In order to make artificial intelligence more ethically sound and practical, it is crucial to enhance the interpretability of deep neural networks.
Transparency around AI efforts can cause headaches for organizations integrating the technology into their daily operations. So what can be done to allay concerns about the need for explainable AI?
The profound benefits of AI in any industry are well known. We realize how this technology is helping thousands of businesses around the world speed up their operations and use their employees more imaginatively. Additionally, the long-term costs and data security benefits of AI have been documented countless times by technology columnists and bloggers. However, artificial intelligence does have its fair share of problems. One of the problems is that the technology's decision-making is sometimes questionable. But more importantly, the bigger issue is the slight lack of explainability whenever AI-driven systems go wrong in embarrassing or catastrophic ways.
Humans make mistakes every day. However, we know exactly how errors arise. A clear set of corrective actions can be taken to avoid the same mistakes in the future. However, some errors in AI are unexplainable because data experts have no idea how the algorithm reaches a specific conclusion in its operation. Therefore, explainable AI should be a top priority for both organizations planning to implement the technology into their daily work and those already incorporating it.
What makes artificial intelligence explainable
A common fallacy about artificial intelligence is that it is completely infallible. Neural networks, especially in their early stages, can make mistakes. At the same time, these networks carry out their orders in a non-transparent manner. As mentioned earlier, the path an AI model takes to reach a specific conclusion is not clear at any point during its operation. Therefore, it is almost impossible for even experienced data experts to explain such errors.
The issue of transparency in artificial intelligence is particularly acute in the healthcare industry. Consider this example: A hospital has a neural network or a black box AI model that diagnoses a patient’s brain disease. The intelligent system is trained to look for patterns in data from past records and patients' existing medical files. With predictive analytics, if a model predicts that a subject will be susceptible to a brain-related disease in the future, the reasons behind the prediction are often not 100 percent clear. For both private and public institutions, here are 4 main reasons to make AI efforts more transparent:
1. Accountability
As mentioned before, stakeholders need to know about AI models The inner workings and reasoning behind the decision-making process, especially for unexpected recommendations and decisions. An explainable AI system can ensure that algorithms make fair and ethical recommendations and decisions in the future. This can increase compliance and trust in AI neural networks within organizations.
2. Greater Control
Explainable artificial intelligence can often prevent system errors from occurring in work operations. More knowledge about existing weaknesses in AI models can be used to eliminate them. As a result, organizations have greater control over the output provided by AI systems.
3. Improvement
As we all know, artificial intelligence models and systems require continuous improvement from time to time. Explainable AI algorithms will become smarter during regular system updates.
4. New discoveries
New information clues will enable mankind to discover solutions to major problems of the current era, such as drugs or therapies to treat HIV AIDS and methods to deal with attention deficit disorder. What's more, these findings will be backed up by solid evidence and a rationale for universal verification.
In AI-driven systems, transparency can be in the form of analytical statements in natural language that humans can understand, visualizations that highlight the data used to make output decisions, and visualizations that show the points that support a given decision. Cases, or statements that highlight why the system rejected other decisions.
In recent years, the field of explainable artificial intelligence has developed and expanded. Most importantly, if this trend continues in the future, businesses will be able to use explainable AI to improve their output while understanding the rationale behind every critical AI-powered decision.
While these are reasons why AI needs to be more transparent, there are some obstacles that prevent the same from happening. Some of these obstacles include:
AI Responsibility Paradox
It is known that explainable AI can improve aspects such as fairness, trust, and legitimacy of AI systems. However, some organizations may be less keen on increasing the accountability of their intelligent systems, as explainable AI could pose a host of problems. Some of these issues are:
Stealing important details of how the AI model operates.
The threat of cyberattacks from external entities due to increased awareness of system vulnerabilities.
Beyond that, many believe that exposing and disclosing confidential decision-making data in AI systems leaves organizations vulnerable to lawsuits or regulatory actions.
To not fall victim to this “transparency paradox,” companies must consider the risks associated with explainable AI versus its clear benefits. Businesses must effectively manage these risks while ensuring that the information generated by explainable AI systems is not diluted.
Additionally, companies must understand two things: First, the costs associated with making AI transparent should not prevent them from integrating such systems. Businesses must develop risk management plans that accommodate interpretable models so that the critical information they provide remains confidential. Second, businesses must improve their cybersecurity frameworks to detect and neutralize vulnerabilities and cyber threats that could lead to data breaches.
The black box problem of artificial intelligence
Deep learning is an integral part of artificial intelligence. Deep learning models and neural networks are often trained in an unsupervised manner. Deep learning neural networks are a key component of artificial intelligence involved in image recognition and processing, advanced speech recognition, natural language processing and system translation. Unfortunately, while this AI component can handle more complex tasks than conventional machine learning models, deep learning also introduces black box issues into everyday operations and tasks.
As we know, neural networks can replicate the work of the human brain. The structure of artificial neural network is to imitate real neural network. Neural networks are created from several layers of interconnected nodes and other "hidden" layers. While these neural nodes perform basic logical and mathematical operations to draw conclusions, they are also smart and intuitive enough to process historical data and generate results from it. Really complex operations involve multiple neural layers and billions of mathematical variables. Therefore, the output generated from these systems has little chance of being fully verified and validated by AI experts in the organization.
Organizations like Deloitte and Google are working to create tools and digital applications that break through the black boxes and reveal the data used to make critical AI decisions to increase transparency in intelligent systems.
To make AI more accountable, organizations must reimagine their existing AI governance strategies. Here are some key areas where improved governance can reduce transparency-based AI issues.
System Design
In the initial stages, organizations can prioritize trust and transparency when building AI systems and training neural networks. Paying close attention to how AI service providers and vendors design AI networks can alert key decision-makers in the organization to early questions about the capabilities and accuracy of AI models. In this way, there is a hands-on approach to revealing some of the transparency-based issues with AI during the system design phase for organizations to observe.
Compliance
As AI regulations around the world become increasingly stringent when it comes to AI responsibilities, organizations can truly benefit from having their AI models and systems comply with these norms and standards. Benefit. Organizations must push their AI vendors to create explainable AI systems. To eliminate bias in AI algorithms, businesses can approach cloud-based service providers instead of hiring expensive data experts and teams. Organizations must ease the compliance burden by clearly instructing cloud service providers to tick all compliance-related boxes during the installation and implementation of AI systems in their workplaces. In addition to these points, organizations can also include points such as privacy and data security in their AI governance plans.
We have made some of the most astounding technological advances since the turn of the century, including artificial intelligence and deep learning. Fortunately, although 100% explainable AI does not yet exist, the concept of AI-powered transparent systems is not an unattainable dream. It’s up to organizations implementing these systems to improve their AI governance and take the risks to achieve this.
The above is the detailed content of Is it possible to make artificial intelligence more transparent?. For more information, please follow other related articles on the PHP Chinese website!
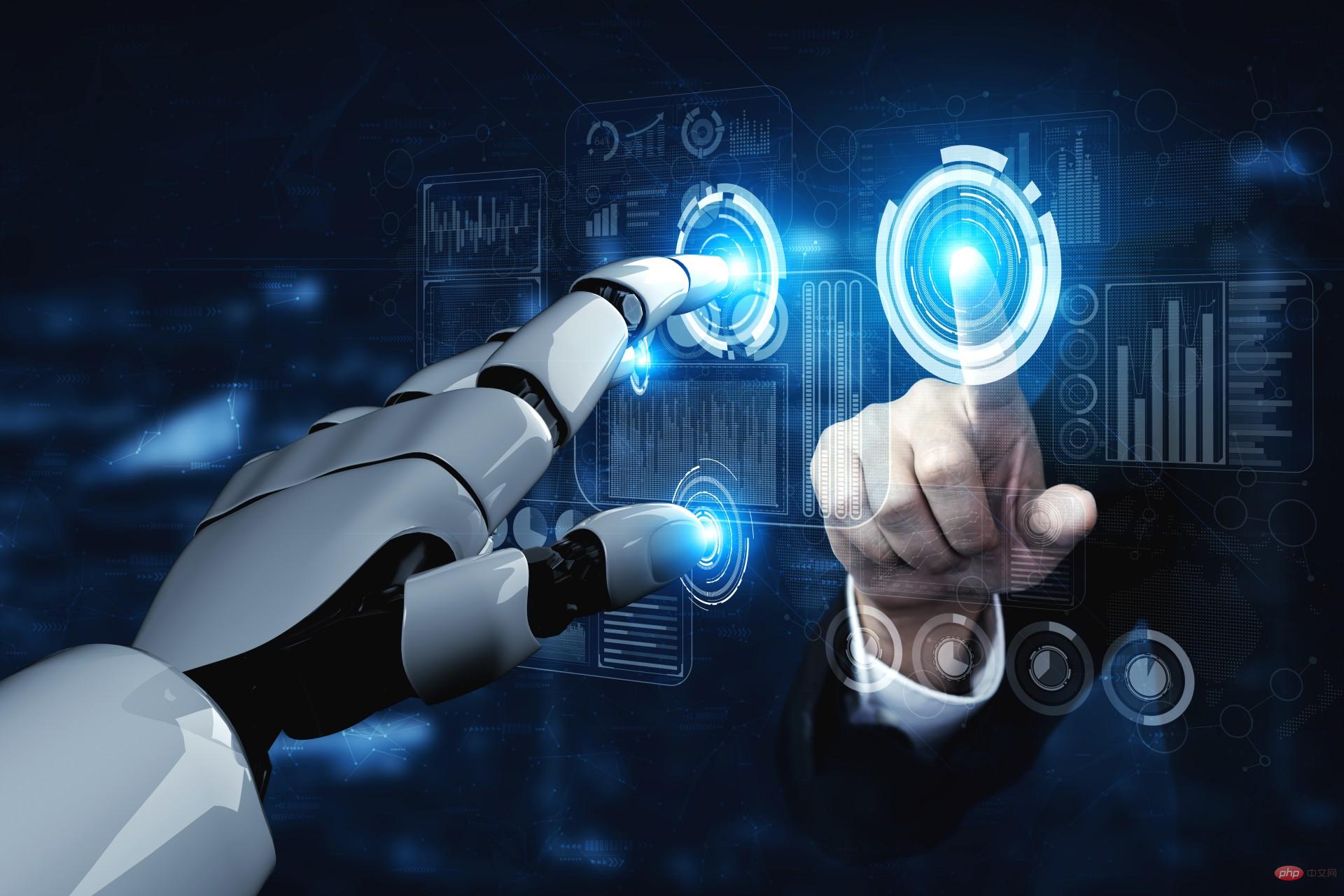
机器学习是一个不断发展的学科,一直在创造新的想法和技术。本文罗列了2023年机器学习的十大概念和技术。 本文罗列了2023年机器学习的十大概念和技术。2023年机器学习的十大概念和技术是一个教计算机从数据中学习的过程,无需明确的编程。机器学习是一个不断发展的学科,一直在创造新的想法和技术。为了保持领先,数据科学家应该关注其中一些网站,以跟上最新的发展。这将有助于了解机器学习中的技术如何在实践中使用,并为自己的业务或工作领域中的可能应用提供想法。2023年机器学习的十大概念和技术:1. 深度神经网

本文将详细介绍用来提高机器学习效果的最常见的超参数优化方法。 译者 | 朱先忠审校 | 孙淑娟简介通常,在尝试改进机器学习模型时,人们首先想到的解决方案是添加更多的训练数据。额外的数据通常是有帮助(在某些情况下除外)的,但生成高质量的数据可能非常昂贵。通过使用现有数据获得最佳模型性能,超参数优化可以节省我们的时间和资源。顾名思义,超参数优化是为机器学习模型确定最佳超参数组合以满足优化函数(即,给定研究中的数据集,最大化模型的性能)的过程。换句话说,每个模型都会提供多个有关选项的调整“按钮
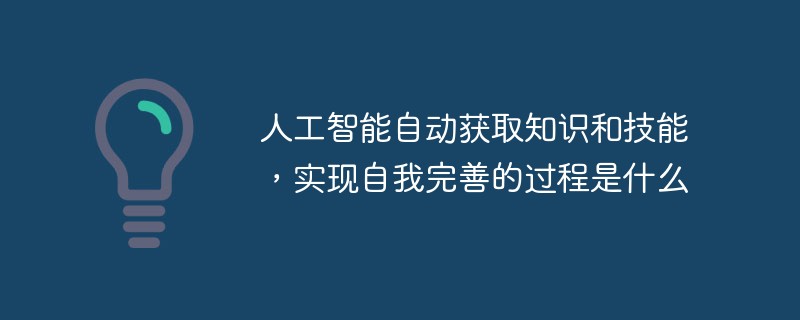
实现自我完善的过程是“机器学习”。机器学习是人工智能核心,是使计算机具有智能的根本途径;它使计算机能模拟人的学习行为,自动地通过学习来获取知识和技能,不断改善性能,实现自我完善。机器学习主要研究三方面问题:1、学习机理,人类获取知识、技能和抽象概念的天赋能力;2、学习方法,对生物学习机理进行简化的基础上,用计算的方法进行再现;3、学习系统,能够在一定程度上实现机器学习的系统。
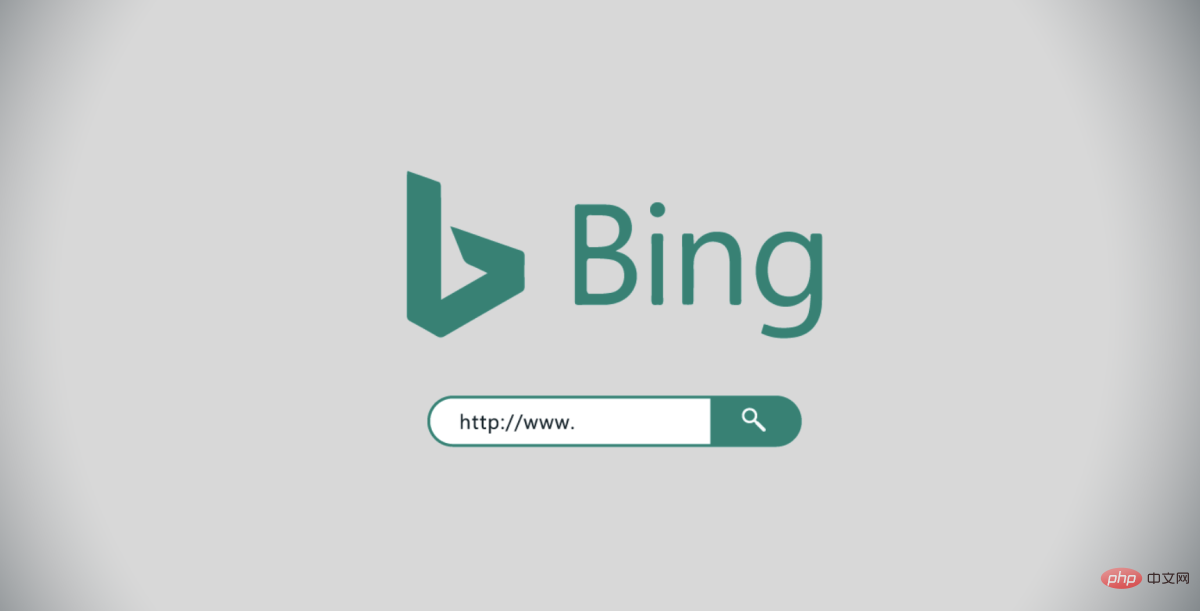
截至3月20日的数据显示,自微软2月7日推出其人工智能版本以来,必应搜索引擎的页面访问量增加了15.8%,而Alphabet旗下的谷歌搜索引擎则下降了近1%。 3月23日消息,外媒报道称,分析公司Similarweb的数据显示,在整合了OpenAI的技术后,微软旗下的必应在页面访问量方面实现了更多的增长。截至3月20日的数据显示,自微软2月7日推出其人工智能版本以来,必应搜索引擎的页面访问量增加了15.8%,而Alphabet旗下的谷歌搜索引擎则下降了近1%。这些数据是微软在与谷歌争夺生
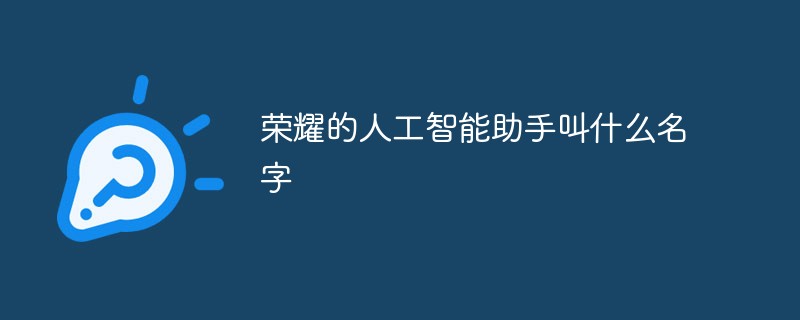
荣耀的人工智能助手叫“YOYO”,也即悠悠;YOYO除了能够实现语音操控等基本功能之外,还拥有智慧视觉、智慧识屏、情景智能、智慧搜索等功能,可以在系统设置页面中的智慧助手里进行相关的设置。
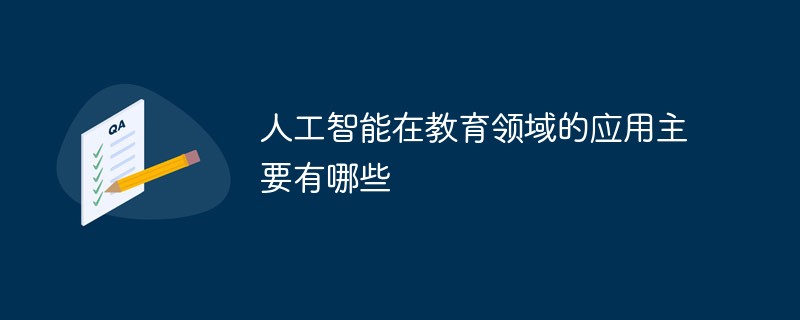
人工智能在教育领域的应用主要有个性化学习、虚拟导师、教育机器人和场景式教育。人工智能在教育领域的应用目前还处于早期探索阶段,但是潜力却是巨大的。
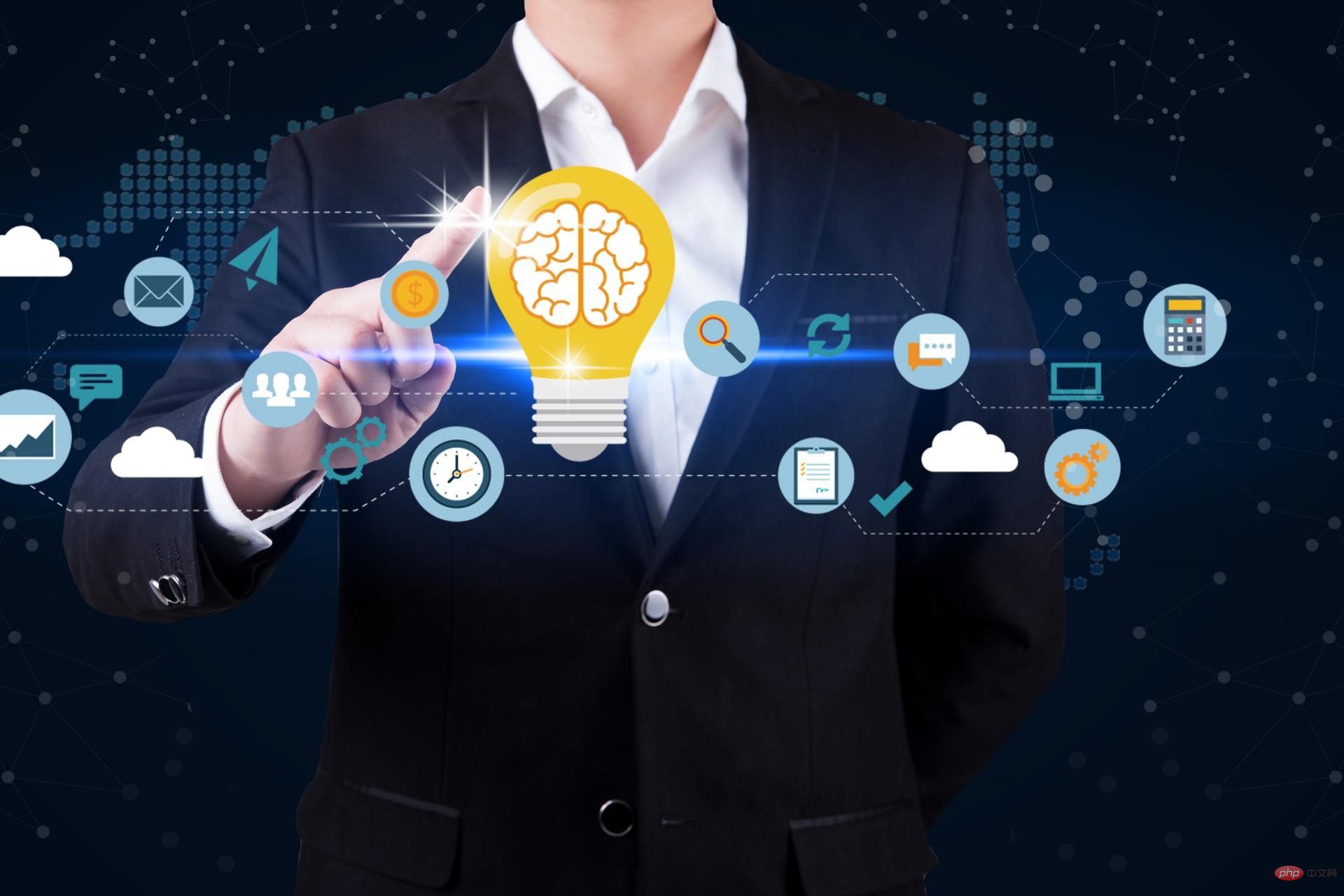
阅读论文可以说是我们的日常工作之一,论文的数量太多,我们如何快速阅读归纳呢?自从ChatGPT出现以后,有很多阅读论文的服务可以使用。其实使用ChatGPT API非常简单,我们只用30行python代码就可以在本地搭建一个自己的应用。 阅读论文可以说是我们的日常工作之一,论文的数量太多,我们如何快速阅读归纳呢?自从ChatGPT出现以后,有很多阅读论文的服务可以使用。其实使用ChatGPT API非常简单,我们只用30行python代码就可以在本地搭建一个自己的应用。使用 Python 和 C
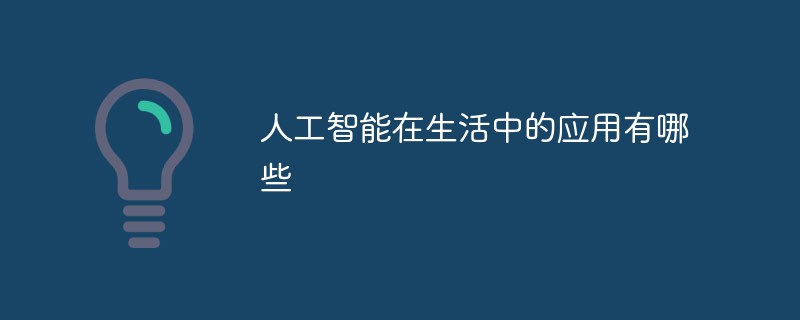
人工智能在生活中的应用有:1、虚拟个人助理,使用者可通过声控、文字输入的方式,来完成一些日常生活的小事;2、语音评测,利用云计算技术,将自动口语评测服务放在云端,并开放API接口供客户远程使用;3、无人汽车,主要依靠车内的以计算机系统为主的智能驾驶仪来实现无人驾驶的目标;4、天气预测,通过手机GPRS系统,定位到用户所处的位置,在利用算法,对覆盖全国的雷达图进行数据分析并预测。


Hot AI Tools

Undresser.AI Undress
AI-powered app for creating realistic nude photos

AI Clothes Remover
Online AI tool for removing clothes from photos.

Undress AI Tool
Undress images for free

Clothoff.io
AI clothes remover

AI Hentai Generator
Generate AI Hentai for free.

Hot Article

Hot Tools

PhpStorm Mac version
The latest (2018.2.1) professional PHP integrated development tool

MantisBT
Mantis is an easy-to-deploy web-based defect tracking tool designed to aid in product defect tracking. It requires PHP, MySQL and a web server. Check out our demo and hosting services.

SublimeText3 Linux new version
SublimeText3 Linux latest version

SecLists
SecLists is the ultimate security tester's companion. It is a collection of various types of lists that are frequently used during security assessments, all in one place. SecLists helps make security testing more efficient and productive by conveniently providing all the lists a security tester might need. List types include usernames, passwords, URLs, fuzzing payloads, sensitive data patterns, web shells, and more. The tester can simply pull this repository onto a new test machine and he will have access to every type of list he needs.
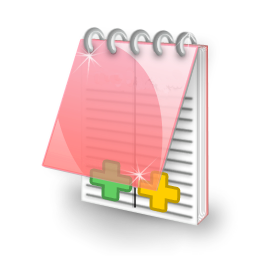
EditPlus Chinese cracked version
Small size, syntax highlighting, does not support code prompt function
