


This article will give you an easy-to-understand understanding of autonomous driving
The principles of autonomous driving can often be best understood from the architecture of autonomous driving. The public’s most understandable understanding of autonomous driving is perception, decision-making, and execution. All robots have this structure.
- Perception answers questions about what is around, similar to human eyes and ears. Obtain information about surrounding obstacles and roads through cameras, radar, maps and other means.
- Decision-making answers the question of what I want to do, similar to the brain. By analyzing the sensed information, the path and vehicle speed are generated.
- Execution is similar to hands and feet, converting decision-making information into brake, accelerator and steering signals to control the vehicle to drive as expected.
Next we go deeper and the problem becomes a little more complicated.
In our daily lives, we may intuitively think that I decide my next decision based on the information I see with my eyes at every moment. , but this is often not the case. There is always a time delay from the eyes to the head to the hands and feet, and the same is true for autonomous driving. But we don’t feel the impact because our brains automatically handle prediction. Even if it is only a few milliseconds, our decisions are based on predictions of what we see to guide the operation of our hands and feet. This is the basis for us to maintain normal functions. Therefore, we will add a prediction module before autonomous driving decisions.
The perception process also contains the universe, and upon careful consideration, it can be divided into two stages: "sensing" and "perception". "Sensing" obtains raw data from sensors such as pictures, while "perception" processes useful information from pictures (such as how many people are in the picture). As the old saying goes, "Seeing is believing, hearing is believing." The useful information of "perception" can be further divided into self-vehicle perception and external perception. People or self-driving cars often have different strategies when processing these two types of information.
- Self-vehicle perception - information obtained by receptors at all times (including cameras, radar, GPS, etc.)
- External perception - information collected and processed by external agents or past memories (including positioning, maps, vehicle-linked information, etc.), which requires the input of self-vehicle positioning perception (GPS).
In addition, the information about obstacles, lanes and other information processed by various sensors through algorithms is often contradictory. If the radar sees an obstacle ahead but the camera tells you there isn't, then you need to add a "fusion" module. Make further connections and judgments about inconsistent information.
Here we often summarize “fusion and prediction” as “world model”. This word is very vivid, whether you are a materialist or an idealist. It is impossible to cram all the "world" into your brain, but what guides our work and life is the "model" of the "world", that is, by processing what we see after birth, and gradually constructing a model in our mind. The Taoist understanding of the world is called "inner view". The core responsibility of the world model is to understand the attributes and relationships of the current environmental elements through "fusion", and to make "predictions" in conjunction with "a priori laws" to provide a more leisurely judgment for decision execution. This time span can range from a few milliseconds to a few seconds. Hour.
Due to the addition of the world model, the entire architecture has become richer, but there is another detail that is often overlooked. That is the flow of information. A simple understanding is that people perceive things through their eyes, process them in the brain, and then hand them over to their hands and feet for execution. However, the actual situation is often more complicated. There are two typical behaviors here that constitute a completely opposite information flow, that is, "plan for goal achievement" and "shift of attention."
How do you understand "plan to achieve goals"? In fact, the beginning of thinking is not perception but "goal". Only when you have a goal can you trigger a meaningful "perception-decision-execution" process. For example, if you want to drive to a destination, you may know several routes, and you will finally choose one of the routes based on the congestion situation. Congestion belongs to the world model, while "getting there" belongs to the decision. This is the process by which decisions are passed to the world model.
How to understand "shift of attention"? Even if it is a picture, neither humans nor machines can obtain all the information hidden inside. Starting from a need and context, we tend to focus on a limited scope and limited categories. This information cannot be obtained from the picture itself, but comes from the "world model" and "goal". It is a process from decision-making to world model and then to perception.
We add some necessary information and rearrange the entire structure. It looks like the following. Is it a little more complicated? It’s not over yet, let’s continue watching.
Autopilot algorithms, like the brain, have a requirement for processing time. The general cycle is between 10ms-100ms, which can meet the response requirements to environmental changes. But the environment is sometimes simple and sometimes very complex. Many algorithm modules cannot meet this time requirement. For example, thinking about the meaning of life may not be something that can be done in 100ms. If you have to think about life every step of the way, it must be a kind of destruction to the brain. The same is true for computers. There are physical limits to computing power and speed. The solution is to introduce a layered framework.
This layered mechanism will generally shorten the processing cycle by 3-10 times as it goes up. Of course, it does not necessarily need to appear completely in the actual framework. In engineering, it is based on on-board resources and algorithms. Usage can be flexibly adjusted. Basically, perception is an upward process that continuously refines specific elements based on attention, providing perceptual information with "depth and direction." Decision-making is a downward process, decomposing actions from the goal to each execution unit layer by layer according to different levels of world models. World models generally have no specific flow direction and are used to construct environmental information at different granular scales.
According to the complexity of the processing tasks, the division of labor and the communication environment will also be appropriately castrated and merged. For example, low-level ADAS functions (ACC) require less computing power and can be designed with only one layer. High-end ADAS functions (AutoPilot) generally have two levels of configuration. As for the autonomous driving function, there are many complex algorithms, and a three-layer design is sometimes necessary. In software architecture design, there are also situations where the world model and the perception or decision-making module at the same layer are merged.
Various autonomous driving companies or industry standards will release their own software architecture designs, but they are often the result of castration based on the status quo and are not It has universal applicability, but in order to facilitate everyone's understanding, I still substitute the current mainstream functional modules. Let's take a look at the comparison relationship, which will be more helpful for understanding the principle.
It needs to be noted in advance that although this already means a bit of software architecture, it is still a description of the principle and the actual software architecture. The design is even more complicated than this. Instead of going into all the details here, we focus on the parts that are easily confused. Let’s focus on it below.
Environment Awareness-ALL IN Deep Learning
In order to ensure that unmanned vehicles understand and grasp the environment, the environment perception part of the unmanned driving system usually needs to obtain a large amount of information about the surrounding environment, including the location of obstacles, speed, the precise shape of the lane ahead, and signs. Card position type, etc. This information is usually obtained by fusing data from multiple sensors such as lidar, peripheral/surround camera, and millimeter wave radar.
The development of deep learning has made it an industry-wide consensus to complete the construction of autonomous driving through neural network algorithms. The algorithm of the perception module is the "pawn" of the entire deep learning process and is the first software module to complete the transformation.
The correlation and difference between positioning map and V2X-self-vehicle perception and external perception
In the traditional sense Understand that external perception is based on GPS positioning signals and converts information in absolute coordinate systems such as high-precision maps and vehicle-to-everything messages (V2X) into the own vehicle coordinate system for use by vehicles. It is similar to the Amap navigator used by people. Combined with the "self-vehicle perception" information originally in the self-vehicle coordinate system, it provides environmental information for autonomous driving.
But the actual design is often more complex. Due to the unreliability of GPS, the IMU needs continuous correction. Mass-produced autonomous driving positioning often uses perceptual map matching to accurately obtain accurate absolute positioning. Position, use the sensing results to correct the IMU to obtain accurate relative position, and form redundancy with the INS system composed of GPS-IMU. Therefore, the positioning signals necessary for "external perception" often rely on "self-vehicle perception" information.
In addition, although the map is strictly part of the "world model", it is limited by the sensitivity of GPS. During the domestic software implementation process, the positioning module and The map module integrates and offsets all GPS data to ensure that no sensitive positioning information is leaked.
Fusion prediction module - the core focus is on the difference between the two
The core of fusion is to solve two problems, one It is a spatio-temporal synchronization problem. Using the coordinate system conversion algorithm and the time synchronization algorithm of software and hardware collaboration, first align the sensing measurement results of lidar, camera and millimeter wave radar to a spatio-temporal point to ensure the unity of the original data of the entire environment perception. The other is to solve the problem of association and anomaly elimination, handle the association of different sensors mapping to the same "world model" element (a person/a lane, etc.), and eliminate anomalies that may be caused by misdetection by a single sensor. However, the fundamental difference between fusion and prediction is that it only processes information from the past and the current moment, and does not process external moments.
The prediction will make a judgment on the future time based on the fusion result. This future time ranges from 10ms to 5 minutes. This includes predicting traffic lights, predicting the driving path of surrounding obstacles, or predicting the cornering position in the distance. Forecasts of different periods will provide planning for corresponding periods and predictions of different granularities, thereby providing greater space for planning adjustments.
Planning Control - Hierarchical Strategy Decomposition
##Planning is when the unmanned vehicle makes some effective decisions to achieve a certain goal. The process of purposeful decision-making. For driverless vehicles, this goal usually refers to reaching the destination from the starting point while avoiding obstacles, and continuously optimizing the driving trajectory and behavior to ensure the safety and comfort of passengers. The structural summary of the planning is to integrate information based on the environment of different granularities, conduct layer-by-layer evaluation and decomposition starting from the external goals, and finally pass it to the executor to form a complete decision-making.
Broken down, the planning module is generally divided into three layers: Mission Planning, Behavioral Planning and Motion Planning. The core is to obtain the global path based on the road network and discrete path search algorithm, which is given a large-scale task type, often with a long cycle. Behavior planning is based on the finite state machine to determine the specific behavior that the vehicle should take in a medium cycle (left change Road, avoidance, E-STOP) and set some boundary parameters and approximate path range. The motion planning layer is often based on sampling or optimization methods to ultimately obtain the only path that meets comfort and safety requirements. Finally, it is handed over to the control module to follow the unique path through feedforward prediction and feedback control algorithms, and manipulate the brake, steering, throttle, body and other actuators to finally execute the command.
I don’t know how far you have understood it, but the above is just an introduction to the principles of autonomous driving. The current theories, algorithms and architecture of autonomous driving are developing very fast, although the above content is relatively basic. The knowledge points will not be outdated for a long time. But the new demands have brought a lot of new understanding to the architecture and principles of autonomous driving.
The above is the detailed content of This article will give you an easy-to-understand understanding of autonomous driving. For more information, please follow other related articles on the PHP Chinese website!
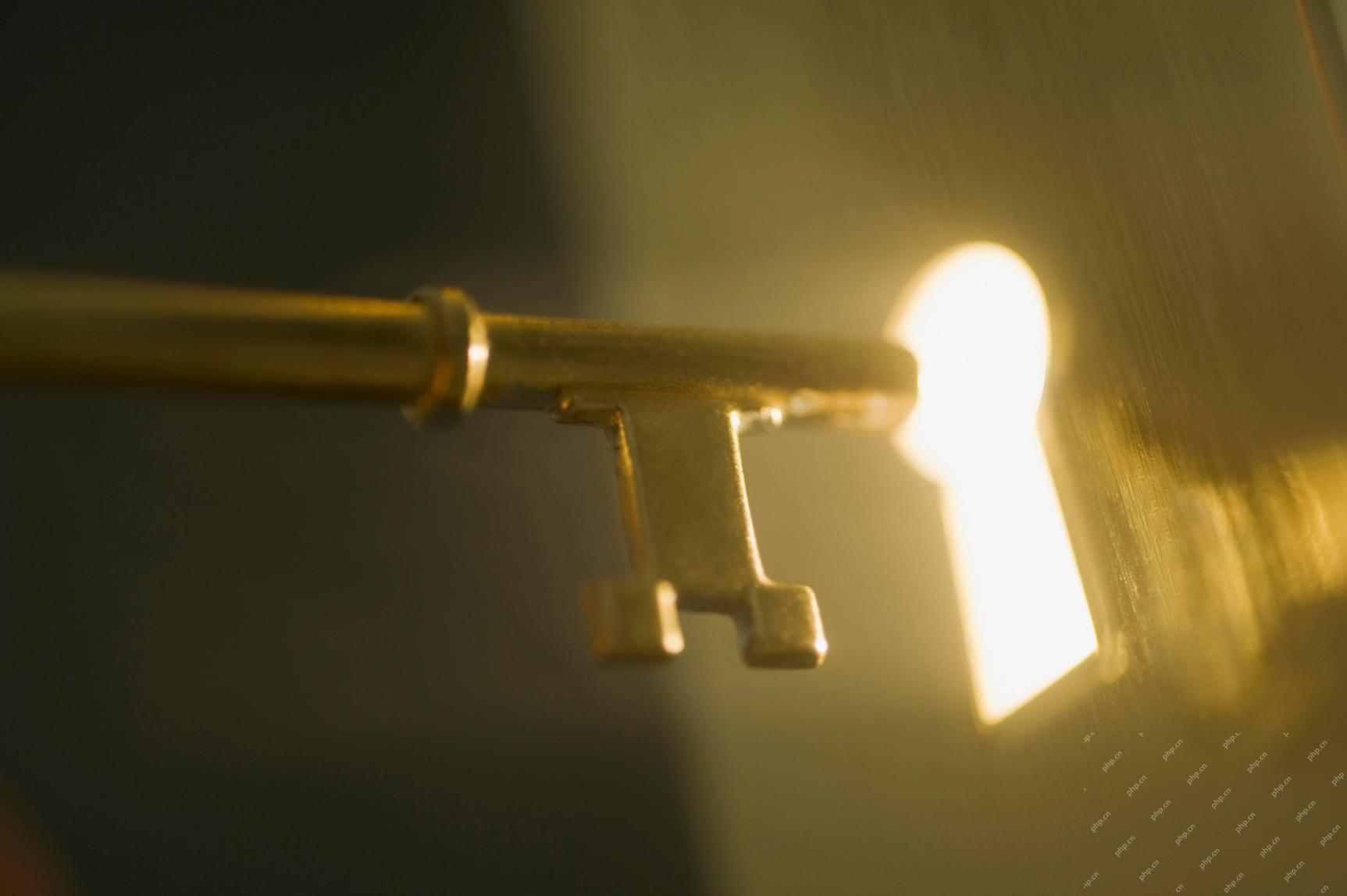
Cyberattacks are evolving. Gone are the days of generic phishing emails. The future of cybercrime is hyper-personalized, leveraging readily available online data and AI to craft highly targeted attacks. Imagine a scammer who knows your job, your f
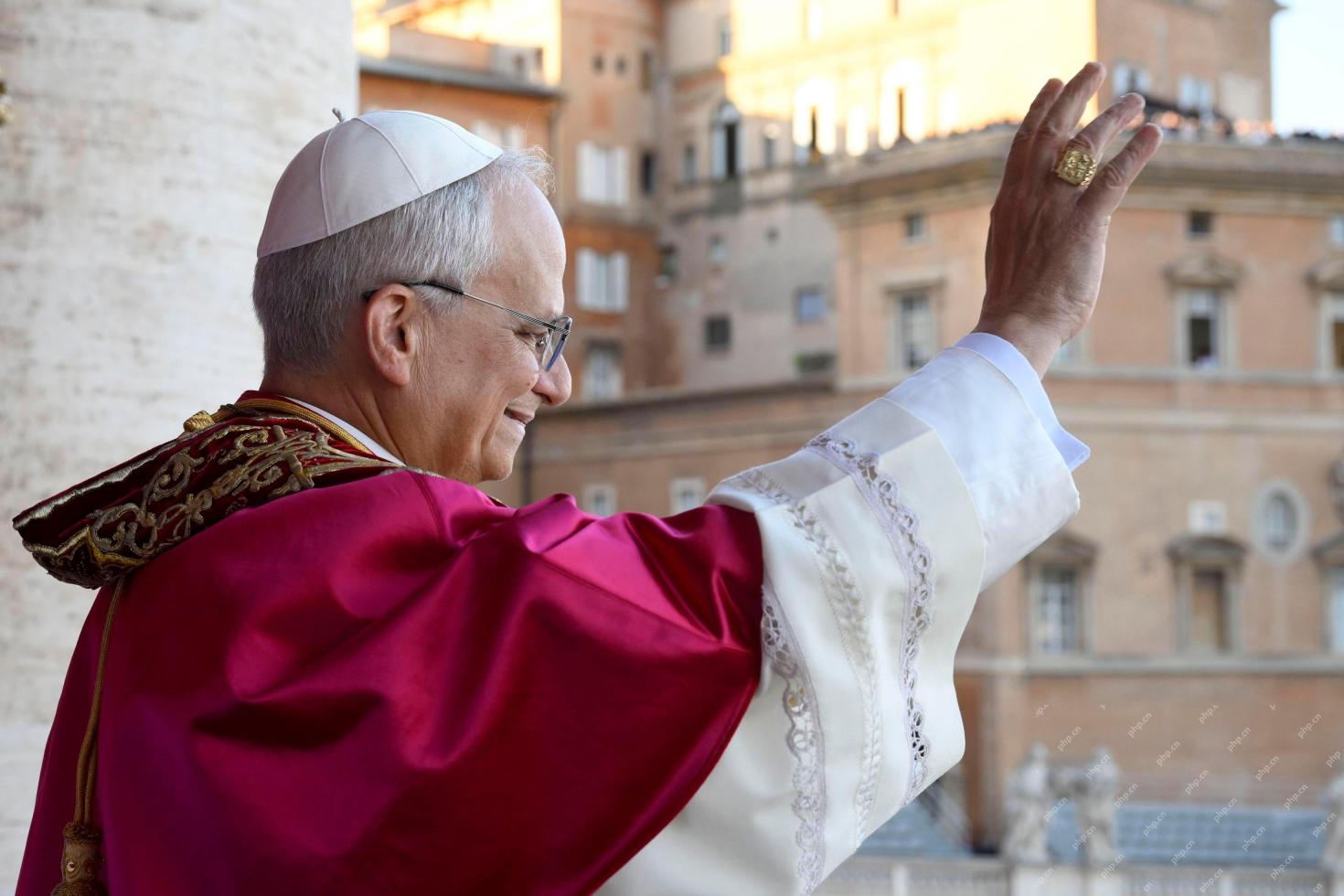
In his inaugural address to the College of Cardinals, Chicago-born Robert Francis Prevost, the newly elected Pope Leo XIV, discussed the influence of his namesake, Pope Leo XIII, whose papacy (1878-1903) coincided with the dawn of the automobile and
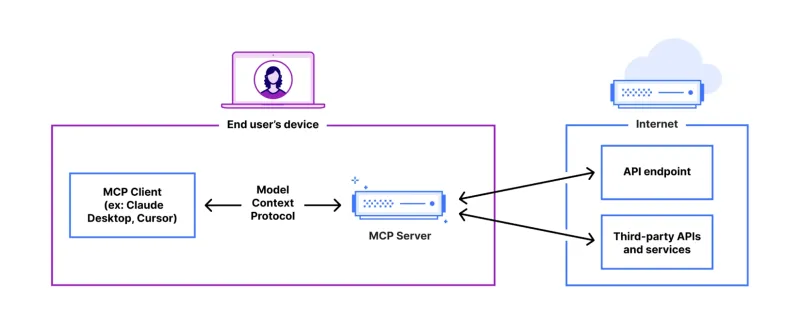
This tutorial demonstrates how to integrate your Large Language Model (LLM) with external tools using the Model Context Protocol (MCP) and FastAPI. We'll build a simple web application using FastAPI and convert it into an MCP server, enabling your L
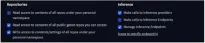
Explore Dia-1.6B: A groundbreaking text-to-speech model developed by two undergraduates with zero funding! This 1.6 billion parameter model generates remarkably realistic speech, including nonverbal cues like laughter and sneezes. This article guide
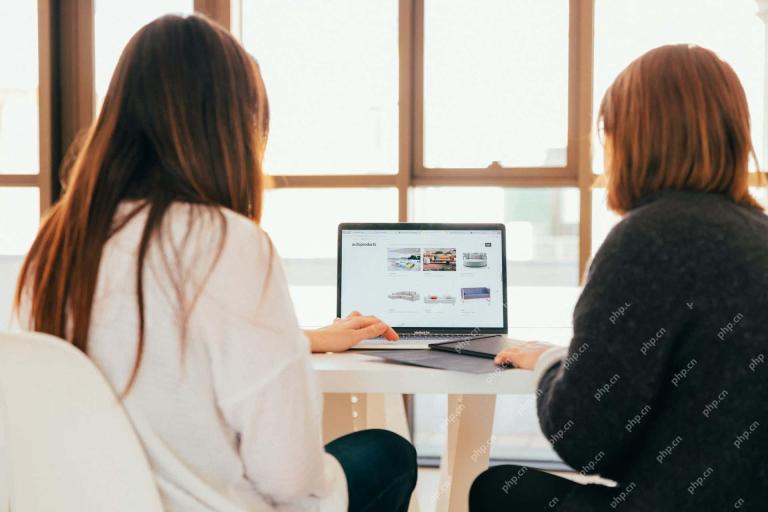
I wholeheartedly agree. My success is inextricably linked to the guidance of my mentors. Their insights, particularly regarding business management, formed the bedrock of my beliefs and practices. This experience underscores my commitment to mentor
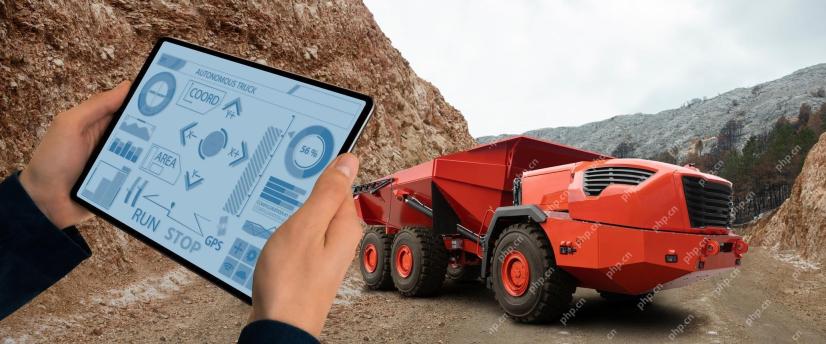
AI Enhanced Mining Equipment The mining operation environment is harsh and dangerous. Artificial intelligence systems help improve overall efficiency and security by removing humans from the most dangerous environments and enhancing human capabilities. Artificial intelligence is increasingly used to power autonomous trucks, drills and loaders used in mining operations. These AI-powered vehicles can operate accurately in hazardous environments, thereby increasing safety and productivity. Some companies have developed autonomous mining vehicles for large-scale mining operations. Equipment operating in challenging environments requires ongoing maintenance. However, maintenance can keep critical devices offline and consume resources. More precise maintenance means increased uptime for expensive and necessary equipment and significant cost savings. AI-driven
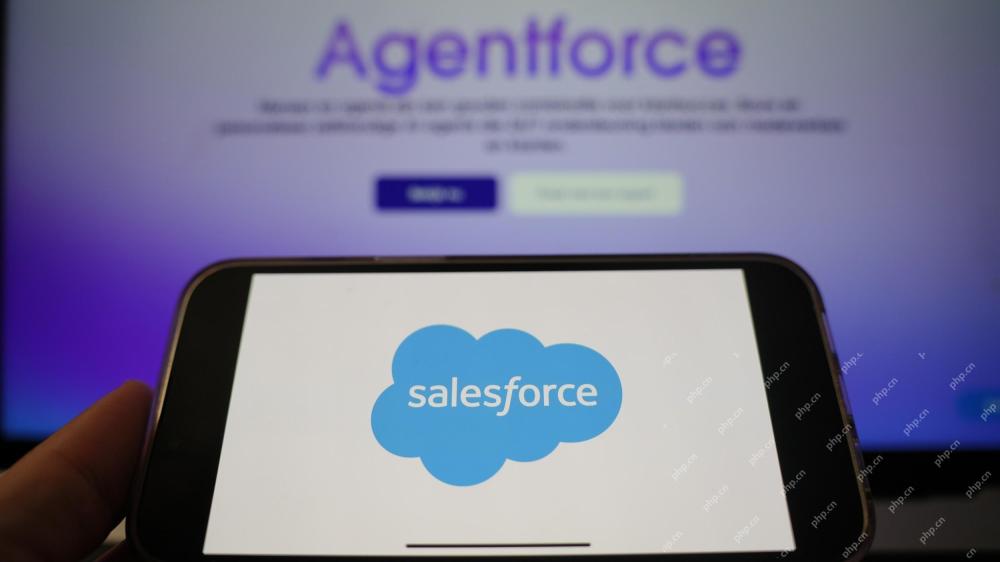
Marc Benioff, Salesforce CEO, predicts a monumental workplace revolution driven by AI agents, a transformation already underway within Salesforce and its client base. He envisions a shift from traditional markets to a vastly larger market focused on
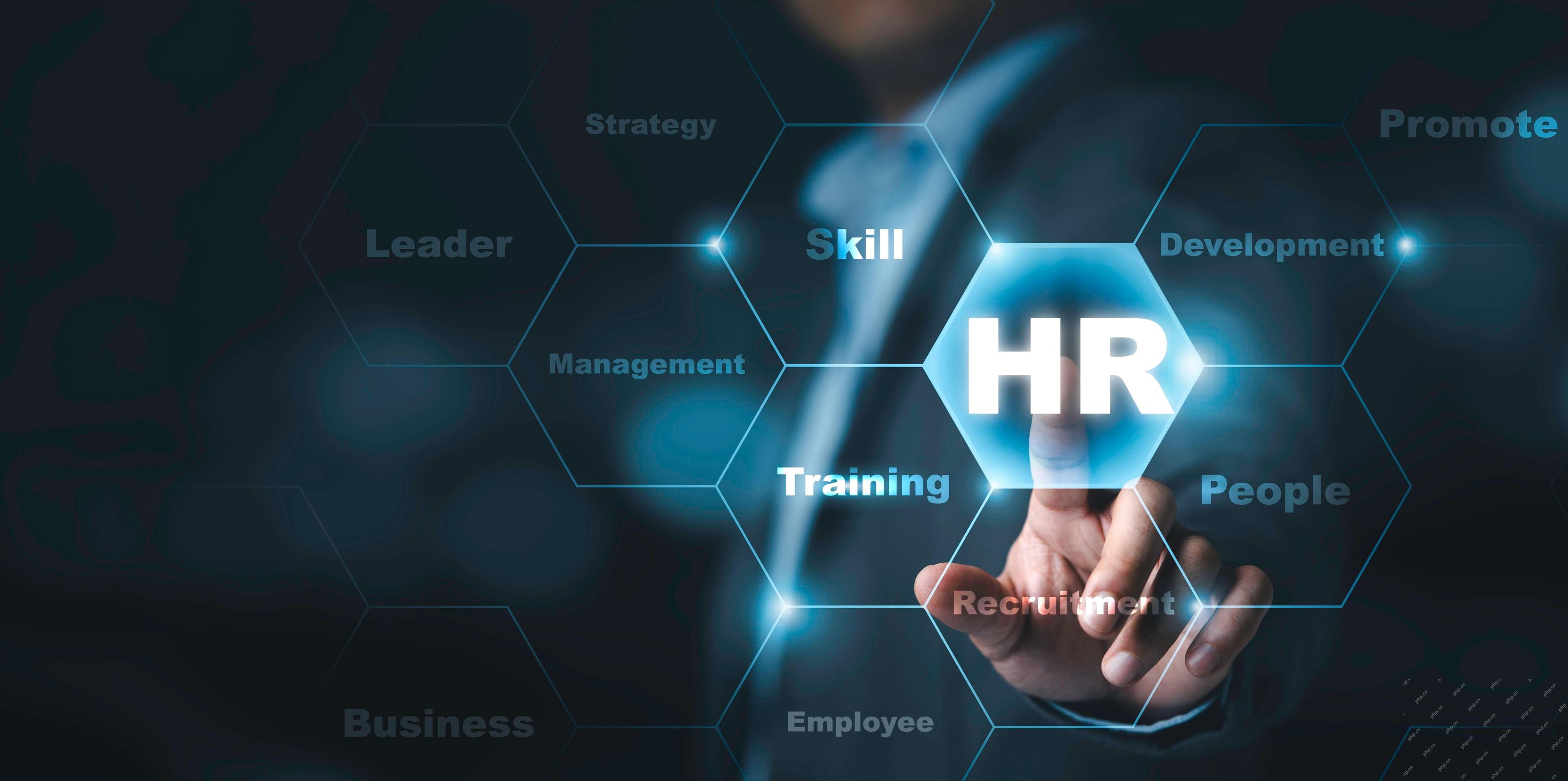
The Rise of AI in HR: Navigating a Workforce with Robot Colleagues The integration of AI into human resources (HR) is no longer a futuristic concept; it's rapidly becoming the new reality. This shift impacts both HR professionals and employees, dem


Hot AI Tools

Undresser.AI Undress
AI-powered app for creating realistic nude photos

AI Clothes Remover
Online AI tool for removing clothes from photos.

Undress AI Tool
Undress images for free

Clothoff.io
AI clothes remover

Video Face Swap
Swap faces in any video effortlessly with our completely free AI face swap tool!

Hot Article

Hot Tools

Notepad++7.3.1
Easy-to-use and free code editor

SublimeText3 Chinese version
Chinese version, very easy to use

Zend Studio 13.0.1
Powerful PHP integrated development environment

SublimeText3 Linux new version
SublimeText3 Linux latest version
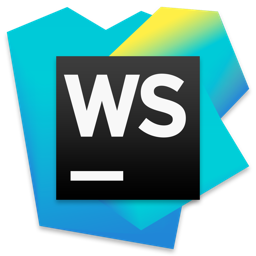
WebStorm Mac version
Useful JavaScript development tools
