Today, as big data proliferates in every aspect of business, IT teams are faced with the daunting task of handling the sheer volume and complexity of operations. As a result, enterprise demand for AIOps is growing.
AIOps (artificial intelligence IT operations) uses big data and machine learning (ML) to predict and identify at a scale and speed that humans cannot achieve , diagnose and solve IT problems.
A recent report from private equity and venture capital firm Insight Partners estimates that the AIOps platform market size will increase at a compound annual growth rate of 32.2% from 2021 to 2028, from approximately $2.83 billion in 2021 Growth to $199.3 billion in 2028. That said, effective AIOps solutions don’t happen overnight.
A complete AIOps solution comes from a solution that has been refined over a long period of time and contains three basic ingredients: data, analysis and expertise in different fields.
Data
Without data, successful AIOps does not exist, and this part is crucial. While data is in ample supply, the challenge is getting it in a usable and reliable form. AIOps relies on hundreds or even thousands of data points from disparate sources such as network performance, business systems, and customer support, all generated on a second-by-second basis, and in many cases at sub-second rates. How you handle large amounts of data can make or break an AIOps solution. Separate pipelines for on-device and off-device data management yield the best results in terms of speed, cost-effectiveness, and maximum efficiency.
The traditional single internal data processing model can no longer cope with the complexity and volume of today’s data sets. Instead, consider building or re-architecting your data processing funnel into two parts: a lean, fast processing pipeline that handles time-critical analysis via a real-time, on-premises data bus, and a more robust pipeline that handles time-critical analysis in the cloud. the remaining data. Reduce on-premises data production to a minimum and allocate the cloud (equipped with elastic computing and more sophisticated storage capabilities) to process the remaining data, enabling faster and more cost-effective data synthesis.
A decoupled pipeline model that manages both internal and external data can enhance an organization’s ability to process millions of data points per hour. Machine learning (ML) algorithms can help prioritize incoming data from each pipeline and transform raw, unstructured data into usable metrics critical to customer service or IT operations teams. The efficiency and speed gained from the two-pronged system also enables organizations to deploy enhanced monitoring capabilities to gain real-time visibility and long-term trend information on network performance.
Analysis
The second key factor to AIOps success is analytics. Analysis in AIOps is divided into two stages, including exploratory analysis (screening out trends or anomalies from raw data that require additional inspection) and advanced statistical analysis (translated into actionable insights). While exploratory research plays an integral role, engineering teams are often eager to jump to advanced statistical analysis as data flows through the pipeline. Bypassing this initial stage can lead to data bias—injecting bias into the AIOps process and incorrectly identifying problems, rendering AI/ML algorithms useless and leading to undesirable operational consequences.
Exploratory analytics rely on ML and data scientists to identify and determine the specific metrics that matter. In the process, IT teams may gravitate toward ML—an exciting and efficient technology. But pure ML is not always the most effective method of analysis. ML attempts to solve a specific problem based on a specific set of parameters. Engineers write ML algorithms based on the metrics they believe are needed to reach conclusion A, B, or C—thus ruling out other possible solutions or statistics.
In contrast, statisticians and data scientists examine raw data not with specific results in mind, but rather with examining the data for patterns or anomalies. Manual data review is tedious, but experts can identify immediate IT solutions without the need for advanced statistical analysis.
When the team is confident that the trends or anomalies identified during the exploration phase are correct, they can move on to advanced statistical analysis and training AI/ML algorithms. Even AI/ML requires trial and error testing and does not produce immediate results. Behind every AIOps solution is a team of domain experts who extensively tune and test AI/ML models to ensure AIOps success.
Different areas of expertise
The third element for successful AIOps implementation is domain expertise. In the creation of AIOps, there isn't a lot of experience to draw from. The successful deployment of AI in any enterprise requires the involvement of experts in different fields. For example, in the field of network operations, network engineers understand the nuances of ML systems and the necessary AI algorithms to accurately solve specific problems. At the same time, non-technical experts bring industry-specific knowledge such as the provenance and availability of data sets, business strategies and operations. A large number of domain experts ensure that AI/ML algorithms reflect real-world operations, provide critical verification of results, and serve as an important tool to check for faulty methods or unintended consequences. For example, a communications system undergoing planned maintenance may exhibit behavior that typically indicates a problem state (such as extremely low network traffic). Adding a business logic layer to the model predictions that communicates with the maintenance ticketing system can eliminate these false alarms.
Domain experts play an important role in explaining to an audience of executives hungry for AIOps solutions. ML tends to operate in a black box, leaving teams unable to clearly illustrate how the model made a specific decision. This can lead to skepticism and hesitancy among business executives about AI-driven insights and actions. On the other hand, explainable artificial intelligence can gain stronger recognition and trust from business leaders who are unfamiliar with AIOps.
AIOps requires three core ingredients, but, like any recipe, the quality of those ingredients, and whose hands they are placed in, will determine the final outcome. Trial and error is part of the innovation process, especially in the complex art of training ML. Ensuring that data is handled correctly, using the right types of analytics and engaging domain experts will help enterprises deliver successful, scalable AIOps solutions that meet the growing demands for operational efficiency.
The above is the detailed content of Three elements for successfully building and deploying AIOps. For more information, please follow other related articles on the PHP Chinese website!
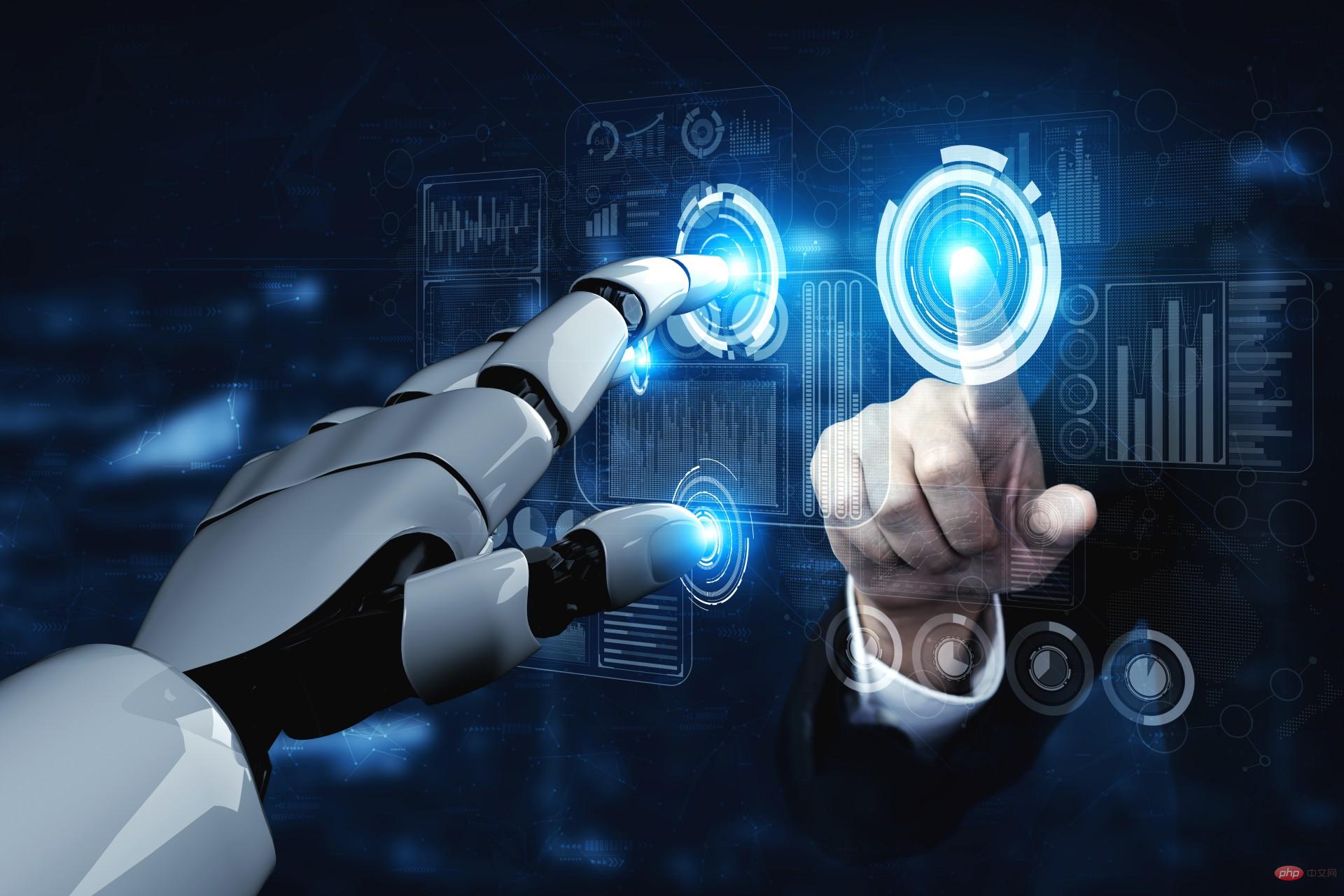
机器学习是一个不断发展的学科,一直在创造新的想法和技术。本文罗列了2023年机器学习的十大概念和技术。 本文罗列了2023年机器学习的十大概念和技术。2023年机器学习的十大概念和技术是一个教计算机从数据中学习的过程,无需明确的编程。机器学习是一个不断发展的学科,一直在创造新的想法和技术。为了保持领先,数据科学家应该关注其中一些网站,以跟上最新的发展。这将有助于了解机器学习中的技术如何在实践中使用,并为自己的业务或工作领域中的可能应用提供想法。2023年机器学习的十大概念和技术:1. 深度神经网
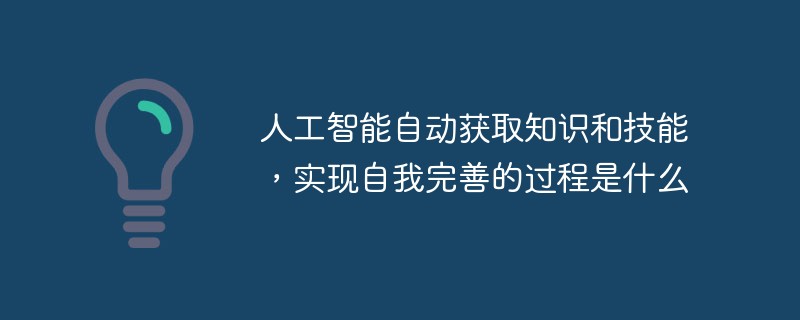
实现自我完善的过程是“机器学习”。机器学习是人工智能核心,是使计算机具有智能的根本途径;它使计算机能模拟人的学习行为,自动地通过学习来获取知识和技能,不断改善性能,实现自我完善。机器学习主要研究三方面问题:1、学习机理,人类获取知识、技能和抽象概念的天赋能力;2、学习方法,对生物学习机理进行简化的基础上,用计算的方法进行再现;3、学习系统,能够在一定程度上实现机器学习的系统。

本文将详细介绍用来提高机器学习效果的最常见的超参数优化方法。 译者 | 朱先忠审校 | 孙淑娟简介通常,在尝试改进机器学习模型时,人们首先想到的解决方案是添加更多的训练数据。额外的数据通常是有帮助(在某些情况下除外)的,但生成高质量的数据可能非常昂贵。通过使用现有数据获得最佳模型性能,超参数优化可以节省我们的时间和资源。顾名思义,超参数优化是为机器学习模型确定最佳超参数组合以满足优化函数(即,给定研究中的数据集,最大化模型的性能)的过程。换句话说,每个模型都会提供多个有关选项的调整“按钮
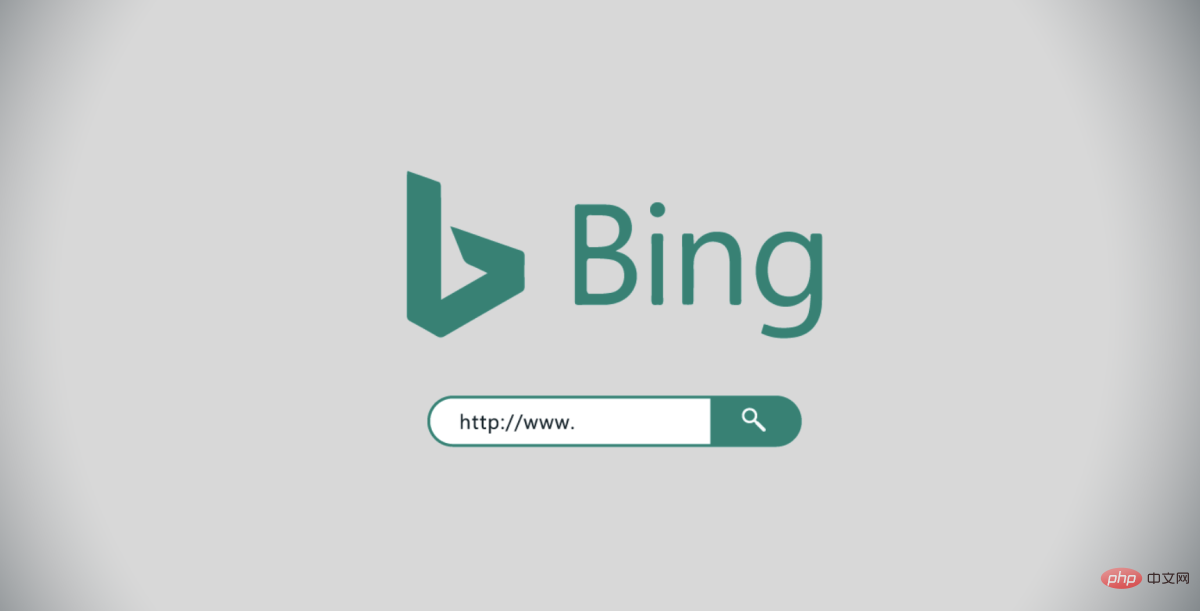
截至3月20日的数据显示,自微软2月7日推出其人工智能版本以来,必应搜索引擎的页面访问量增加了15.8%,而Alphabet旗下的谷歌搜索引擎则下降了近1%。 3月23日消息,外媒报道称,分析公司Similarweb的数据显示,在整合了OpenAI的技术后,微软旗下的必应在页面访问量方面实现了更多的增长。截至3月20日的数据显示,自微软2月7日推出其人工智能版本以来,必应搜索引擎的页面访问量增加了15.8%,而Alphabet旗下的谷歌搜索引擎则下降了近1%。这些数据是微软在与谷歌争夺生
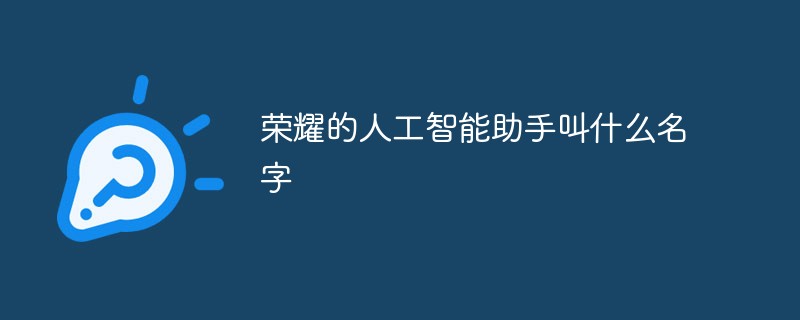
荣耀的人工智能助手叫“YOYO”,也即悠悠;YOYO除了能够实现语音操控等基本功能之外,还拥有智慧视觉、智慧识屏、情景智能、智慧搜索等功能,可以在系统设置页面中的智慧助手里进行相关的设置。
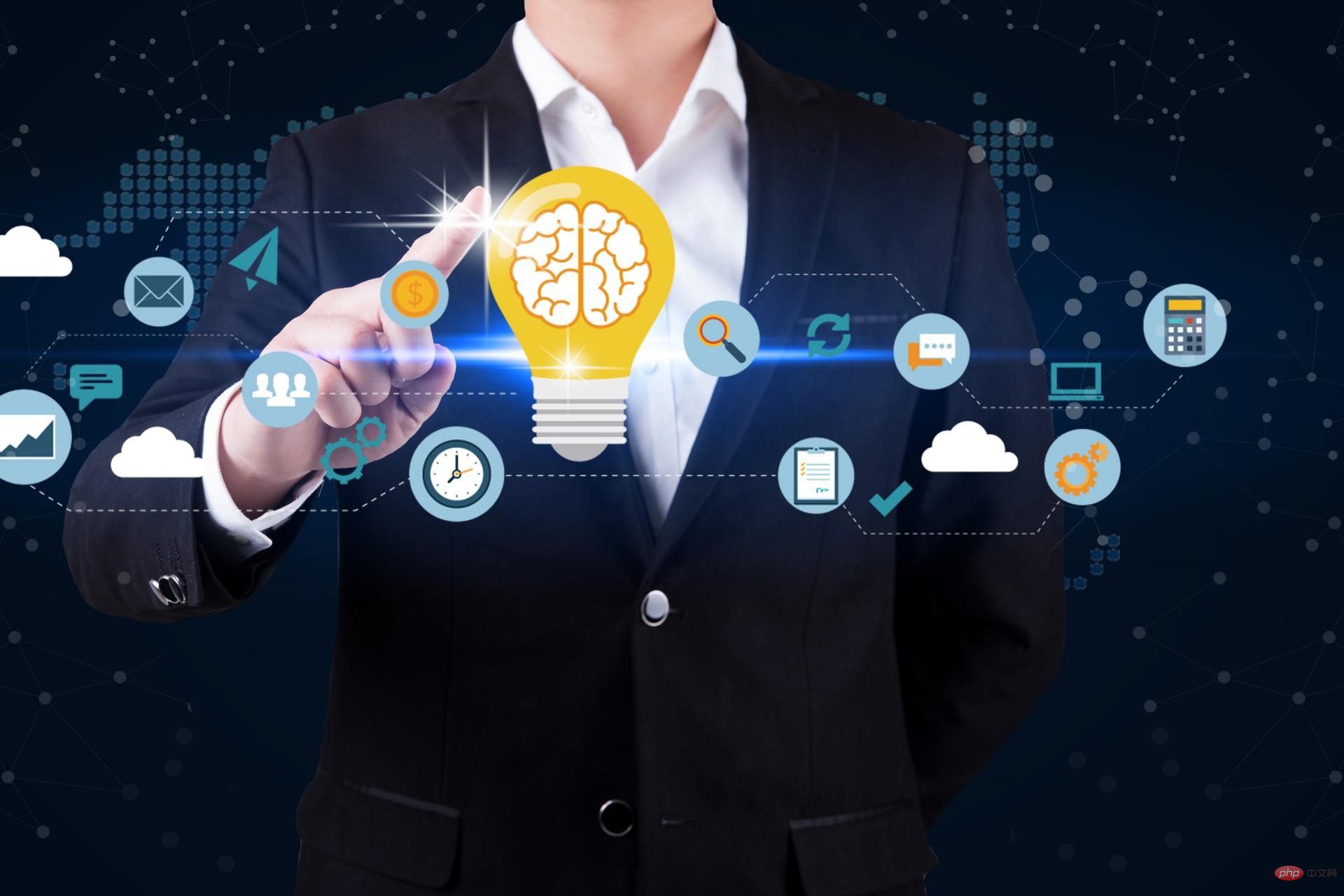
阅读论文可以说是我们的日常工作之一,论文的数量太多,我们如何快速阅读归纳呢?自从ChatGPT出现以后,有很多阅读论文的服务可以使用。其实使用ChatGPT API非常简单,我们只用30行python代码就可以在本地搭建一个自己的应用。 阅读论文可以说是我们的日常工作之一,论文的数量太多,我们如何快速阅读归纳呢?自从ChatGPT出现以后,有很多阅读论文的服务可以使用。其实使用ChatGPT API非常简单,我们只用30行python代码就可以在本地搭建一个自己的应用。使用 Python 和 C
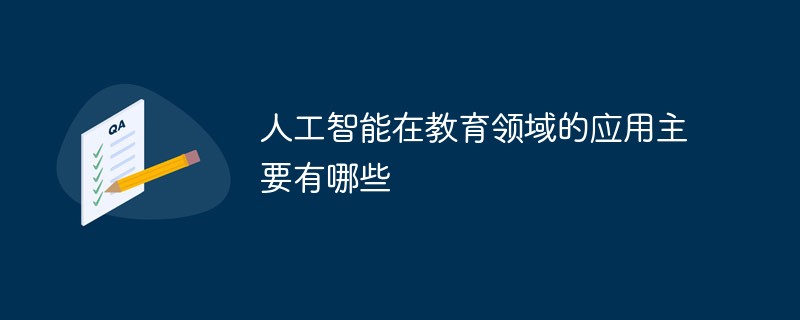
人工智能在教育领域的应用主要有个性化学习、虚拟导师、教育机器人和场景式教育。人工智能在教育领域的应用目前还处于早期探索阶段,但是潜力却是巨大的。
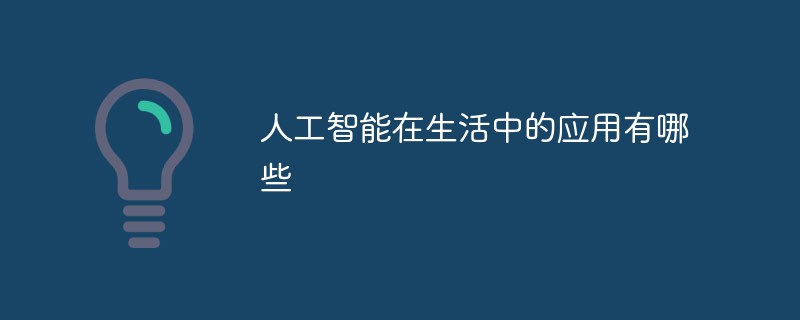
人工智能在生活中的应用有:1、虚拟个人助理,使用者可通过声控、文字输入的方式,来完成一些日常生活的小事;2、语音评测,利用云计算技术,将自动口语评测服务放在云端,并开放API接口供客户远程使用;3、无人汽车,主要依靠车内的以计算机系统为主的智能驾驶仪来实现无人驾驶的目标;4、天气预测,通过手机GPRS系统,定位到用户所处的位置,在利用算法,对覆盖全国的雷达图进行数据分析并预测。


Hot AI Tools

Undresser.AI Undress
AI-powered app for creating realistic nude photos

AI Clothes Remover
Online AI tool for removing clothes from photos.

Undress AI Tool
Undress images for free

Clothoff.io
AI clothes remover

AI Hentai Generator
Generate AI Hentai for free.

Hot Article

Hot Tools

SublimeText3 Chinese version
Chinese version, very easy to use

MinGW - Minimalist GNU for Windows
This project is in the process of being migrated to osdn.net/projects/mingw, you can continue to follow us there. MinGW: A native Windows port of the GNU Compiler Collection (GCC), freely distributable import libraries and header files for building native Windows applications; includes extensions to the MSVC runtime to support C99 functionality. All MinGW software can run on 64-bit Windows platforms.

Zend Studio 13.0.1
Powerful PHP integrated development environment
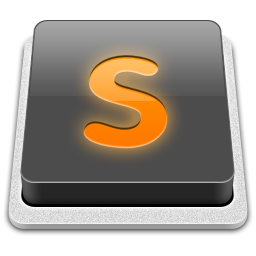
SublimeText3 Mac version
God-level code editing software (SublimeText3)
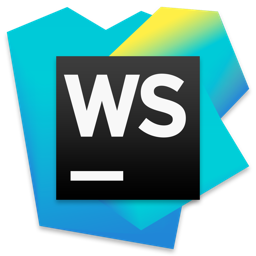
WebStorm Mac version
Useful JavaScript development tools