


Helping artificial intelligence move to a new stage, the YLearn causal learning open source project is released
On July 12, 2022, Jiuzhang Yunji DataCanvas Company released another breakthrough open source technology achievement-YLearn causal learning open source project, and successfully held an online press conference.
The conference is themed "From prediction to decision-making, understandable AI", and specially invites experts in the field of causal learning & artificial intelligence: Jiuzhang Yunji DataCanvas co-founder and CTOShang Mingdong, Founder & Chairman of CSDN, Founding Partner of Geek Bang Venture Capital Jiang Tao, Permanent Associate Professor and Doctoral Supervisor of Department of Computer Science, Tsinghua University Cui Peng and YLearn R&D Team , jointly discuss the latest research results of causal learning in academia and industry, and jointly promote the rapid development of causal science.
YLearn - the AI key that opens the door to "automated decision-making"
YLearn causal learning open source project, is global The first one-stop open source algorithm toolkit that handles the complete process of causal learning. It is the first to solve the "causal discovery, causal quantity identification, causal effect estimation, counterfactual inference and strategy learning" in causal learning. The five key issues have the characteristics of one-stop, new and comprehensive, and wide-ranging uses. It reduces the threshold for "decision makers" to the lowest level and helps governments and enterprises effectively improve their automated "decision-making" capabilities.
YLearn causal learning open source project is Jiuzhang Yunji DataCanvas company’s successor to DAT automatic machine learning toolkit and DingoDB real-time interactive analysis database After that, the third open source heavy tool was released. Since then, Jiuzhang Yunji DataCanvas' open source basic software has further expanded its territory. The open source basic tool series integrating cutting-edge AI technologies such as AutoML and causal learning will further accelerate the release of the value of data intelligence in the government and the entire industry.
By combining innovative insights from cutting-edge academic fields and market applications, the Jiuzhang Yunji DataCanvas open source project R&D team found that although the currently widely used business "prediction" results based on machine learning are improving business income The effect in this regard has been very significant, but as governments and enterprises have increasingly strong demands for "autonomous AI" and "intelligent decision-making", decision-makers need an understandable "reason" that can explain why a decision was made. The presentation of "causal relationships" has thus become an indispensable function for data analysis and intelligent decision-making, but machine learning that only provides data "correlation" cannot do this. The integration of
with "Causal Learning" technology will become the best solution to this problem, and the YLearn causal learning open source project was born.
YLearn causal learning open source project (hereinafter referred to as "YLearn") also has the "open source, flexible and automatic" gene of Jiuzhang Yunji DataCanvas product. Based on the open source community, YLearn aims to fill the gap in the market that lacks a complete, comprehensive, end-to-end causal learning toolkit, and work with global open source contributors to create an end-to-end, most complete, and most systematic's causal learning algorithm toolkit directly reduces the usage cost of "decision makers" from the tool side.
Currently, YLearn consists of CausalDiscovery, CausalModel, EstimatorModel, Policy, Interpreter and other components. Each component supports independent use and unified packaging. Through these flexible components, YLearn implements functions such as using causal diagrams to represent causal relationships in data sets, identifying causal effects, probability expressions, and various estimation models, and will continue to add and improve performance following cutting-edge research.
In order to further lower the threshold of use, in addition to making the usage process clear and simple, YLearn will also integrate AutoML automatic machine learning, the core technology of Jiuzhang Yunji DataCanvas Company. With the support of AutoML technology, YLearn will realize advanced "automated" functions such as automatic parameter adjustment, automatic optimization, and one-click automatic generation of multiple decision-making solutions corresponding to the result "Y"; in addition, YLearn will also realize a visual decision-making map based on causal relationships. , such as setting operational indicators for enterprise operations, and deduce the impact and benefits of different decisions in an interactive way.
The YLearn causal learning open source project, which provides automated causality analysis, will provide important support for decision-makers to understand the logic of AI decision-making and enhance the credibility of AI decision-making. It will also open the door to "automated decision-making" for governments and enterprises. AI key.
Causal learning - leading artificial intelligence to a new stage
The potential of causal learning and its influence on the future direction of artificial intelligence technology have been recognized by academia and industry. Judea Pearl, winner of the Turing Award in 2011 and the father of Bayesian networks, once mentioned that "without the ability to reason about causal relationships, the development of AI will be fundamentally limited."
Tsinghua University Department of Computer Science, permanent associate professor and doctoral supervisorCui Peng pointed out at this conference that "causal statistics will play an important role in the theoretical foundation of the new generation of artificial intelligence." The root cause of the current limitations of artificial intelligence is "knowing it, but not knowing why." Among them, the "ran" in "knowing it" refers to the "correlation" relationship between data, and "so-ran" refers to the "causal" relationship between data. Through years of research on introducing causal statistics into machine learning, Professor Cui's team found that causal statistics have outstanding performance in solving the stability problems, interpretability problems, and algorithm fairness problems of machine learning.
The commercial market also calls for accelerating the industrial application of causal learning technology. Gartner's latest causal learning innovation insight report "Innovation Insight: Causal
AI" points out that "artificial intelligence must move beyond correlation-based predictions toward causality-based solutions to achieve better decisions and more efficient Big automation. ...Causal artificial intelligence is crucial to the future."
Causal learning technology will greatly improve the autonomy, explainability, adaptability and robustness of artificial intelligence technology. These features will further reduce costs and increase efficiency for governments and enterprises that implement digital intelligence upgrades based on AI technology, and reap unexpected data value.
Open source tool - the engine of innovative application of AI technology
A cutting-edge technology can achieve successful large-scale application in the commercial market, which cannot be separated from the boost of powerful open source tools and catalysis.
Just like Sklearn (one of the most well-known programming modules in the field of machine learning) is to the application of machine learning technology, and TensorFlow and PyTorch (two full-featured frameworks for building deep learning models) are to The great significance and value of the application of deep learning technology also urgently requires an "open source tool" to break through application bottlenecks in the field of causal learning.
The emergence of the YLearn causal learning open source toolkit solves the "stuck" problem of the lack of a powerful and complete causal learning toolkit on the market, accelerating the introduction of causal learning technology from the "laboratory" to the "industry" application". Jiang Tao, founder & chairman of CSDN and founding partner of Geek Bang Venture Capital, said, "China's open source is at the right time. Only when technology becomes more popular will there be a bigger market. YLearn will be more sophisticated about AI technology in various industries." Going deeper will have great impetus. The development of my country's software industry is the foundation for the growth of the open source industry and provides it with growth soil. The country attaches great importance to the development of the open source industry and has implemented it in the "14th Five-Year Plan" "For the first time, open source has been incorporated into the top-level design in the plan. Shang Mingdong, co-founder and CTO of Jiuzhang Yunji DataCanvas, said in his speech at the press conference, "2022 has entered the year of take-off for open source. We believe that in the field of AI, software is infrastructure. Compared with application software, open source is the ‘main battlefield’ for basic software. ”
Adhering to the product culture of Jiuzhang Yunji DataCanvas Company that closely revolves around the technological innovation concept of “data intelligence” and “integrating and applying AI technology to actual business scenarios”, Jiuzhang Yunji DataCanvas open source project R&D team innovates and iterates While open source tools, we continue to absorb the needs and feedback from practical applications in various scenarios from the government and industry. At the same time, the AI basic software product series of Jiuzhang Yunji DataCanvas is being continuously integrated and applied with independently developed open source tools, and will also accelerate Government and enterprise customers enjoy the business value brought by the application of AI fusion technology.
The above is the detailed content of Helping artificial intelligence move to a new stage, the YLearn causal learning open source project is released. For more information, please follow other related articles on the PHP Chinese website!
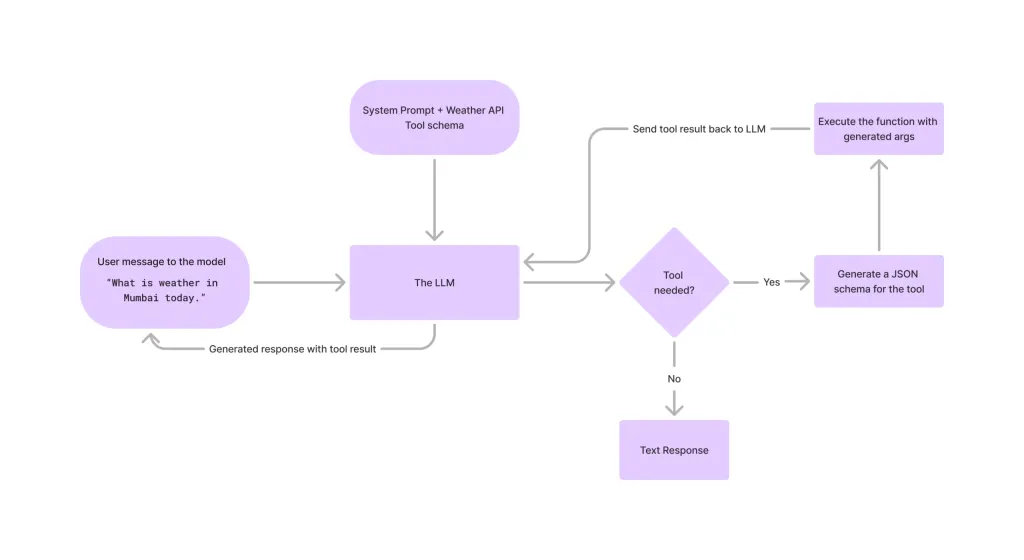
Large language models (LLMs) have surged in popularity, with the tool-calling feature dramatically expanding their capabilities beyond simple text generation. Now, LLMs can handle complex automation tasks such as dynamic UI creation and autonomous a
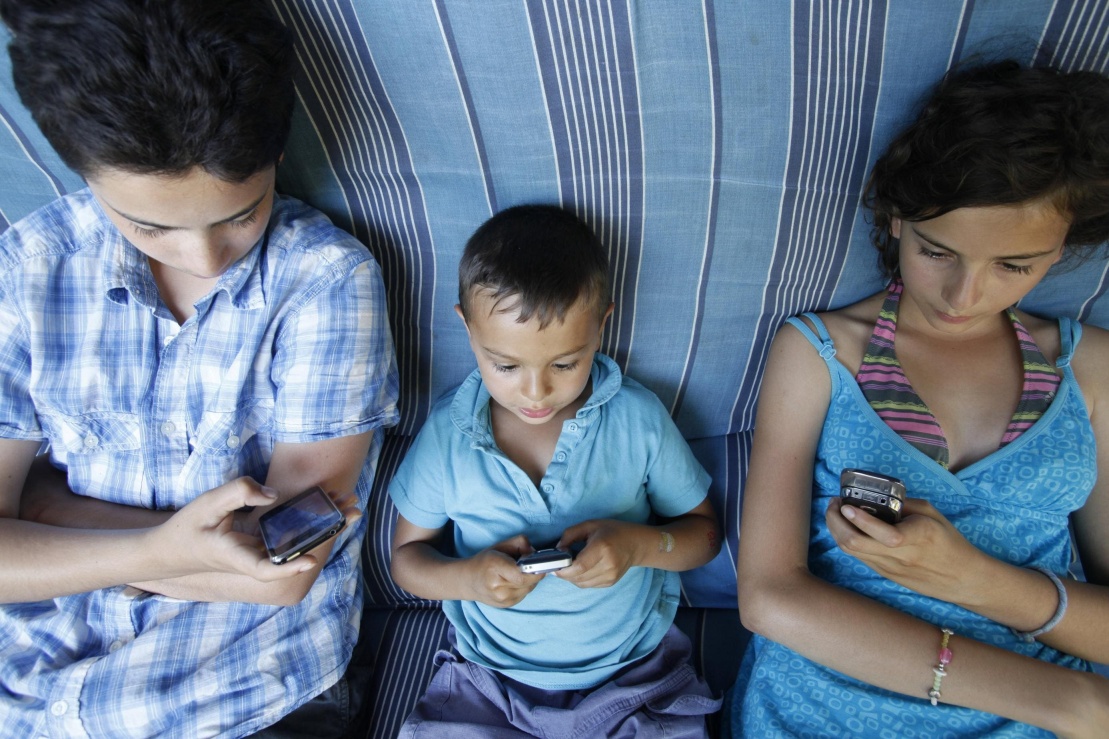
Can a video game ease anxiety, build focus, or support a child with ADHD? As healthcare challenges surge globally — especially among youth — innovators are turning to an unlikely tool: video games. Now one of the world’s largest entertainment indus
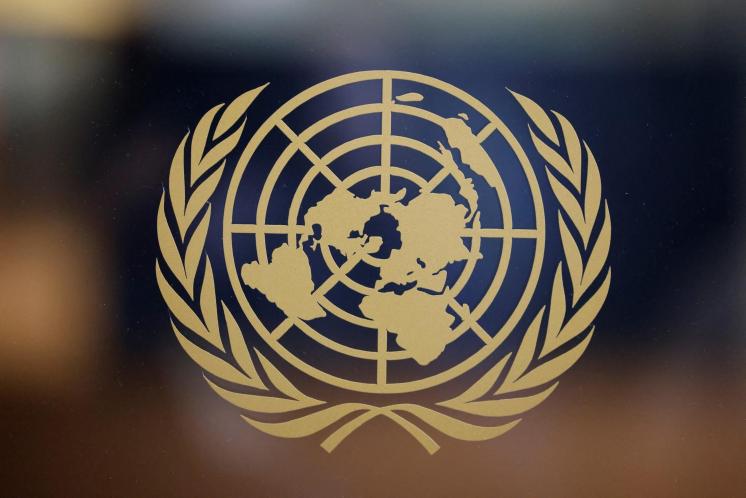
“History has shown that while technological progress drives economic growth, it does not on its own ensure equitable income distribution or promote inclusive human development,” writes Rebeca Grynspan, Secretary-General of UNCTAD, in the preamble.
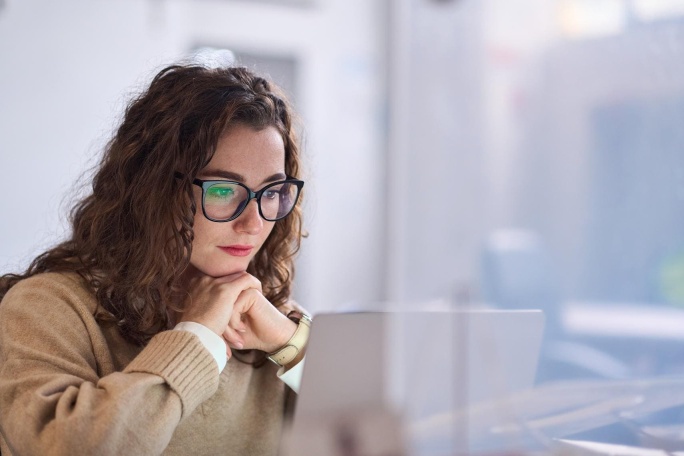
Easy-peasy, use generative AI as your negotiation tutor and sparring partner. Let’s talk about it. This analysis of an innovative AI breakthrough is part of my ongoing Forbes column coverage on the latest in AI, including identifying and explaining
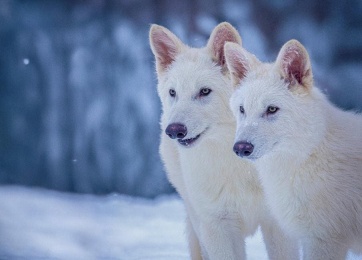
The TED2025 Conference, held in Vancouver, wrapped its 36th edition yesterday, April 11. It featured 80 speakers from more than 60 countries, including Sam Altman, Eric Schmidt, and Palmer Luckey. TED’s theme, “humanity reimagined,” was tailor made
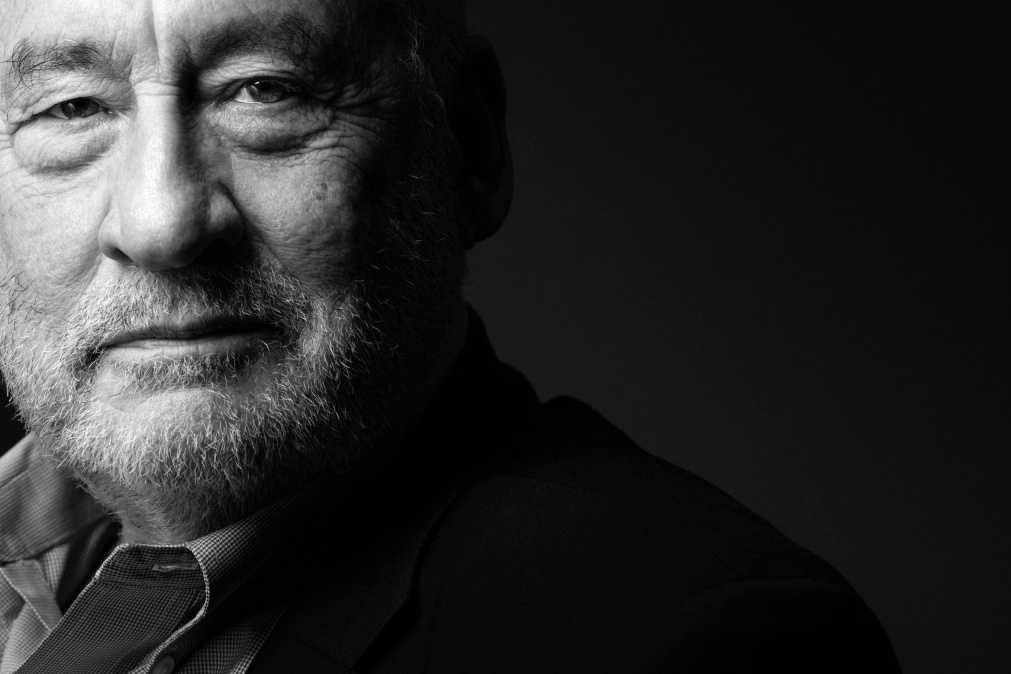
Joseph Stiglitz is renowned economist and recipient of the Nobel Prize in Economics in 2001. Stiglitz posits that AI can worsen existing inequalities and consolidated power in the hands of a few dominant corporations, ultimately undermining economic
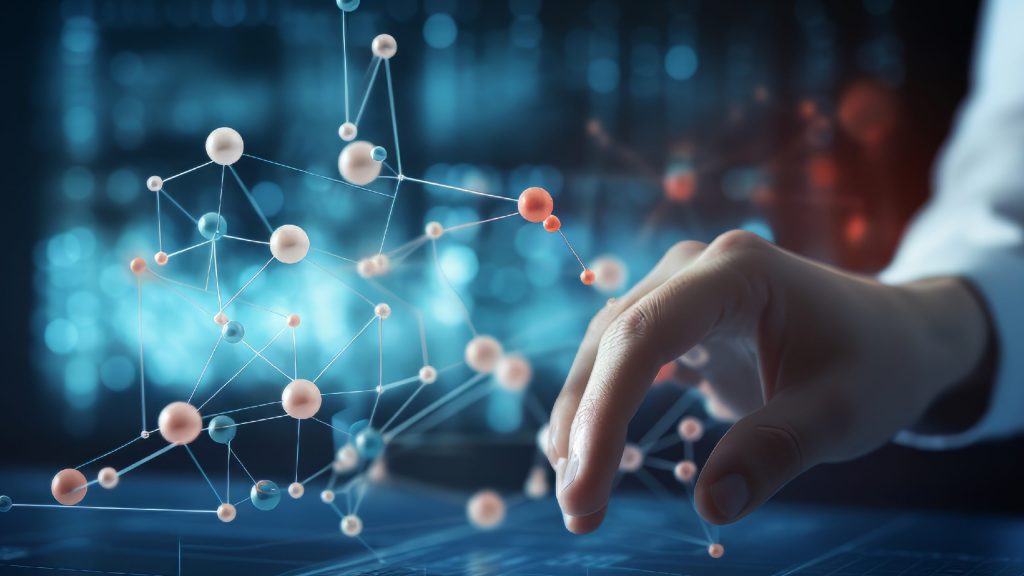
Graph Databases: Revolutionizing Data Management Through Relationships As data expands and its characteristics evolve across various fields, graph databases are emerging as transformative solutions for managing interconnected data. Unlike traditional
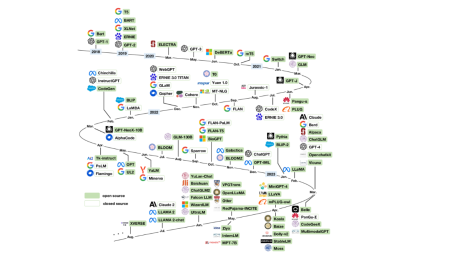
Large Language Model (LLM) Routing: Optimizing Performance Through Intelligent Task Distribution The rapidly evolving landscape of LLMs presents a diverse range of models, each with unique strengths and weaknesses. Some excel at creative content gen


Hot AI Tools

Undresser.AI Undress
AI-powered app for creating realistic nude photos

AI Clothes Remover
Online AI tool for removing clothes from photos.

Undress AI Tool
Undress images for free

Clothoff.io
AI clothes remover

AI Hentai Generator
Generate AI Hentai for free.

Hot Article

Hot Tools
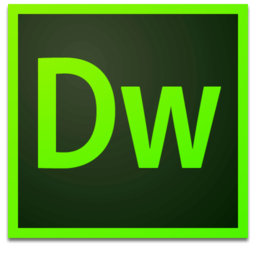
Dreamweaver Mac version
Visual web development tools

SublimeText3 English version
Recommended: Win version, supports code prompts!

Notepad++7.3.1
Easy-to-use and free code editor

Atom editor mac version download
The most popular open source editor

SAP NetWeaver Server Adapter for Eclipse
Integrate Eclipse with SAP NetWeaver application server.