


Diffusion + target detection = controllable image generation! The Chinese team proposed GLIGEN to perfectly control the spatial position of objects
With the open source of Stable Diffusion, the use of natural language for image generation has gradually become popular. Many AIGC problems have also been exposed, such as AI cannot draw hands, cannot understand action relationships, and is difficult to control the position of objects. wait.
The main reason is that the "input interface" only has natural language, cannot achieve fine control of the screen.
Recently, research hotspots from the University of Wisconsin-Madison, Columbia University and Microsoft have proposed a brand new method GLIGEN, which uses grounding input as a condition to convert existing "pre-trained text to image" The functionality of Diffusion Model has been expanded.
Paper link: https://arxiv.org/pdf/2301.07093.pdf
Project home page: https://gligen.github.io/
Experience link: https: //huggingface.co/spaces/gligen/demo
In order to retain the large amount of conceptual knowledge of the pre-trained model, the researchers did not choose to fine-tune the model, but through gating The mechanism injects different input grounding conditions into new trainable layers to achieve control over open-world image generation.
Currently GLIGEN supports four inputs.
(Upper left) Text entity box (Upper right) Image entity box
(Bottom left) Image style text box (Bottom right) Text entity key points
The experimental results also show that GLIGEN has better performance in COCO and LVIS The zero-shot performance is significantly better than the current supervised layout-to-image baseline.
Controllable image generation
Before the diffusion model, generative adversarial networks (GANs) have always been the leader in the field of image generation, and their latent space and conditional input are in " The aspects of "controllable operations" and "generation" have been fully studied.
Text conditional autoregressive and diffusion models exhibit amazing image quality and concept coverage, thanks to their more stable learning objectives and large-scale access to network image-text paired data Train and quickly get out of the circle, becoming a tool to assist art design and creation.
However, existing large-scale text-image generation models cannot be conditioned on other input modes "besides text" and lack the concept of precise positioning or the use of reference images to control the generation process. Ability limits the expression of information.
For example, it is difficult to describe the precise location of an object using text, while bounding boxes (bounding
boxes) or keypoints (keypoints ) can be easily implemented.
Some existing tools such as inpainting, layout2img generation, etc. can use modal input other than text, but they are These inputs are rarely combined for controlled text2img generation.
In addition, previous generative models are usually trained independently on task-specific datasets, while in the field of image recognition, the long-standing paradigm is to learn from "large-scale image data" Or pre-trained basic models on "image-text pairs" to start building models for specific tasks.
Diffusion models have been trained on billions of image-text pairs. A natural question is: can we build on existing pre-trained diffusion models? , give them a new conditional input mode?
Due to the large amount of conceptual knowledge possessed by the pre-trained model, it may be possible to achieve better performance on other generation tasks while obtaining more data than existing text-image generation models. control.
GLIGEN
Based on the above purposes and ideas, the GLIGEN model proposed by the researchers still retains the text title as input, but also enables other input modalities, such as the grounding concept The bounding box, grounding reference image and key points of the grounding part.
The key problem here is to retain a large amount of original conceptual knowledge in the pre-trained model while learning to inject new grounding information.
In order to prevent knowledge forgetting, researchers proposed to freeze the original model weights and add a new trainable gated Transformer layer to absorb new grouding inputs. The following uses bounding boxes as an example. .
Command input
Each grouding text entity is represented as a bounding box, containing the coordinate values of the upper left corner and lower right corner.
It should be noted that existing layout2img related work usually requires a concept dictionary and can only handle close-set entities (such as COCO categories) during the evaluation phase. Researchers found that using Text encoders encoding image descriptions can generalize the location information in the training set to other concepts.
Training data
is used to generate grounding images The training data requires text c and grounding entity e as conditions. In practice, the data requirements can be relaxed by considering more flexible inputs.
There are three main types of data
1. grounding data
#Each image is associated with a caption that describes the entire image; noun entities are extracted from the caption and labeled with bounding boxes.
Since noun entities are taken directly from natural language titles, they can cover a richer vocabulary, which is beneficial to the grounding generation of open-world vocabulary.
2. Detection data
The noun entity is a predefined close-set category (for example, in COCO 80 object categories), choose to use the empty title token in the classifier-free guide as the title.
The amount of detection data (millions level) is larger than the basic data (thousands of levels), so the overall training data can be greatly increased.
3. Detection and Caption data
The noun entity is the same as the noun entity in the detection data , while the image is described with a text title alone, there may be cases where the noun entity is not completely consistent with the entity in the title.
For example, the title only gives a high-level description of the living room and does not mention the objects in the scene, while the detection annotation provides finer object-level details.
Gated attention mechanism
Researcher The goal is to give existing large-scale language-image generative models new spatially based capabilities.
#Large-scale diffusion models have been pre-trained on network-scale image text to obtain The knowledge required to synthesize realistic images based on diverse and complex language instructions. Since pre-training is expensive and performs well, it is important to retain this knowledge in the model weights while extending new capabilities. This can be achieved by Adapt new modules to accommodate new capabilities over time.
During the training process, the gating mechanism is used to gradually integrate new grounding information into the pre-trained model. This design allows flexibility in the sampling process during generation to improve quality and controllability.
The experiment also proved that using the complete model (all layers) in the first half of the sampling step, and only using the original layer (no gated Transformer layer) in the second half, generates The results can more accurately reflect grounding conditions and have higher image quality.
Experimental part
In the open set grounded text to image generation task, first use only the basic annotations of COCO (COCO2014CD) for training, and evaluate whether GLIGEN can generate basic entities other than the COCO category. .
It can be seen that GLIGEN can learn new concepts such as "blue jay", "croissant", or new Object attributes such as "brown wooden table", and this information does not appear in the training category.
The researchers believe this is because GLIGEN's gated self-attention learned to reposition visual features corresponding to grounded entities in the title for the following cross-attention layer, and Generalization capabilities are gained due to the shared text space in these two layers.
The experiment also quantitatively evaluated the zero-shot generation performance of this model on LVIS, which contains 1203 long-tail object categories. Use GLIP to predict bounding boxes from generated images and calculate AP, named GLIP score; compare it with state-of-the-art models designed for the layout2img task,
It can be found that although the GLIGEN model is only trained on COCO annotations, it is much better than the supervised baseline, probably because the baseline trained from scratch is difficult to start from Learning from limited annotations, while the GLIGEN model can utilize the large amount of conceptual knowledge of the pre-trained model.
Overall, this paper:
1. A new text2img generation method is proposed, which gives the existing text2img diffusion model new grounding controllability;
2. By retaining the pre-trained weights And learning gradually integrates new positioning layers, the model realizes open-world grounded text2img generation and bounding box input, that is, it integrates new positioning concepts not observed in training;
3. The zero-shot performance of this model on the layout2img task is significantly better than the previous state-of-the-art level, proving that large-scale pre-trained generative models can improve the performance of downstream tasks
The above is the detailed content of Diffusion + target detection = controllable image generation! The Chinese team proposed GLIGEN to perfectly control the spatial position of objects. For more information, please follow other related articles on the PHP Chinese website!
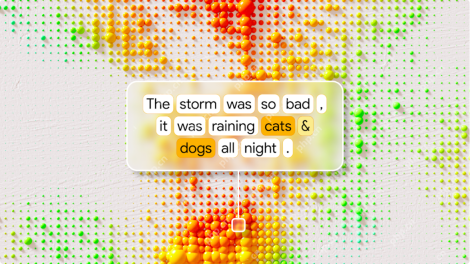
Exploring the Inner Workings of Language Models with Gemma Scope Understanding the complexities of AI language models is a significant challenge. Google's release of Gemma Scope, a comprehensive toolkit, offers researchers a powerful way to delve in
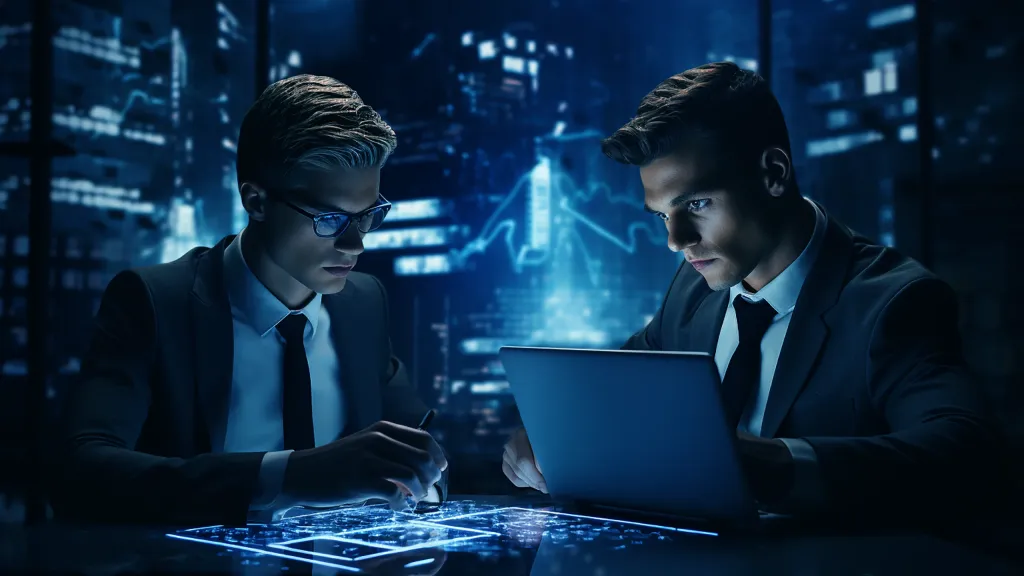
Unlocking Business Success: A Guide to Becoming a Business Intelligence Analyst Imagine transforming raw data into actionable insights that drive organizational growth. This is the power of a Business Intelligence (BI) Analyst – a crucial role in gu

SQL's ALTER TABLE Statement: Dynamically Adding Columns to Your Database In data management, SQL's adaptability is crucial. Need to adjust your database structure on the fly? The ALTER TABLE statement is your solution. This guide details adding colu
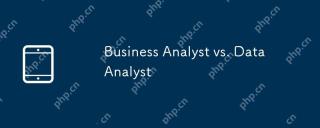
Introduction Imagine a bustling office where two professionals collaborate on a critical project. The business analyst focuses on the company's objectives, identifying areas for improvement, and ensuring strategic alignment with market trends. Simu
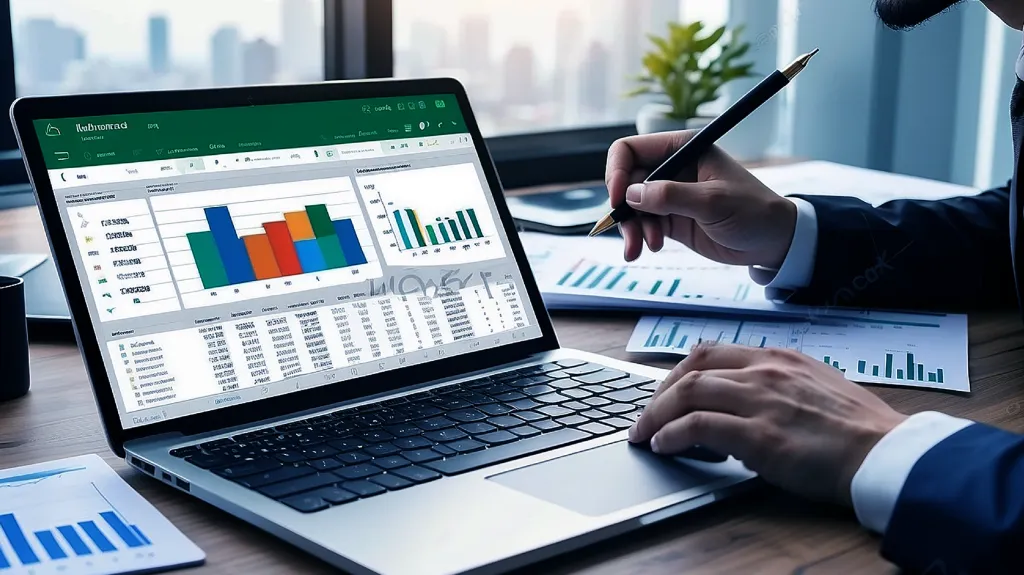
Excel data counting and analysis: detailed explanation of COUNT and COUNTA functions Accurate data counting and analysis are critical in Excel, especially when working with large data sets. Excel provides a variety of functions to achieve this, with the COUNT and COUNTA functions being key tools for counting the number of cells under different conditions. Although both functions are used to count cells, their design targets are targeted at different data types. Let's dig into the specific details of COUNT and COUNTA functions, highlight their unique features and differences, and learn how to apply them in data analysis. Overview of key points Understand COUNT and COU
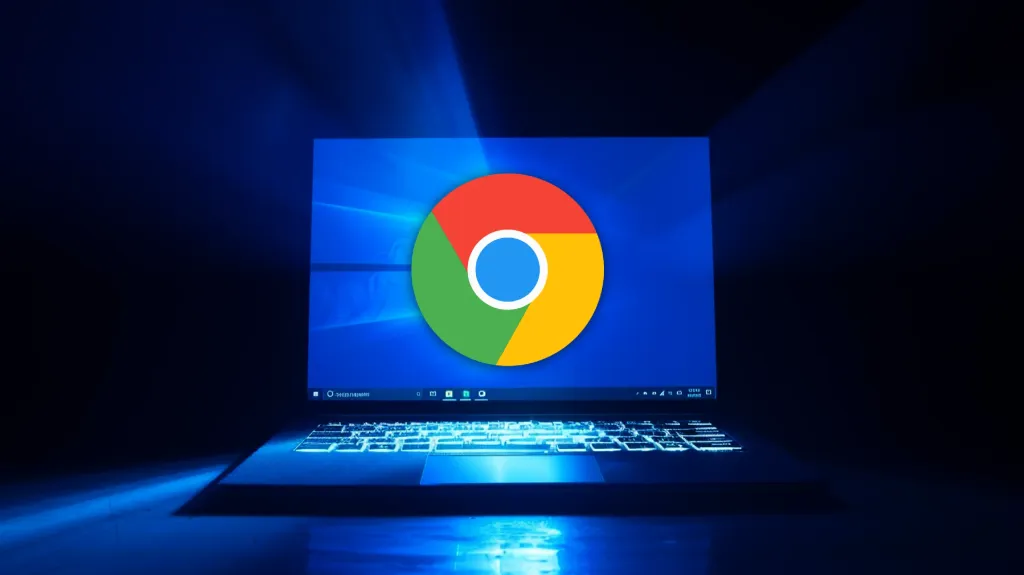
Google Chrome's AI Revolution: A Personalized and Efficient Browsing Experience Artificial Intelligence (AI) is rapidly transforming our daily lives, and Google Chrome is leading the charge in the web browsing arena. This article explores the exciti
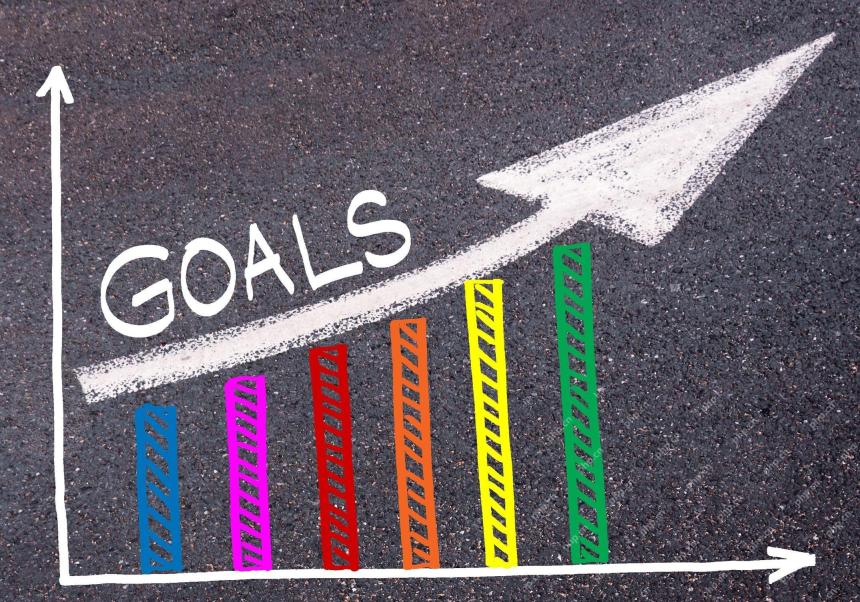
Reimagining Impact: The Quadruple Bottom Line For too long, the conversation has been dominated by a narrow view of AI’s impact, primarily focused on the bottom line of profit. However, a more holistic approach recognizes the interconnectedness of bu
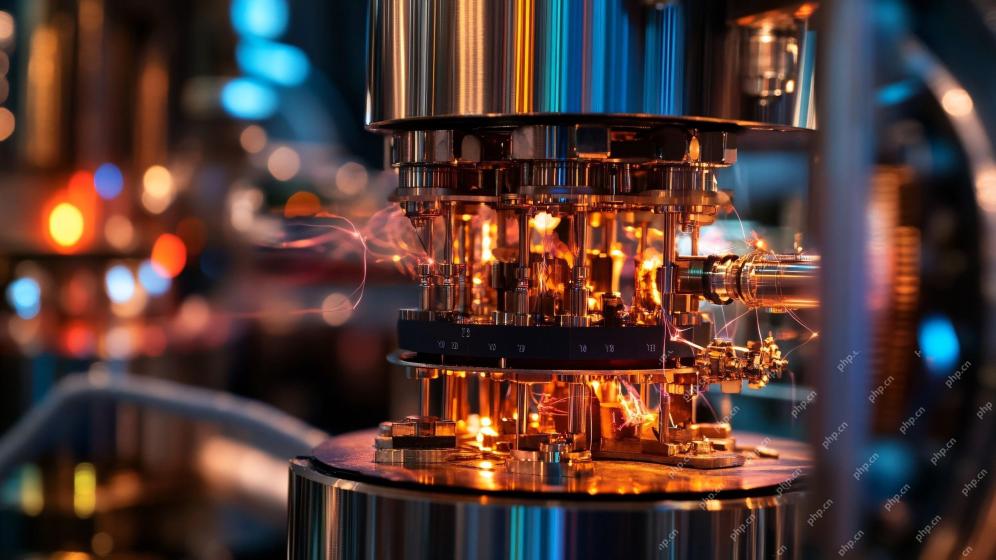
Things are moving steadily towards that point. The investment pouring into quantum service providers and startups shows that industry understands its significance. And a growing number of real-world use cases are emerging to demonstrate its value out


Hot AI Tools

Undresser.AI Undress
AI-powered app for creating realistic nude photos

AI Clothes Remover
Online AI tool for removing clothes from photos.

Undress AI Tool
Undress images for free

Clothoff.io
AI clothes remover

AI Hentai Generator
Generate AI Hentai for free.

Hot Article

Hot Tools

Notepad++7.3.1
Easy-to-use and free code editor
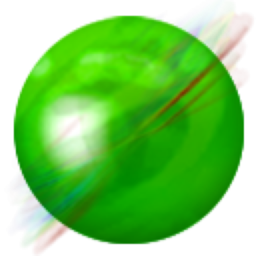
ZendStudio 13.5.1 Mac
Powerful PHP integrated development environment
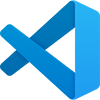
VSCode Windows 64-bit Download
A free and powerful IDE editor launched by Microsoft

MantisBT
Mantis is an easy-to-deploy web-based defect tracking tool designed to aid in product defect tracking. It requires PHP, MySQL and a web server. Check out our demo and hosting services.

SecLists
SecLists is the ultimate security tester's companion. It is a collection of various types of lists that are frequently used during security assessments, all in one place. SecLists helps make security testing more efficient and productive by conveniently providing all the lists a security tester might need. List types include usernames, passwords, URLs, fuzzing payloads, sensitive data patterns, web shells, and more. The tester can simply pull this repository onto a new test machine and he will have access to every type of list he needs.