


Guests|Tan Zhongyi, Zhu Shihu
Compiled|Xu Jiecheng
##2022 is destined to be a year worth remembering for artificial intelligence. In this year In the middle of the year, artificial intelligence has achieved great development and great progress at both the technical and industrial levels, and a large number of innovative technologies have moved from concept to practice. At the beginning of the year, the Winter Olympics powered by AI brought us a brand new viewing experience; in the middle of the year, AI painting moved from illusion to reality and won multiple competition awards; at the end of the year, the emergence of ChatGPT will once again change the entire year. The carnival reaches its climax... Whether it is the breakthroughs in underlying technology or the successive blooming of various applications, in the past year, artificial intelligence has moved towards We showed what's possible. In order to gain a more comprehensive understanding of the current development status and future trends in the field of artificial intelligence, the 51CTO Content Center specially invited two senior experts in the industry, Tan Zhongyi, architect of the Fourth Paradigm, and Zhu Shihu, deputy general manager of the Information Technology Department of Everbright Trust, to discuss the relevant topics of artificial intelligence. Five topics on intelligent technology and industry, I hope to get more answers from them. 1. Keywords in the field of artificial intelligence in 2022 Tan Zhongyi: At the technical level, the hottest keyword in 2022 is undoubtedly "AIGC", and at the industrial level, I I think it should be "AI engineering" or MLOps. Because nowadays, whether domestic or foreign, technology media, products, and venture capital fields are paying very high attention to AI engineering. The core concept of AI engineering and MLOps is to enable machine learning to be implemented on a large scale within the enterprise more quickly. This has also been the focus of artificial intelligence-related industries in the past year. Zhu Shihu: From a policy perspective, the government will lead the national strategy of data elements in 2022, proposing that data elements are a key force in reorganizing global resources, reshaping the global economic structure, and changing the competitive landscape. At present, China's underlying IT standards, architecture, products, and ecology are mostly determined by foreign technology manufacturers. Therefore, there are many underlying technology, information security and other issues. Therefore, as the global IT ecosystem develops from the single level in the past to the multi-level direction in the future, we need to build our own IT underlying framework. Therefore, in the context of Xinchuang, I believe that the key word for artificial intelligence and even the entire technology industry in 2022 should be "technology has national borders." 2. Current status of technology development and application in the field of cutting-edge artificial intelligenceTan Zhongyi: At the application level, in the field of AI engineering, the fourth paradigm has brought a large number of applications in the past year. The self-developed technology accumulation has been open sourced to the outside world, including OpenMLDB, a production-level feature platform with online and offline consistency, which can realize real-time prediction, risk control and scenario recommendation within rapid development enterprises, and solve time series features, efficient reading and online and online Lower consistency issues. Since open source has been open for more than a year, we have formed good cooperation with the upstream and downstream open source technology ecosystem and achieved good results. At the technical level, 4Paradigm has always insisted on in-depth cultivation of AI. At this stage, it mainly focuses on the implementation of two types of AI within the enterprise. One is the implementation of perceptual AI, such as CV and NLP. The other is the implementation of decision-making AI to formulate strategies for enterprises, such as risk control, recommendations, predictions and other scenarios closely related to enterprise operations. There are two important key points in this process. One is to quickly bring the model online with high quality; the other is to conduct continuous training, feedback and adjustment of the model to keep the model cutting-edge and efficient. sex. For enterprises with many AI scenarios, in this case, an enterprise can generate large-scale benefits, realize the transition from quantitative change to qualitative change, and enable the enterprise to change from Traditional data companies have evolved into AI companies to achieve the purpose of improving core competitiveness through AI. Zhu Shihu: The application of artificial intelligence technology in the financial industry has become relatively mature in recent years. The main thing we have done in the past year and the next few years is to use financial business to lead the development of financial technology. For example, in the field of consumer finance, when banks are developing access models for consumer finance, the first generation is a rule-driven model, the second generation is a rule-driven model, and the third generation is a big data-driven model. At this stage, a core problem that intelligent algorithms have to solve is insufficient samples. In the actual process of doing intelligent risk control, I summarized a set of manuals. For example, when the dimensions of the data are insufficient, the first way is to use the new feature combination method AutoCross to increase the dimension. The second way is to use transfer learning when there are insufficient samples. When the cost of labeling samples is high and many samples are not labeled, the Active learning method can be used. When the sample labeling error rate is relatively high, for example, if there is some external data and the data labeling error rate is relatively high, we need to use the Confident Learning algorithm. When there are very few sample labels, such as when used to prevent transaction fraud, you can choose to use a semi-supervised learning algorithm. This is also a relatively mature response plan summarized in the past year or two.3. The evolution direction of the market environment for the upstream and downstream artificial intelligence industries
Tan Zhongyi: Technology companies engaged in AI applications in the future may be divided into three types. The first is companies that have the resources to build large models, such as massive data and massive computing power; companies with top scientists in the industry can build large models with high accuracy and good universality, like AutoAI, they may Provide external services in the form of API.
The second category is enterprises with rich scenarios. For various scenarios, for example, AIGC can be used in various places where content is needed to make various applications.
The third category is between the two, providing more tools to help the two make better connections, such as ensuring data accuracy, ensuring line Enterprises such as stability and monitoring of online services, model versions and Beta.
From an industry perspective, the current implementation of AI in China is mainly concentrated in industries related to the intelligent transformation of enterprises. The most popular industry at present is the financial industry, which has scenarios and money. It is an industry that almost every AI technology company must compete for.
The second promising option is new consumption and new retail. In the current state of new consumption and new retail, many companies and many industries are constantly launching new products. The explosion of new products is quite fast, and they are done through many new media and new channels. For marketing, logistics, There are many, many demands for AI in the supply chain.
The third type is some manufacturing industries. Currently, the focus is still on the traditional CV fields such as safety inspection, safe production, and fault detection. The CV field can also improve a certain degree of efficiency. , but it is not yet time to completely change the working model of the industry. It may take several years for complete disruption.
Zhu Shihu: From the career changes of an AI practitioner, we can easily understand the changing trends of the artificial intelligence industry. Taking a personal example as an example, I first worked in the artificial intelligence algorithm industry, then switched from algorithms to technology, then from technology to data, and now from data to business. There is actually a very profound logic in this. of.
When I first made the model, I found that if there was no technology to carry it, the model could only be embedded in the risk management of the enterprise. It could only be embedded in the management process, but not in the specific In business, the model must be embedded into the technology system before it can be transferred to specific business, so I switched from modeling to technology.
After I started working in technology, I discovered the second problem, which is that for models and algorithms, the contribution of data to the model is far greater than the contribution of the algorithm to the model. I realized that After realizing the importance of data, we turned to using technology to create data. After finishing the data, we turned to business. There are two logics in this. First, the model is based on data, the data is based on statistics, the statistics is based on caliber, and the caliber is determined by the business department. However, when business departments formulate business calibers, they often strategically blur the business calibers out of business needs. Secondly, the digital transformation of most industries is essentially led by business, and the same is true in terms of industrial development.
4. The evolution path and possible implementation scenarios of generative AI
Tan Zhongyi: Generative AI has recently received widespread attention from the industry. This is indeed a Good thing. In the past two years in the field of AI, new scenarios for large models have been relatively scarce. Nowadays, the reason why ChatGPT is refreshing to everyone is that it really makes users feel that it has solved many problems that could not be solved by multiple rounds of dialogue before, and it has also used large models to generate some more practical answers, giving everyone more information. confidence. The market always needs technological hot spots. The existence of technological hot spots can maintain the confidence of capitalists, and the confidence of capitalists can push them to spend money to implement these technologies faster.
The commercialization of generative AI may be divided into three types. The first one is that OpenAI, which has money, people, and algorithms, can generate enough revenue through API. For example, AutoCAD, a company whose Photoshop has now integrated some functions of AIGC, and several other AIGC companies have also generated tens of millions of revenue. This is something that only a few companies that make large models can do.
The second type is to combine AIGC's capabilities with business scenarios. Business integrators need to find many specific business scenarios, and then think about issues such as cost and efficiency, which requires a lot of design. , only if progress can be achieved in three aspects: rigid demand, profit, and promotion, can a good model be formed.
The third type of model was also mentioned by the CEO of OpenAI recently. Between the two, there is a third type of company that can do Finetune for ordinary and universal large models. As a technology provider, it can target a specific business scenario, and then finetune the large model for this specific business scenario, and can also achieve commercial implementation.
Going back to the fundamentals, we still need to solve business problems or solve practical problems of users, and this requires constant exploration and experimentation. I am very happy to see the popularity of AIGC, which gives everyone more confidence in the cause of AI and allows more venture capital to invest money. I think this is all a good thing.
Zhu Shihu: Taking OpenAI’s ChatGPT as an example, the most attractive thing about it is that every time you communicate with it, you get different content. This is also the most interesting thing about it. But from an application perspective, there is a very serious problem here. When the user enters the same variable into the system every time, the variables it returns are uncontrollable, which means that it is difficult for the AI to take responsibility.
In the past and now, the intelligent customer service we use has made users feel very stupid, and its corpus is preset. But it is precisely this kind of "silliness" that makes many industries dare to use intelligent customer service, because every piece of information it feeds back to users is controlled, and every word it says is the company's responsibility. But when intelligent customer service is uncontrollable, many problems will arise in practice. For example, when a federated learning company recommends a federated learning algorithm, it will introduce what this algorithm can do. But for many industries, what they focus on is that I federated my data to you, and the scope of my data usage is certain. To be controlled, it must not be able to do certain things. What I focus on is what it cannot do, not what it can do.
Similarly, for ChatGPT, what I am concerned about is that your statement must be within the range that we can bear, and your statement must be under our control. You cannot be whimsical. Do some divergent ideas of your own. But technically speaking, unfortunately we are still unable to achieve this. AI that is too flexible and too smart can do many things that we cannot imagine and cannot bear the consequences. This is the difference between doing technology and doing industry.
Generally speaking, the relationship between technology and business models is more indirect. Generative AI can indeed help us do some creative work at this stage, but The business model here is currently not fully operational. In jobs that require higher accuracy, generative AI still has a long way to go.
5. The future trend of integration of artificial intelligence technology and industry
Tan Zhongyi: AI is an algorithm, and its integration with various enterprises is inevitable, especially with the As various enterprises carry out digitalization and intelligent transformation, AI in various enterprise scenarios, that is, AI everything, has shown an increasingly faster trend.
I personally think that the killer app in the next few years will be autonomous driving. Autonomous driving is an application that integrates AI. It is an application that can greatly facilitate people’s travel. When it is combined with cars , and roads are well combined, various algorithms can play a very good role, and at the same time, this kind of rules and regulations are improved. Killer apps that will be implemented in large numbers in the next five years and that will refresh everyone are likely to be L4 or even L5 level autonomous driving.
Zhu Shihu: In the next five years, automated trading of securities and stocks will become more mature and even replace humans. It is a very obvious trend that machines replace people in this field. And what is worthy of attention is that the previous algorithms based on the stock price factor library are being gradually abandoned. The biggest problem that AI will face in the field of automated trading in the future will no longer be changes in stock prices, but that AI1 and AI2 will become counterparties, and there will be a model confrontation between the two.
That is, learn how your opponent will deal with this stock. When the AI simulation is almost the same, the user can make money. Therefore, AI algorithms will replace 90% or even 99% of humans in the field of automated trading in the future, and the algorithms for automated trading in the future will no longer be the commonly used model based on factor libraries, but will be a set of theories based on game theory model confrontation. . All people have to do is select goals and strategies, and leave the rest to AI.
The above is the detailed content of Year-end interview: Development and transformation of artificial intelligence technology and industry. For more information, please follow other related articles on the PHP Chinese website!
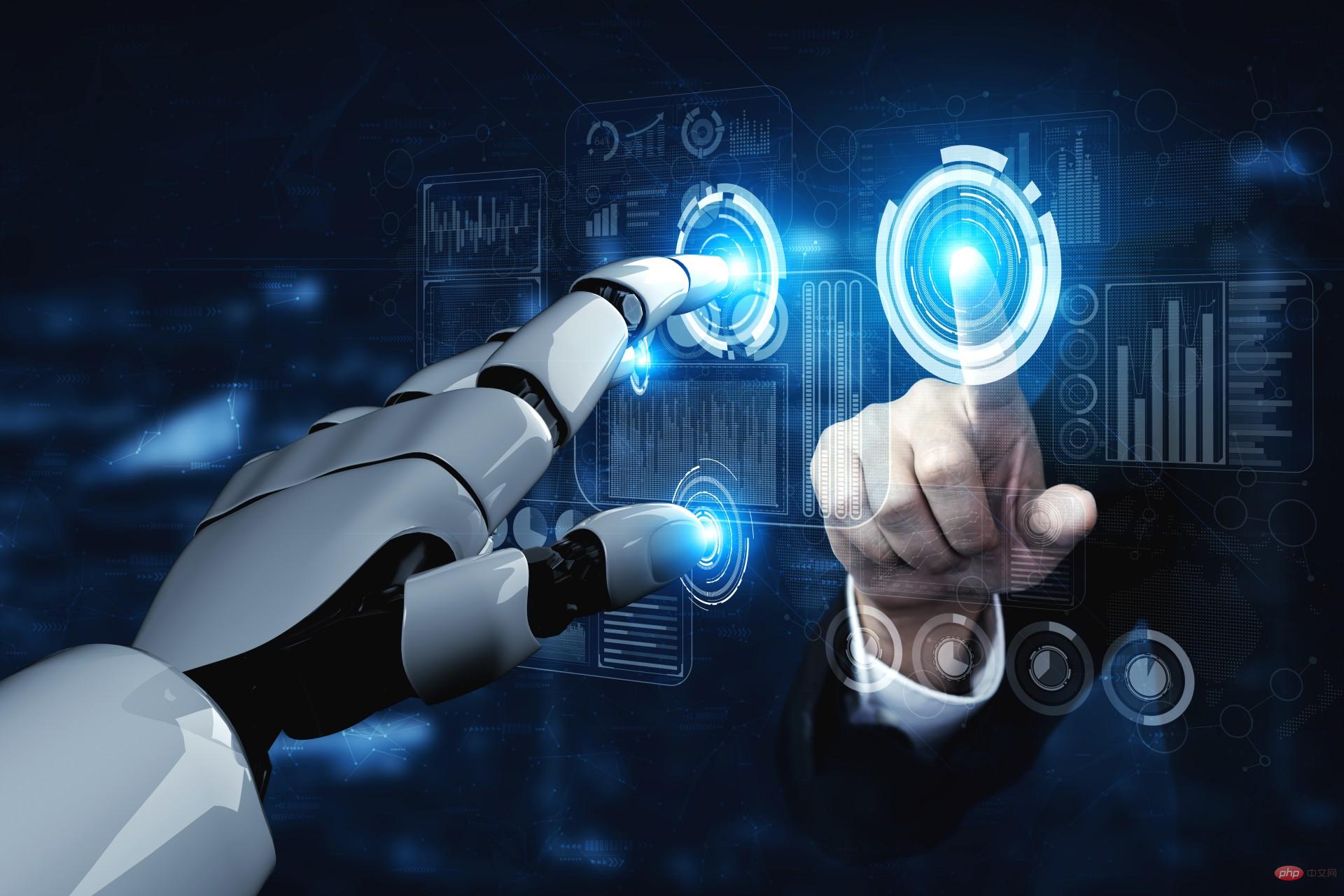
机器学习是一个不断发展的学科,一直在创造新的想法和技术。本文罗列了2023年机器学习的十大概念和技术。 本文罗列了2023年机器学习的十大概念和技术。2023年机器学习的十大概念和技术是一个教计算机从数据中学习的过程,无需明确的编程。机器学习是一个不断发展的学科,一直在创造新的想法和技术。为了保持领先,数据科学家应该关注其中一些网站,以跟上最新的发展。这将有助于了解机器学习中的技术如何在实践中使用,并为自己的业务或工作领域中的可能应用提供想法。2023年机器学习的十大概念和技术:1. 深度神经网
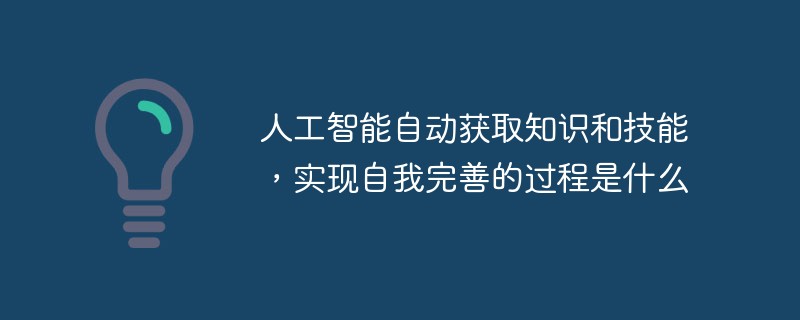
实现自我完善的过程是“机器学习”。机器学习是人工智能核心,是使计算机具有智能的根本途径;它使计算机能模拟人的学习行为,自动地通过学习来获取知识和技能,不断改善性能,实现自我完善。机器学习主要研究三方面问题:1、学习机理,人类获取知识、技能和抽象概念的天赋能力;2、学习方法,对生物学习机理进行简化的基础上,用计算的方法进行再现;3、学习系统,能够在一定程度上实现机器学习的系统。

本文将详细介绍用来提高机器学习效果的最常见的超参数优化方法。 译者 | 朱先忠审校 | 孙淑娟简介通常,在尝试改进机器学习模型时,人们首先想到的解决方案是添加更多的训练数据。额外的数据通常是有帮助(在某些情况下除外)的,但生成高质量的数据可能非常昂贵。通过使用现有数据获得最佳模型性能,超参数优化可以节省我们的时间和资源。顾名思义,超参数优化是为机器学习模型确定最佳超参数组合以满足优化函数(即,给定研究中的数据集,最大化模型的性能)的过程。换句话说,每个模型都会提供多个有关选项的调整“按钮
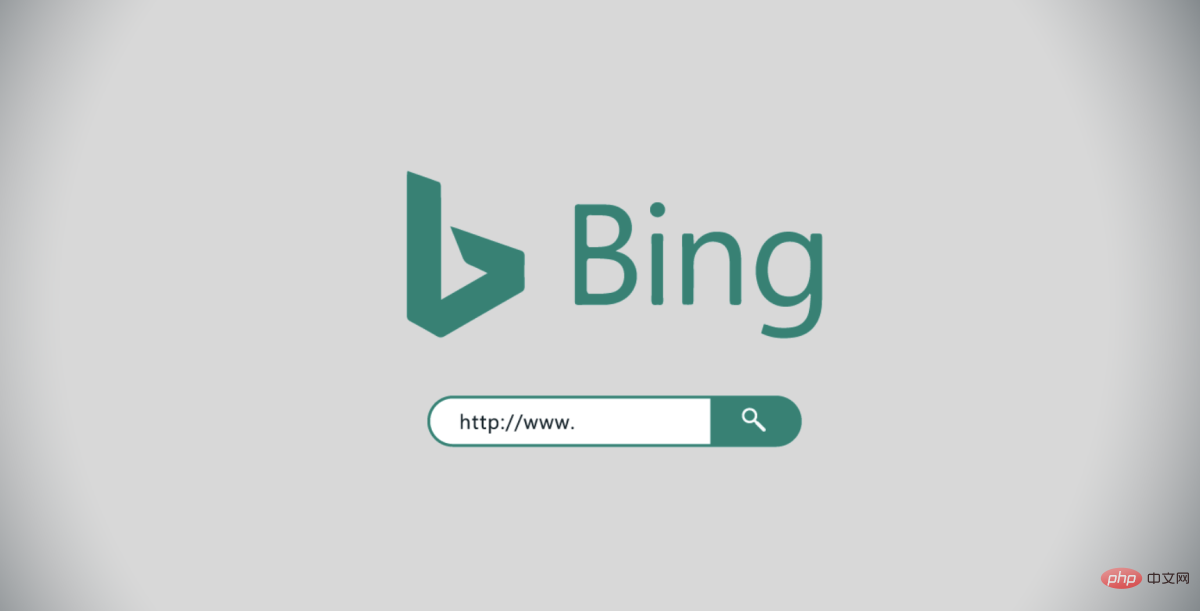
截至3月20日的数据显示,自微软2月7日推出其人工智能版本以来,必应搜索引擎的页面访问量增加了15.8%,而Alphabet旗下的谷歌搜索引擎则下降了近1%。 3月23日消息,外媒报道称,分析公司Similarweb的数据显示,在整合了OpenAI的技术后,微软旗下的必应在页面访问量方面实现了更多的增长。截至3月20日的数据显示,自微软2月7日推出其人工智能版本以来,必应搜索引擎的页面访问量增加了15.8%,而Alphabet旗下的谷歌搜索引擎则下降了近1%。这些数据是微软在与谷歌争夺生
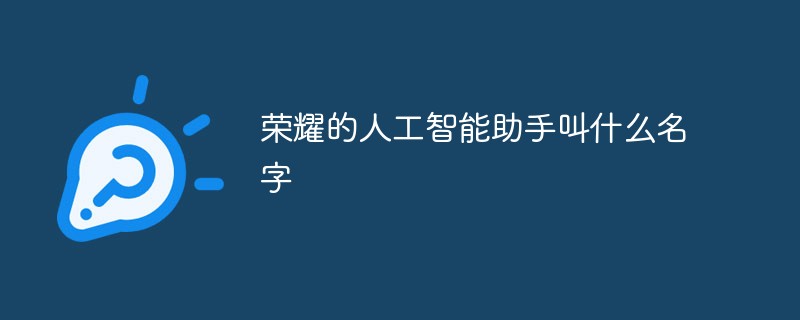
荣耀的人工智能助手叫“YOYO”,也即悠悠;YOYO除了能够实现语音操控等基本功能之外,还拥有智慧视觉、智慧识屏、情景智能、智慧搜索等功能,可以在系统设置页面中的智慧助手里进行相关的设置。
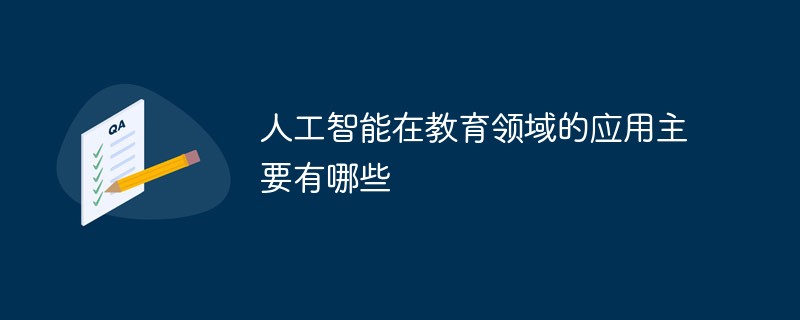
人工智能在教育领域的应用主要有个性化学习、虚拟导师、教育机器人和场景式教育。人工智能在教育领域的应用目前还处于早期探索阶段,但是潜力却是巨大的。
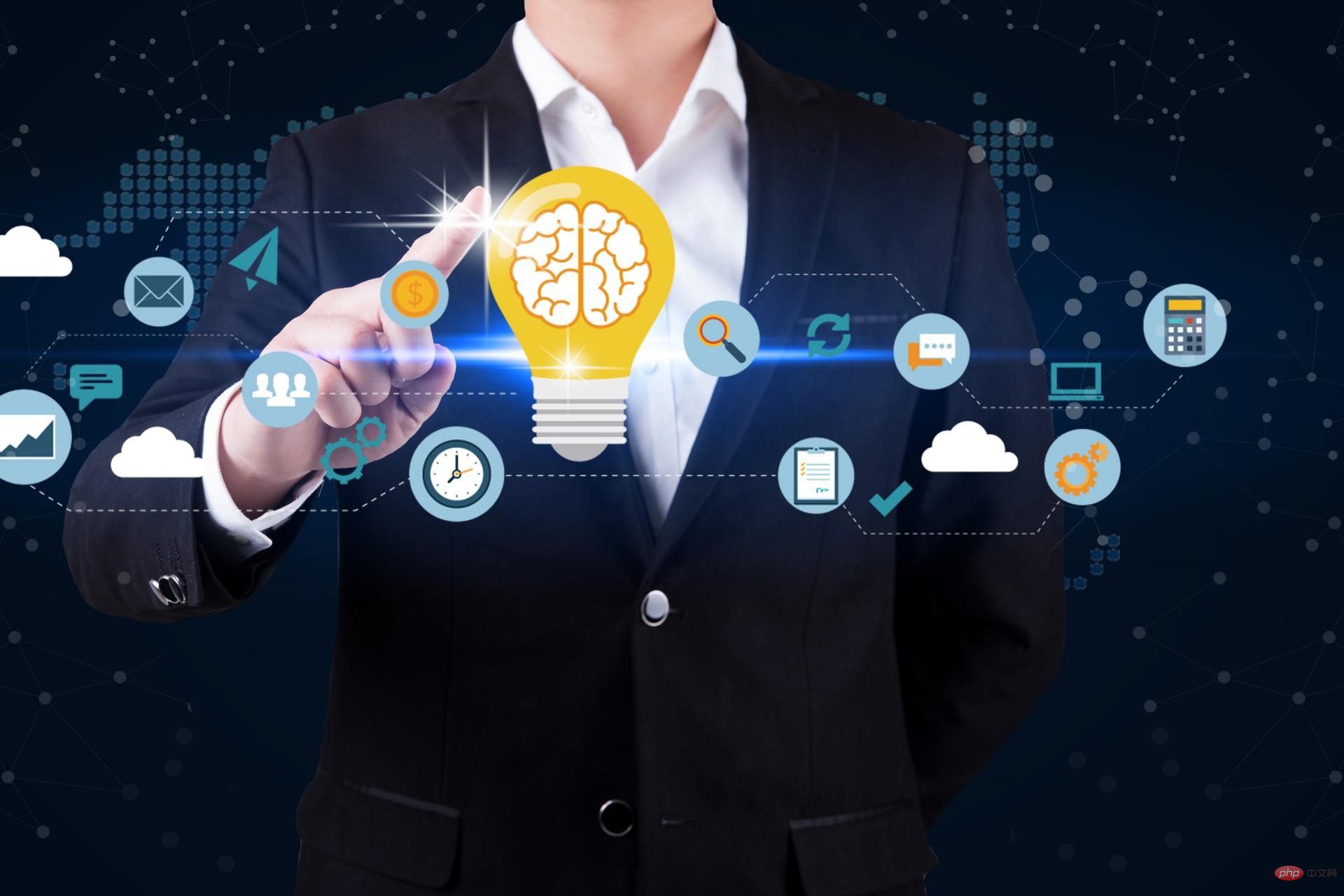
阅读论文可以说是我们的日常工作之一,论文的数量太多,我们如何快速阅读归纳呢?自从ChatGPT出现以后,有很多阅读论文的服务可以使用。其实使用ChatGPT API非常简单,我们只用30行python代码就可以在本地搭建一个自己的应用。 阅读论文可以说是我们的日常工作之一,论文的数量太多,我们如何快速阅读归纳呢?自从ChatGPT出现以后,有很多阅读论文的服务可以使用。其实使用ChatGPT API非常简单,我们只用30行python代码就可以在本地搭建一个自己的应用。使用 Python 和 C
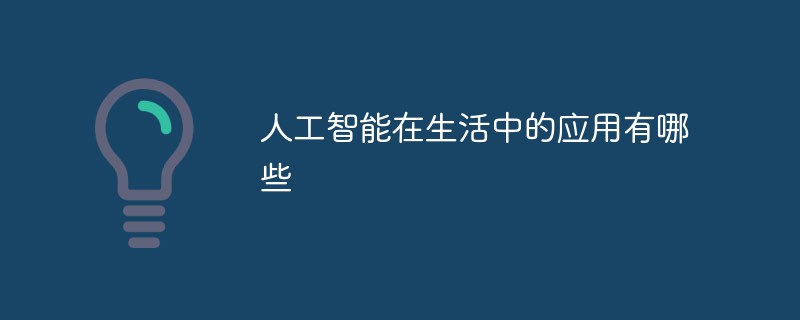
人工智能在生活中的应用有:1、虚拟个人助理,使用者可通过声控、文字输入的方式,来完成一些日常生活的小事;2、语音评测,利用云计算技术,将自动口语评测服务放在云端,并开放API接口供客户远程使用;3、无人汽车,主要依靠车内的以计算机系统为主的智能驾驶仪来实现无人驾驶的目标;4、天气预测,通过手机GPRS系统,定位到用户所处的位置,在利用算法,对覆盖全国的雷达图进行数据分析并预测。


Hot AI Tools

Undresser.AI Undress
AI-powered app for creating realistic nude photos

AI Clothes Remover
Online AI tool for removing clothes from photos.

Undress AI Tool
Undress images for free

Clothoff.io
AI clothes remover

AI Hentai Generator
Generate AI Hentai for free.

Hot Article

Hot Tools

SublimeText3 Linux new version
SublimeText3 Linux latest version

MinGW - Minimalist GNU for Windows
This project is in the process of being migrated to osdn.net/projects/mingw, you can continue to follow us there. MinGW: A native Windows port of the GNU Compiler Collection (GCC), freely distributable import libraries and header files for building native Windows applications; includes extensions to the MSVC runtime to support C99 functionality. All MinGW software can run on 64-bit Windows platforms.

SAP NetWeaver Server Adapter for Eclipse
Integrate Eclipse with SAP NetWeaver application server.
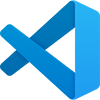
VSCode Windows 64-bit Download
A free and powerful IDE editor launched by Microsoft

Notepad++7.3.1
Easy-to-use and free code editor
