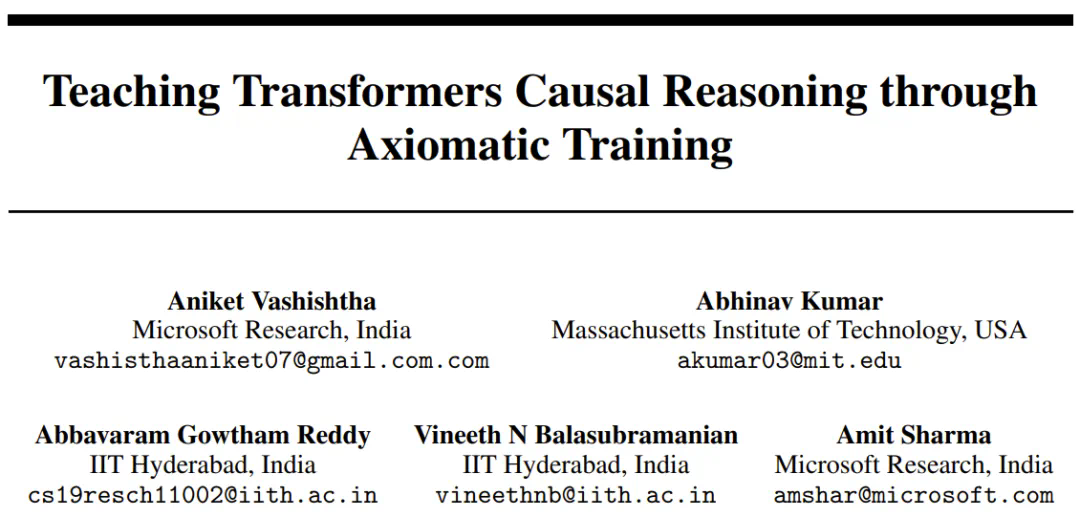
把因果链展示给LLM,它就能学会公理。AI已经在帮助数学家和科学家做研究了,比如著名数学家陶哲轩就曾多次分享自己借助GPT等AI工具研究探索的经历。AI要在这些领域大战拳脚,强大可靠的因果推理能力是必不可少的。本文要介绍的这项研究发现:在小图谱的因果传递性公理演示上训练的Transformer模型可以泛化用于大图谱的传递性公理。也就是说,如果让Transformer学会执行简单的因果推理,就可能将其用于更为复杂的因果推理。该团队提出的公理训练框架是一种基于被动数据来学习因果推理的新范式,只有演示
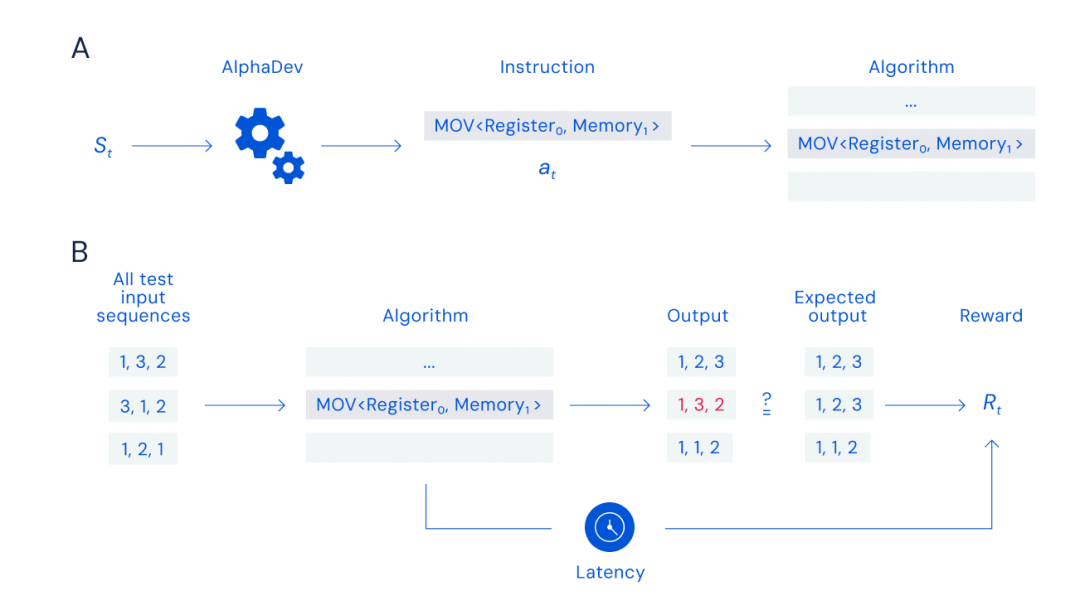
整理|核子可乐,褚杏娟接触过基础计算机科学课程的朋友们,肯定都曾亲自动手设计排序算法——也就是借助代码将无序列表中的各个条目按升序或降序方式重新排列。这是个有趣的挑战,可行的操作方法也多种多样。人们曾投入大量时间探索如何更高效地完成排序任务。作为一项基础操作,大多数编程语言的标准库中都内置有排序算法。世界各地的代码库中使用了许多不同的排序技术和算法来在线组织大量数据,但至少就与LLVM编译器配套使用的C++库而言,排序代码已经有十多年没有任何变化了。近日,谷歌DeepMindAI小组如今开发出一
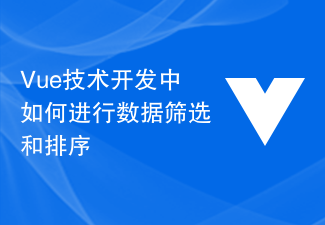
Vue技术开发中如何进行数据筛选和排序在Vue技术开发中,数据筛选和排序是非常常见和重要的功能。通过数据筛选和排序,我们可以快速查询和展示我们需要的信息,提高用户体验。本文将介绍在Vue中如何进行数据筛选和排序,并提供具体的代码示例,帮助读者更好地理解和运用这些功能。一、数据筛选数据筛选是指根据特定的条件筛选出符合要求的数据。在Vue中,我们可以通过comp
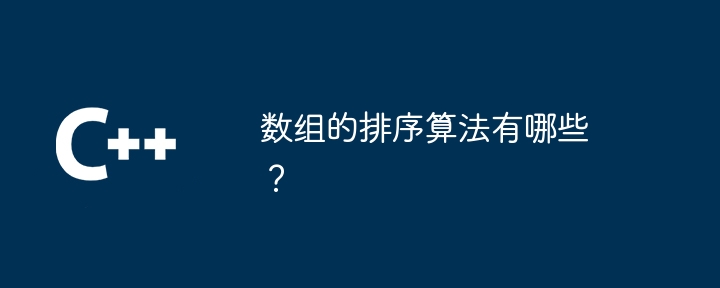
数组排序算法用于按特定顺序排列元素。常见的算法类型包括:冒泡排序:通过比较相邻元素交换位置。选择排序:找出最小元素交换到当前位置。插入排序:逐个插入元素到正确位置。快速排序:分治法,选择枢纽元素划分数组。合并排序:分治法,递归排序和合并子数组。
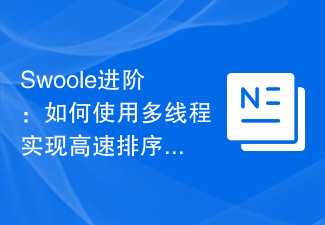
Swoole是一款基于PHP语言的高性能网络通信框架,它支持多种异步IO模式和多种高级网络协议的实现。在Swoole的基础上,我们可以利用其多线程功能实现高效的算法运算,例如高速排序算法。高速排序算法(QuickSort)是一种常见的排序算法,通过定位一个基准元素,将元素分为两个子序列,小于基准元素的放在左侧,大于等于基准元素的放在右侧,再对左右子序列递归
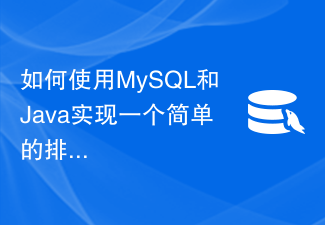
如何使用MySQL和Java实现一个简单的排序算法功能导言:在软件开发中,排序算法是非常基础且常用的功能之一。本文将介绍如何使用MySQL和Java实现一个简单的排序算法功能,并提供具体代码示例。一、排序算法概述排序算法是将一组数据按照特定规则进行排列的算法,常用的排序算法有冒泡排序、插入排序、选择排序、快速排序等。本文将以冒泡排序为例进行讲解及实现。二、M
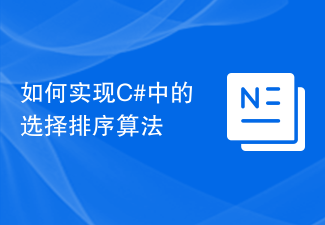
如何实现C#中的选择排序算法选择排序(SelectionSort)是一种简单直观的排序算法,其基本思想是每次从待排序元素中选择最小(或最大)的元素,放到已排序的序列末尾。通过重复这个过程,直到所有元素都排序完成。下面我们来详细了解如何在C#中实现选择排序算法,同时附上具体的代码示例。创建选择排序方法首先,我们需要创建一个用于实现选择排序的方法。该方法接受一


Hot AI Tools

Undresser.AI Undress
AI-powered app for creating realistic nude photos

AI Clothes Remover
Online AI tool for removing clothes from photos.

Undress AI Tool
Undress images for free

Clothoff.io
AI clothes remover

AI Hentai Generator
Generate AI Hentai for free.

Hot Article

Hot Tools

SublimeText3 Chinese version
Chinese version, very easy to use

mPDF
mPDF is a PHP library that can generate PDF files from UTF-8 encoded HTML. The original author, Ian Back, wrote mPDF to output PDF files "on the fly" from his website and handle different languages. It is slower than original scripts like HTML2FPDF and produces larger files when using Unicode fonts, but supports CSS styles etc. and has a lot of enhancements. Supports almost all languages, including RTL (Arabic and Hebrew) and CJK (Chinese, Japanese and Korean). Supports nested block-level elements (such as P, DIV),

SublimeText3 Linux new version
SublimeText3 Linux latest version

MantisBT
Mantis is an easy-to-deploy web-based defect tracking tool designed to aid in product defect tracking. It requires PHP, MySQL and a web server. Check out our demo and hosting services.

SAP NetWeaver Server Adapter for Eclipse
Integrate Eclipse with SAP NetWeaver application server.
