


Can the model be directly connected to AGI as long as it 'gets bigger'? Marcus bombarded again: Three crises have emerged!
In May of this year, DeepMind released Gato, a multi-modal artificial intelligence system that can perform more than 600 different tasks simultaneously with only one set of model parameters, which temporarily aroused heated discussions about general artificial intelligence (AGI) in the industry. .
Nando de Freitas, director of the research department of DeepMind, also tweeted at the time that AI can be passed as long as the scale continues to increase!
What we have to do is to make the model bigger, safer, more computationally efficient, faster sampling, smarter storage, more modalities, on the data Innovation, online/offline, etc.
AGI can be achieved by solving scale problems. The industry needs to pay more attention to these issues!
Recently, Gary Marcus, a well-known AI scholar, founder and CEO of Robust.AI, and emeritus professor at New York University, published another blog, believing that this statement is "too early" and has already begun There is a crisis!
Marcus continues to pay attention to the development of the AI industry, but is critical of the hype of AI. He has expressed objections such as "deep learning hits a wall" and "GPT-3 is completely meaningless".
What should I do if I can’t play with the large model?
Nando believes that artificial intelligence does not require a paradigm shift, it only requires more data, higher efficiency and larger servers.
Marcus paraphrased this hypothesis as: Without fundamental new innovation, AGI may emerge from larger-scale models. This assumption can also be called scaling-über-alles.
His hypothesis, now often referred to as scaling maximalism, remains very popular, largely because larger and larger models are indeed very powerful for tasks such as image generation. Large models are required.
But that’s only so far.
The problem is that some of the technologies that have been improved over months and years are actually nowhere near the scale we need.
There are more and more Ponzi schemes. The performance advantage brought by scale is only the result of empirical observation and cannot be guaranteed to be correct.
Marcus shares three recent signs that may signal the end of the scale maximization hypothesis.
1. There may not be enough data in the world to support maximum scale.
Many people have begun to worry about this.
Researchers William Merrill, Alex Warstadt, and Tal Linzen from New York University and ETH Zurich recently presented a demonstration that "current neural language models are not well suited to extracting natural language without large amounts of data." The semantics of language".
Paper link: https://arxiv.org/pdf/2209.12407.pdf
Although this proof contains too many presumptions, So much so that it cannot be taken as a rebuttal, but if this hypothesis is even close to being correct, there could be real trouble on the scale very soon.
2. There may not be enough available computing resources in the world to support maximum scale.
Miguel Solano recently sent Marcus a co-authored manuscript in which the author believes that reaching current super benchmarks such as BIG-bench would require 2022 U.S. electricity consumption More than a quarter of that.
https://www.php.cn/link/e21bd8ab999859f3642d2227e682e66f
BIG-bench is a crowdsourced benchmark dataset designed to explore large language models and infer their future capabilities, containing over 200 tasks.3. Some important tasks may simply not scale at scale.
The most obvious example is a recent linguistics assignment by Ruis, Khan, Biderman, Hooker, Rocktäschl and Grefenstette, who studied the pragmatic meaning of language. For example, for the question "Did you leave fingerprints?", the answer received may be "I wore gloves", whose semantics is "no". As Marcus has long argued, making a model aware of this without cognitive models and common sense is really difficult.Scale plays little role in this type of task. Even the best model only has an accuracy of 80.6%. For most models, the effect of scale is negligible at best.
And, you can easily imagine a more complex version of this task, where the performance of the model will be further reduced.
What hit Marcus even more was that even for a single important task like this, about 80% performance may mean that a large-scale game cannot continue to be played.
If the model only learns syntax and semantics, but fails in pragmatic or common sense reasoning, then you may not be able to obtain trustworthy AGI at all
"Moore's Law" is not as effective as It has taken us so far and so fast as initially expected, because it is not the law of cause and effect in the universe that will always hold true.
Maximizing scale is just an interesting assumption. It will not allow us to reach general artificial intelligence. For example, solving the above three problems will force us to make a paradigm shift.
Netizen Frank van der Velde said that followers who maximize scale tend to use vague terms such as "big" and "more".
The training data used by deep learning models is too large compared to the training data used by humans in learning languages.
But compared with the real semantic collection of human language, these so-called massive data are still insignificant. It would take about 10 billion people to generate a sentence per second, and it would last 300 years to obtain such a large-scale training set.
Netizen Rebel Science even bluntly said that maximizing scale is not an interesting hypothesis, but a stupid hypothesis. It will not only lose on the AI track, but also die ugly.
Maximizing scale is too extreme
Raphaël Millière, a lecturer in the Department of Philosophy at Columbia University and a Ph.D. at Oxford University, also expressed some of his own opinions when the battle over "maximizing scale" was at its fiercest.
Maximizing scale, once seen as a catch-all for critics of deep learning (such as Gary Marcus), is now at loggerheads with industry insiders such as Nando de Freitas and Alex Dimakis joining the debate.
The responses from practitioners are mostly mixed, but not too negative. At the same time, the forecast date for AGI implementation on the forecasting platform Metaculus has been advanced to a historical low (May 2028), which may also increase the largest scale. ation credibility.
People's growing trust in "scale" may be due to the release of new models, such as the success of PaLM, DALL-E 2, Flamingo and Gato, adding fuel to the fire of maximizing scale.
Sutton's "Bitter Lesson" throws out many points in the discussion about maximizing scale, but they are not completely equivalent. He believes that building human knowledge into artificial intelligence models (for example, feature engineering) The efficiency is lower than using data and computing to learn.
Article link: http://www.incompleteideas.net/IncIdeas/BitterLesson.html
While not without controversy, Sutton’s point seems obvious Not as radical as maximizing scale.
It does emphasize the importance of scale, but it does not reduce every problem in artificial intelligence research to a mere challenge of scale.
In fact, it is difficult to determine the specific meaning of maximizing scale. Literally understood, "Scaling is all you need" indicates that we do not need any algorithm innovation or architectural changes to achieve AGI and can expand existing model and force input of more data.
This literal explanation seems absurd: even models like Palm, DALL-E 2, Flamingo or Gato still require architectural changes from previous approaches.
It would be really surprising if anyone really thought we could extend an off-the-shelf autoregressive Transformer to AGI.
It’s unclear how much algorithmic innovation people who believe in maximizing scale feel AGI requires, which makes it difficult to generate falsifiable predictions from this perspective.
Scaling may be a necessary condition for building any system that deserves the label "general artificial intelligence," but we shouldn't mistake necessity for a sufficient condition.
The above is the detailed content of Can the model be directly connected to AGI as long as it 'gets bigger'? Marcus bombarded again: Three crises have emerged!. For more information, please follow other related articles on the PHP Chinese website!
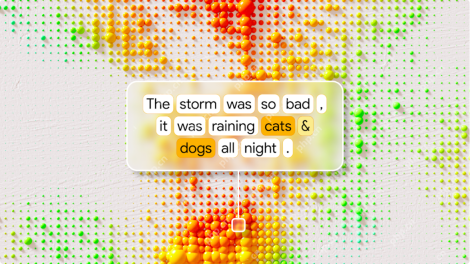
Exploring the Inner Workings of Language Models with Gemma Scope Understanding the complexities of AI language models is a significant challenge. Google's release of Gemma Scope, a comprehensive toolkit, offers researchers a powerful way to delve in
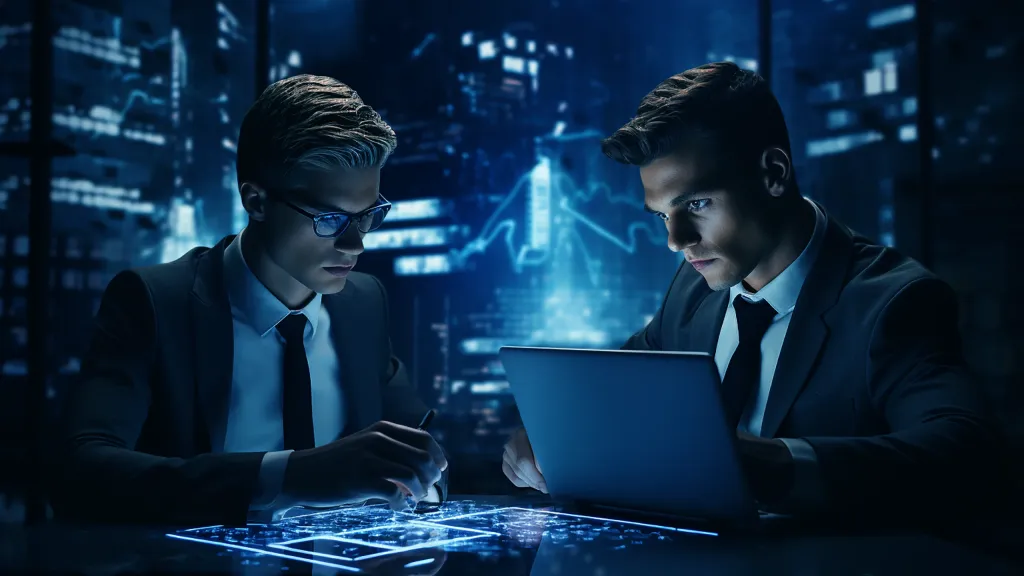
Unlocking Business Success: A Guide to Becoming a Business Intelligence Analyst Imagine transforming raw data into actionable insights that drive organizational growth. This is the power of a Business Intelligence (BI) Analyst – a crucial role in gu

SQL's ALTER TABLE Statement: Dynamically Adding Columns to Your Database In data management, SQL's adaptability is crucial. Need to adjust your database structure on the fly? The ALTER TABLE statement is your solution. This guide details adding colu
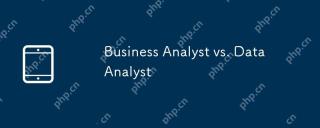
Introduction Imagine a bustling office where two professionals collaborate on a critical project. The business analyst focuses on the company's objectives, identifying areas for improvement, and ensuring strategic alignment with market trends. Simu
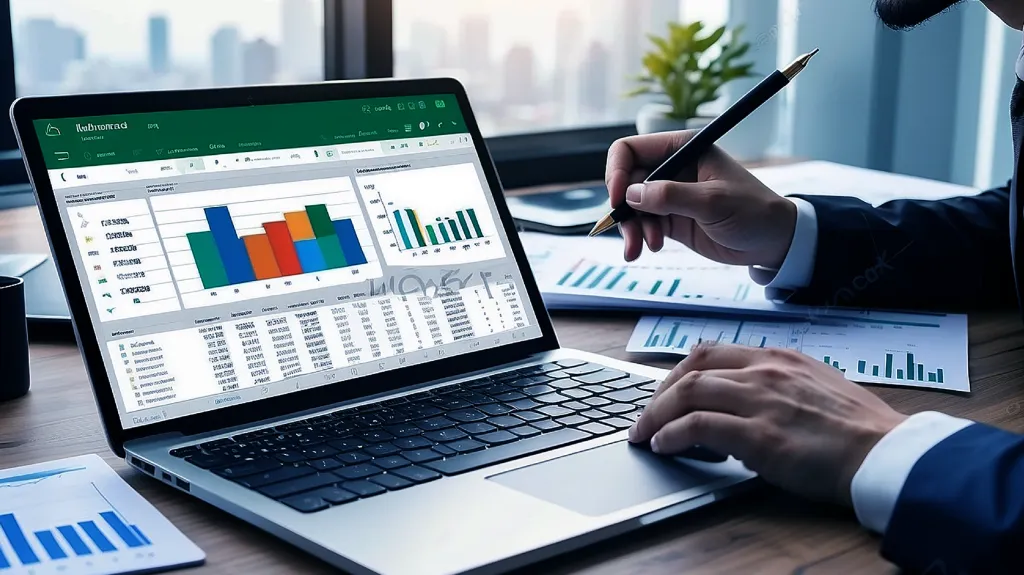
Excel data counting and analysis: detailed explanation of COUNT and COUNTA functions Accurate data counting and analysis are critical in Excel, especially when working with large data sets. Excel provides a variety of functions to achieve this, with the COUNT and COUNTA functions being key tools for counting the number of cells under different conditions. Although both functions are used to count cells, their design targets are targeted at different data types. Let's dig into the specific details of COUNT and COUNTA functions, highlight their unique features and differences, and learn how to apply them in data analysis. Overview of key points Understand COUNT and COU
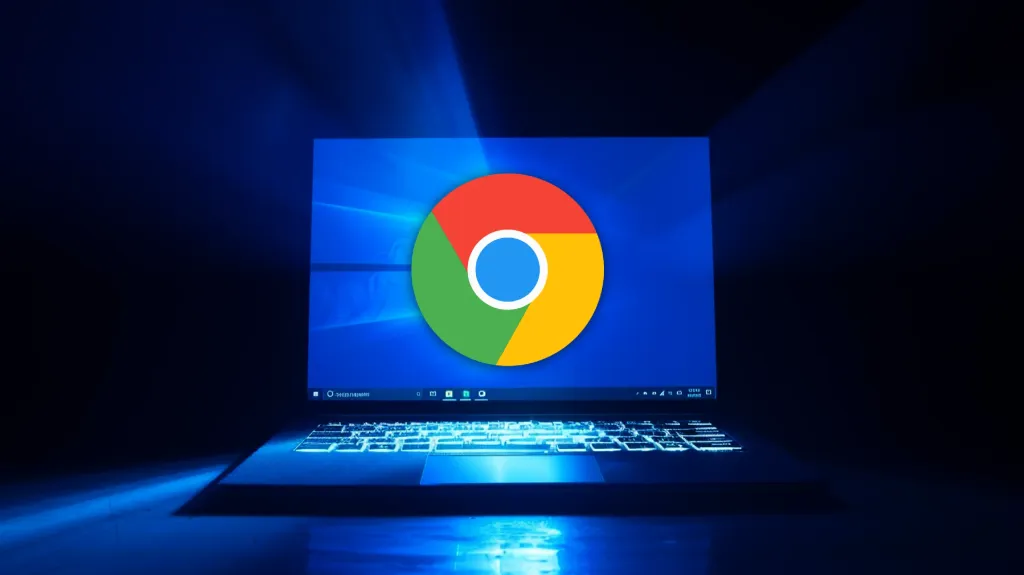
Google Chrome's AI Revolution: A Personalized and Efficient Browsing Experience Artificial Intelligence (AI) is rapidly transforming our daily lives, and Google Chrome is leading the charge in the web browsing arena. This article explores the exciti
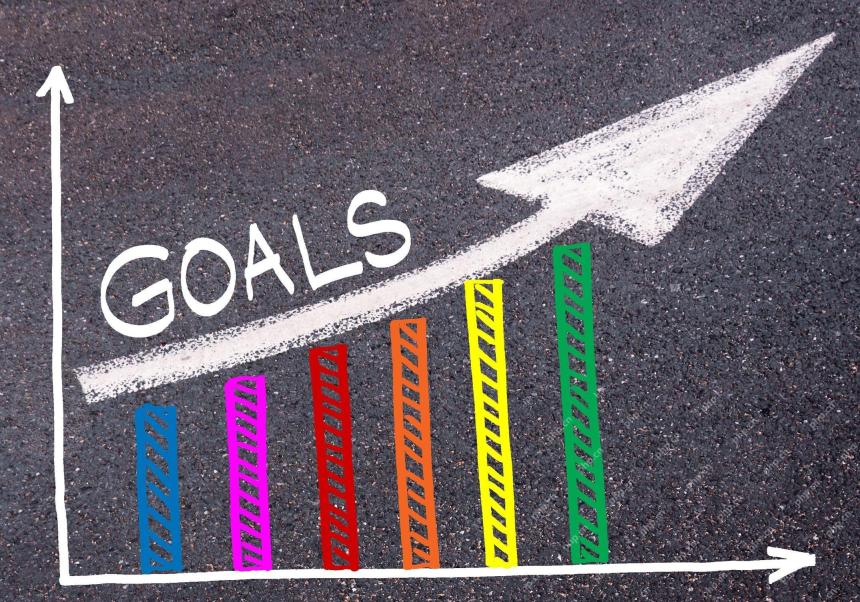
Reimagining Impact: The Quadruple Bottom Line For too long, the conversation has been dominated by a narrow view of AI’s impact, primarily focused on the bottom line of profit. However, a more holistic approach recognizes the interconnectedness of bu
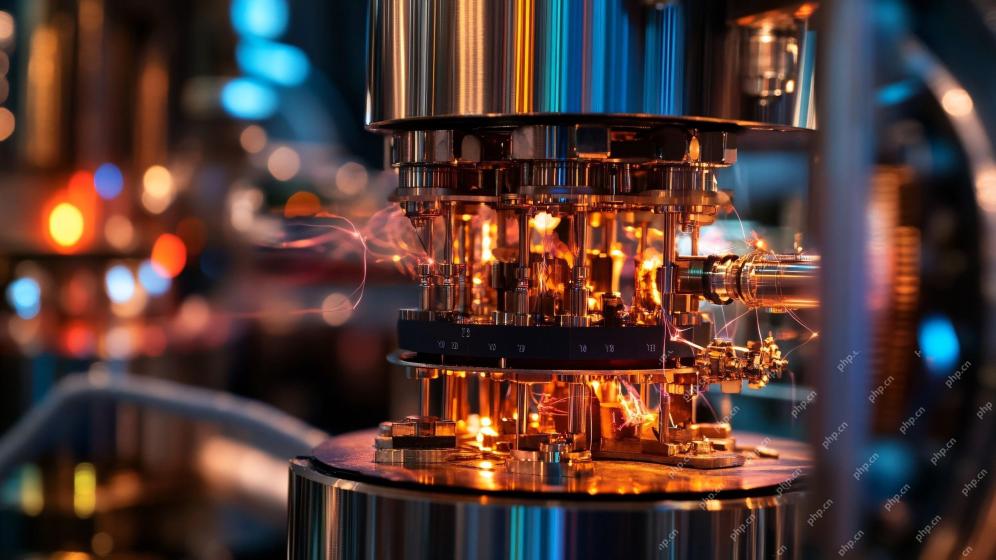
Things are moving steadily towards that point. The investment pouring into quantum service providers and startups shows that industry understands its significance. And a growing number of real-world use cases are emerging to demonstrate its value out


Hot AI Tools

Undresser.AI Undress
AI-powered app for creating realistic nude photos

AI Clothes Remover
Online AI tool for removing clothes from photos.

Undress AI Tool
Undress images for free

Clothoff.io
AI clothes remover

AI Hentai Generator
Generate AI Hentai for free.

Hot Article

Hot Tools

Zend Studio 13.0.1
Powerful PHP integrated development environment

SublimeText3 English version
Recommended: Win version, supports code prompts!
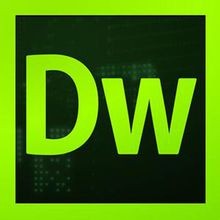
Dreamweaver CS6
Visual web development tools

MantisBT
Mantis is an easy-to-deploy web-based defect tracking tool designed to aid in product defect tracking. It requires PHP, MySQL and a web server. Check out our demo and hosting services.
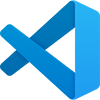
VSCode Windows 64-bit Download
A free and powerful IDE editor launched by Microsoft