


With huge advances in machine learning and quantum computing, we now have new and more powerful tools to collaborate with researchers across industries in new ways and radically accelerate the progress of groundbreaking scientific discoveries. .
The theme of this year-end summary of Google is "Natural Science". The author of the article is John Platt, an outstanding scientist at Google Research. He graduated from the California Institute of Technology with a Ph.D. in 1989 University.
Since joining Google Research eight years ago, I have been privileged to be part of a community of talented researchers dedicated to Focused on applying cutting-edge computing technologies to advance the possibilities of applied science, the team is currently exploring topics in the physical and natural sciences, from helping to organize the world's protein and genomic information to benefit people life, to using quantum computers to improve our understanding of the nature of the universe.
Use machine learning to solve the mysteries of biology
The extraordinary complexity of biology has fascinated countless researchers, from exploring the mysteries of the brain to exploring the structure of proteins , to the genome encoding the language of life, Google has been collaborating with scientists from other leading organizations around the world to address grand challenges in connectomics, protein function prediction, and genomics, and to make innovations available to the broader community. used by the scientific community.
Neurobiology
In 2018, an application developed by Google is to explore information is transmitted through neuronal pathways in the zebrafish brain, providing insight into how zebrafish engage in social behaviors like swarming.
Paper link: https://www.nature.com/articles/s41592-018-0049-4
Working with researchers at the Max Planck Institute for Biology Intelligence, researchers used computers to recreate part of a zebra 3D electron microscope image of a fish brain.
This is also a milestone in the use of imaging and computational pipelines to map neuronal circuits in the cerebellum, It is also another advancement in the field of connectomics.
The technology involved in this work can even be applied to fields beyond neuroscience. For example, to solve the problem of processing large connectomics data sets, researchers at Google developed and Released TensorStore, an open source C and Python software library specifically designed to store and operate n-dimensional data, and is also suitable for storing large data sets in other fields.
## Code link: https://github.com/google/tensorstore
By comparing human language processing to autoregressive deep language models (DLMs), researchers have used machine learning to shed light on how the human brain performs functions as distinctive as language.
Paper link: https://www.nature.com/articles/s41593-022-01026 -4
In this study, Google teamed up with researchers from Princeton University and NYU Grossman School of Medicine to have participants listen to a 30-minute podcast. Their brain activity was also recorded using electrocorticography.
The recorded results show that the human brain and DLM share computational principles for processing language, including continuous next word prediction, context-dependent embedding, and post-onset suprise calculation based on word matching, that is, the human brain can measure The degree of surprise of the word and correlate the surprise signal with the degree of prediction of the word by DLM.
These results provide new conclusions about language processing in the human brain and suggest that DLM can be used to reveal valuable insights into the neural basis of language.
Biochemistry
Machine learning has also led to significant advances in understanding biological sequences Progress, researchers leverage recent advances in deep learning to accurately predict protein function from raw amino acid sequences.
Paper link: https://www.nature.com/articles/s41587-021-01179-w
Google is also working closely with the European Molecular Biology Laboratory's European Bioinformatics Institute (EMBL-EBI) to carefully evaluate the performance of the models and has added hundreds of millions to the public protein databases UniProt, Pfam/interPro and MGnify function annotation.
Paper link: https://www.nature.com/articles/s41587-021-01179 -w.epdf
Human annotation of protein databases may be an arduous and slow process, but the machine learning method proposed by Google has made the annotation speed a huge leap. .
For example, Pfam has added more annotations than all other efforts combined in the past decade, and the millions of scientists around the world who access these databases each year can now leverage that annotation for research. .
Although the first draft of the human genome was released in 2003, it was not completed due to technical limitations of sequencing technology. incomplete.
In 2022, the Telomere-2-Telomere (T2T) consortium is addressing these previously unobtainable regions (including 5 complete chromosome arms and nearly 200 million bases of new DNA sequence). Remarkable achievements have been made in studying areas that are both interesting and important to questions of human biology, evolution, and disease.
Google's open source genome variant caller, DeepVariant, is one of the tools used by the T2T Consortium to prepare for the release of a complete 3.055 billion base pair human genome sequence.
## Paper link: https://www.nature.com/articles/nbt.4235
The T2T consortium is also using Google’s open-source approach DeepConsensus to provide on-device error correction for Pacific Biosciences long-read sequencing instruments, in T2T’s latest study of comprehensive pan-genome resources. , can represent the breadth of human genetic diversity.
Paper link: https://www.nature.com/articles/s41587-022-01435 -7.epdf
Application of quantum computing in new physical discoveriesQuantum computing is still in its infancy in promoting scientific discovery, but it has There is great potential, so Google is exploring ways to improve quantum computing capabilities so that quantum computing can become a tool for scientific discoveries and breakthroughs.
By collaborating with physicists from around the world, the researchers are starting to use existing quantum computers to create completely new physics experiments. One of the quantum experimental problems is: when a sensor measures a When detecting objects, a computer is needed to process the data from the sensors.
In the traditional processing process, the sensor data needs to be converted into classical information before processing.
For quantum computing, quantum data from sensors can be directly processed, and the data from quantum sensors can be directly provided to quantum algorithms without measurement, which will have greater advantages than traditional computers.
Paper link: https://www.science.org/doi/10.1126/science.abn7293
In a Science paper recently published by Google in collaboration with researchers from multiple universities, experimental results show that as long as a quantum computer is directly coupled to a quantum sensor and runs a Learning algorithms, quantum computing can extract information from far fewer experiments than classical computing.
Even on currently immature mid-scale quantum computers, "quantum machine learning" can produce exponential advantages on data sets.
Paper link: https://arxiv.org/abs/2112.00778
Since experimental data is often the limiting factor in scientific discovery, quantum machine learning algorithms have the potential to fully unleash the power of quantum computers. What’s even better is that the results of this work also apply to learning The output of quantum computing, such as the output of quantum simulations, is difficult to extract.
Even without quantum machine learning, a promising application of quantum computers is the experimental exploration of quantum systems that cannot be observed or simulated.
In 2022, the Quantum AI team used this method to observe the first experimental evidence of multiple microwave photons in a bound state using a superconducting qubit.
Paper link: https://www.nature.com/articles/s41586-022-05348 -y
Photons usually require additional nonlinear elements to interact, and Google's quantum computer's simulation of these interactions surprised the researchers: They originally expected these The existence of bound states relies on fragile conditions, but they were actually found to be robust even to relatively strong perturbations.
Given Google’s initial success in applying quantum computing to achieve breakthroughs in physics, researchers are excited about the technology The possibilities also hold great promise, allowing future breakthrough discoveries to have as significant a social impact as the creation of the transistor or the Global Positioning System.
Quantum computing as a scientific tool is very promising!
The above is the detailed content of Explore the origins of nature! The seventh bullet of Google's 2022 year-end summary: How can 'Biochemical Environmental Materials” reap the dividends of machine learning?. For more information, please follow other related articles on the PHP Chinese website!
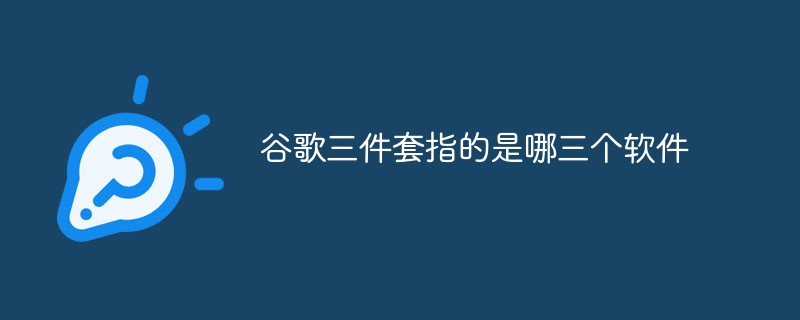
谷歌三件套指的是:1、google play商店,即下载各种应用程序的平台,类似于移动助手,安卓用户可以在商店下载免费或付费的游戏和软件;2、Google Play服务,用于更新Google本家的应用和Google Play提供的其他第三方应用;3、谷歌服务框架(GMS),是系统软件里面可以删除的一个APK程序,通过谷歌平台上架的应用和游戏都需要框架的支持。
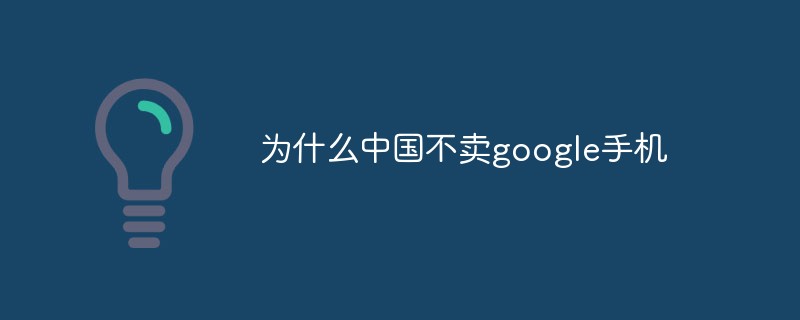
中国不卖google手机的原因:谷歌已经全面退出中国市场了,所以不能在中国销售,在国内是没有合法途径销售。在中国消费市场中,消费者大都倾向于物美价廉以及功能实用的产品,所以竞争实力本就因政治因素大打折扣的谷歌手机主体市场一直不在中国大陆。
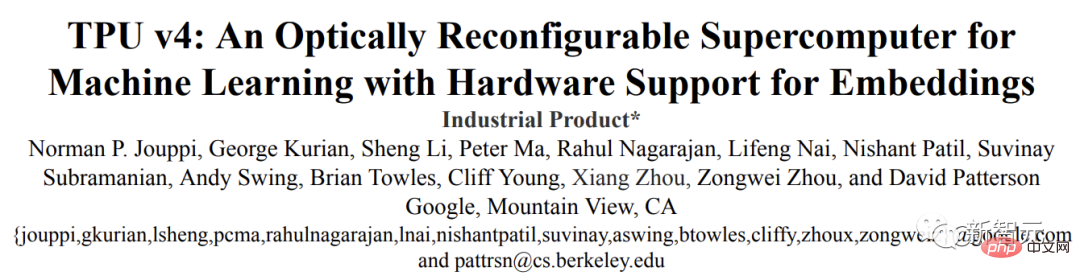
虽然谷歌早在2020年,就在自家的数据中心上部署了当时最强的AI芯片——TPU v4。但直到今年的4月4日,谷歌才首次公布了这台AI超算的技术细节。论文地址:https://arxiv.org/abs/2304.01433相比于TPU v3,TPU v4的性能要高出2.1倍,而在整合4096个芯片之后,超算的性能更是提升了10倍。另外,谷歌还声称,自家芯片要比英伟达A100更快、更节能。与A100对打,速度快1.7倍论文中,谷歌表示,对于规模相当的系统,TPU v4可以提供比英伟达A100强1.
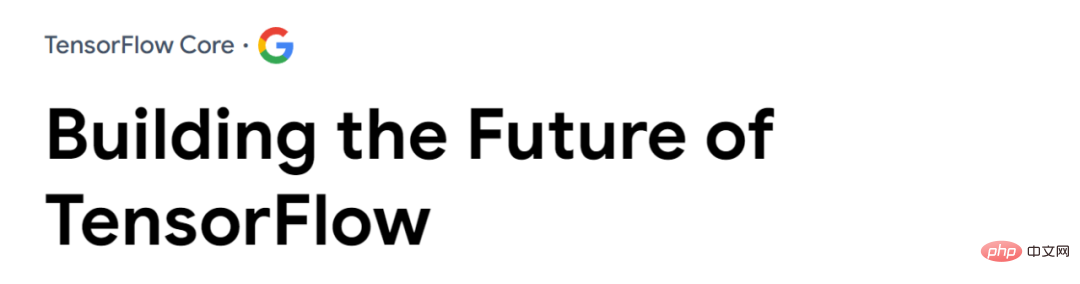
2015 年,谷歌大脑开放了一个名为「TensorFlow」的研究项目,这款产品迅速流行起来,成为人工智能业界的主流深度学习框架,塑造了现代机器学习的生态系统。从那时起,成千上万的开源贡献者以及众多的开发人员、社区组织者、研究人员和教育工作者等都投入到这一开源软件库上。然而七年后的今天,故事的走向已经完全不同:谷歌的 TensorFlow 失去了开发者的拥护。因为 TensorFlow 用户已经开始转向 Meta 推出的另一款框架 PyTorch。众多开发者都认为 TensorFlow 已经输掉
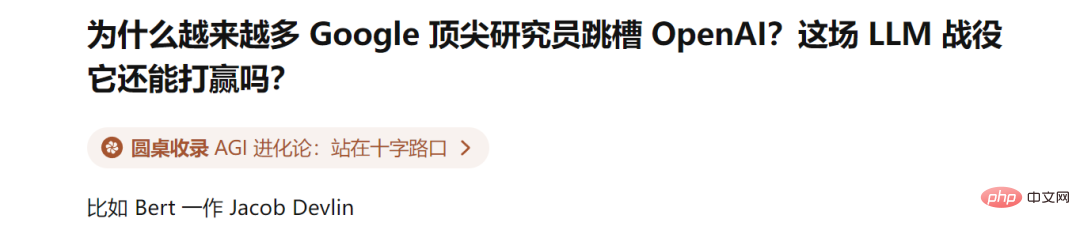
前几天,谷歌差点遭遇一场公关危机,Bert一作、已跳槽OpenAI的前员工Jacob Devlin曝出,Bard竟是用ChatGPT的数据训练的。随后,谷歌火速否认。而这场争议,也牵出了一场大讨论:为什么越来越多Google顶尖研究员跳槽OpenAI?这场LLM战役它还能打赢吗?知友回复莱斯大学博士、知友「一堆废纸」表示,其实谷歌和OpenAI的差距,是数据的差距。「OpenAI对LLM有强大的执念,这是Google这类公司完全比不上的。当然人的差距只是一个方面,数据的差距以及对待数据的态度才
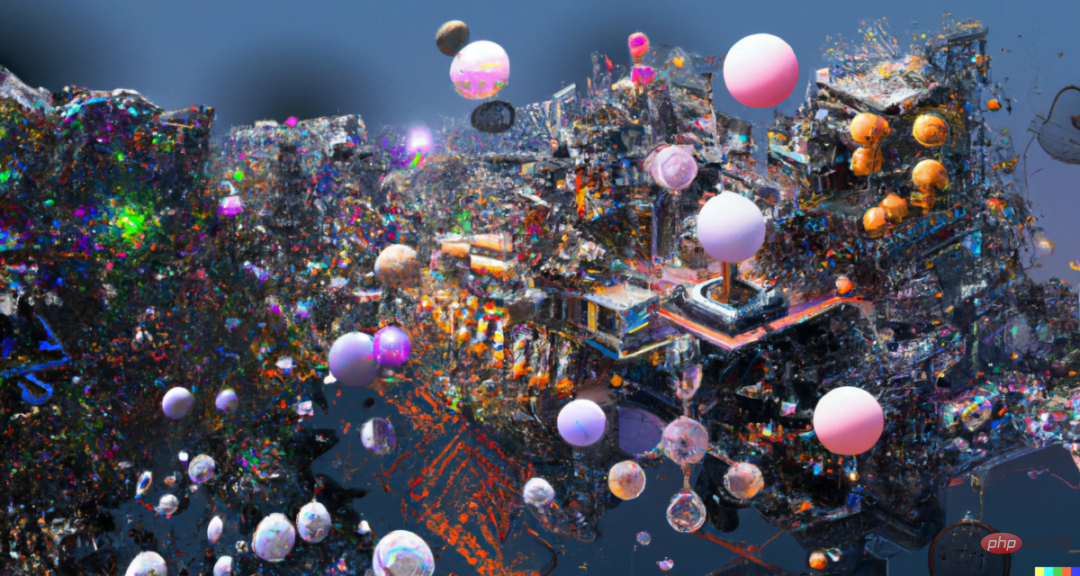
由于可以做一些没训练过的事情,大型语言模型似乎具有某种魔力,也因此成为了媒体和研究员炒作和关注的焦点。当扩展大型语言模型时,偶尔会出现一些较小模型没有的新能力,这种类似于「创造力」的属性被称作「突现」能力,代表我们向通用人工智能迈进了一大步。如今,来自谷歌、斯坦福、Deepmind和北卡罗来纳大学的研究人员,正在探索大型语言模型中的「突现」能力。解码器提示的 DALL-E神奇的「突现」能力自然语言处理(NLP)已经被基于大量文本数据训练的语言模型彻底改变。扩大语言模型的规模通常会提高一系列下游N
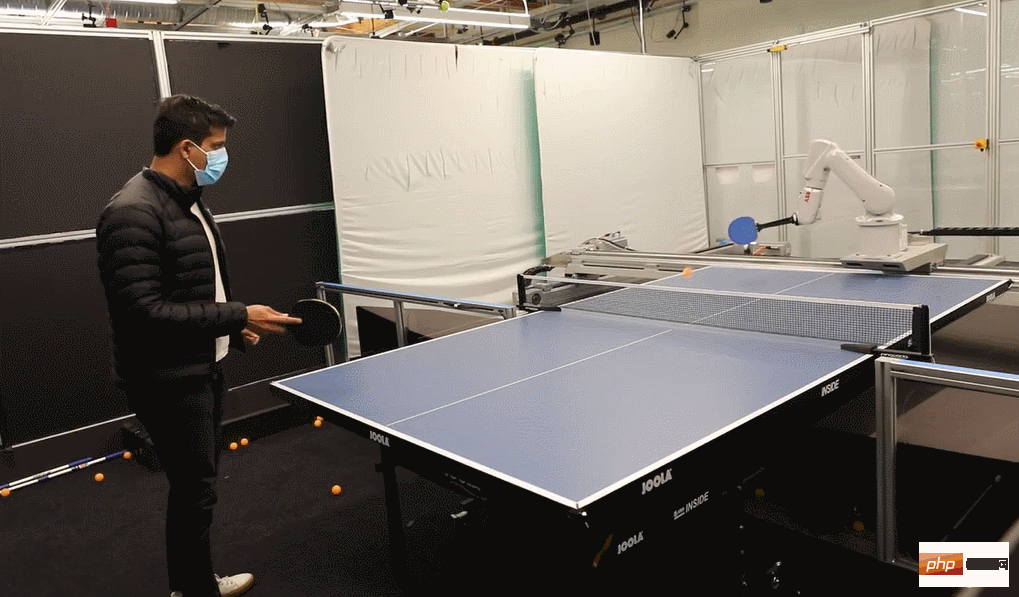
让一位乒乓球爱好者和机器人对打,按照机器人的发展趋势来看,谁输谁赢还真说不准。机器人拥有灵巧的可操作性、腿部运动灵活、抓握能力出色…… 已被广泛应用于各种挑战任务。但在与人类互动紧密的任务中,机器人的表现又如何呢?就拿乒乓球来说,这需要双方高度配合,并且球的运动非常快速,这对算法提出了重大挑战。在乒乓球比赛中,首要的就是速度和精度,这对学习算法提出了很高的要求。同时,这项运动具有高度结构化(具有固定的、可预测的环境)和多智能体协作(机器人可以与人类或其他机器人一起对打)两大特点,使其成为研究人
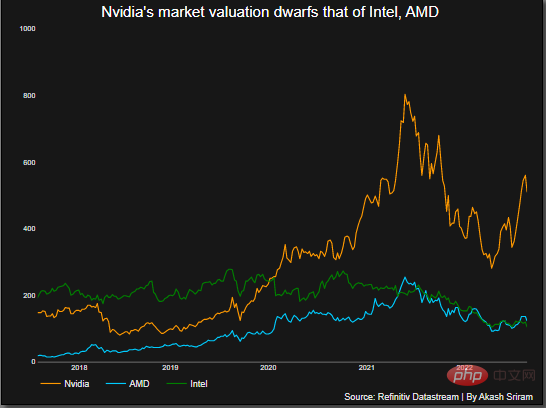
ChatGPT在手,有问必答。你可知,与它每次对话的计算成本简直让人泪目。此前,分析师称ChatGPT回复一次,需要2美分。要知道,人工智能聊天机器人所需的算力背后烧的可是GPU。这恰恰让像英伟达这样的芯片公司豪赚了一把。2月23日,英伟达股价飙升,使其市值增加了700多亿美元,总市值超5800亿美元,大约是英特尔的5倍。在英伟达之外,AMD可以称得上是图形处理器行业的第二大厂商,市场份额约为20%。而英特尔持有不到1%的市场份额。ChatGPT在跑,英伟达在赚随着ChatGPT解锁潜在的应用案


Hot AI Tools

Undresser.AI Undress
AI-powered app for creating realistic nude photos

AI Clothes Remover
Online AI tool for removing clothes from photos.

Undress AI Tool
Undress images for free

Clothoff.io
AI clothes remover

AI Hentai Generator
Generate AI Hentai for free.

Hot Article

Hot Tools

SublimeText3 Linux new version
SublimeText3 Linux latest version
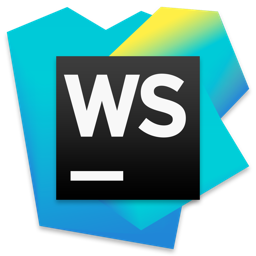
WebStorm Mac version
Useful JavaScript development tools
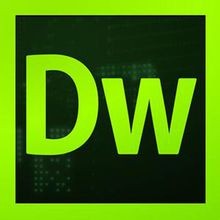
Dreamweaver CS6
Visual web development tools

SAP NetWeaver Server Adapter for Eclipse
Integrate Eclipse with SAP NetWeaver application server.

SublimeText3 Chinese version
Chinese version, very easy to use
