


Many studies have found that AI is so ashamed that it has learned to discriminate against people.
How can this be done?
Recently, a study by Tsinghua & Fudan gave suggestions for this:
If you want to drag AI back from the road of sexism, a scolding will not be effective. .
The best way is to understand why the child is like this, and then prescribe the right medicine to reason with him.
Because if you just teach without being reasonable and correct violently, the AI will be frightened and stupid (performance will decrease)!
Oh mai Karma, it is difficult to raise a four-legged gold-eating beast. Is it so difficult to raise a (xun) cyber child?
Let’s take a look at what suggestions this group of AI “nurses and dads” put forward for training children.
To be honest, AI can reduce gender discrimination
Before this time, it was not that no one was grabbing the ears of AI that had fallen behind, trying to get rid of the bad habit of favoring boys over girls.
However, most current debiasing methods will degrade the performance of the model on other tasks.
For example, if you let AI weaken gender discrimination, it will produce this annoying result:
It will either not be able to tell whether the gender of "dad" is male or female, or it will make grammatical errors. Error, forgetting to give the verb s that follows the third person.
What’s even more annoying is that this degradation mechanism has not yet been studied clearly.
Otherwise, we should simply abandon models with obvious gender bias——
In 2018, Amazon noticed that the model used to automatically screen resumes discriminated against female job seekers, so it removed this system Hidden in snow.
Otherwise, you will have to endure the performance degradation.
Does it mean that if you want AI to no longer be a mistake AI or a problem AI, then AI will definitely lose its mind?
Tsinghua & Fudan research said no to this.
Their research area is pre-trained language models.
This is because it shows magical power in various NLP tasks and has many practical scenarios.
It’s not a good idea when gender bias is used in social work such as online advertising, automated resume screening systems, and education.
The research proposes a theoretical framework for the origin of AI gender bias, a causal framework, which is used to explain how data imbalance leads to gender bias in the model during the pre-training process.
They define the gender bias of the pre-trained model when performing a specific prediction task as follows:
Among them, M is the model, and Y is to use M For the predicted word, B is the degree of gender bias of M.
Y0|W is the ground truth, the probability of being a male-related word or a female-related word is one-half, Y|W is the prediction of M.
If M's predicted Y is unbalanced and distributed between genders, then model M has gender bias when predicting Y0 based on w.
During the pre-training process, the optimization algorithm will determine the parameters in the embedding part and K based on the pre-training data D.
Therefore, data imbalance D misleads the model into incorrect parameters.
For example, if the word "doctor" in the training data is more often associated with male vocabulary, the model will take it for granted that "doctor" is associated with "male gender".
Have you seen this triangle? Let’s use it to explain why the current method of correcting AI will make it stupid.
When applying the pre-trained model to predict Y based on W, the model first converts W into extracted X, and then determines the mean of Y based on X and K.
Due to misleading parameters in the dive part, W was converted to an incorrect X, and K was also incorrect.
After one operation, the wrong X and the wrong K together lead to an error in Y.
These errors, and their interaction, lead to gender bias through three potential mechanisms.
In other words, at this point, gender bias has arisen.
How does the current debiasing method for educational AI work?
All current debiasing methods intervene in one or two of the three mechanisms.
The details are as follows:
- Enhance data intervention on D and intervene in all three mechanisms.
- By eliminating the geometric projection of X on the gender space in K, the path of D→X→K→Y is cut off.
- The gender equality regularization method either distorts the relationship between D and X or the relationship between D and K, so this type of method interferes with the mechanisms of D→X→Y and D→X→K→Y .
After explaining the bias-performance dilemma existing in current debiasing methods, the team tried to propose a fine-tuning method.
They found that among the three mechanisms, D→X→Y is the only one that leads to gender bias and has nothing to do with the transformer.
If the fine-tuning method only corrects the bias through D→X→Y, it can reduce gender bias while maintaining the performance of the model.
Based on the decomposition theorem, the team conducted numerical experiments.
It turns out that this approach can bring double dividends:
Reduce some gender bias while avoiding performance degradation.
After experiments, team members located the source of AI gender bias in the two architectures of the pre-training model: word embedding and conversion.
Accordingly, the research team proposed the C4D method, which reduces gender bias by adjusting marker embedding.
The core idea of this method is to reduce the TDE function by correcting the misguided X, thereby reducing the total deviation.
Although the team does not know what the correct marker embedding is, they developed a gradient-based method to infer the underlying ground truth.
Everything is ready, the team applied the C4D method to the GPT-2 test debiasing results.
The results show that among all test methods, the C4D method has the lowest perplexity on small, medium and ultra-large GPT-2.
In the large-scale GPT-2, C4D’s perplexity ranked second, only 0.4% worse than the highest score.
Moreover, the method with the highest score has a lower debiasing effect on gender discrimination than C4D.
On the GLUE dataset, the C4D method obtained the highest average score.
This shows that C4D can significantly reduce gender bias and maintain model performance.
After listening to so many theoretical introductions, let’s look at an illustration to get an intuitive feel.
In the three pictures below, the blue dots represent hidden male bias, and the red dots represent female bias.
Picture (a) is the original understanding of AI; Picture (b) is the understanding of AI after humans scolded them aimlessly; Picture (c) is AI’s understanding after humans found the reason and patiently explained it understand.
In figures (b) and (c), the embedding of male bias and female bias is more concentrated, which means the level of bias is lower.
At the same time, it can be noted that the embedding in Figure (c) still maintains the topology in Figure (a), which is why the C4D method can maintain model performance.
Researcher: It may also be able to reduce other biases in AI
"Although this method can effectively alleviate AI's gender bias in language models, it is still not enough to completely eliminate it."
——Researchers pointed out this issue truthfully.
If you want to further correct AI bias without reducing AI performance, you need to better understand the mechanism of language models.
How can we understand it better?
On the one hand, we use the “C4D method” proposed in this study to test other biases in AI.
The main research object of this experiment is: gender bias in the workplace.
In fact, because AI has been constantly learning all kinds of information before, it is the type that accepts everyone. As a result, it accidentally contracted the inherent social problems such as religious discrimination, discriminating against blacks and loving whites... …
So, you might as well go to GPT-2 and test the final effect of removing other biases.
On the other hand, you can try the "C4D method" on a variety of large models.
In addition to GPT-2 used in this study, the classic NLP pre-training model BERT developed by Google is also a good test scenario.
However, if you want to transplant it to other models, you need to regenerate the correction template, and you may need to use the multi-variable TDE (Template Driven Extraction) function.
By using the TDE function, you can directly put content into the index without modifying the document structure.
Some netizens came with a dog’s head:
Generally speaking, it is inevitable to become a “misstep AI” when entering society.
But if you want to turn back the prodigal son of "AI that has made a mistake", find the right method and reason with it, it will still have good results~
In addition, one of the members of the research team, Yu Yang from Tsinghua University On his personal Weibo, he stated that a website for querying gender discrimination in AI models will be launched in the next two days.
You can look forward to it!
Paper address: https://arxiv.org/abs/2211.07350 Reference link: https://weibo.com/1645372340/Mi4E43PUY#comment
The above is the detailed content of To save an AI that has made a mistake, you cannot just rely on beatings and scoldings.. For more information, please follow other related articles on the PHP Chinese website!
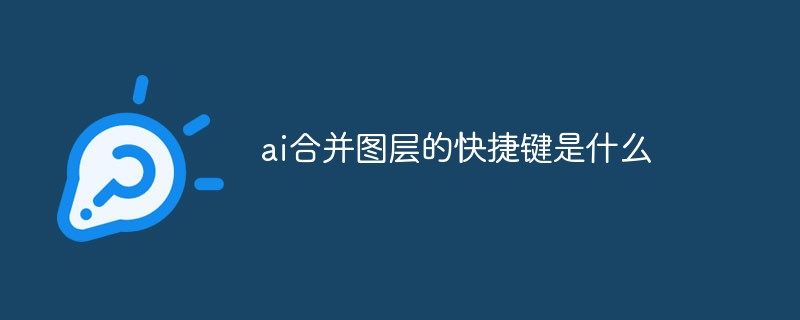
ai合并图层的快捷键是“Ctrl+Shift+E”,它的作用是把目前所有处在显示状态的图层合并,在隐藏状态的图层则不作变动。也可以选中要合并的图层,在菜单栏中依次点击“窗口”-“路径查找器”,点击“合并”按钮。
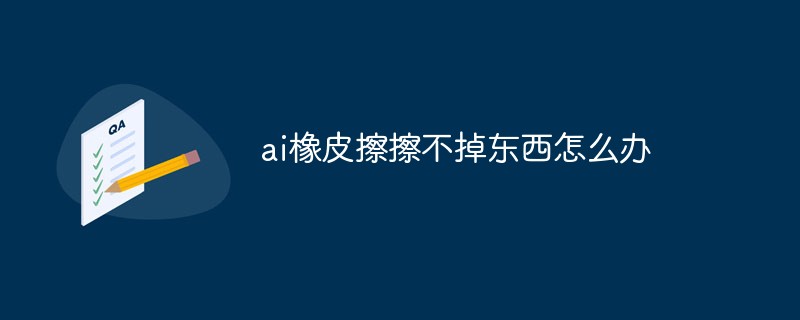
ai橡皮擦擦不掉东西是因为AI是矢量图软件,用橡皮擦不能擦位图的,其解决办法就是用蒙板工具以及钢笔勾好路径再建立蒙板即可实现擦掉东西。
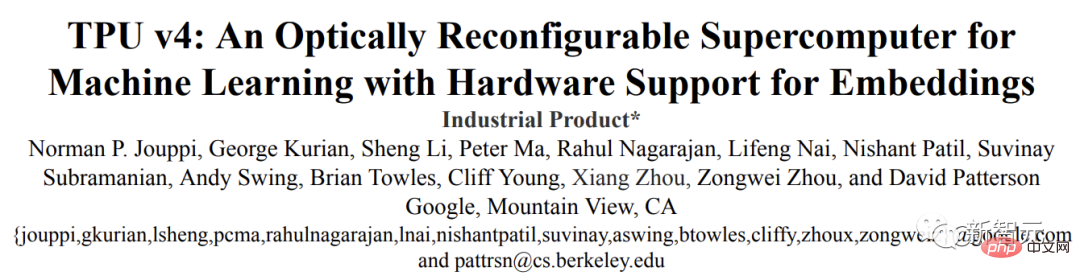
虽然谷歌早在2020年,就在自家的数据中心上部署了当时最强的AI芯片——TPU v4。但直到今年的4月4日,谷歌才首次公布了这台AI超算的技术细节。论文地址:https://arxiv.org/abs/2304.01433相比于TPU v3,TPU v4的性能要高出2.1倍,而在整合4096个芯片之后,超算的性能更是提升了10倍。另外,谷歌还声称,自家芯片要比英伟达A100更快、更节能。与A100对打,速度快1.7倍论文中,谷歌表示,对于规模相当的系统,TPU v4可以提供比英伟达A100强1.
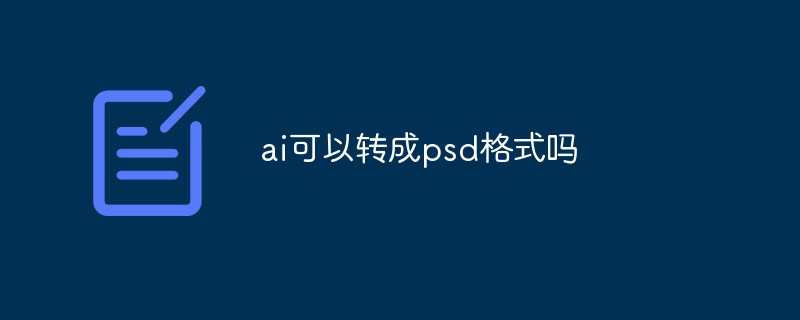
ai可以转成psd格式。转换方法:1、打开Adobe Illustrator软件,依次点击顶部菜单栏的“文件”-“打开”,选择所需的ai文件;2、点击右侧功能面板中的“图层”,点击三杠图标,在弹出的选项中选择“释放到图层(顺序)”;3、依次点击顶部菜单栏的“文件”-“导出”-“导出为”;4、在弹出的“导出”对话框中,将“保存类型”设置为“PSD格式”,点击“导出”即可;
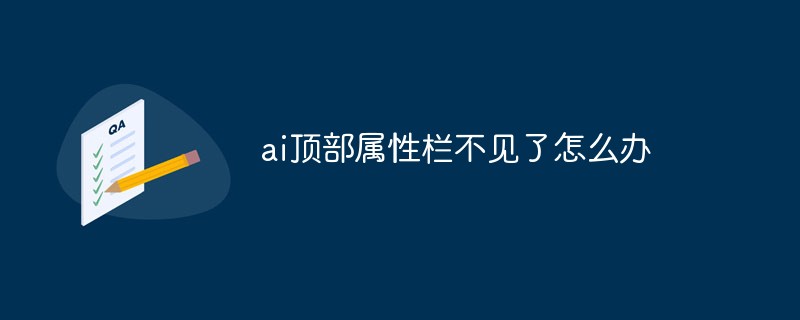
ai顶部属性栏不见了的解决办法:1、开启Ai新建画布,进入绘图页面;2、在Ai顶部菜单栏中点击“窗口”;3、在系统弹出的窗口菜单页面中点击“控制”,然后开启“控制”窗口即可显示出属性栏。
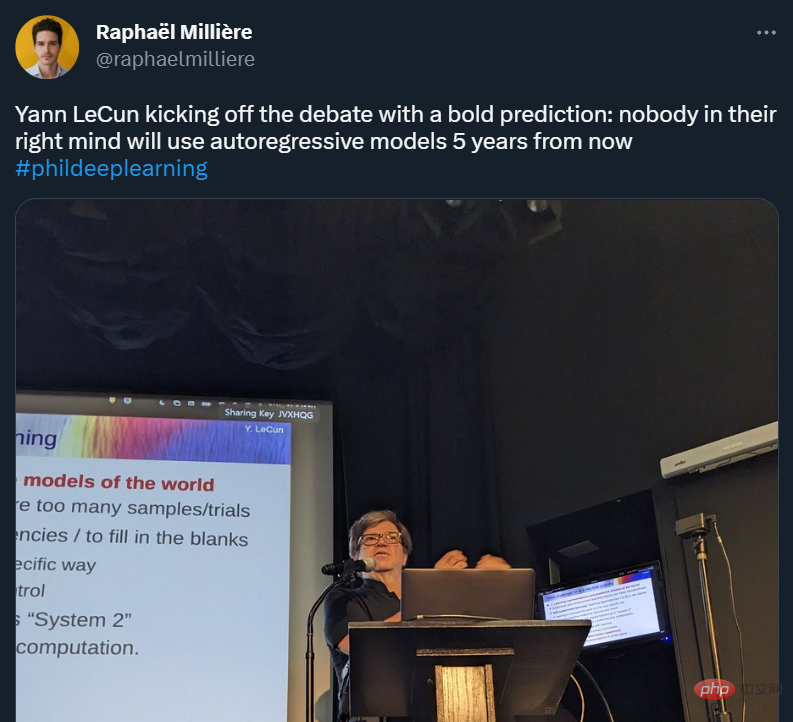
Yann LeCun 这个观点的确有些大胆。 「从现在起 5 年内,没有哪个头脑正常的人会使用自回归模型。」最近,图灵奖得主 Yann LeCun 给一场辩论做了个特别的开场。而他口中的自回归,正是当前爆红的 GPT 家族模型所依赖的学习范式。当然,被 Yann LeCun 指出问题的不只是自回归模型。在他看来,当前整个的机器学习领域都面临巨大挑战。这场辩论的主题为「Do large language models need sensory grounding for meaning and u
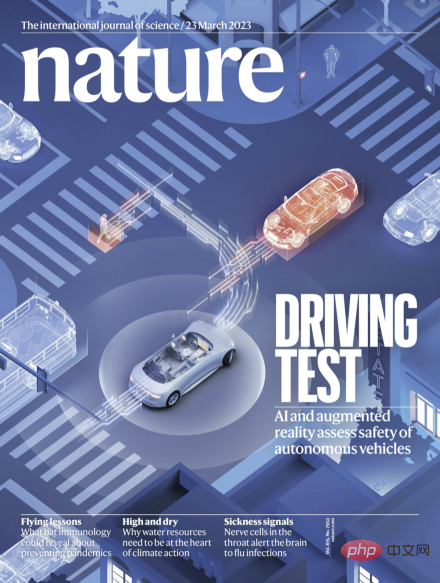
引入密集强化学习,用 AI 验证 AI。 自动驾驶汽车 (AV) 技术的快速发展,使得我们正处于交通革命的风口浪尖,其规模是自一个世纪前汽车问世以来从未见过的。自动驾驶技术具有显着提高交通安全性、机动性和可持续性的潜力,因此引起了工业界、政府机构、专业组织和学术机构的共同关注。过去 20 年里,自动驾驶汽车的发展取得了长足的进步,尤其是随着深度学习的出现更是如此。到 2015 年,开始有公司宣布他们将在 2020 之前量产 AV。不过到目前为止,并且没有 level 4 级别的 AV 可以在市场
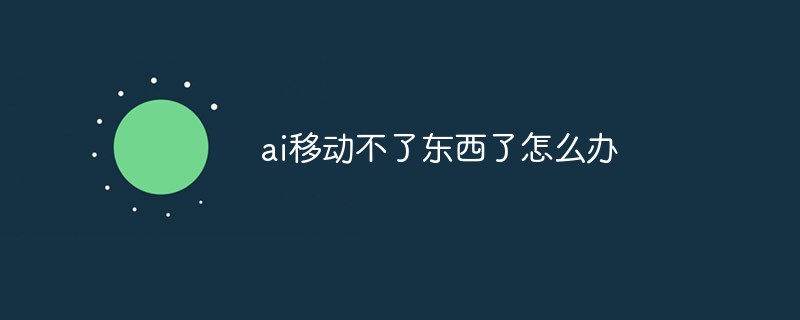
ai移动不了东西的解决办法:1、打开ai软件,打开空白文档;2、选择矩形工具,在文档中绘制矩形;3、点击选择工具,移动文档中的矩形;4、点击图层按钮,弹出图层面板对话框,解锁图层;5、点击选择工具,移动矩形即可。


Hot AI Tools

Undresser.AI Undress
AI-powered app for creating realistic nude photos

AI Clothes Remover
Online AI tool for removing clothes from photos.

Undress AI Tool
Undress images for free

Clothoff.io
AI clothes remover

AI Hentai Generator
Generate AI Hentai for free.

Hot Article

Hot Tools

SublimeText3 English version
Recommended: Win version, supports code prompts!
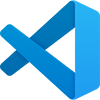
VSCode Windows 64-bit Download
A free and powerful IDE editor launched by Microsoft

MantisBT
Mantis is an easy-to-deploy web-based defect tracking tool designed to aid in product defect tracking. It requires PHP, MySQL and a web server. Check out our demo and hosting services.
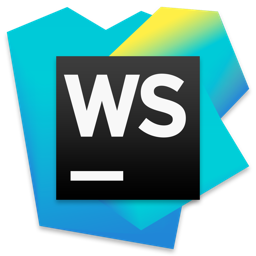
WebStorm Mac version
Useful JavaScript development tools
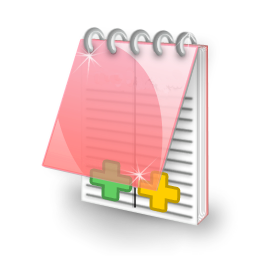
EditPlus Chinese cracked version
Small size, syntax highlighting, does not support code prompt function
