


During the Qingli period of Renzong in the Northern Song Dynasty 980 years ago, a revolution in knowledge was quietly taking place in China.
#The trigger for all this is not the words of the sages who live in temples, but the clay bricks with regular inscriptions fired piece by piece.
This revolution is "movable type printing".
The subtlety of movable type printing lies in the idea of "building block assembly": the craftsman first makes the reverse character mold of the single character, and then puts the single character according to the manuscript. Selected and printed with ink, these fonts can be used as many times as needed.
#Compared with the cumbersome process of "one print, one version" of woodblock printing, Modularization-Assembled on demand-Multiple uses This working mode geometrically improves the efficiency of printing and lays the foundation for the development and inheritance of human civilization for thousands of years.
Returning to the field of deep learning, today with the popularity of large pre-trained models, how to migrate the capabilities of a series of large models to specific downstream tasks has become a problem. The key issue.
The previous knowledge transfer or reuse method is similar to "block printing": we often need to train a new complete model according to task requirements. These methods are often accompanied by huge training costs and are difficult to scale to a large number of tasks.
So a very natural idea came up: Can we regard the neural network as an assembly of building blocks? And obtain a new network by reassembling the existing network, and use it to perform transfer learning?
##
Paper link: https://arxiv.org/abs/2210.17409
Code link: https://github.com/Adamdad/DeRy
Project homepage: https://adamdad.github.io/dery/
OpenReview: https://openreview.net/forum?id=gtCPWaY5bNh
The author comes first Disassemble the existing pre-trained model into a sub-network based on functional similarity, and then reassemble the sub-network to build an efficient and easy-to-use model for specific tasks.
The paper was accepted by NeurIPS with a score of 886 and recommended as Paper Award Nomination.
In this article, the author explores a new knowledge transfer task called Deep Model Reassembly (DeRy), using for general model reuse.
Given a set of pre-trained models trained on different data and heterogeneous architectures, deep model restructuring first splits each model into independent model chunks and then selectively to reassemble sub-model pieces within hardware and performance constraints.
This method is similar to treating the deep neural network model as building blocks: dismantle the existing large building blocks into small building blocks, and then The parts are assembled as required. The assembled new model should not only have stronger performance; the assembly process should not change the structure and parameters of the original module as much as possible to ensure its efficiency.
Breaking up and reorganizing the deep model
The method in this article can be divided into two parts. DeRy first solves a Set Cover Problem and splits all pre-trained networks according to functional levels; in the second step, DeRy formalizes the model assembly into a 0-1 integer programming problem to ensure that the assembled model is Best performance on specific tasks.
##Deep Model Reassembly
First, the author defines the problem of deep model reassembly: given a trained deep model, it is called a model library.
Each model is composed of layer links, represented by . Different networks can have completely different structures and operations, as long as the model is connected layer by layer.
Given a task, we hope to find the layer mixture model with the best performance, and the calculation amount of the model meets certain restrictions:
Performance on the task; Represents the layer operation of the th model;
This problem requires searching all permutations of all model layers in order to Maximize revenue. In essence, this task involves an extremely complex combinatorial optimization.
In order to simplify the search cost, this article first splits the model library model from the depth direction to form some shallower and smaller sub-networks; then performs splicing search at the sub-network level.
Split the network according to functional levels
DeRy’s first step is Take apart deep learning models like building blocks. The author adopts a deep network splitting method to split the deep model into some shallower small models.
The article hopes that the disassembled sub-models have different functions as much as possible. This process can be compared to the process of dismantling building blocks and putting them into toy boxes into categories: Similar building blocks are put together, and different building blocks are taken apart.
For example, split the model into the bottom layer and the high layer, and expect that the bottom layer is mainly responsible for identifying local patterns such as curves or shapes, while the high layer can judge the overall semantics of the sample.
Using the general feature similarity measurement index, the functional similarity of any model can be quantitatively measured.
The key idea is that for similar inputs, neural networks with the same function can produce similar outputs.
So, for the input tensors X and X' corresponding to the sum of the two networks, their functional similarity is defined as:
Then the model library can be divided into functional equivalence sets through functional similarity.
The subnetworks in each equivalence set have high functional similarity, and the division of each model ensures the separability of the model library.
One of the core benefits of such disassembly is that due to functional similarities, the subnetworks in each equivalent set can be regarded as approximately commutative, that is, a network block can be Replaced by another subnetwork of the same equivalence set without affecting network prediction.
The above splitting problem can be formalized as a three-layer constrained optimization problem:
The inner-level optimization of this problem is very similar to the general covering set problem or graph segmentation problem. Therefore, the author uses a heuristic Kernighan-Lin (KL) algorithm to optimize the inner layer.
The general idea is that for two randomly initialized sub-models, one layer of operations is exchanged each time. If the exchange can increase the value of the evaluation function, the exchange is retained; otherwise, it is given up. This exchange.
The outer loop here adopts a K-Means clustering algorithm.
For each network division, each subnetwork is always assigned to the function set with the largest center distance. Since the inner and outer loops are iterative and have convergence guarantee, the optimal subnetwork split according to functional levels can be obtained by solving the above problem.
Network assembly based on integer optimization
Network splitting divides each network into sub-networks, each sub-network Belongs to an equivalence set. This can be used as a search space to find the optimal network splicing for downstream tasks.
Due to the diversity of sub-models, this network assembly is a combinatorial optimization problem with a large search space, and certain search conditions are defined: Each network combination takes a network block from the same functional set and places it according to its position in the original network; the synthesized network needs to meet the computational limit. This process is described as optimization of a 0-1 integer optimization problem.
In order to further reduce the training overhead for each calculation of the combined model performance, the author draws on an alternative function in NAS training that does not require training, called for NASWOT. From this, the true performance of the network can be approximated simply by using the network's inference on a specified data set.
Through the above split-recombine process, different pre-trained models can be spliced and fused to obtain a new and stronger model.
Experimental results
Model reorganization is suitable for transfer learning
The author combines a model containing 30 different pre-trained networks The library was painstakingly disassembled and reassembled, and performance evaluated on ImageNet and 9 other downstream classification tasks.
Two different training methods were used in the experiment: Full-Tuning, which means that all parameters of the spliced model are trained; Freeze- Tuning means that only the spliced connection layer is trained.
In addition, five scale models were selected and compared, called DeRy(, ,).
As you can see in the picture above, on the ImageNet data set, the models of different scales obtained by DeRy can be better than or equal to the models of similar size in the model library.
It can be found that even if only the parameters of the link part are trained, the model can still obtain strong performance gains. For example, the DeRy(4,90,20) model achieved a Top1 accuracy of 78.6% with only 1.27M parameters trained.
At the same time, nine transfer learning experiments also verified the effectiveness of DeRy. It can be seen that without pre-training, DeRy's model can outperform other models in comparisons of various model sizes; by continuously pre-training the reassembled model, the model performance can be greatly improved. Reach the red curve.
Compared with other transfer learning methods from the model library such as LEEP or LogME, DeRy can surpass the performance limitations of the model library itself, and even be better than the best model in the original model library. Best model.
Exploring the nature of model reorganization
The author is also very curious about the model reorganization proposed in this article properties, such as "What pattern will the model be split according to?" and "What rules will the model be reorganized according to?". The author provides experiments for analysis.
Functional similarity, reassembly location and reassembly performance
The author explores how the same network block is used by other After replacing network blocks with different functional similarities, Freeze-Tuning Performance comparison of 20 epochs.
For ResNet50 trained on ImageNet, use the network blocks of the 3rd and 4th stages, Replacement with different network blocks for ResNet101, ResNeXt50 and RegNetY8G.
It can be observed that the replacement position has a great impact on performance.
For example, if the third stage is replaced by the third stage of another network, the performance of the reorganized network will be particularly strong . At the same time, functional similarity is also positively matched with recombination performance.
Network model blocks at the same depth have greater similarity, resulting in stronger model capabilities after training. This points to the dependence and positive relationship between similarity-recombination position-recombination performance.
Observation of splitting results
In the figure below, the author draws the The result of one step splitting. The color represents the similarity between the network block and the network block at the center of the equivalence set of the song.
It can be seen that the division proposed in this article tends to cluster the sub-networks together according to depth and split them. At the same time, the functional similarity data between CNN and Transformer is small, but the functional similarity between CNN and CNNs of different architectures is usually larger.
##Using NASWOT as a performance indicator
Since this article applies NASWOT for zero-training transfer prediction for the first time, the author also tested the reliability of this indicator.
In the figure below, the author calculates the NASWOT scores of different models on different data sets, and compares them with the accuracy of transfer learning plus one.
It can be observed that the NASWOT scores have obtained a more accurate performance ranking (Kendall's Tau correlation). This shows that the zero training index used in this article can effectively predict the performance of the model on downstream data.
Summary
This paper proposes a new knowledge transfer task called deep model restructuring (Deep Model Reassembly, DeRy for short). He constructs a model adapted to downstream tasks by breaking up existing heterogeneous pre-trained models and reassembling them.
The author proposes a simple two-stage implementation to accomplish this task. First, DeRy solves a covering set problem and splits all pre-trained networks according to functional levels; in the second step, DeRy formalizes the model assembly into a 0-1 integer programming problem to ensure the performance of the assembled model on specific tasks. optimal.
This work not only achieved strong performance improvements, but also mapped the possible connectivity between different neural networks.
The above is the detailed content of The correct way to play building-block deep learning! National University of Singapore releases DeRy, a new transfer learning paradigm that turns knowledge transfer into movable type printing. For more information, please follow other related articles on the PHP Chinese website!
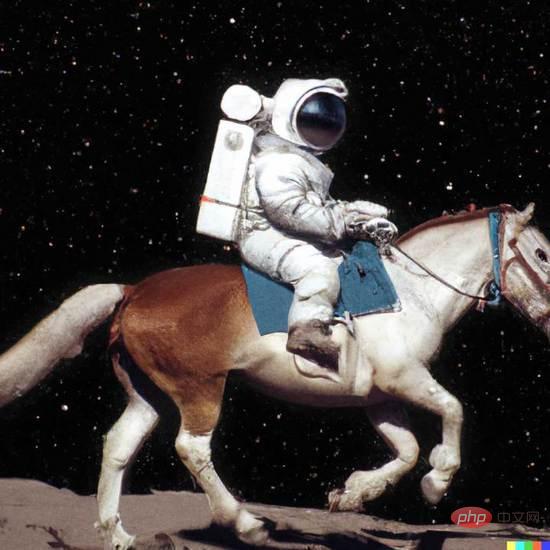
1 前言在发布DALL·E的15个月后,OpenAI在今年春天带了续作DALL·E 2,以其更加惊艳的效果和丰富的可玩性迅速占领了各大AI社区的头条。近年来,随着生成对抗网络(GAN)、变分自编码器(VAE)、扩散模型(Diffusion models)的出现,深度学习已向世人展现其强大的图像生成能力;加上GPT-3、BERT等NLP模型的成功,人类正逐步打破文本和图像的信息界限。在DALL·E 2中,只需输入简单的文本(prompt),它就可以生成多张1024*1024的高清图像。这些图像甚至
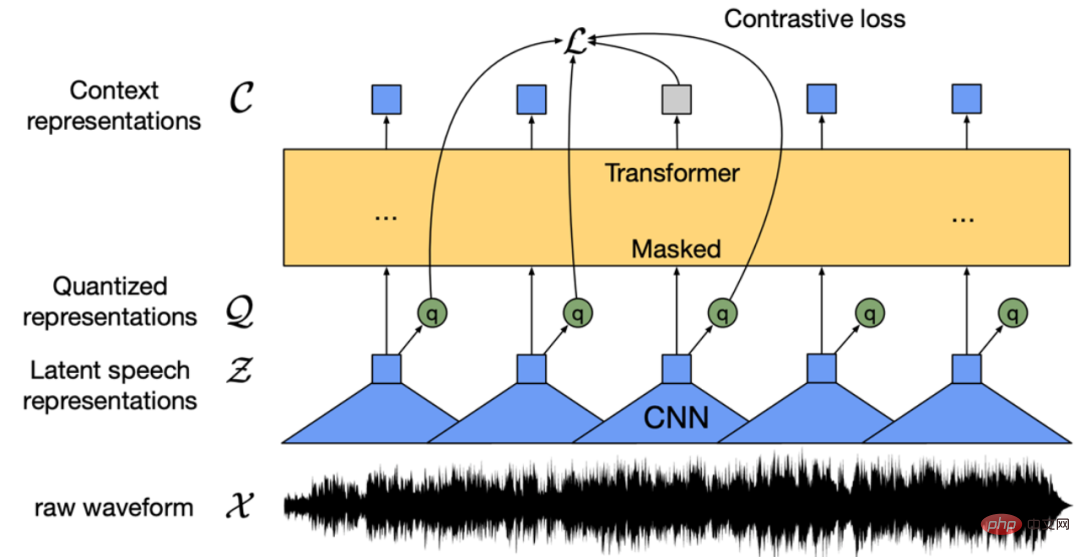
Wav2vec 2.0 [1],HuBERT [2] 和 WavLM [3] 等语音预训练模型,通过在多达上万小时的无标注语音数据(如 Libri-light )上的自监督学习,显著提升了自动语音识别(Automatic Speech Recognition, ASR),语音合成(Text-to-speech, TTS)和语音转换(Voice Conversation,VC)等语音下游任务的性能。然而这些模型都没有公开的中文版本,不便于应用在中文语音研究场景。 WenetSpeech [4] 是
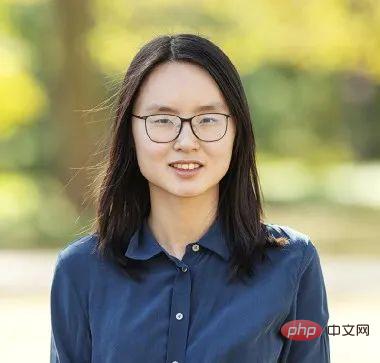
“Making large models smaller”这是很多语言模型研究人员的学术追求,针对大模型昂贵的环境和训练成本,陈丹琦在智源大会青源学术年会上做了题为“Making large models smaller”的特邀报告。报告中重点提及了基于记忆增强的TRIME算法和基于粗细粒度联合剪枝和逐层蒸馏的CofiPruning算法。前者能够在不改变模型结构的基础上兼顾语言模型困惑度和检索速度方面的优势;而后者可以在保证下游任务准确度的同时实现更快的处理速度,具有更小的模型结构。陈丹琦 普
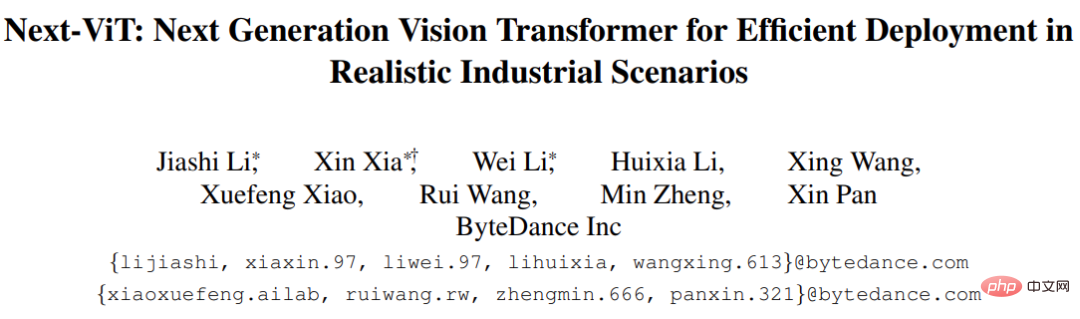
由于复杂的注意力机制和模型设计,大多数现有的视觉 Transformer(ViT)在现实的工业部署场景中不能像卷积神经网络(CNN)那样高效地执行。这就带来了一个问题:视觉神经网络能否像 CNN 一样快速推断并像 ViT 一样强大?近期一些工作试图设计 CNN-Transformer 混合架构来解决这个问题,但这些工作的整体性能远不能令人满意。基于此,来自字节跳动的研究者提出了一种能在现实工业场景中有效部署的下一代视觉 Transformer——Next-ViT。从延迟 / 准确性权衡的角度看,
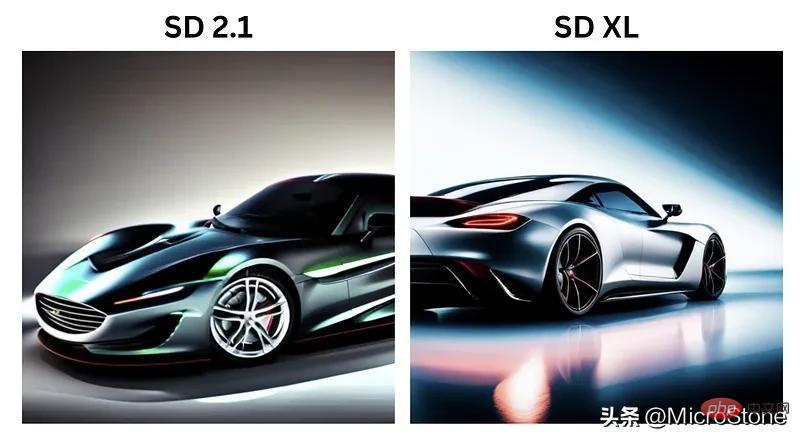
3月27号,Stability AI的创始人兼首席执行官Emad Mostaque在一条推文中宣布,Stable Diffusion XL 现已可用于公开测试。以下是一些事项:“XL”不是这个新的AI模型的官方名称。一旦发布稳定性AI公司的官方公告,名称将会更改。与先前版本相比,图像质量有所提高与先前版本相比,图像生成速度大大加快。示例图像让我们看看新旧AI模型在结果上的差异。Prompt: Luxury sports car with aerodynamic curves, shot in a
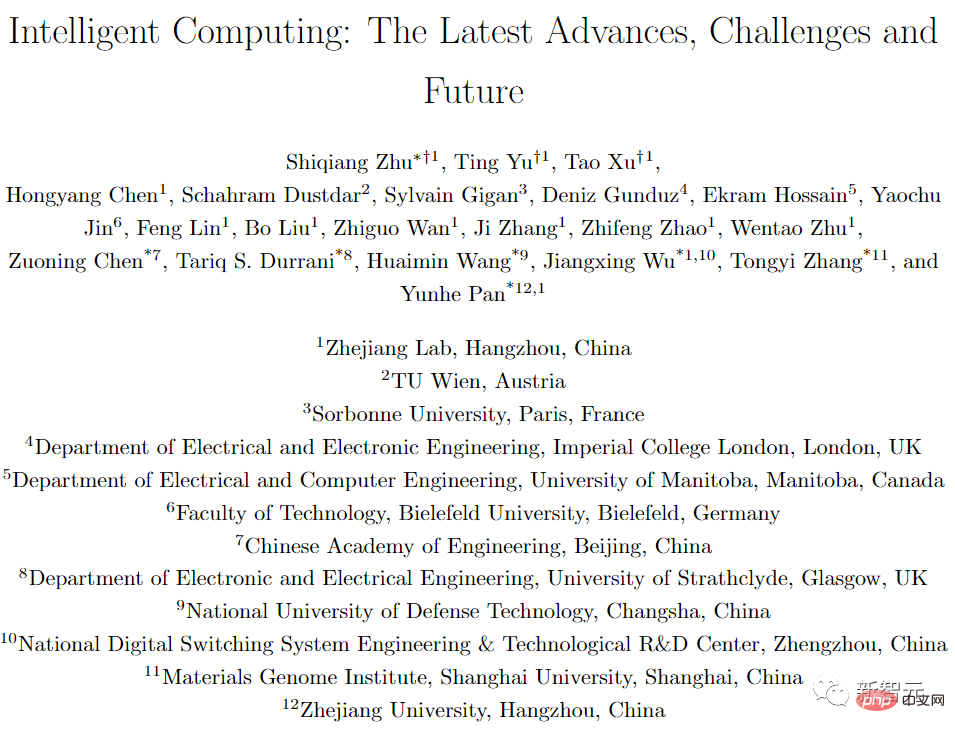
人工智能就是一个「拼财力」的行业,如果没有高性能计算设备,别说开发基础模型,就连微调模型都做不到。但如果只靠拼硬件,单靠当前计算性能的发展速度,迟早有一天无法满足日益膨胀的需求,所以还需要配套的软件来协调统筹计算能力,这时候就需要用到「智能计算」技术。最近,来自之江实验室、中国工程院、国防科技大学、浙江大学等多达十二个国内外研究机构共同发表了一篇论文,首次对智能计算领域进行了全面的调研,涵盖了理论基础、智能与计算的技术融合、重要应用、挑战和未来前景。论文链接:https://spj.scien
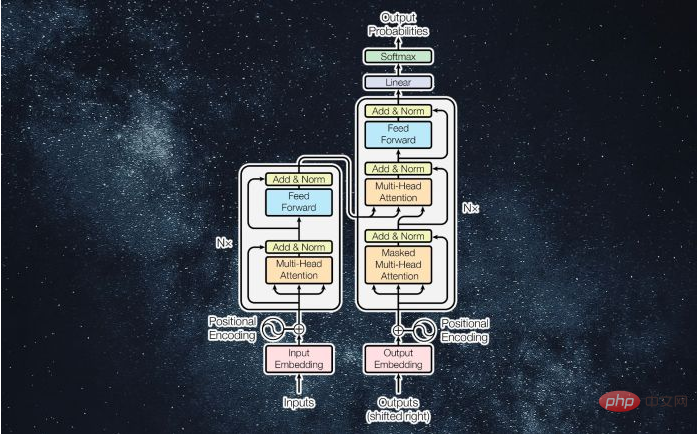
译者 | 李睿审校 | 孙淑娟近年来, Transformer 机器学习模型已经成为深度学习和深度神经网络技术进步的主要亮点之一。它主要用于自然语言处理中的高级应用。谷歌正在使用它来增强其搜索引擎结果。OpenAI 使用 Transformer 创建了著名的 GPT-2和 GPT-3模型。自从2017年首次亮相以来,Transformer 架构不断发展并扩展到多种不同的变体,从语言任务扩展到其他领域。它们已被用于时间序列预测。它们是 DeepMind 的蛋白质结构预测模型 AlphaFold
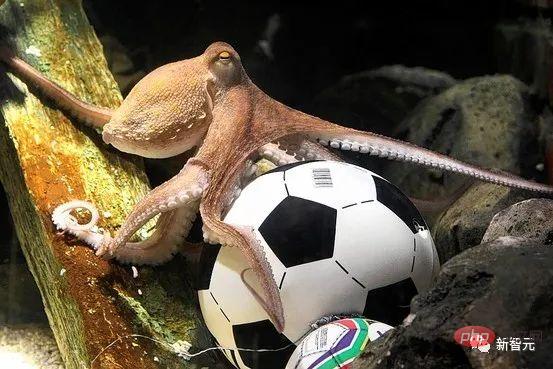
说起2010年南非世界杯的最大网红,一定非「章鱼保罗」莫属!这只位于德国海洋生物中心的神奇章鱼,不仅成功预测了德国队全部七场比赛的结果,还顺利地选出了最终的总冠军西班牙队。不幸的是,保罗已经永远地离开了我们,但它的「遗产」却在人们预测足球比赛结果的尝试中持续存在。在艾伦图灵研究所(The Alan Turing Institute),随着2022年卡塔尔世界杯的持续进行,三位研究员Nick Barlow、Jack Roberts和Ryan Chan决定用一种AI算法预测今年的冠军归属。预测模型图


Hot AI Tools

Undresser.AI Undress
AI-powered app for creating realistic nude photos

AI Clothes Remover
Online AI tool for removing clothes from photos.

Undress AI Tool
Undress images for free

Clothoff.io
AI clothes remover

AI Hentai Generator
Generate AI Hentai for free.

Hot Article

Hot Tools

Zend Studio 13.0.1
Powerful PHP integrated development environment
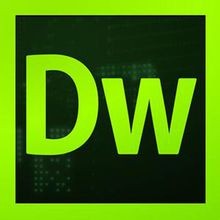
Dreamweaver CS6
Visual web development tools
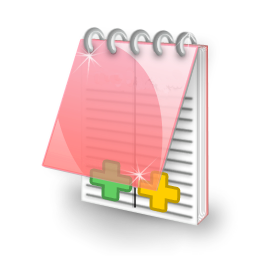
EditPlus Chinese cracked version
Small size, syntax highlighting, does not support code prompt function
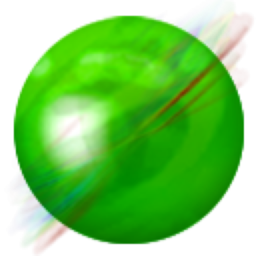
ZendStudio 13.5.1 Mac
Powerful PHP integrated development environment

SublimeText3 Linux new version
SublimeText3 Linux latest version
