


With the emergence of large text-image models, generating an attractive image has become very simple. All the user needs to do is to enter a simple prompt with the movement of their fingers. After obtaining the image through a series of operations, we will inevitably have several questions: Can the image generated based on prompt meet our requirements? What kind of architecture should we build to handle the various requirements raised by users? Can large models still maintain the advantages and capabilities gained from billions of images in specific tasks?
In order to answer these questions, researchers from Stanford conducted a large number of investigations on various image processing applications and came to the following three findings:
First of all, the available data in a specific field is actually less than the data for training general models. This is mainly reflected in the fact that for example, the largest data set on a specific problem (such as gesture understanding, etc.) is usually less than 100k, which is smaller than large-scale, The multimodal text image dataset LAION 5B is 5 × 10^4 orders of magnitude smaller. This requires the neural network to be robust to avoid model overfitting and to have good generalization when targeting specific problems.
Secondly, when using data-driven processing of image tasks, large computing clusters are not always available. This is where fast training methods become important, methods that can optimize large models for specific tasks within acceptable time and memory space. Furthermore, fine-tuning, transfer learning and other operations may be required in subsequent processing.
Finally, various problems encountered in the image processing process will have different forms of definition. When solving these problems, although the image diffusion algorithm can be adjusted in a "procedural" way, for example, constraining the denoising process, editing multi-head attention activation, etc., these hand-crafted rules are basically dictated by human instructions ,Considering some specific tasks such as depth-image, pose-person, etc., these problems essentially require the interpretation of raw inputs into object-level or scene-level understanding, which makes hand-crafted procedural approaches less feasible. Therefore, to provide solutions in multiple tasks, end-to-end learning is essential.
Based on the above findings, this paper proposes an end-to-end neural network architecture ControlNet, which can control the diffusion model (such as Stable Diffusion) by adding additional conditions, thereby improving the graph. Picture effect, and can generate full-color pictures from line drawings, generate pictures with the same depth structure, and optimize the generation of hands through key points of the hands.
Paper address: https://arxiv.org/pdf/2302.05543.pdf
Project Address: https://github.com/lllyasviel/ControlNet
Effect display
So what is the effect of ControlNet?
Canny Edge Detection: By extracting line drawing from the original image, an image of the same composition can be generated.
Depth detection: By extracting the depth information in the original image, a map with the same depth structure can be generated.
ControlNet with semantic segmentation:
Using learning-based The deep Hough transform detects straight lines from Places2 and then uses BLIP to generate subtitles.
HED edge detection illustration.
Illustration of human posture recognition.
Method Introduction
ControlNet is a neural network architecture that enhances pre-trained image diffusion models with task-specific conditions. Let's first look at the basic structure of ControlNet.
ControlNet manipulates the input conditions of neural network blocks, thereby further controlling the overall behavior of the entire neural network. Here "network block" refers to a group of neural layers that are put together as a common unit for building neural networks, such as resnet block, multi-head attention block, and Transformer block.
Taking 2D features as an example, given a feature map x ϵ R^h×w×c, where {h, w, c} are the height, width and number of channels respectively. A neural network block F (・; Θ) with a set of parameters Θ transforms x into another feature map y as shown in equation (1) below.
This process is shown in Figure 2-(a) below.
Neural network blocks are connected by a unique convolution layer called "zero convolution", which is the weight 1×1 convolutional layer with zero initialization and bias. The researcher represents the zero convolution operation as Z (・;・) and uses two parameter instances {Θ_z1, Θ_z2} to form the ControlNet structure, as shown in the following formula (2).
where y_c becomes the output of the neural network block, as shown in Figure 2-(b) below.
ControlNet in image diffusion model
##The researcher took Stable Diffusion as an example to introduce how to use ControlNet control Large-scale diffusion models with task-specific conditions. Stable Diffusion is a large-scale text-to-image diffusion model trained on billions of images, essentially a U-net consisting of an encoder, intermediate blocks, and a residual-connected decoder.
As shown in Figure 3 below, the researcher uses ControlNet to control each layer of U-net. Note that the way ControlNet is connected here is computationally efficient: since the original weights are locked, the gradient calculation on the original encoder does not require training. And because half of the gradient calculations on the original model are reduced, training can be accelerated and GPU memory can be saved. Training a Stable Diffusion model using ControlNet only requires approximately 23% more GPU memory and 34% more time per training iteration (tested on a single Nvidia A100 PCIE 40G).
#Specifically, the researchers used ControlNet to create 12 encoding blocks and 1 Stable Diffusion intermediate block that are trainable copy. The 12 coding blocks come in 4 resolutions, 64×64, 32×32, 16×16, and 8×8, with 3 blocks in each resolution. The output is added to the U-net with 12 residual connections and 1 intermediate block. Since Stable Diffusion is a typical U-net structure, it is likely that this ControlNet architecture can be used in other diffusion models.
Training and Boosting Training
Given an image z_0, the diffusion algorithm progressively adds noise to the image and generates noise Image z_t, t is the number of times noise is added. When t is large enough, the image approximates pure noise. Given a set of conditions including time step t, text prompts c_t, and task-specific conditions c_f, the image diffusion algorithm learns a network ϵ_θ to predict the noise added to a noisy image z_t, as shown in Equation (10) below.
During the training process, the researchers randomly replaced 50% of the text prompts c_t with empty strings, which is beneficial to ControlNet's ability to identify semantic content from the input condition map.
In addition, the researchers also discussed several strategies to improve the training of ControlNets, especially when computing devices are very limited (such as laptops) or very powerful (such as with large-scale GPUs available). computing cluster).
Please refer to the original paper for more technical details.
The above is the detailed content of AI dimensionality reduction attacks human painters, Vincentian graphs are introduced into ControlNet, and depth and edge information are fully reusable. For more information, please follow other related articles on the PHP Chinese website!
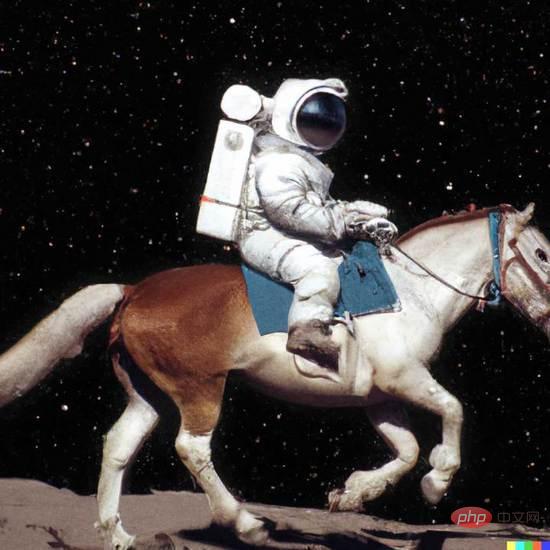
1 前言在发布DALL·E的15个月后,OpenAI在今年春天带了续作DALL·E 2,以其更加惊艳的效果和丰富的可玩性迅速占领了各大AI社区的头条。近年来,随着生成对抗网络(GAN)、变分自编码器(VAE)、扩散模型(Diffusion models)的出现,深度学习已向世人展现其强大的图像生成能力;加上GPT-3、BERT等NLP模型的成功,人类正逐步打破文本和图像的信息界限。在DALL·E 2中,只需输入简单的文本(prompt),它就可以生成多张1024*1024的高清图像。这些图像甚至
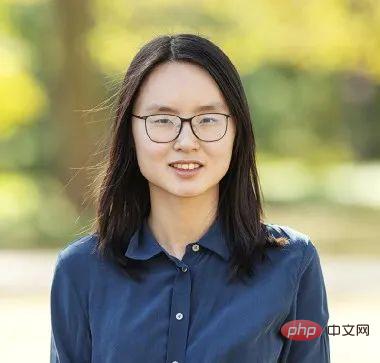
“Making large models smaller”这是很多语言模型研究人员的学术追求,针对大模型昂贵的环境和训练成本,陈丹琦在智源大会青源学术年会上做了题为“Making large models smaller”的特邀报告。报告中重点提及了基于记忆增强的TRIME算法和基于粗细粒度联合剪枝和逐层蒸馏的CofiPruning算法。前者能够在不改变模型结构的基础上兼顾语言模型困惑度和检索速度方面的优势;而后者可以在保证下游任务准确度的同时实现更快的处理速度,具有更小的模型结构。陈丹琦 普
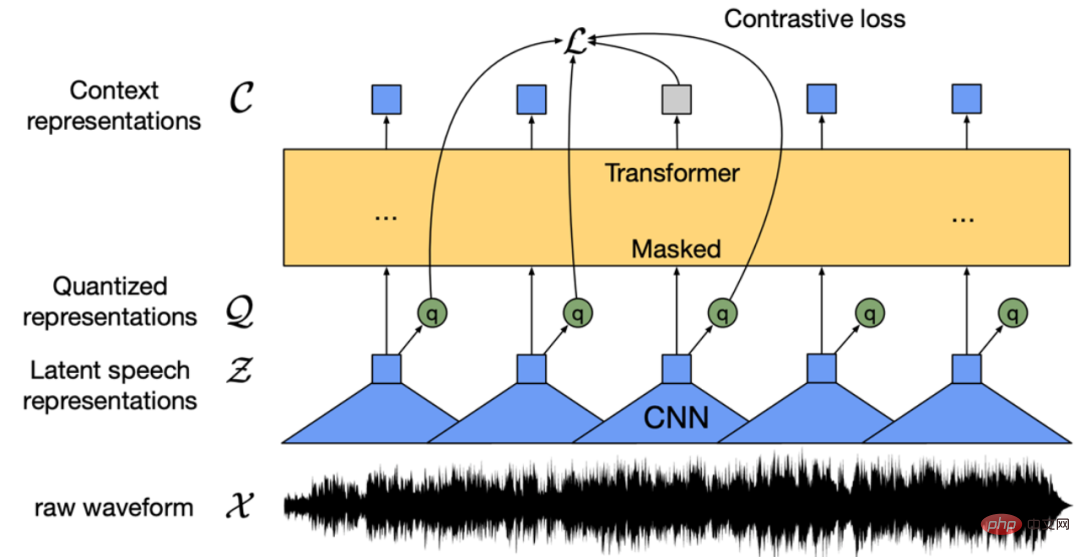
Wav2vec 2.0 [1],HuBERT [2] 和 WavLM [3] 等语音预训练模型,通过在多达上万小时的无标注语音数据(如 Libri-light )上的自监督学习,显著提升了自动语音识别(Automatic Speech Recognition, ASR),语音合成(Text-to-speech, TTS)和语音转换(Voice Conversation,VC)等语音下游任务的性能。然而这些模型都没有公开的中文版本,不便于应用在中文语音研究场景。 WenetSpeech [4] 是
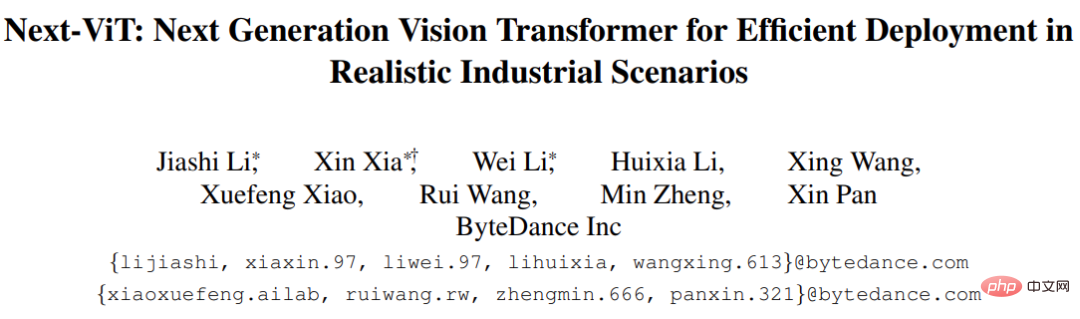
由于复杂的注意力机制和模型设计,大多数现有的视觉 Transformer(ViT)在现实的工业部署场景中不能像卷积神经网络(CNN)那样高效地执行。这就带来了一个问题:视觉神经网络能否像 CNN 一样快速推断并像 ViT 一样强大?近期一些工作试图设计 CNN-Transformer 混合架构来解决这个问题,但这些工作的整体性能远不能令人满意。基于此,来自字节跳动的研究者提出了一种能在现实工业场景中有效部署的下一代视觉 Transformer——Next-ViT。从延迟 / 准确性权衡的角度看,
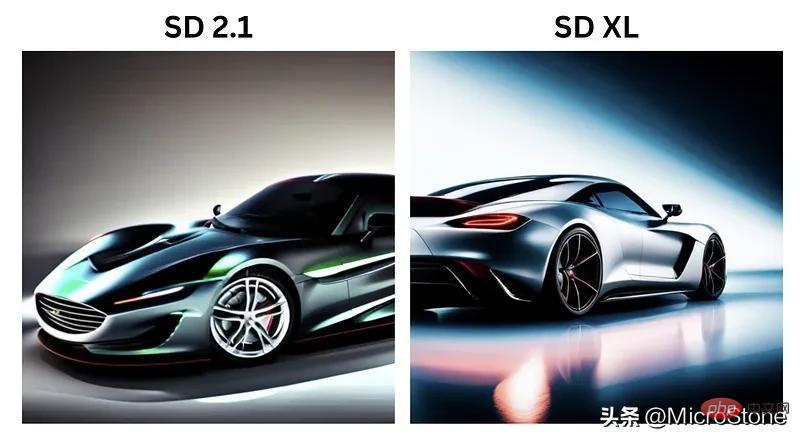
3月27号,Stability AI的创始人兼首席执行官Emad Mostaque在一条推文中宣布,Stable Diffusion XL 现已可用于公开测试。以下是一些事项:“XL”不是这个新的AI模型的官方名称。一旦发布稳定性AI公司的官方公告,名称将会更改。与先前版本相比,图像质量有所提高与先前版本相比,图像生成速度大大加快。示例图像让我们看看新旧AI模型在结果上的差异。Prompt: Luxury sports car with aerodynamic curves, shot in a
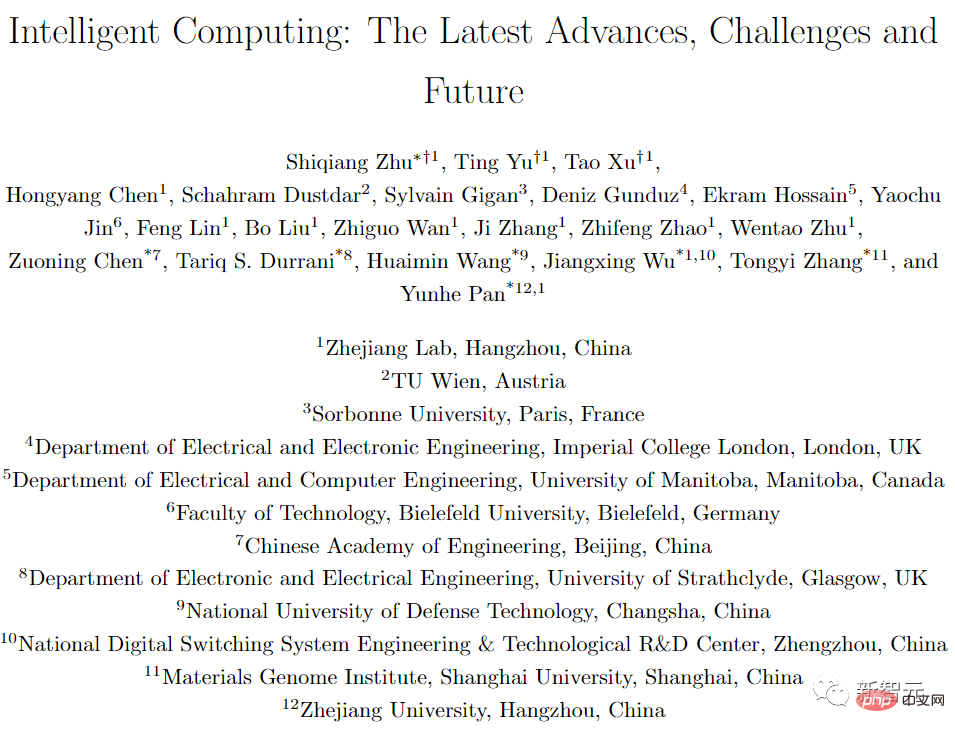
人工智能就是一个「拼财力」的行业,如果没有高性能计算设备,别说开发基础模型,就连微调模型都做不到。但如果只靠拼硬件,单靠当前计算性能的发展速度,迟早有一天无法满足日益膨胀的需求,所以还需要配套的软件来协调统筹计算能力,这时候就需要用到「智能计算」技术。最近,来自之江实验室、中国工程院、国防科技大学、浙江大学等多达十二个国内外研究机构共同发表了一篇论文,首次对智能计算领域进行了全面的调研,涵盖了理论基础、智能与计算的技术融合、重要应用、挑战和未来前景。论文链接:https://spj.scien
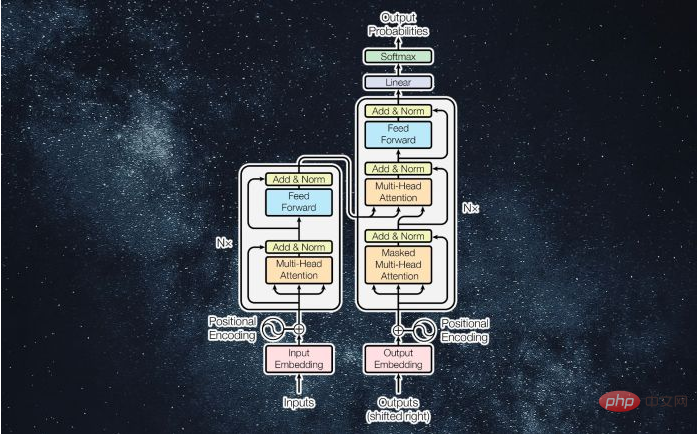
译者 | 李睿审校 | 孙淑娟近年来, Transformer 机器学习模型已经成为深度学习和深度神经网络技术进步的主要亮点之一。它主要用于自然语言处理中的高级应用。谷歌正在使用它来增强其搜索引擎结果。OpenAI 使用 Transformer 创建了著名的 GPT-2和 GPT-3模型。自从2017年首次亮相以来,Transformer 架构不断发展并扩展到多种不同的变体,从语言任务扩展到其他领域。它们已被用于时间序列预测。它们是 DeepMind 的蛋白质结构预测模型 AlphaFold
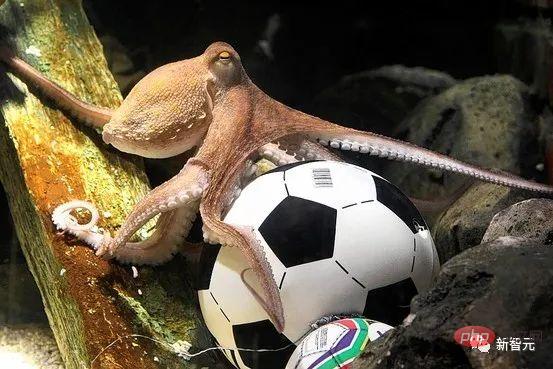
说起2010年南非世界杯的最大网红,一定非「章鱼保罗」莫属!这只位于德国海洋生物中心的神奇章鱼,不仅成功预测了德国队全部七场比赛的结果,还顺利地选出了最终的总冠军西班牙队。不幸的是,保罗已经永远地离开了我们,但它的「遗产」却在人们预测足球比赛结果的尝试中持续存在。在艾伦图灵研究所(The Alan Turing Institute),随着2022年卡塔尔世界杯的持续进行,三位研究员Nick Barlow、Jack Roberts和Ryan Chan决定用一种AI算法预测今年的冠军归属。预测模型图


Hot AI Tools

Undresser.AI Undress
AI-powered app for creating realistic nude photos

AI Clothes Remover
Online AI tool for removing clothes from photos.

Undress AI Tool
Undress images for free

Clothoff.io
AI clothes remover

AI Hentai Generator
Generate AI Hentai for free.

Hot Article

Hot Tools

PhpStorm Mac version
The latest (2018.2.1) professional PHP integrated development tool

Safe Exam Browser
Safe Exam Browser is a secure browser environment for taking online exams securely. This software turns any computer into a secure workstation. It controls access to any utility and prevents students from using unauthorized resources.

SublimeText3 English version
Recommended: Win version, supports code prompts!
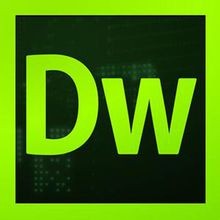
Dreamweaver CS6
Visual web development tools
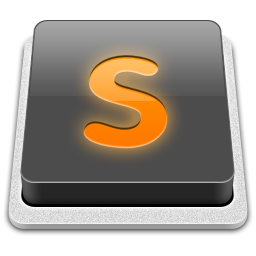
SublimeText3 Mac version
God-level code editing software (SublimeText3)
