


- ##Paper address: https://arxiv.org/pdf/2302.09778v2.pdf
- Project address: https://github.com/damo-vilab/composer
The latest research provides a new generative paradigm that can flexibly control the output image (such as spatial layout and color palette) while maintaining composition quality and model creation. force.
This research takes compositionality as the core idea. It first decomposes the image into representative factors, and then trains a diffusion model conditioned on these factors to reorganize the input. During the inference phase, rich intermediate representations serve as composable elements, providing a huge design space for the creation of customizable content (i.e., exponentially proportional to the number of decomposition factors). It is worth noting that the method named Composer supports various levels of conditions, such as text descriptions as global information, depth maps and sketches as local guidance, color histograms as low-level details, etc.
In addition to improving controllability, the study confirms that Composer can serve as a general framework that facilitates a wide range of classical generation tasks without the need for retraining.
Method
The framework introduced in this article includes a decomposition stage (the image is divided into a set of independent components) and a synthesis stage (the components are recombined using a conditional diffusion model) . Here we first briefly introduce the diffusion model and guidance direction implemented using Composer, and then detail the implementation of image decomposition and synthesis.
2.1. Diffusion model
The diffusion model is a generative model that generates data from Gaussian noise through an iterative denoising process. generate data. Usually a simple mean square error is used as the denoising target:
where x_0 is an optional condition For the training data of c,
is additive Gaussian noise, a_t and σ_t are scalar functions of t, and is a diffusion model with learnable parameters θ. Classifier-free bootstrapping has been most widely used in recent work for conditional data sampling of diffusion models, where the predicted noise is adjusted by:
In the formula
Guidance direction: Composer is a diffusion model that can accept a variety of conditions and can achieve various directions without classifier guidance:
c_1 and c_2 are two sets of conditions. Different choices of c_1 and c_2 represent different emphasis on the condition.
The conditions within (c_2 c_1) are emphasized as ω, the conditions within (c_1 c_2) are suppressed as (1−ω), and the guidance weight of the conditions within c1∩c2 is 1.0. . Bidirectional guidance: By using condition c_1 to invert the image x_0 to the underlying x_T, and then using another condition c_2 to sample from x_T, we are able to use Composer to manipulate the image in a disentangled way, where the direction of manipulation is between c_2 and c_1 defined by differences.
Decomposition
Study on decomposing images into decoupled representations that capture various aspects of the image, and describe the task The eight representations used in , these representations are extracted in real time during the training process.
Description (Caption) : Study the direct use of title or description information in image-text training data ( For example, LAION-5B (Schuhmann et al., 2022) ) as an image illustration. Pretrained images can also be leveraged to illustrate the model when annotations are not available. We characterize these titles using sentence and word embeddings extracted from the pre-trained CLIP ViT-L /14@336px (Radford et al., 2021) model.
Semantics and style: Study images extracted using the pre-trained CLIP ViT-L/14@336px model Embeddings are used to characterize the semantics and style of images, similar to unCLIP.
Color: Study the color statistics of images using smoothed CIELab histograms. Quantize the CIELab color space into 11 hue values, 5 saturation and 5 light values, using a smoothing sigma of 10. From experience, this setting works better.
Sketch : Study the application of edge detection models and then use sketch simplification algorithms to extract sketches of images. Sketch captures local details of an image with less semantics.
Instances: Study using the pre-trained YOLOv5 model to apply instance segmentation to images to extract their instance masks. Instance segmentation masks reflect the category and shape information of visual objects.
Depthmap : Study the use of pre-trained monocular depth estimation model to extract the depth map of the image and roughly capture the image Layout.
Intensity: The study introduces the original grayscale image as a representation, forcing the model to learn to deal with the disentangled degree of freedom of color. To introduce randomness, we uniformly sample from a set of predefined RGB channel weights to create grayscale images.
Masking : Study the introduction of image masks to enable Composer to limit image generation or operations to editable areas . A 4-channel representation is used, where the first 3 channels correspond to the masked RGB image and the last channel corresponds to the binary mask.
It should be noted that although this article conducted experiments using the above eight conditions, users can freely customize the conditions using Composer.
Composition
Studies using diffusion models to recombine images from a set of representations. Specifically, the study exploits the GLIDE architecture and modifies its tuning module. The study explores two different mechanisms to adapt models based on representations:
Global conditioning: For global representations including CLIP sentence embeddings, image embeddings, and color palettes, we project and add them to time-step embeddings. Additionally, we project image embeddings and color palettes into eight additional tokens and concatenate them with CLIP word embeddings, which are then used as context for cross-attention in GLIDE, similar to unCLIP. Since conditions are either additive or can be selectively masked in cross-attention, conditions can be dropped directly during training and inference, or new global conditions introduced.
Localization conditioning: For localized representations, including sketches, segmentation masks, depth maps, intensity images, and mask images, we use stacked convolutional layers to project them with noise The latent x_t has uniform-dimensional embeddings with the same spatial size. The sum of these embeddings is then calculated and the result is concatenated to x_t, which is then fed into UNet. Since embeddings are additive, it is easy to adapt missing conditions or incorporate new localized conditions.
Joint training strategy: It is important to design a joint training strategy that enables the model to learn to decode images from various combinations of conditions. The study experimented with several configurations and identified a simple yet effective configuration that uses an independent exit probability of 0.5 for each condition, a probability of 0.1 for removing all conditions, and a probability of 0.1 for keeping all conditions. A special dropout probability of 0.7 is used for intensity images since they contain the vast majority of information about the image and may weaken other conditions during training.
The basic diffusion model produces a 64 × 64 resolution image. To generate high-resolution images, we trained two unconditional diffusion models for upsampling, respectively upsampling images from 64 × 64 to 256 × 256, and from 256 × 256 to 1024 × 1024 resolution. The architecture of the upsampling model is modified from unCLIP, where the use of more channels in low-resolution layers is studied and self-attention blocks are introduced to expand the capacity. An optional prior model is also introduced that generates image embeddings from subtitles. Empirically, prior models can improve the diversity of generated images under specific combinations of conditions.
Experiment
Variation: Using Composer you can create a new image that is similar to a given image, but conditioned on a specific subset of its representation. It's different in some ways. By carefully choosing combinations of different representations, one can flexibly control the range of image changes (Fig. 2a). After incorporating more conditions, the method presented in the study generates a variant of unCLIP that only conditions on the image embedding: using Composer it is possible to create new images that are similar to a given image, but conditional on a specific subset of its representation. Reflection, is different in some ways. By carefully choosing combinations of different representations, one can flexibly control the range of image changes (Fig. 2a). After incorporating more conditions, the proposed method achieves higher reconstruction accuracy than unCLIP, which is only conditioned on image embeddings.
The above is the detailed content of New ideas for AI painting: Domestic open source new model with 5 billion parameters, achieving a leap in synthetic controllability and quality. For more information, please follow other related articles on the PHP Chinese website!
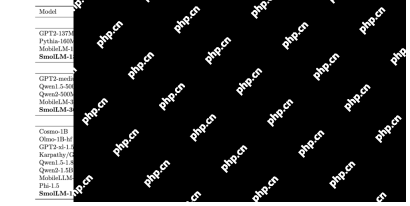
Harness the Power of On-Device AI: Building a Personal Chatbot CLI In the recent past, the concept of a personal AI assistant seemed like science fiction. Imagine Alex, a tech enthusiast, dreaming of a smart, local AI companion—one that doesn't rely
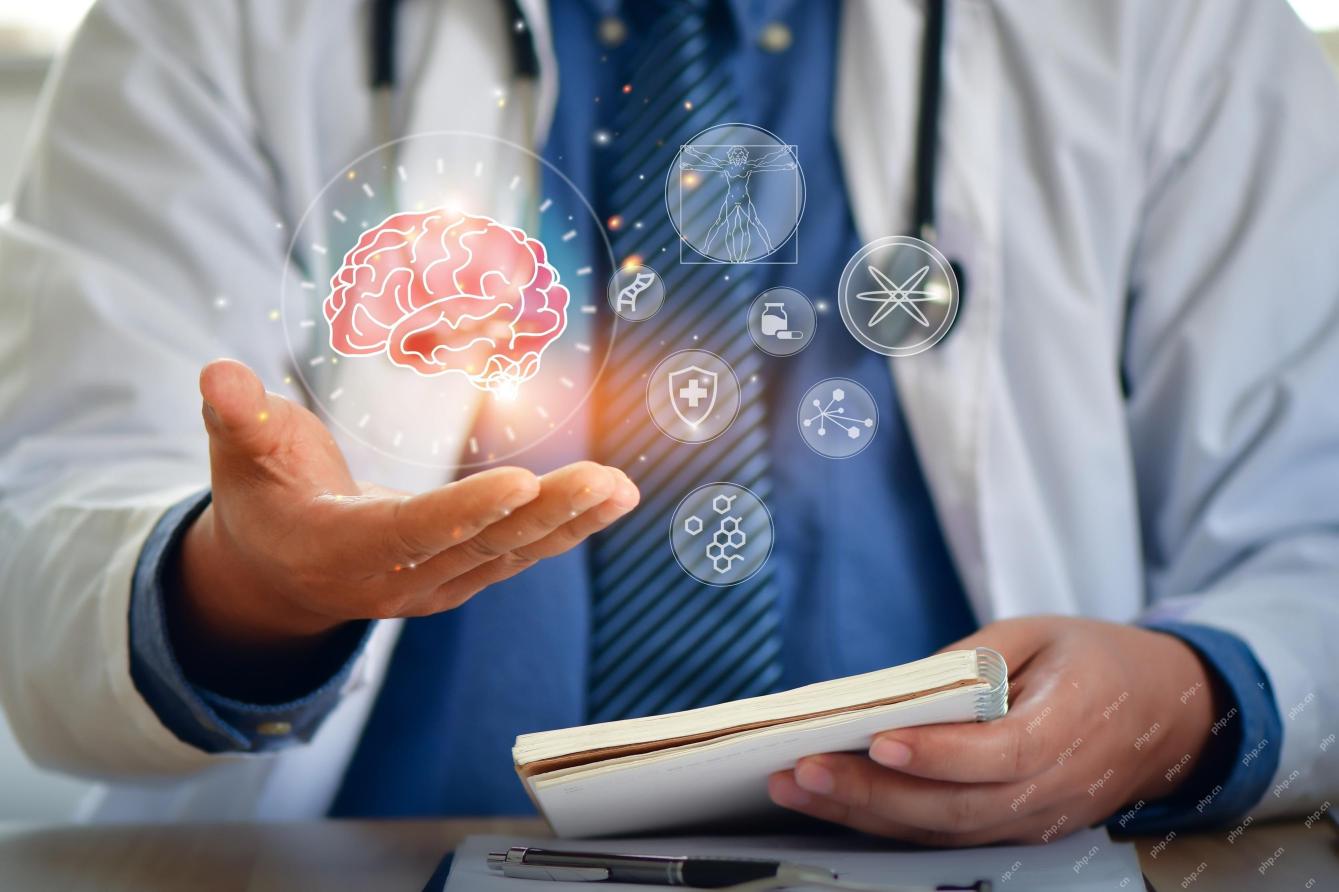
Their inaugural launch of AI4MH took place on April 15, 2025, and luminary Dr. Tom Insel, M.D., famed psychiatrist and neuroscientist, served as the kick-off speaker. Dr. Insel is renowned for his outstanding work in mental health research and techno
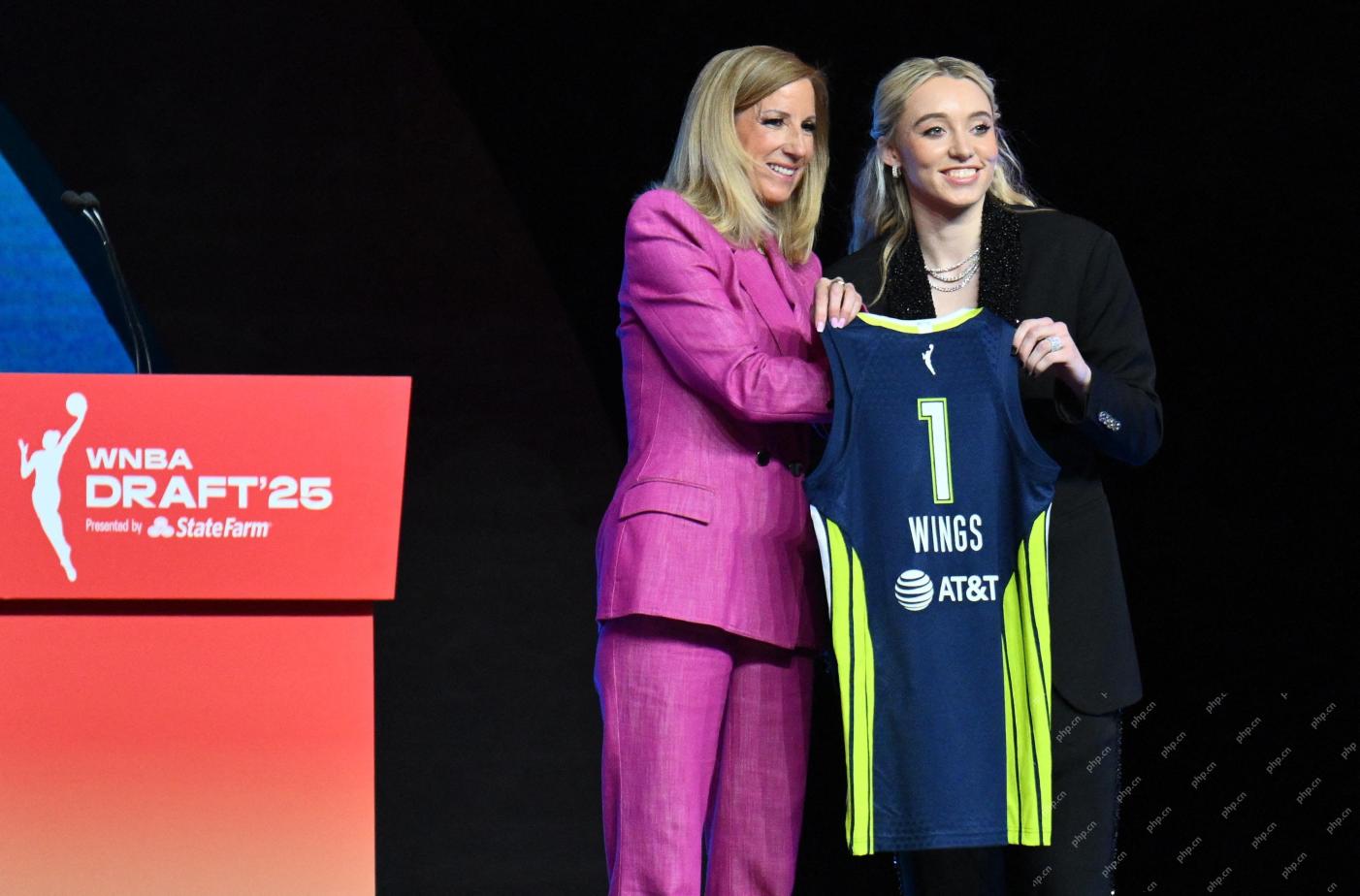
"We want to ensure that the WNBA remains a space where everyone, players, fans and corporate partners, feel safe, valued and empowered," Engelbert stated, addressing what has become one of women's sports' most damaging challenges. The anno
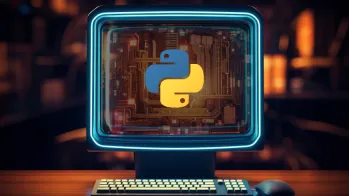
Introduction Python excels as a programming language, particularly in data science and generative AI. Efficient data manipulation (storage, management, and access) is crucial when dealing with large datasets. We've previously covered numbers and st
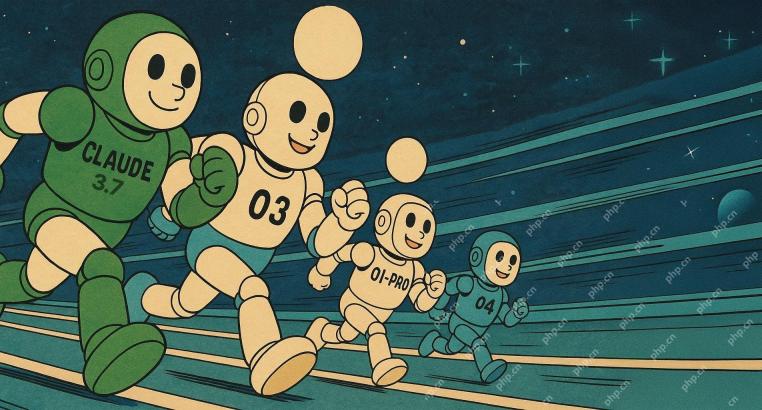
Before diving in, an important caveat: AI performance is non-deterministic and highly use-case specific. In simpler terms, Your Mileage May Vary. Don't take this (or any other) article as the final word—instead, test these models on your own scenario
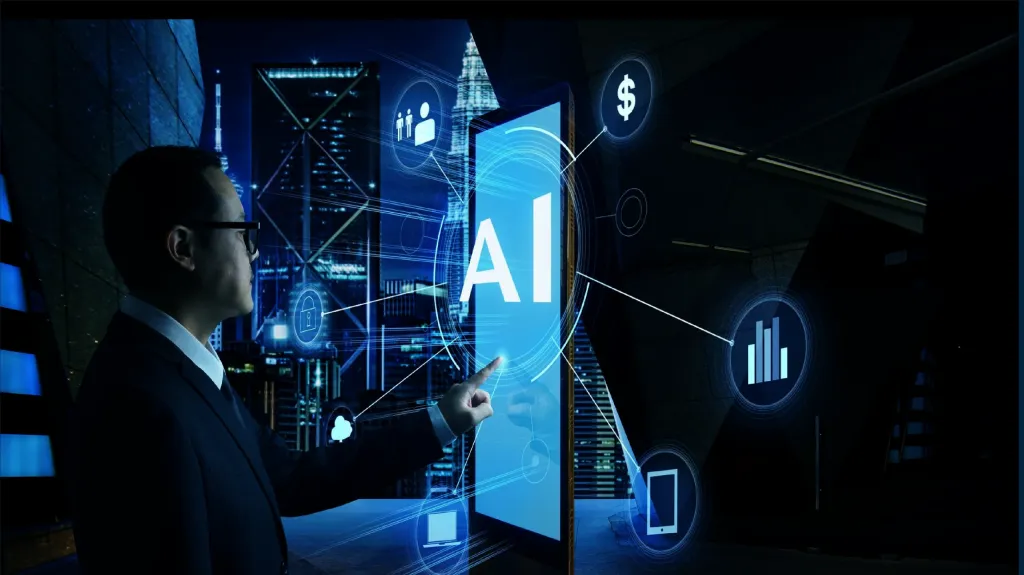
Building a Standout AI/ML Portfolio: A Guide for Beginners and Professionals Creating a compelling portfolio is crucial for securing roles in artificial intelligence (AI) and machine learning (ML). This guide provides advice for building a portfolio
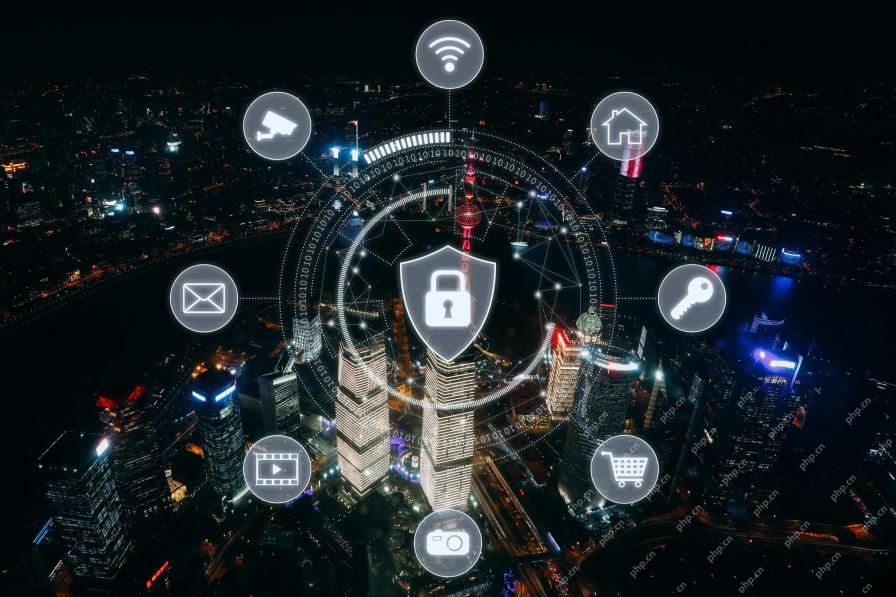
The result? Burnout, inefficiency, and a widening gap between detection and action. None of this should come as a shock to anyone who works in cybersecurity. The promise of agentic AI has emerged as a potential turning point, though. This new class
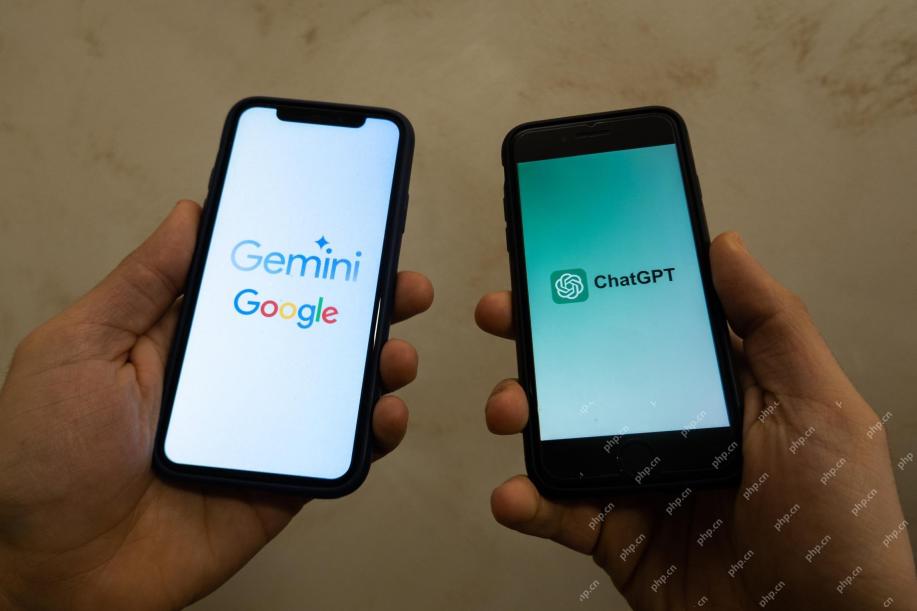
Immediate Impact versus Long-Term Partnership? Two weeks ago OpenAI stepped forward with a powerful short-term offer, granting U.S. and Canadian college students free access to ChatGPT Plus through the end of May 2025. This tool includes GPT‑4o, an a


Hot AI Tools

Undresser.AI Undress
AI-powered app for creating realistic nude photos

AI Clothes Remover
Online AI tool for removing clothes from photos.

Undress AI Tool
Undress images for free

Clothoff.io
AI clothes remover

AI Hentai Generator
Generate AI Hentai for free.

Hot Article

Hot Tools

mPDF
mPDF is a PHP library that can generate PDF files from UTF-8 encoded HTML. The original author, Ian Back, wrote mPDF to output PDF files "on the fly" from his website and handle different languages. It is slower than original scripts like HTML2FPDF and produces larger files when using Unicode fonts, but supports CSS styles etc. and has a lot of enhancements. Supports almost all languages, including RTL (Arabic and Hebrew) and CJK (Chinese, Japanese and Korean). Supports nested block-level elements (such as P, DIV),
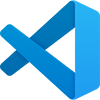
VSCode Windows 64-bit Download
A free and powerful IDE editor launched by Microsoft
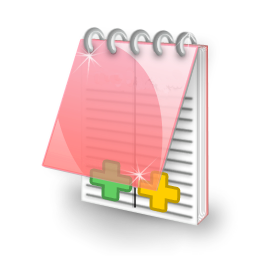
EditPlus Chinese cracked version
Small size, syntax highlighting, does not support code prompt function

MantisBT
Mantis is an easy-to-deploy web-based defect tracking tool designed to aid in product defect tracking. It requires PHP, MySQL and a web server. Check out our demo and hosting services.

SublimeText3 Chinese version
Chinese version, very easy to use