


Artificial intelligence (AI) and machine learning (ML) have received widespread attention in recent years because of their potential to set new paradigms in healthcare delivery. Machine learning is said to be set to transform many aspects of healthcare delivery, with radiology and pathology among the first specialties to take advantage of the technology.
In the coming years, medical imaging professionals will have access to a rapidly expanding AI diagnostic toolkit for detecting, classifying, segmenting and extracting quantitative imaging features. It will ultimately lead to accurate medical data interpretation, enhanced diagnostic processes and improved clinical outcomes. Advances in deep learning (DL) and other artificial intelligence methods have shown efficacy in supporting clinical practice with increased precision and productivity.
Barriers to the application of artificial intelligence in health care
Although artificial intelligence can enhance the capabilities of health care and diagnostic processes through automated integration, there are still some challenges. The lack of annotated data makes training deep learning algorithms very difficult. In addition, the black-box nature leads to the opacity of the results of deep learning algorithms. Clinical practices face significant challenges when incorporating artificial intelligence into healthcare workflows.
The main challenges in successfully implementing artificial intelligence in medical practice are as follows:
- Ethical and legal issues of data sharing
- Training healthcare practitioners and patients to operate complex AI models
- Managing strategic changes to bring AI innovation into practice
1. Ethical and legal issues that prevent AI developers from accessing high-quality data sets
Whether integrating artificial intelligence in medical imaging or using deep learning techniques to manipulate clinical diagnostic procedures, high-quality healthcare datasets are the key to success. When we tried to identify key barriers to developing AI models for healthcare, we found that ethical and legal issues have been by far the biggest barriers to developing AI-driven machine learning models.
Because patient health information is private and confidential and protected by law, healthcare providers must adhere to strict privacy and data security policies. However, this places an ethical and legal obligation on the healthcare practitioner not to provide data to any third party. This prevents AI developers from accessing high-quality datasets to develop AI training data for healthcare machine learning models.
In addition to the ambiguity of existing laws and the challenges associated with sharing data between organizations, uncertainty arises about the responsibilities and permissible scope of the design and implementation of artificial intelligence systems, raising legal and ethical questions. question.
2. Training healthcare practitioners and patients to use complex AI models
Integrating artificial intelligence systems can improve medical efficiency without affecting quality, allowing patients to receive better and more Personalized care. Investigation, assessment and treatment can be simplified and improved through the use of smart and efficient artificial intelligence systems. However, implementing AI in healthcare is challenging as it needs to be user-friendly and deliver value to patients and healthcare professionals.
Artificial intelligence systems should be easy to use, user-friendly, self-learning, and do not require extensive prior knowledge or training. In addition to being easy to use, AI systems should save time and not require a different digital operating system to run. In order for healthcare practitioners to effectively operate AI-driven machines and applications, the features and functionality of AI models must be simple.
3. Managing strategic change to put AI innovation into practice
Healthcare experts point out that implementing an AI system in county councils will be a challenge due to the healthcare system’s internal strategic change management capabilities. difficult. In order to improve the ability to implement strategic cooperation with artificial intelligence systems at the regional level, experts emphasized the need to establish infrastructure and joint ventures with familiar structures and processes. The goals, objectives and mission of the organization need to be achieved through this action to achieve lasting improvements throughout the organization.
Healthcare professionals can only partially determine how organizations implement change because change is a complex process. In the Comprehensive Framework for Implementation Research (CFIR), we need to focus on organizational capabilities, environment, culture, and leadership, which all play a role in the "internal environment." Maintaining a well-functioning organization and delivery system is part of the ability to apply innovation to healthcare practice.
Integrating artificial intelligence into medical imaging through data annotation to enhance healthcare
An imaging technology that can see inside the body without opening it through surgery is called medical imaging technology (MIT). The use of artificial intelligence in clinical diagnosis has demonstrated some of the most promising applications, including x-ray photography, computed tomography, magnetic resonance imaging, and ultrasound imaging.
Machine learning will improve the radiology patient experience every step of the way. The application of machine learning in medical imaging initially focused on image analysis and developing tools to improve the efficiency and productivity of radiologists. The same tools often enable more precise diagnosis and treatment planning, or help reduce missed diagnoses, thereby improving patient outcomes.
Artificial intelligence and machine learning have a broader role in radiology beyond clinical decision-making and can help improve the patient experience throughout the entire imaging process—from initial imaging exam planning to the end of diagnosis and follow-up.
Looking at trends in the healthcare system, we can see that the application of machine learning has expanded beyond diagnostics and medical imaging. It enhances the data acquisition process, ensuring the highest image quality for every examination, and assists imaging departments to efficiently maximize operational performance.
Summary
As the healthcare industry is at the dawn of a new wave of technological innovation driven by artificial intelligence, it is time for healthcare providers to develop a roadmap for integrating artificial intelligence into clinical practice . As the global population continues to grow, healthcare practitioners must invest in technologies that can improve patient care and transform clinical workflows. Among technologies capable of revolutionizing clinical processes, the application of artificial intelligence in healthcare delivery is undoubtedly at the forefront.
The above is the detailed content of Challenges for successful implementation of artificial intelligence in healthcare. For more information, please follow other related articles on the PHP Chinese website!
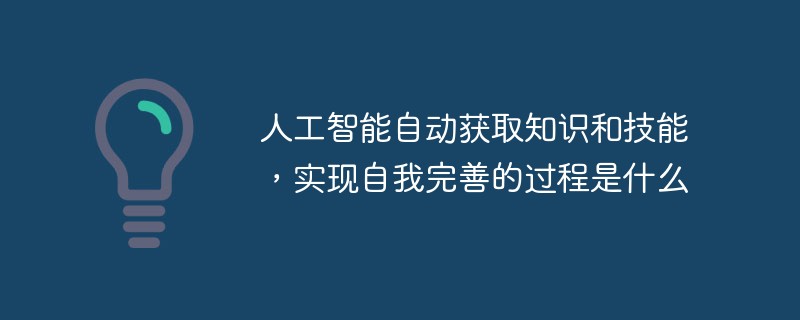
实现自我完善的过程是“机器学习”。机器学习是人工智能核心,是使计算机具有智能的根本途径;它使计算机能模拟人的学习行为,自动地通过学习来获取知识和技能,不断改善性能,实现自我完善。机器学习主要研究三方面问题:1、学习机理,人类获取知识、技能和抽象概念的天赋能力;2、学习方法,对生物学习机理进行简化的基础上,用计算的方法进行再现;3、学习系统,能够在一定程度上实现机器学习的系统。
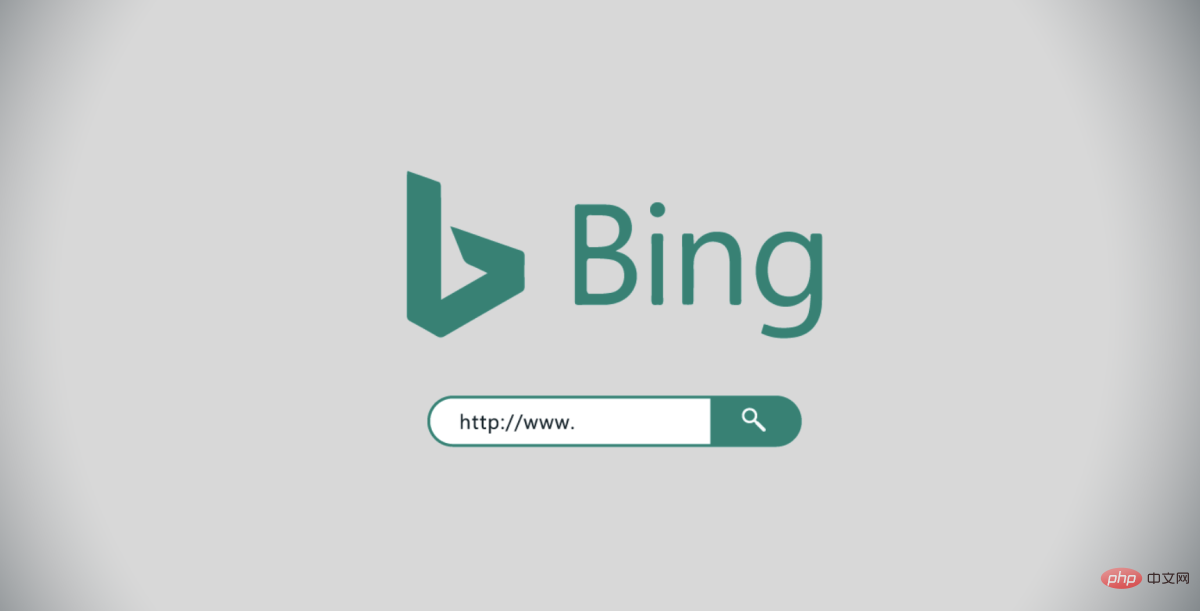
截至3月20日的数据显示,自微软2月7日推出其人工智能版本以来,必应搜索引擎的页面访问量增加了15.8%,而Alphabet旗下的谷歌搜索引擎则下降了近1%。 3月23日消息,外媒报道称,分析公司Similarweb的数据显示,在整合了OpenAI的技术后,微软旗下的必应在页面访问量方面实现了更多的增长。截至3月20日的数据显示,自微软2月7日推出其人工智能版本以来,必应搜索引擎的页面访问量增加了15.8%,而Alphabet旗下的谷歌搜索引擎则下降了近1%。这些数据是微软在与谷歌争夺生
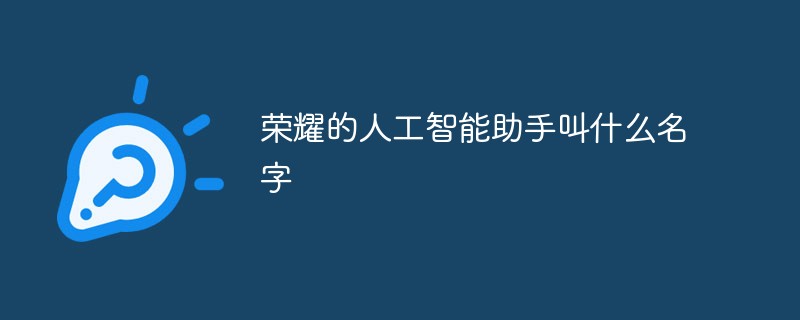
荣耀的人工智能助手叫“YOYO”,也即悠悠;YOYO除了能够实现语音操控等基本功能之外,还拥有智慧视觉、智慧识屏、情景智能、智慧搜索等功能,可以在系统设置页面中的智慧助手里进行相关的设置。
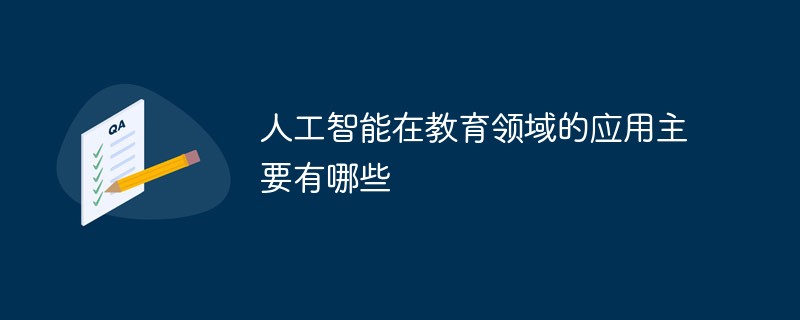
人工智能在教育领域的应用主要有个性化学习、虚拟导师、教育机器人和场景式教育。人工智能在教育领域的应用目前还处于早期探索阶段,但是潜力却是巨大的。
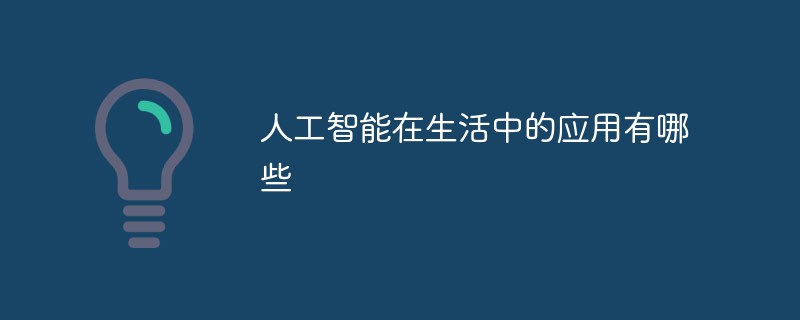
人工智能在生活中的应用有:1、虚拟个人助理,使用者可通过声控、文字输入的方式,来完成一些日常生活的小事;2、语音评测,利用云计算技术,将自动口语评测服务放在云端,并开放API接口供客户远程使用;3、无人汽车,主要依靠车内的以计算机系统为主的智能驾驶仪来实现无人驾驶的目标;4、天气预测,通过手机GPRS系统,定位到用户所处的位置,在利用算法,对覆盖全国的雷达图进行数据分析并预测。
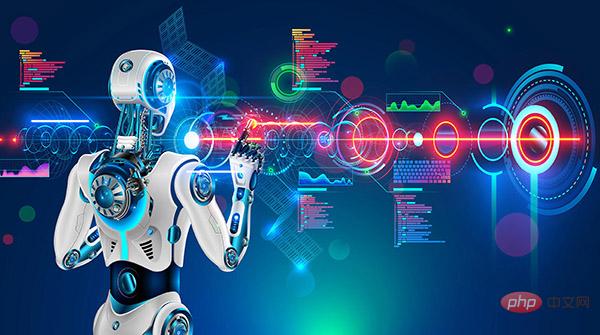
自从人工智能(AI)概念大热之后,其进入各个产业领域的速度加快。不过,鉴于医疗领域的复杂性,AI在医疗产业的拓展并不顺利,唯一获得规模化的正向增长的是手术机器人,其他在经历了多年的发展,整个领域仍属于不成熟的早期市场,难以真正商业化。 如果按照人工智能进入医疗的领域来区分,主要分类是药物研发、诊疗、影像识别、手术机器人和健康管理5大方向。但从实践来看,药物研发领域虽有一些进展,但离真正规模化仍有一定距离,而诊疗领域的工具属性更强,对医疗的作用只具备信息化附属的角色。真正在过去5年吸引市场的领域是
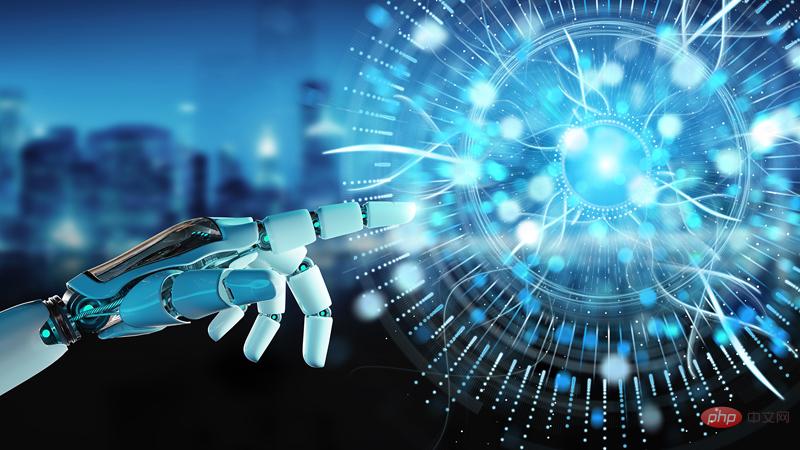
建筑行业正在迅速拥抱数字化以及人工智能和机器学习的使用,有可能改变建筑物的设计、建造和运营方式。 长期以来,建筑行业以其传统的、通常是手动的操作流程而为人所知,但随着技术的兴起,这种情况正在迅速改变。人工智能 (AI) 和机器学习 (ML) 在建筑行业中变得越来越重要,为提高效率、准确性和安全性提供了新的机会。这些技术正在改变建筑物的设计、建造和运营方式,并催生了“智能建筑”和“智能建设”的概念。智能建筑是那些采用各种技术来优化其运营并提高能源效率、舒适度和安全性的建筑。这包括智能照明和HVAC
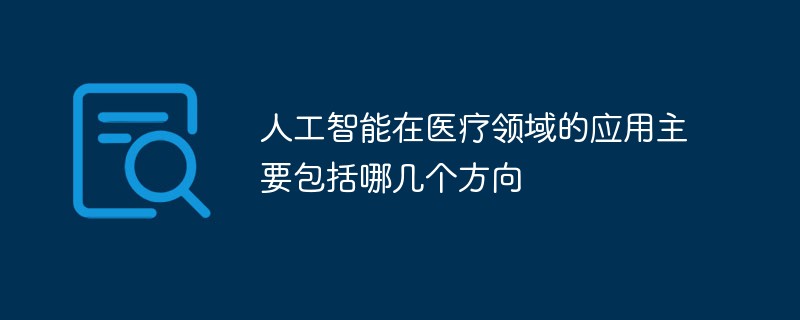
人工智能在医疗领域的应用主要包括医疗机器人、智能药物研发、智能诊疗、智能影像识别和智能健康管理等五个方向。其中医疗机器人主要分为能够读取人体神经信号的可穿戴型机器人和能够承担手术或医疗保健功能的机器人。


Hot AI Tools

Undresser.AI Undress
AI-powered app for creating realistic nude photos

AI Clothes Remover
Online AI tool for removing clothes from photos.

Undress AI Tool
Undress images for free

Clothoff.io
AI clothes remover

AI Hentai Generator
Generate AI Hentai for free.

Hot Article

Hot Tools
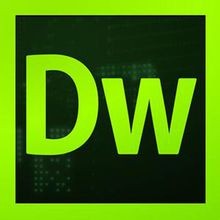
Dreamweaver CS6
Visual web development tools

Zend Studio 13.0.1
Powerful PHP integrated development environment
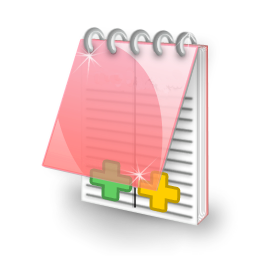
EditPlus Chinese cracked version
Small size, syntax highlighting, does not support code prompt function

SublimeText3 English version
Recommended: Win version, supports code prompts!
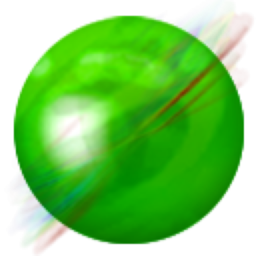
ZendStudio 13.5.1 Mac
Powerful PHP integrated development environment
