


As the EU moves towards implementing the Artificial Intelligence Bill, AI ethical issues such as bias, transparency and explainability are becoming increasingly important, which the Bill will effectively regulate The use of artificial intelligence and machine learning technologies across all industries. AI experts say this is a good time for AI users to familiarize themselves with ethical concepts.
Europe’s latest version of the Artificial Intelligence Act, introduced last year, is moving quickly through the review process and could be implemented as early as 2023. While the law is still being developed, the European Commission appears ready to make strides in regulating artificial intelligence.
For example, the law will set new requirements for the use of artificial intelligence systems and ban certain use cases entirely. So-called high-risk AI systems, such as those used in self-driving cars and decision support systems for education, immigration and employment, will require users to conduct impact assessments and audits of AI applications. Some AI use cases will be closely tracked in databases, while others will require sign-off from external auditors before they can be used.
Nick Carrel, director of data analytics consulting at EPAM Systems, a software engineering firm based in Newtown, Pa., said there is a strong need for unexplainability and interpretability as part of MLOps engagements or data science consulting engagements. . The EU's Artificial Intelligence Bill is also pushing companies to seek insights and answers about ethical AI, he said.
“There’s a lot of demand right now for what’s called ML Ops, which is the science of operating machine learning models. We very much see ethical AI as one of the key foundations of that process,” Carrel said. “We have additional requests from customers... as they learn about the EU legislation that is coming into force around artificial intelligence systems at the end of this year and they want to be prepared.”
Inexplicability and explainability are Separate but related concepts. A model's interpretability refers to the extent to which humans can understand and predict what decisions a model will make, while explainability refers to the ability to accurately describe how a model actually works. You can have one without the other, says Andrey Derevyanka, head of data science and machine learning at EPAM Systems.
"Imagine you are doing some experiment, maybe some chemistry experiment mixing two liquids. This experiment is open to interpretation because, you see what you are doing here. You take a item, plus another item and we get the result,” Derevyanka said. "But for this experiment to be interpretable, you need to know the chemical reaction, you need to know how the reaction is created, how it works, and you need to know the internal details of the process."
Derevyanka said, in particular Deep learning models can explain but cannot explain specific situations. "You have a black box and it works in a certain way, but you know you don't know what's inside," he said. “But you can explain: If you give this input, you get this output.”
Eliminating Bias
Bias is another important topic when it comes to ethical AI. It’s impossible to completely eliminate bias from data, but it’s important for organizations to work to eliminate bias from AI models, said Umit Cakmak, head of the data and AI practice at EPAM Systems.
“These things have to be analyzed over time,” Cakmak said. "It's a process because bias is baked into historical data. There's no way to clean bias out of the data. So as a business you have to set up some specific processes so that your decisions get better over time, which will improve the quality of your data over time, so you will be less and less biased over time."
Artificial in the European Union SMART Act would classify the use of artificial intelligence by risk It’s important to trust that AI models won’t make wrong decisions based on biased data.
Cakmak said there are many examples in the literature of data bias leaking into automated decision-making systems, including racial bias showing up in models used to evaluate employee performance or select job applicants from resumes. Being able to show how the model reaches its conclusions is important to show that steps have been taken to eliminate data bias in the model.
Cakmak recalls how a lack of explainability led a healthcare company to abandon an AI system developed for cancer diagnosis. "AI worked to some extent, but then the project was canceled because they couldn't build trust and confidence in the algorithm," he said. “If you can’t explain why the outcome is happening, then you can’t proceed with treatment.”
EPAM Systems helps companies implement artificial intelligence in a trustworthy way. The company typically follows a specific set of guidelines, starting with how to collect data, to how to prepare a machine learning model, to how to validate and interpret the model. Ensuring that AI teams successfully pass and document these checks, or "quality gates," is an important element of ethical AI, Cakmak said.
Ethics and Artificial Intelligence Act
Steven Mills, chief AI ethics officer for Global GAMMA at Boston Consulting Group, said the largest and best-run companies are already aware of the need for responsible AI.
However, as the AI Bill gets closer to becoming law, we will see more companies around the world accelerate their responsible AI projects to ensure they do not fall foul of the changing regulatory environment and new expectations.
“There are a lot of companies that have started implementing AI and are realizing that we’re not as hopeful as we’d like to be about all the potential unintended consequences and we need to address that as quickly as possible,” Mills said. “This is the most important thing. People don't feel like they're just haphazard and how they apply it."
The pressure to implement AI in an ethical way comes from the top of organizations. In some cases, it comes from outside investors who don't want their investment risk compromised by using AI in a bad way, Mills said.
"We're seeing a trend where investors, whether they're public companies or venture funds, want to make sure AI is built responsibly," he said. "It may not be obvious. It's It may not be obvious to everyone. But behind the scenes, some of these VC firms are thinking about where they are putting their money to make sure these startups are doing things the right way."
Carrel said, While the details of the Artificial Intelligence Act are also vague at the moment, the law has the potential to clarify the use of artificial intelligence, which would benefit both companies and consumers.
“My first reaction was that this was going to be very rigorous,” said Carrel, who implemented machine learning models in the financial services industry before joining EPAM Systems. "I've been trying to push the boundaries of financial services decision-making for years, and all of a sudden there's legislation coming out that would undermine the work we do.
But the more he looks at the pending law, the more he likes it See.
"I think this will also gradually increase public confidence in the use of artificial intelligence in different industries," Carrel said. "Legislation says you have to register high-risk artificial intelligence systems in the EU, which means you Know that somewhere there will be a very clear list of every AI high-risk system in use. This gives auditors a lot of power, which means naughty boys and bad players will gradually be punished, and hopefully over time we will create more opportunities for those who want to use AI and machine learning for better causes. People leave behind best practices – the responsible way. ”
The above is the detailed content of Europe's new artificial intelligence bill will strengthen ethical review. For more information, please follow other related articles on the PHP Chinese website!
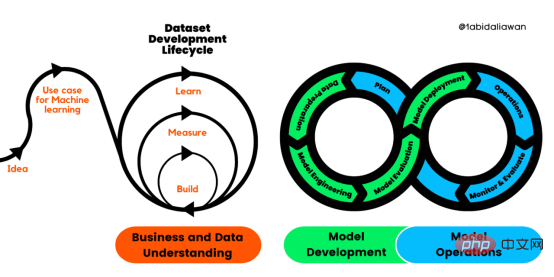
译者 | 布加迪审校 | 孙淑娟目前,没有用于构建和管理机器学习(ML)应用程序的标准实践。机器学习项目组织得不好,缺乏可重复性,而且从长远来看容易彻底失败。因此,我们需要一套流程来帮助自己在整个机器学习生命周期中保持质量、可持续性、稳健性和成本管理。图1. 机器学习开发生命周期流程使用质量保证方法开发机器学习应用程序的跨行业标准流程(CRISP-ML(Q))是CRISP-DM的升级版,以确保机器学习产品的质量。CRISP-ML(Q)有六个单独的阶段:1. 业务和数据理解2. 数据准备3. 模型
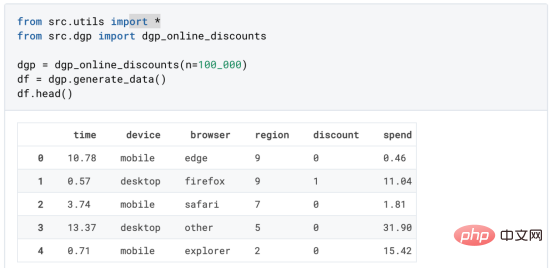
译者 | 朱先忠审校 | 孙淑娟在我之前的博客中,我们已经了解了如何使用因果树来评估政策的异质处理效应。如果你还没有阅读过,我建议你在阅读本文前先读一遍,因为我们在本文中认为你已经了解了此文中的部分与本文相关的内容。为什么是异质处理效应(HTE:heterogenous treatment effects)呢?首先,对异质处理效应的估计允许我们根据它们的预期结果(疾病、公司收入、客户满意度等)选择提供处理(药物、广告、产品等)的用户(患者、用户、客户等)。换句话说,估计HTE有助于我
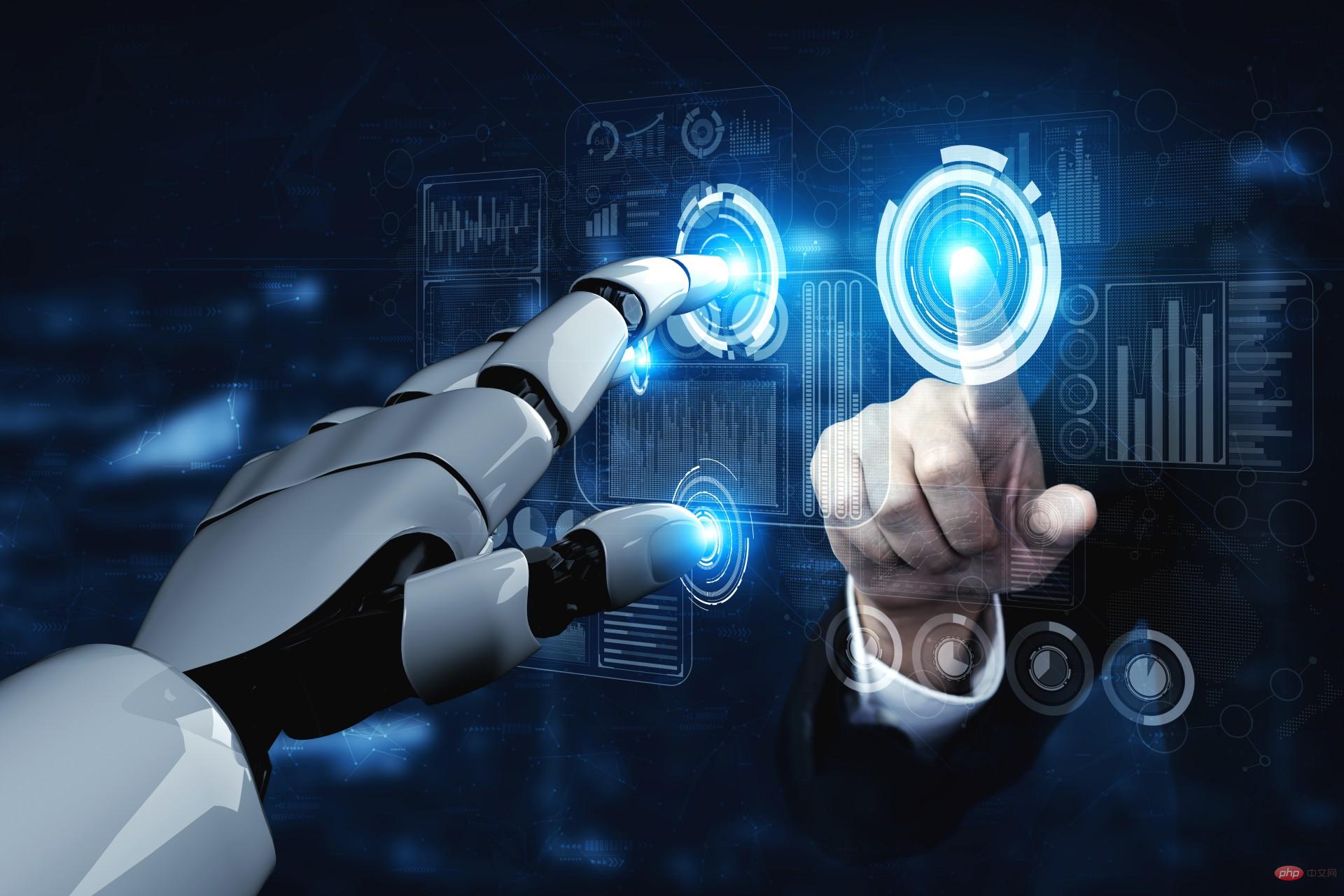
机器学习是一个不断发展的学科,一直在创造新的想法和技术。本文罗列了2023年机器学习的十大概念和技术。 本文罗列了2023年机器学习的十大概念和技术。2023年机器学习的十大概念和技术是一个教计算机从数据中学习的过程,无需明确的编程。机器学习是一个不断发展的学科,一直在创造新的想法和技术。为了保持领先,数据科学家应该关注其中一些网站,以跟上最新的发展。这将有助于了解机器学习中的技术如何在实践中使用,并为自己的业务或工作领域中的可能应用提供想法。2023年机器学习的十大概念和技术:1. 深度神经网
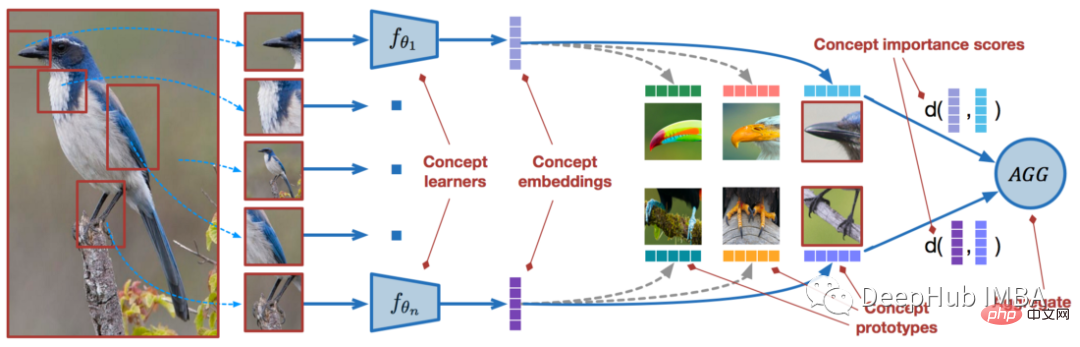
近年来,基于深度学习的模型在目标检测和图像识别等任务中表现出色。像ImageNet这样具有挑战性的图像分类数据集,包含1000种不同的对象分类,现在一些模型已经超过了人类水平上。但是这些模型依赖于监督训练流程,标记训练数据的可用性对它们有重大影响,并且模型能够检测到的类别也仅限于它们接受训练的类。由于在训练过程中没有足够的标记图像用于所有类,这些模型在现实环境中可能不太有用。并且我们希望的模型能够识别它在训练期间没有见到过的类,因为几乎不可能在所有潜在对象的图像上进行训练。我们将从几个样本中学习
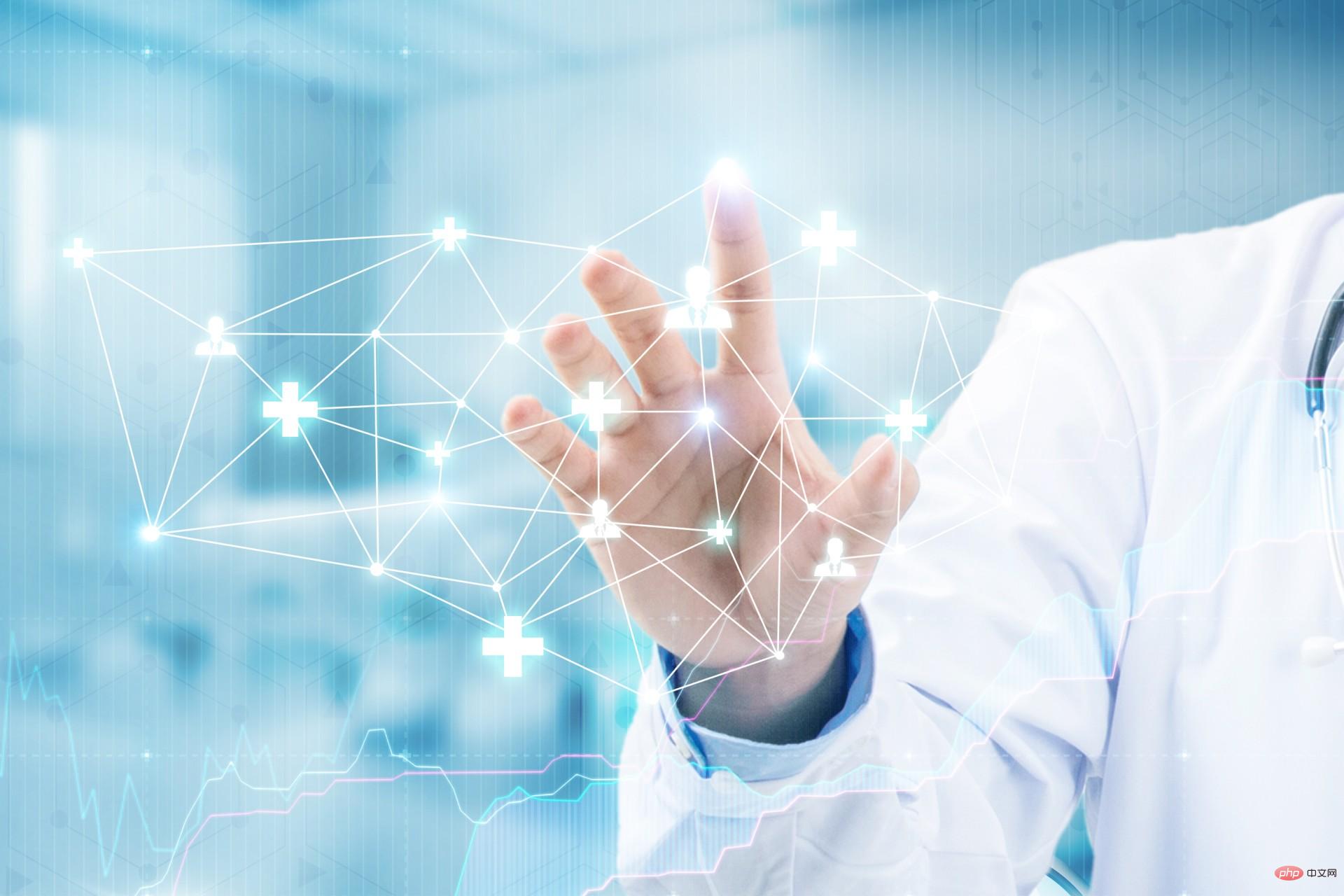
本文讨论使用LazyPredict来创建简单的ML模型。LazyPredict创建机器学习模型的特点是不需要大量的代码,同时在不修改参数的情况下进行多模型拟合,从而在众多模型中选出性能最佳的一个。 摘要本文讨论使用LazyPredict来创建简单的ML模型。LazyPredict创建机器学习模型的特点是不需要大量的代码,同时在不修改参数的情况下进行多模型拟合,从而在众多模型中选出性能最佳的一个。本文包括的内容如下:简介LazyPredict模块的安装在分类模型中实施LazyPredict
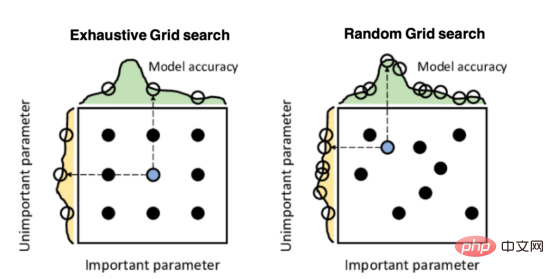
译者 | 朱先忠审校 | 孙淑娟引言模型超参数(或模型设置)的优化可能是训练机器学习算法中最重要的一步,因为它可以找到最小化模型损失函数的最佳参数。这一步对于构建不易过拟合的泛化模型也是必不可少的。优化模型超参数的最著名技术是穷举网格搜索和随机网格搜索。在第一种方法中,搜索空间被定义为跨越每个模型超参数的域的网格。通过在网格的每个点上训练模型来获得最优超参数。尽管网格搜索非常容易实现,但它在计算上变得昂贵,尤其是当要优化的变量数量很大时。另一方面,随机网格搜索是一种更快的优化方法,可以提供更好的
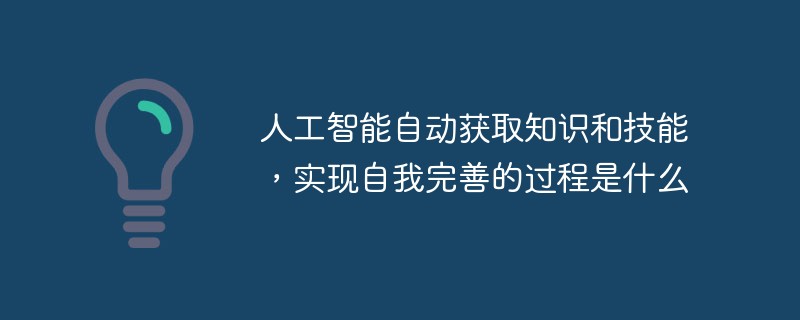
实现自我完善的过程是“机器学习”。机器学习是人工智能核心,是使计算机具有智能的根本途径;它使计算机能模拟人的学习行为,自动地通过学习来获取知识和技能,不断改善性能,实现自我完善。机器学习主要研究三方面问题:1、学习机理,人类获取知识、技能和抽象概念的天赋能力;2、学习方法,对生物学习机理进行简化的基础上,用计算的方法进行再现;3、学习系统,能够在一定程度上实现机器学习的系统。

本文将详细介绍用来提高机器学习效果的最常见的超参数优化方法。 译者 | 朱先忠审校 | 孙淑娟简介通常,在尝试改进机器学习模型时,人们首先想到的解决方案是添加更多的训练数据。额外的数据通常是有帮助(在某些情况下除外)的,但生成高质量的数据可能非常昂贵。通过使用现有数据获得最佳模型性能,超参数优化可以节省我们的时间和资源。顾名思义,超参数优化是为机器学习模型确定最佳超参数组合以满足优化函数(即,给定研究中的数据集,最大化模型的性能)的过程。换句话说,每个模型都会提供多个有关选项的调整“按钮


Hot AI Tools

Undresser.AI Undress
AI-powered app for creating realistic nude photos

AI Clothes Remover
Online AI tool for removing clothes from photos.

Undress AI Tool
Undress images for free

Clothoff.io
AI clothes remover

AI Hentai Generator
Generate AI Hentai for free.

Hot Article

Hot Tools

SAP NetWeaver Server Adapter for Eclipse
Integrate Eclipse with SAP NetWeaver application server.
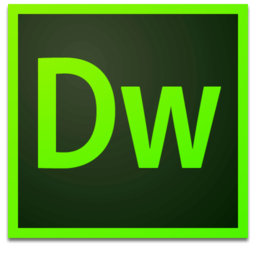
Dreamweaver Mac version
Visual web development tools
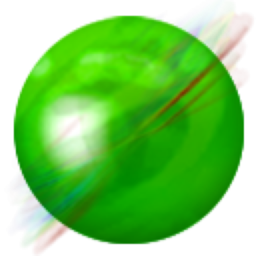
ZendStudio 13.5.1 Mac
Powerful PHP integrated development environment

Atom editor mac version download
The most popular open source editor

SublimeText3 Linux new version
SublimeText3 Linux latest version
