


A complete set of tutorials for adapting the Diffusers framework is here! From T2I-Adapter to the popular ControlNet
Not long after ChatGPT came out of the circle, the emergence of ControlNet quickly gained many developers and ordinary users on the English and Chinese Internet. Some users even promoted that the emergence of ControlNet brought AI creation into the era of upright walking. . It is no exaggeration to say that, including ControlNet, the T2I-Adapter, Composer, and LoRA training techniques of the same period, controllable generation, as the last high wall of AI creation, is very likely to have further breakthroughs in the foreseeable time, thus greatly Reduce the user's creation costs and improve the playability of creation. In just two weeks since ControlNet was open sourced, its official Star count has exceeded 10,000. This popularity is undoubtedly unprecedented.
At the same time, the open source community has also greatly lowered the threshold for users. For example, the Hugging Face platform provides basic model weights and general model training framework diffusers, stable-diffusion-webui A complete Demo platform has been developed, and Civitai has contributed a large number of stylized LoRA weights.
##Although webui is currently the most popular The visualization tool has quickly supported various recently launched generative models and supports many options for users to set. Because it focuses on the ease of use of the front-end interface, the code structure behind it is actually very complex and not friendly enough for developers. For example, although webui supports multiple types of loading and inference, it cannot support conversion under different frameworks, nor can it support flexible training of models. In community discussions, we discovered many pain points that have not yet been solved by existing open source code.
First of all, the code framework is not compatible. Currently popular models, such as ControlNet and T2I-Adapter, are not compatible with the mainstream Stable Diffusion training library. diffusers is not compatible, ControlNet pre-trained models cannot be used directly in the diffusers framework.
Secondly, Model loading is limited. Currently, models are saved in various formats, such as .bin, .ckpt, .pth, .satetensors, etc. , in addition to webui, the diffusers framework currently has limited support for these model formats. Considering that most LoRA models are mainly saved in safetensors, it is difficult for users to directly load LoRA models into existing models trained based on the diffusers framework.
Third, The basic model is limited. Currently, ControlNet and T2I-Adapter are trained based on Stable-Diffusion-1.5, and only The model weights under SD1.5 are open sourced. Considering specific scenarios, high-quality animation models such as anything-v4 and ChilloutMix already exist. Even if controllable information is introduced, the final generated results are still limited by the capabilities of UNet in SD1.5.
Finally, model training is limited. Currently, LoRA has been widely verified to be one of the most effective methods for style transfer and maintaining a specific image IP. 1. However, the diffusers framework currently only supports UNet's LoRA embedding and cannot support text encoder embedding, which will limit LoRA training.
After discussing with the open source community, we learned that the diffusers framework, as a general code library, is planning to adapt to the recently launched generation models at the same time; because it involves rewriting many underlying interfaces, it is still It will take some time to update. To this end, we started from the above actual problems and took the lead in proposing self-developed solutions for each problem to quickly help developers develop more easily.
Full adaptation solution from LoRA, ControlNet, T2I-Adapter to diffusers##LoRA for diffusers
This solution is to flexibly embed LoRA weights in various formats in the diffusers framework, that is, the model saved based on diffusers training. Since the training of LoRA usually freezes the base model, it can be easily embedded into existing models as pluggable modules as style or IP conditional constraints. LoRA itself is a general training technique. Its basic principle is that through low-rank decomposition, the number of parameters of the module can be greatly reduced. Currently, in image generation, it is generally used to train pluggable modules that are independent of the base model. , the actual use is to merge it with the output of the base model in the form of residuals.The first is the embedding of LoRA weights. Currently, the weights provided on the Civitai platform are mainly stored in ckpt or safetensors format, divided into the following two situations. (1) Full model (base model LoRA module) If the full model is in safetensors format, it can be converted by the following diffusers script If the full model is in ckpt format, it can be converted through the following diffusers script After the conversion is completed, the model can be loaded directly using the API of diffusers (2) LoRA only (only contains LoRA module) Currently diffusers officially cannot support loading only LoRA weights, and on the open source platform The LoRA weights are basically stored in this form. Essentially, it completes the remapping of key-value in LoRA weights and adapts it to the diffusers model. For this reason, we support this feature ourselves and provide conversion scripts. Only need to specify the model in diffusers format, and the LoRA weights stored in safetensors format. We provide an example conversion. In addition, due to its lightweight, LoRA itself can quickly complete training with small data and can be embedded into other networks. In order not to be limited to the existing LoRA weights, we support multi-module (UNet text encoder) training of LoRA in the diffusers framework and have submitted a PR in the official code base (https://github.com/huggingface/diffusers/pull/ 2479), and supports training LoRA in ColossalAI. The code is open source at: https://github.com/haofanwang/Lora-for-Diffusers ControlNet for diffuserspython ./scripts/convert_original_stable_diffusion_to_diffusers.py --checkpoint_path xxx.safetensors--dump_path save_dir --from_safetensors
python ./scripts/convert_original_stable_diffusion_to_diffusers.py --checkpoint_path xxx.ckpt--dump_path save_dir
from diffusers import StableDiffusionPipeline
pipeline = StableDiffusionPipeline.from_pretrained (save_dir,torch_dtype=torch.float32)
pipeline = StableDiffusionPipeline.from_pretrained (model_id,torch_dtype=torch.float32)
model_path = "onePieceWanoSagaStyle_v2Offset.safetensors"
state_dict = load_file (model_path)
# the default mergering ratio is 0.75, you can manually set it
python convert_lora_safetensor_to_diffusers.py
##This solution is to support the use of ControlNet in the diffusers framework. Based on some attempts of the open source community, we provide a complete use case for ControlNet Anything-V3, supporting the replacement of the base model from the original SD1.5 to the anything-v3 model, so that ControlNet has better animation generation capabilities.
In addition, we also support ControlNet Inpainting and provide a pipeline adapted to diffusers,
and Multi-ControlNet for multi-condition control.
The code is open source at: https://github.com/haofanwang/ControlNet-for-Diffusers
T2I-Adapter for diffusers
The code is open source at: https://github.com/haofanwang/T2I-Adapter-for-Diffusers Currently, the above three adaptation solutions have been open sourced to the community, and have been officially acknowledged in ControlNet and T2I-Adapter respectively. They have also received thanks from the author of stable-diffusion-webui-colab. We are maintaining discussions with diffusers officials and will complete the integration of the above solution into the official code base in the near future. You are also welcome to try our work in advance. If you have any questions, you can directly raise an issue and we will reply as soon as possible.
The above is the detailed content of A complete set of tutorials for adapting the Diffusers framework is here! From T2I-Adapter to the popular ControlNet. For more information, please follow other related articles on the PHP Chinese website!
![Can't use ChatGPT! Explaining the causes and solutions that can be tested immediately [Latest 2025]](https://img.php.cn/upload/article/001/242/473/174717025174979.jpg?x-oss-process=image/resize,p_40)
ChatGPT is not accessible? This article provides a variety of practical solutions! Many users may encounter problems such as inaccessibility or slow response when using ChatGPT on a daily basis. This article will guide you to solve these problems step by step based on different situations. Causes of ChatGPT's inaccessibility and preliminary troubleshooting First, we need to determine whether the problem lies in the OpenAI server side, or the user's own network or device problems. Please follow the steps below to troubleshoot: Step 1: Check the official status of OpenAI Visit the OpenAI Status page (status.openai.com) to see if the ChatGPT service is running normally. If a red or yellow alarm is displayed, it means Open
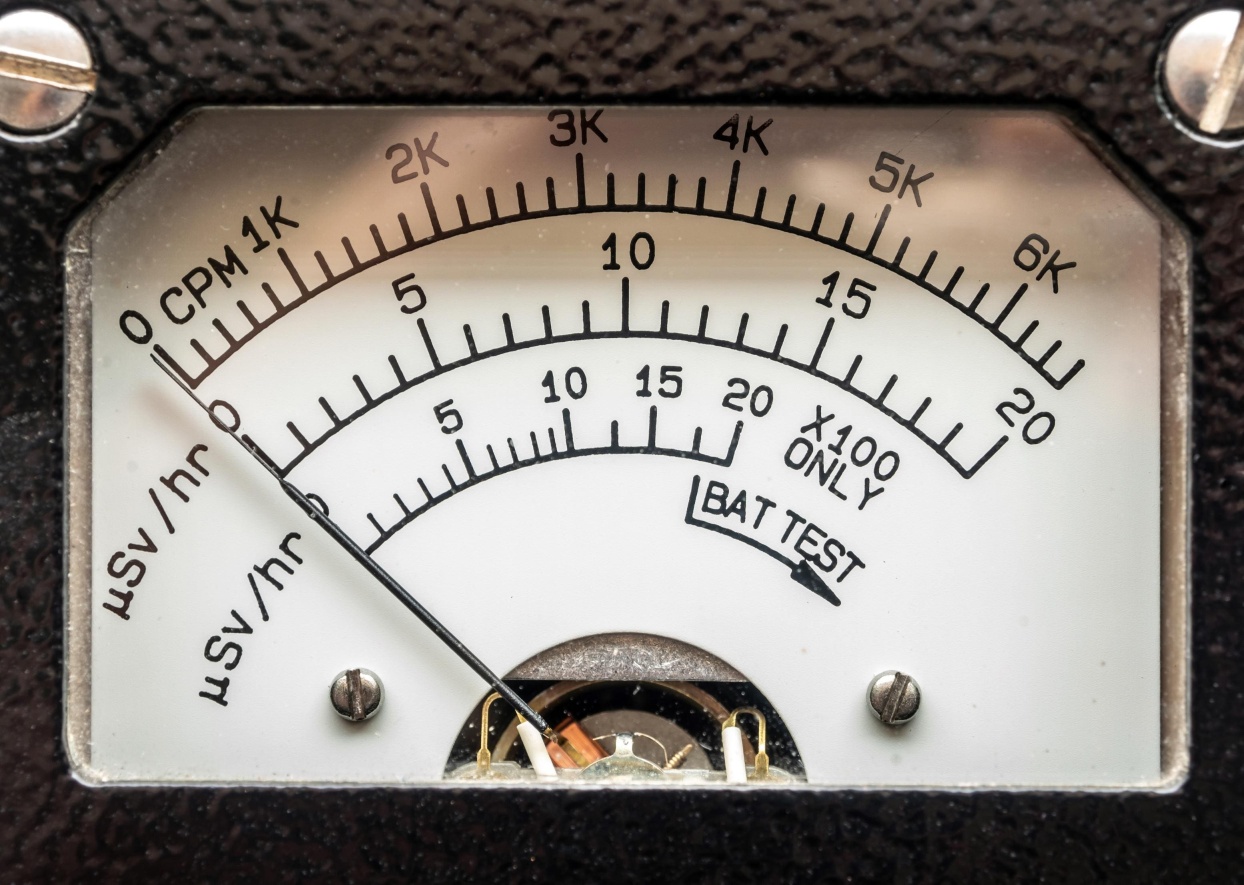
On 10 May 2025, MIT physicist Max Tegmark told The Guardian that AI labs should emulate Oppenheimer’s Trinity-test calculus before releasing Artificial Super-Intelligence. “My assessment is that the 'Compton constant', the probability that a race to
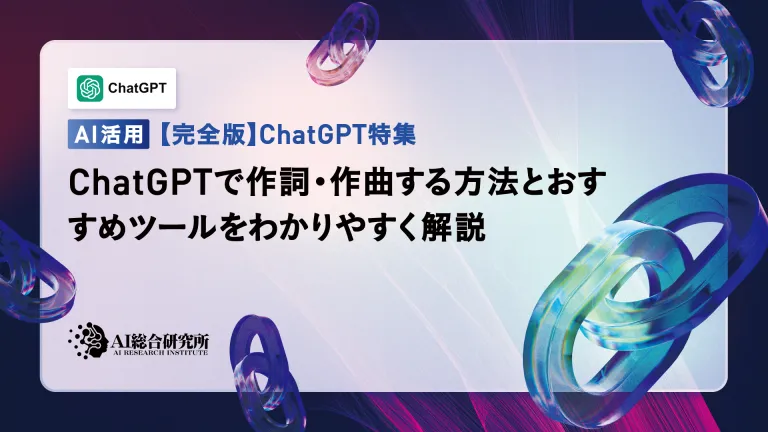
AI music creation technology is changing with each passing day. This article will use AI models such as ChatGPT as an example to explain in detail how to use AI to assist music creation, and explain it with actual cases. We will introduce how to create music through SunoAI, AI jukebox on Hugging Face, and Python's Music21 library. Through these technologies, everyone can easily create original music. However, it should be noted that the copyright issue of AI-generated content cannot be ignored, and you must be cautious when using it. Let’s explore the infinite possibilities of AI in the music field together! OpenAI's latest AI agent "OpenAI Deep Research" introduces: [ChatGPT]Ope
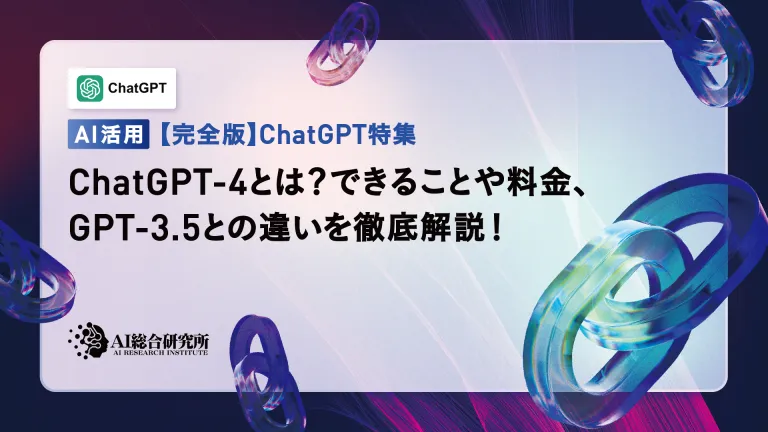
The emergence of ChatGPT-4 has greatly expanded the possibility of AI applications. Compared with GPT-3.5, ChatGPT-4 has significantly improved. It has powerful context comprehension capabilities and can also recognize and generate images. It is a universal AI assistant. It has shown great potential in many fields such as improving business efficiency and assisting creation. However, at the same time, we must also pay attention to the precautions in its use. This article will explain the characteristics of ChatGPT-4 in detail and introduce effective usage methods for different scenarios. The article contains skills to make full use of the latest AI technologies, please refer to it. OpenAI's latest AI agent, please click the link below for details of "OpenAI Deep Research"
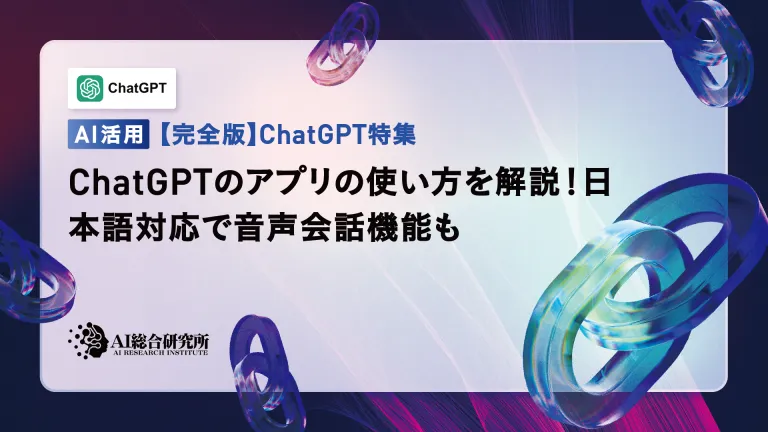
ChatGPT App: Unleash your creativity with the AI assistant! Beginner's Guide The ChatGPT app is an innovative AI assistant that handles a wide range of tasks, including writing, translation, and question answering. It is a tool with endless possibilities that is useful for creative activities and information gathering. In this article, we will explain in an easy-to-understand way for beginners, from how to install the ChatGPT smartphone app, to the features unique to apps such as voice input functions and plugins, as well as the points to keep in mind when using the app. We'll also be taking a closer look at plugin restrictions and device-to-device configuration synchronization
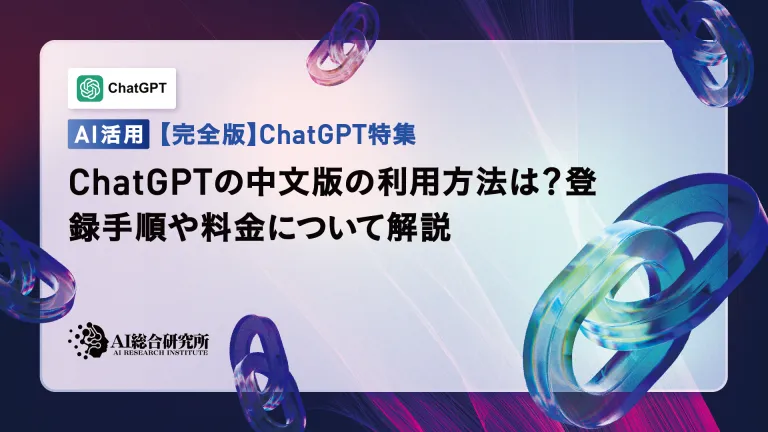
ChatGPT Chinese version: Unlock new experience of Chinese AI dialogue ChatGPT is popular all over the world, did you know it also offers a Chinese version? This powerful AI tool not only supports daily conversations, but also handles professional content and is compatible with Simplified and Traditional Chinese. Whether it is a user in China or a friend who is learning Chinese, you can benefit from it. This article will introduce in detail how to use ChatGPT Chinese version, including account settings, Chinese prompt word input, filter use, and selection of different packages, and analyze potential risks and response strategies. In addition, we will also compare ChatGPT Chinese version with other Chinese AI tools to help you better understand its advantages and application scenarios. OpenAI's latest AI intelligence
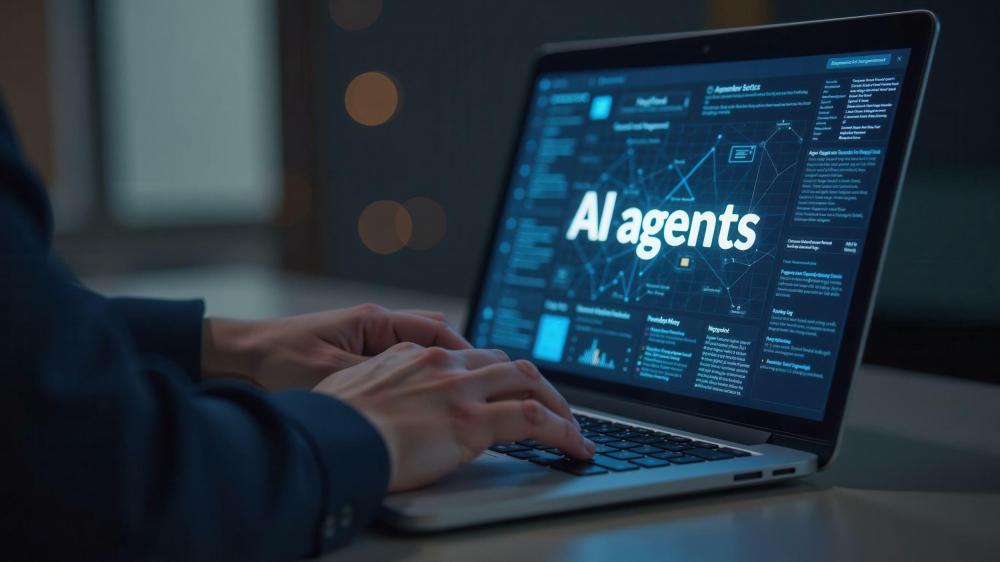
These can be thought of as the next leap forward in the field of generative AI, which gave us ChatGPT and other large-language-model chatbots. Rather than simply answering questions or generating information, they can take action on our behalf, inter
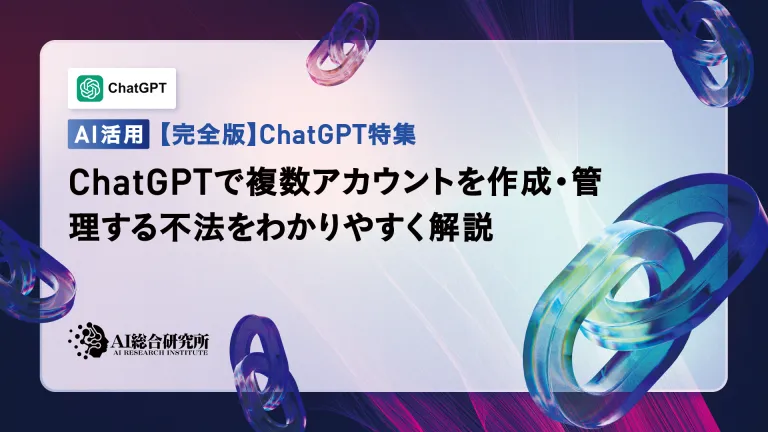
Efficient multiple account management techniques using ChatGPT | A thorough explanation of how to use business and private life! ChatGPT is used in a variety of situations, but some people may be worried about managing multiple accounts. This article will explain in detail how to create multiple accounts for ChatGPT, what to do when using it, and how to operate it safely and efficiently. We also cover important points such as the difference in business and private use, and complying with OpenAI's terms of use, and provide a guide to help you safely utilize multiple accounts. OpenAI


Hot AI Tools

Undresser.AI Undress
AI-powered app for creating realistic nude photos

AI Clothes Remover
Online AI tool for removing clothes from photos.

Undress AI Tool
Undress images for free

Clothoff.io
AI clothes remover

Video Face Swap
Swap faces in any video effortlessly with our completely free AI face swap tool!

Hot Article

Hot Tools

SublimeText3 Linux new version
SublimeText3 Linux latest version

MantisBT
Mantis is an easy-to-deploy web-based defect tracking tool designed to aid in product defect tracking. It requires PHP, MySQL and a web server. Check out our demo and hosting services.

Zend Studio 13.0.1
Powerful PHP integrated development environment

SAP NetWeaver Server Adapter for Eclipse
Integrate Eclipse with SAP NetWeaver application server.
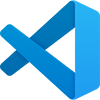
VSCode Windows 64-bit Download
A free and powerful IDE editor launched by Microsoft
