


Text analysis is a powerful discipline capable of discovering and annotating every example of customer opinion, regardless of which language the customer speaks.
For business executives who are waking up to the vast amounts of unstructured data surrounding their businesses, the language-agnostic possibilities of AI for text analysis are a critical (but easily overlooked) )The problem.
After all, unstructured data (UD) is not structured data in a format such as spreadsheets, but is usually large amounts of data in various social media, blogs, website comments, call center calls, private chats, etc. – and this data represents a vast resource with even greater value for businesses interested in improving customer experience (CX).
Most data is unstructured data. According to estimates from MIT, 80% to 90% of data today is unstructured data, and it is growing rapidly. And this fact means that all opinions from customers can be collated and analyzed by businesses that have invested in technology and expertise.
This is the role of text analysis artificial intelligence. This results in every customer who comments on a business brand on any platform having unprecedented access to their thoughts, opinions and ideas. It allows companies to accurately and quickly identify customer pain points that are prioritized, thereby reducing customer churn.
Given this generality, it is particularly important to recognize the value of language agnosticism. Limiting analysis and annotation to English perspectives only (when other perspectives exist) undermines the scale of unstructured data and the generalizability of this text analysis.
Therefore, it is necessary to understand how multilingual AI analytics works and its potential to gather a comprehensive overview of customer opinions.
The Power of Natural Language Processing
The foundation of AI-driven text analysis is the combination of machine learning (ML) and natural language processing (NLP).
Machine learning is an artificial intelligence method designed to imitate human learning. While traditional programming requires the execution of rules created by humans, machine learning uses data analysis to learn extremely complex patterns that can be used for inference, making machine learning very good at solving problems and performing complex tasks.
At the same time, natural language processing (NLP) belongs to processing languages. In fact, it can be understood as one of the complex tasks supported by machine learning.
In this context, the uses of natural language processing (NLP) are diverse. It can be used for simpler goals, such as counting how often a given term or word appears in a text. Or one can take on the more difficult challenge of determining the mood or even emotion of a given text.
Obviously, both are of great use to businesses that want to understand in detail the opinions of all available customers.
These uses of natural language processing (NLP) allow businesses to evaluate large amounts of data to discover how often their brand is being talked about online or offline, as well as understand whether the comments are positive or negative, or related to a The series is about more nuanced emotions.
Multi-lingual approach
Crucially, the benefit of this approach is its ability to include all customer opinions – text analysis applies to each opinion rather than a sample or selection .
However, in order to achieve this goal, the language in which a given opinion is expressed cannot be limited, but AI needs to be completely language-agnostic, especially if a business is a multinational organization.
This can be achieved through the use of unsupervised and supervised machine learning. Supervised machine learning means that the algorithms involved are "trained" by humans annotating training data, and AI can do better than humans at tasks involving large amounts of data (also known as big data).
To ensure that all language needs are met, the researchers leveraged a team of approximately 300 native speakers of a variety of languages who read, understood and manually annotated the unstructured data. For example, determine whether a tweet is positive or negative, whether there is sarcasm in its subject, or even what the customer journey is suggested by the content of an email or chat message.
Once the AI is trained in its native language (without the need for translation into English and machine learning models using English) to achieve its goals (whether establishing emotions or identifying topics) with great accuracy, the results can be easily used Visualization in English to unlock all customer opinions in a language they can understand for customer experience (CX) professionals, customer retention managers, and more.
The most important thing is that the accuracy of artificial intelligence can continue to improve. For example, when a person annotates a small subset of tweets with a certain emotion, its accuracy can be measured. You can see that 80% to 90% or more of the content matches the algorithm, no matter what language the tweets are written in.
This shows how powerful these AI technologies have become, given the subjective nature of expressing emotions.
Finding the needle in the haystack of unstructured data
Unstructured data (UD) is everywhere and it represents an opportunity to understand the opinions of all customers, rather than, by definition, like polls Only sample-based customer opinions can be provided.
However, to truly realize this ability to gain unfettered access to consumer opinions, multinational companies will not only need to hire AI experts and technicians, but also ensure that their AI systems can obtain data in all relevant languages. The same high-precision training as in English.
This way, text analysis is not only source-independent but also language-independent. Allow business leaders to confidently assert that their understanding of customer perspectives, pain points, and gain points is detailed, precise, and comprehensive.
The above is the detailed content of Multilingual AI analytics are key to unlocking the potential of customer experience to drive business growth. For more information, please follow other related articles on the PHP Chinese website!
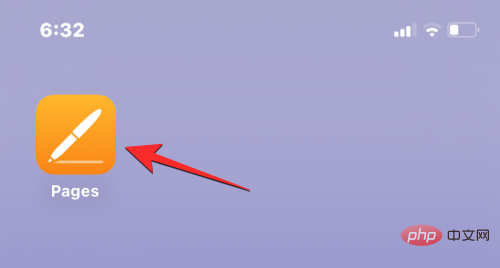
颜色有助于我们在视觉上处理事物的方式,因此在文档、电子邮件、锁定屏幕和其他元素中使用各种颜色看起来更美观。与字体样式一样,选择不同的字体颜色可能是避免手机上的文字看起来单调的好方法。如何在Pages应用程序中更改字体颜色您可以在iPhone上更改文档的文本颜色,也可以在iOS上打开Pages应用程序来完成。在Pages内,点击要打开的文档。如果文档在屏幕视图中打开,请点击右上角的编辑按钮。所选文档现在将进入编辑模式。要更改此文档中文本的字体颜色,请点击所需文本以突出显示它。突出显
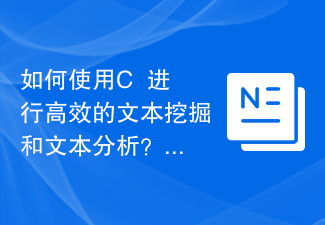
如何使用C++进行高效的文本挖掘和文本分析?概述:文本挖掘和文本分析是现代数据分析和机器学习领域中的重要任务。在本文中,我们将介绍如何使用C++语言来进行高效的文本挖掘和文本分析。我们将着重讨论文本预处理、特征提取和文本分类等方面的技术,并配以代码示例。文本预处理:在进行文本挖掘和文本分析之前,通常需要对原始文本进行预处理。预处理包括去除标点符号、停用词和特
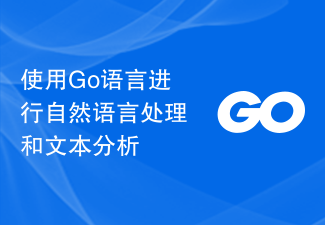
自然语言处理(NaturalLanguageProcessing,NLP)是一种涉及计算机科学、人工智能、语言学等多个学科的交叉领域。其目的是帮助计算机理解、解释和生成自然语言的能力。文本分析(TextAnalysis)则是NLP的其中一个重要方向,其主要目的是从大量文本数据中提取有意义的信息,以支持商业决策、语言学研究、舆情分析等应用场景。Go语言在
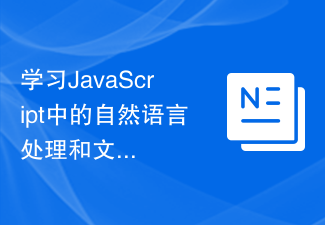
学习JavaScript中的自然语言处理和文本分析,需要具体代码示例自然语言处理(NaturalLanguageProcessing,简称NLP)是一门涉及人工智能和计算机科学的学科,它研究计算机与人类自然语言之间的交互。在当今信息技术高速发展的背景下,NLP在各个领域都有广泛应用,例如智能客服、机器翻译、文本挖掘等。JavaScript作为一门前端开发
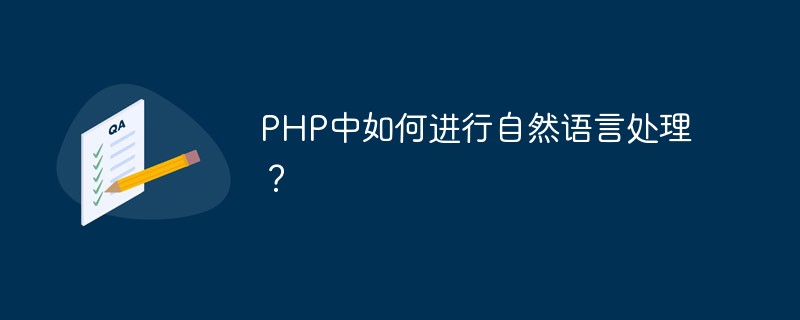
PHP是一种强大的编程语言,它是一种流行的Web开发语言,被广泛用于网站和应用程序的开发中。除了用于网站编程,PHP还可以用于自然语言处理。在这篇文章中,我们将介绍如何在PHP中进行自然语言处理。自然语言处理(NaturalLanguageProcessing,NLP)指的是将计算机科学和人类语言学相结合的一种领域。NLP主要用于使计算机能够理解和处理人
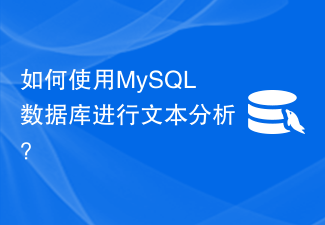
如何使用MySQL数据库进行文本分析?随着大数据时代的到来,文本分析成为了一项非常重要的技术。而MySQL作为一种流行的关系型数据库,也可以用于进行文本分析。本文将介绍如何使用MySQL数据库进行文本分析,并提供相应的代码示例。创建数据库和表首先,我们需要创建一个MySQL数据库和表来存储文本数据。可以使用如下的SQL语句创建一个名为"analysis"的数
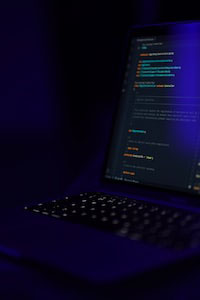
情感分析,又称意见挖掘,是自然语言处理的重要分支,旨在理解和识别文本中的情绪和情感。情感分析在许多领域都有广泛的应用,例如舆情分析、客户满意度分析、产品评价分析等。在本教程中,我们将使用pythonNLTK库来实现情感分析,并演示如何洞察用户的情绪。首先,我们需要导入必要的库:importnltkimportnumpyasnpimportpandasaspdimportmatplotlib.pyplotasplt接下来,我们需要下载并加载情感词典。NLTK提供了许多情感词典,其中一个常用的词典是
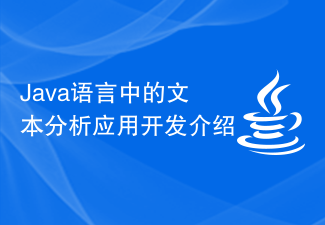
Java语言作为一种通用的编程语言,已经成为了程序员们广泛使用的首选语言之一。它不仅可以用来开发各种桌面应用程序和基于Web的应用程序,还可以用来开发数据分析、人工智能、机器学习等应用程序。其中,文本分析应用也是其中的一个非常重要的应用领域。文本分析应用是指通过对文本数据进行分析,从中提取出有用的信息,如关键词、情感极性、主题等,为用户提供更加高效的信息处理


Hot AI Tools

Undresser.AI Undress
AI-powered app for creating realistic nude photos

AI Clothes Remover
Online AI tool for removing clothes from photos.

Undress AI Tool
Undress images for free

Clothoff.io
AI clothes remover

AI Hentai Generator
Generate AI Hentai for free.

Hot Article

Hot Tools

SAP NetWeaver Server Adapter for Eclipse
Integrate Eclipse with SAP NetWeaver application server.

SublimeText3 Linux new version
SublimeText3 Linux latest version

MinGW - Minimalist GNU for Windows
This project is in the process of being migrated to osdn.net/projects/mingw, you can continue to follow us there. MinGW: A native Windows port of the GNU Compiler Collection (GCC), freely distributable import libraries and header files for building native Windows applications; includes extensions to the MSVC runtime to support C99 functionality. All MinGW software can run on 64-bit Windows platforms.
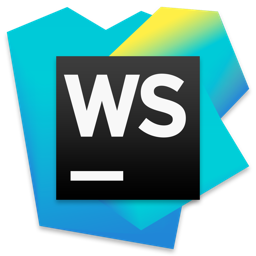
WebStorm Mac version
Useful JavaScript development tools
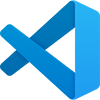
VSCode Windows 64-bit Download
A free and powerful IDE editor launched by Microsoft
