


What KPIs can be used to measure the success of artificial intelligence projects?
A research report released by the research firm IDC in June 2020 showed that approximately 28% of artificial intelligence plans failed. Reasons cited in the report were a lack of expertise, a lack of relevant data and a lack of a sufficiently integrated development environment. In order to establish a process for continuous improvement of machine learning and avoid getting stuck, identifying key performance indicators (KPIs) is now a priority.
#In the upper reaches of the industry, data scientists can define the technical performance indicators of the model. They will vary depending on the type of algorithm used. In the case of a regression aimed at predicting someone's height as a function of their age, for example, one can resort to linear determination coefficients.
An equation to measure the quality of the prediction can be used: If the square of the correlation coefficient is zero, the regression line determines the 0% point distribution. On the other hand, if the coefficient is 100%, the number is equal to 1. Therefore, this indicates that the quality of the predictions is very good.
Deviation of predictions from reality
Another metric for evaluating regression is the least squares method, which refers to the loss function. It involves quantifying the error by calculating the sum of squared deviations between the actual value and the predicted line, and then fitting the model by minimizing the squared error. In the same logic, one can utilize the mean absolute error method, which consists in calculating the average of the fundamental values of the deviations.
Charlotte Pierron-Perlès, who is responsible for strategy, data and artificial intelligence services at French consultancy Capgemini, concluded: "In any case, this amounts to measuring the gap with what we are trying to predict."
For example, In classification algorithms for spam detection, it is necessary to look for false positives and false negatives of spam. Pierron Perlès explains: "For example, we developed a machine learning solution for a cosmetics group that optimizes the efficiency of a production line. The aim was to identify defective cosmetics at the beginning of the production line that could cause production interruptions. We worked closely with the factory operators Discussions followed with them seeking a model to complete the detection even if it meant detecting false positives, that is, qualified cosmetics could be mistaken for defective."
Based on false positives and false negatives The concept of three other metrics allows the evaluation of classification models:
(1) Recall (R) refers to a measure of model sensitivity. It is the ratio of correctly identified true positives (taking positive coronavirus tests as an example) to all true positives that should have been detected (positive coronavirus tests and negative coronavirus tests were actually positive): R = true positives / true positives false Negative.
(2) Precision (P) refers to the measure of accuracy. It is the ratio of correct true positives (positive COVID-19 tests) to all results determined to be positive (positive COVID-19 tests negative COVID-19 tests): P = true positives / true positives false positives.
(3) Harmonic mean (F-score) measures the model’s ability to give correct predictions and reject other predictions: F=2×precision×recall/precision-recall
of the model Promotion
DavidTsangHinSun, chief senior data scientist at French ESNKeyrus company, emphasized: "Once a model is built, its generalization ability will become a key indicator."
So how to estimate it? By measuring the difference between predictions and expected results, and then understanding how that difference evolves over time. He explains, "After a period of time, we may encounter divergence. This may be due to underlearning (or overfitting) due to insufficient training of the data set in terms of quality and quantity."
So what is the solution? For example, in the case of image recognition models, adversarial generative networks can be used to increase the number of pictures learned through rotation or distortion. Another technique (applicable to classification algorithms): synthetic minority oversampling, which consists of increasing the number of low-occurrence examples in the data set through oversampling.
Disagreement can also occur in the case of over-learning. In this configuration, the model will not be restricted to the expected correlations after training, but due to overspecialization, it will capture the noise generated by the field data and produce inconsistent results. DavidTsangHinSun pointed out, "It is then necessary to check the quality of the training data set and possibly adjust the weight of the variables."
While the economic key performance indicators (KPIs) remain. Stéphane Roder, CEO of French consulting firm AIBuilders, believes: “We have to ask ourselves whether the error rate is consistent with the business challenges. For example, the insurance company Lemonade has developed a machine learning module that can respond to customer requests within 3 minutes after filing a claim. information (including photos) to pay insurance benefits to the customer. Taking into account the savings, a certain error rate incurs costs. Over the entire life cycle of the model, especially compared to the total cost of ownership (TCO), from development to maintenance , it is very important to check this measurement value."
Adoption Level
Even within the same company, expected key performance indicators (KPIs) may vary. Charlotte Pierron Perlès of Capgemini noted: "We developed a consumption forecasting engine for a French retailer with an international standing. It turned out that the precise targeting of the model differed between products sold in department stores and new products. Sales of the latter Dynamics depend on factors, especially those related to market reaction, which are, by definition, less controllable."
The final key performance indicator is adoption levels. Charlotte Pierron-Perlès said: "Even if a model is of good quality, it is not enough on its own. This requires the development of artificial intelligence products with a user-oriented experience that can be used for business and realize the promise of machine learning."
Stéphane Roder concluded: “This user experience will also allow users to provide feedback, which will help provide artificial intelligence knowledge outside the daily production data flow.”
The above is the detailed content of What KPIs can be used to measure the success of artificial intelligence projects?. For more information, please follow other related articles on the PHP Chinese website!
![[Ghibli-style images with AI] Introducing how to create free images with ChatGPT and copyright](https://img.php.cn/upload/article/001/242/473/174707263295098.jpg?x-oss-process=image/resize,p_40)
The latest model GPT-4o released by OpenAI not only can generate text, but also has image generation functions, which has attracted widespread attention. The most eye-catching feature is the generation of "Ghibli-style illustrations". Simply upload the photo to ChatGPT and give simple instructions to generate a dreamy image like a work in Studio Ghibli. This article will explain in detail the actual operation process, the effect experience, as well as the errors and copyright issues that need to be paid attention to. For details of the latest model "o3" released by OpenAI, please click here⬇️ Detailed explanation of OpenAI o3 (ChatGPT o3): Features, pricing system and o4-mini introduction Please click here for the English version of Ghibli-style article⬇️ Create Ji with ChatGPT
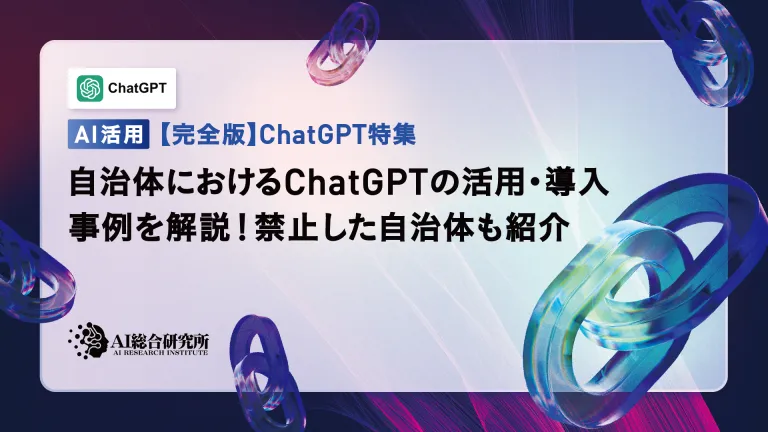
As a new communication method, the use and introduction of ChatGPT in local governments is attracting attention. While this trend is progressing in a wide range of areas, some local governments have declined to use ChatGPT. In this article, we will introduce examples of ChatGPT implementation in local governments. We will explore how we are achieving quality and efficiency improvements in local government services through a variety of reform examples, including supporting document creation and dialogue with citizens. Not only local government officials who aim to reduce staff workload and improve convenience for citizens, but also all interested in advanced use cases.
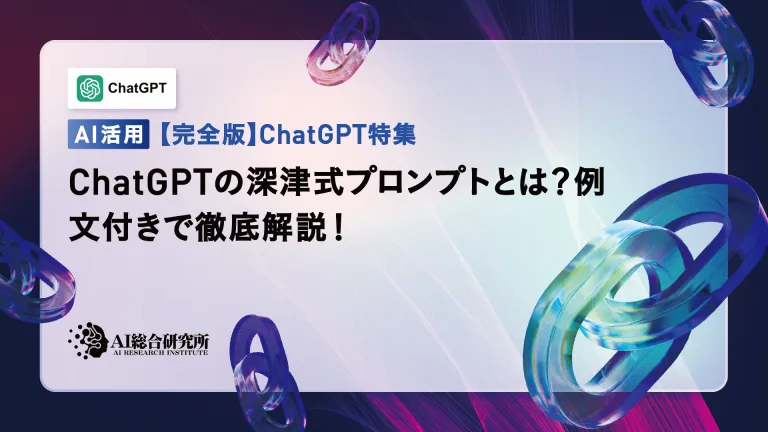
Have you heard of a framework called the "Fukatsu Prompt System"? Language models such as ChatGPT are extremely excellent, but appropriate prompts are essential to maximize their potential. Fukatsu prompts are one of the most popular prompt techniques designed to improve output accuracy. This article explains the principles and characteristics of Fukatsu-style prompts, including specific usage methods and examples. Furthermore, we have introduced other well-known prompt templates and useful techniques for prompt design, so based on these, we will introduce C.
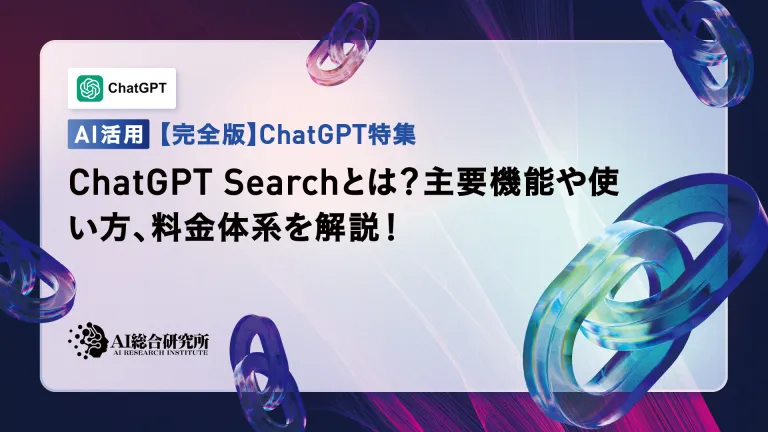
ChatGPT Search: Get the latest information efficiently with an innovative AI search engine! In this article, we will thoroughly explain the new ChatGPT feature "ChatGPT Search," provided by OpenAI. Let's take a closer look at the features, usage, and how this tool can help you improve your information collection efficiency with reliable answers based on real-time web information and intuitive ease of use. ChatGPT Search provides a conversational interactive search experience that answers user questions in a comfortable, hidden environment that hides advertisements
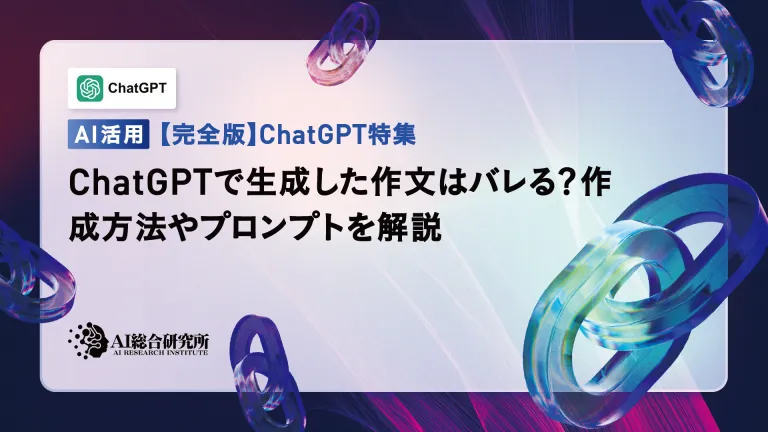
In a modern society with information explosion, it is not easy to create compelling articles. How to use creativity to write articles that attract readers within a limited time and energy requires superb skills and rich experience. At this time, as a revolutionary writing aid, ChatGPT attracted much attention. ChatGPT uses huge data to train language generation models to generate natural, smooth and refined articles. This article will introduce how to effectively use ChatGPT and efficiently create high-quality articles. We will gradually explain the writing process of using ChatGPT, and combine specific cases to elaborate on its advantages and disadvantages, applicable scenarios, and safe use precautions. ChatGPT will be a writer to overcome various obstacles,
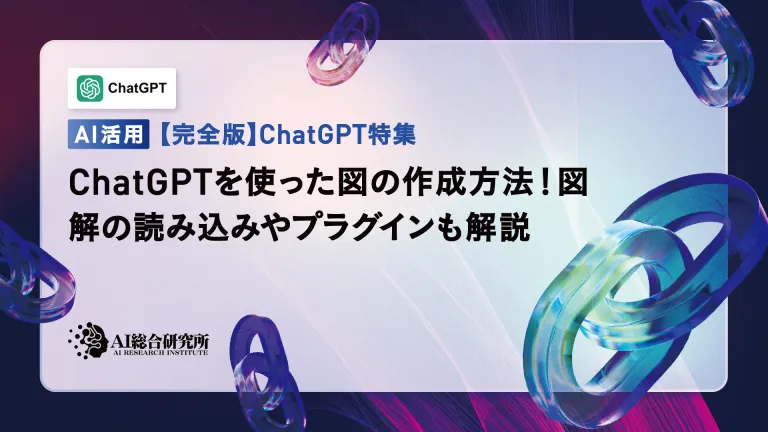
An efficient guide to creating charts using AI Visual materials are essential to effectively conveying information, but creating it takes a lot of time and effort. However, the chart creation process is changing dramatically due to the rise of AI technologies such as ChatGPT and DALL-E 3. This article provides detailed explanations on efficient and attractive diagram creation methods using these cutting-edge tools. It covers everything from ideas to completion, and includes a wealth of information useful for creating diagrams, from specific steps, tips, plugins and APIs that can be used, and how to use the image generation AI "DALL-E 3."
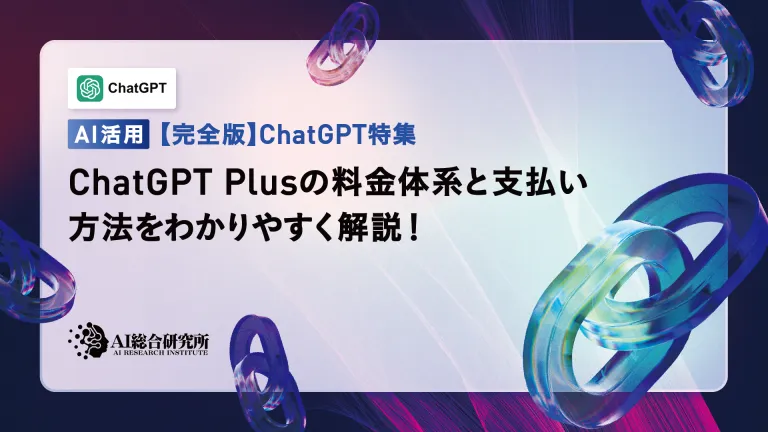
Unlock ChatGPT Plus: Fees, Payment Methods and Upgrade Guide ChatGPT, a world-renowned generative AI, has been widely used in daily life and business fields. Although ChatGPT is basically free, the paid version of ChatGPT Plus provides a variety of value-added services, such as plug-ins, image recognition, etc., which significantly improves work efficiency. This article will explain in detail the charging standards, payment methods and upgrade processes of ChatGPT Plus. For details of OpenAI's latest image generation technology "GPT-4o image generation" please click: Detailed explanation of GPT-4o image generation: usage methods, prompt word examples, commercial applications and differences from other AIs Table of contents ChatGPT Plus Fees Ch
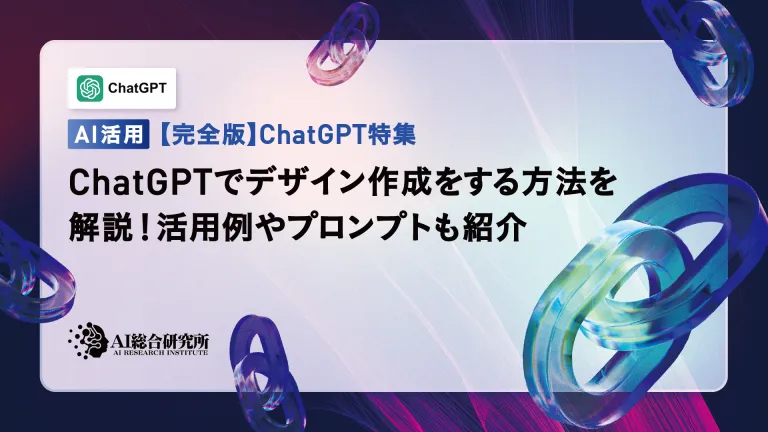
How to use ChatGPT to streamline your design work and increase creativity This article will explain in detail how to create a design using ChatGPT. We will introduce examples of using ChatGPT in various design fields, such as ideas, text generation, and web design. We will also introduce points that will help you improve the efficiency and quality of a variety of creative work, such as graphic design, illustration, and logo design. Please take a look at how AI can greatly expand your design possibilities. table of contents ChatGPT: A powerful tool for design creation


Hot AI Tools

Undresser.AI Undress
AI-powered app for creating realistic nude photos

AI Clothes Remover
Online AI tool for removing clothes from photos.

Undress AI Tool
Undress images for free

Clothoff.io
AI clothes remover

Video Face Swap
Swap faces in any video effortlessly with our completely free AI face swap tool!

Hot Article

Hot Tools
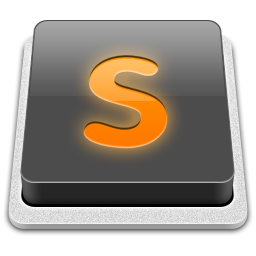
SublimeText3 Mac version
God-level code editing software (SublimeText3)
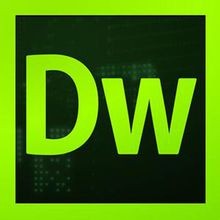
Dreamweaver CS6
Visual web development tools
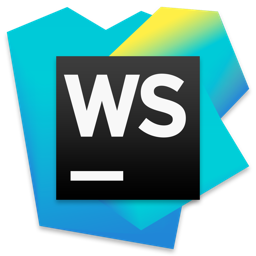
WebStorm Mac version
Useful JavaScript development tools

PhpStorm Mac version
The latest (2018.2.1) professional PHP integrated development tool

mPDF
mPDF is a PHP library that can generate PDF files from UTF-8 encoded HTML. The original author, Ian Back, wrote mPDF to output PDF files "on the fly" from his website and handle different languages. It is slower than original scripts like HTML2FPDF and produces larger files when using Unicode fonts, but supports CSS styles etc. and has a lot of enhancements. Supports almost all languages, including RTL (Arabic and Hebrew) and CJK (Chinese, Japanese and Korean). Supports nested block-level elements (such as P, DIV),
