AI vs ML: An Overview of Artificial Intelligence and Machine Learning
Artificial intelligence and machine learning are closely related, but ultimately different.
The idea that machines could replicate or even surpass human thinking became the inspiration for advanced computing frameworks—and now, countless companies are making huge investments. At the core of this concept are artificial intelligence (AI) and machine learning (ML).
These terms are often synonyms and can be used interchangeably. In reality, artificial intelligence and machine learning represent two different things—although they are related. Essentially:
Artificial intelligence can be defined as the ability of computing systems to imitate or imitate human thinking and behavior.
Machine learning is a subset of artificial intelligence that refers to a system that can learn without being explicitly programmed or directly managed by humans.
Today, artificial intelligence and machine learning play an important role in almost every industry and business. They power business systems and consumer devices. Natural language processing, machine vision, robotics, predictive analytics, and many other digital frameworks rely on one or both of these technologies to function effectively.
A Brief History of Artificial Intelligence and Machine Learning
The idea of creating machines that can think like humans has always fascinated society as a whole. In the 1940s and 1950s, researchers and scientists, including Alan Turing, began exploring the idea of creating an "artificial brain." In 1956, a group of researchers at Dartmouth College began exploring the idea more thoroughly. At a seminar held at the school, the term "artificial intelligence" was born.
Over the next few decades, progress was made in the field. In 1964, Joseph Weizenbaum of the MIT Artificial Intelligence Laboratory invented a program called ELIZA. It demonstrates the feasibility of natural language and conversation on machines. ELIZA relies on basic pattern matching algorithms to simulate real-world conversations.
In the 1980s, with the emergence of more powerful computers, artificial intelligence research began to accelerate. In 1982, John Hopfield showed that neural networks could process information in a more advanced way. Various forms of artificial intelligence began to take shape, with the first artificial neural network (ANN) appearing in 1980.
Over the past two decades, this field has made significant progress due to tremendous advances in computing power and software. Artificial intelligence and machine learning are now widely used in various enterprise deployments. These technologies are used in natural language systems such as Siri and Alexa, self-driving cars and robots, automated decision-making systems in computer games, recommendation engines such as Netflix, and extended reality (XR) such as virtual reality (VR) and augmented reality (AR). tool.
Machine learning is especially booming. It is increasingly used by government entities, businesses, and others to identify complex and elusive patterns involving statistical and other forms of structured and unstructured data. This includes areas such as epidemiology and healthcare, financial modeling and predictive analytics, cybersecurity, chatbots and other tools for customer sales and support. In fact, many vendors offer machine learning as part of cloud computing and analytics applications.
What are the impacts of artificial intelligence?
The ability of machines to imitate human thinking and behavior profoundly changes the relationship between these two entities. Artificial intelligence unlocks large-scale automation and supports a range of more advanced digital technologies and tools, including VR, AR, digital twins, image and facial recognition, connected devices and systems, robots, personal assistants and a variety of highly interactive systems.
This includes self-driving cars that navigate the real world, smart assistants that answer questions and turn lights on and off, automated financial investment systems, and airport cameras and facial recognition. The latter include biometric boarding passes used by airlines at the gate, and Global Entry systems that simply scan your face to get through security.
In fact, companies are putting artificial intelligence to work in new and innovative ways. For example, the travel industry uses dynamic pricing models that measure supply and demand in real time and adjust flight and hotel prices based on changing conditions.
Artificial intelligence technology is used to better understand supply changing dynamics and adjust procurement models and forecasts. In warehouses, machine vision technology (powered by artificial intelligence) can detect small problems such as missing pallets and production defects that are invisible to the human eye. Chatbots, meanwhile, analyze customer input and provide contextual answers in real time.
As you can see, these capabilities are evolving rapidly—especially when connected systems are added to the mix. Smart buildings, smart transportation networks, and even smart cities are taking shape. As data flows in, the AI system determines the next best step or adjustment.
Similarly, digital twins are increasingly used by airlines, energy companies, manufacturers and other businesses to simulate actual systems and equipment and explore various virtual options. These advanced simulators can predict maintenance and failures, as well as provide insights into cheaper, more sophisticated ways of doing business.
What is the impact of machine learning?
In recent years, machine learning has also made significant progress. By using statistical algorithms, machine learning unlocks insights traditionally associated with data mining and human analysis.
It uses sample data (called training data) to identify patterns and apply them to algorithms that may change over time. Deep learning is a type of machine learning that uses artificial neural networks to simulate the way the human brain works.
The following are the main methods of using machine learning:
- Supervised learning, which requires a human to identify the required signals and outputs.
- Unsupervised learning allows systems to operate independently of humans and find valuable outputs.
- Semi-supervised learning and reinforcement learning, which involve a computer program interacting with a dynamic environment to achieve defined goals and outcomes. An example of the latter is the computer chess game. In some cases, data scientists use a hybrid approach that combines multiple elements from these methods.
Multiple Algorithms
Several types of machine learning algorithms play a key role:
- Neural Networks: Neural networks simulate the way the human brain thinks. . They are ideal for recognizing patterns and are widely used in natural language processing, image recognition, and speech recognition.
- Linear Regression: This technique is valuable for predicting numerical values, such as predicting flights or real estate prices.
- Logistic Regression: This method typically uses a binary classification model (such as "yes/no") to label or classify something. A common use of this technology is to identify spam in emails and blacklist unwanted code or malware.
- Clustering: This machine learning tool uses unsupervised learning to discover patterns that humans might miss. An example of clustering is how suppliers perform the same product in different facilities. This approach might have applications in health care, for example, to understand how different lifestyles affect health and longevity.
- Decision Tree: This method predicts numerical values but also performs classification functions. Unlike other forms of machine learning, it provides a clear way to review results. This approach is also suitable for random forests combined with decision trees.
Regardless of the exact method used, machine learning is increasingly used by businesses to better understand data and make decisions. This in turn enables more sophisticated artificial intelligence and automation. For example, sentiment analysis can plug into historical sales data, social media data, and even weather conditions to dynamically adjust production, marketing, pricing, and sales strategies. Other machine learning applications provide recommendation engines for medical diagnosis, fraud detection, and image classification.
One of the advantages of machine learning is that it can dynamically adapt as conditions and data change or as the organization adds more data. Therefore, an ML model can be built and then adjusted dynamically. For example, marketers might develop an algorithm based on customer behavior and interests, and then adjust messages and content based on changes in customer behavior, interests, or purchasing patterns.
How are artificial intelligence and machine learning developing in enterprises?
As mentioned earlier, most software vendors—covering a wide range of enterprise applications—offer AI and ML in their products. These systems make it increasingly easier to use powerful tools without extensive data science knowledge.
However, there are some things to pay attention to. For customers, understanding AI and some expertise is often necessary in order to take full advantage of AI and ML systems. When choosing a product, it's also crucial to avoid vendor hype. AI and ML cannot solve underlying business problems—in some cases, they create new challenges, concerns, and questions.
What are the ethical and legal issues?
AI and ML are at the center of a growing debate over how they should be used wisely and carefully. They have been linked to hiring and insurance bias, racial discrimination, and a variety of other issues, including misuse of data, inappropriate surveillance, and deepfakes, fake news, and information.
There is growing evidence that facial recognition systems are far less accurate at identifying people of color, which can lead to racial profiling. Additionally, there are growing concerns about the use of facial recognition by governments and other entities for mass surveillance. So far, there has been little regulation of AI practices. However, ethical AI is becoming a key consideration.
What is the future of artificial intelligence and machine learning?
Artificial intelligence technology is developing rapidly and will play an increasingly important role in businesses and people's lives. AI and ML tools can significantly reduce costs, increase productivity, facilitate automation, and drive innovation and business transformation.
As digital transformation advances, various forms of AI will become the sun surrounding various digital technologies. Artificial intelligence will lead to more advanced natural speech systems, machine vision tools, autonomous technologies, and more.
The above is the detailed content of AI vs ML: An Overview of Artificial Intelligence and Machine Learning. For more information, please follow other related articles on the PHP Chinese website!
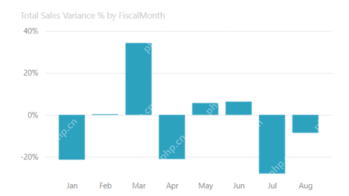
Harnessing the Power of Data Visualization with Microsoft Power BI Charts In today's data-driven world, effectively communicating complex information to non-technical audiences is crucial. Data visualization bridges this gap, transforming raw data i
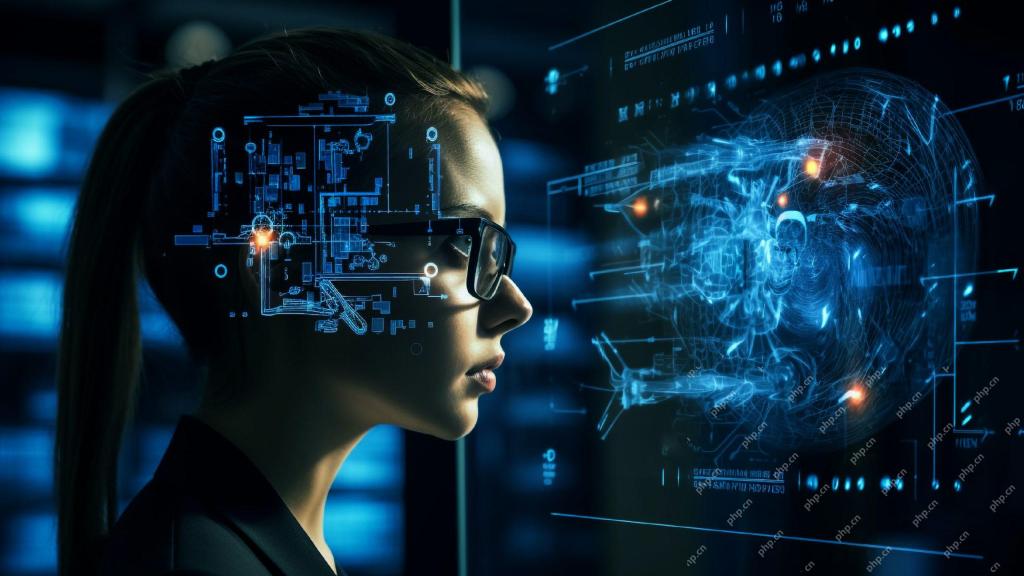
Expert Systems: A Deep Dive into AI's Decision-Making Power Imagine having access to expert advice on anything, from medical diagnoses to financial planning. That's the power of expert systems in artificial intelligence. These systems mimic the pro
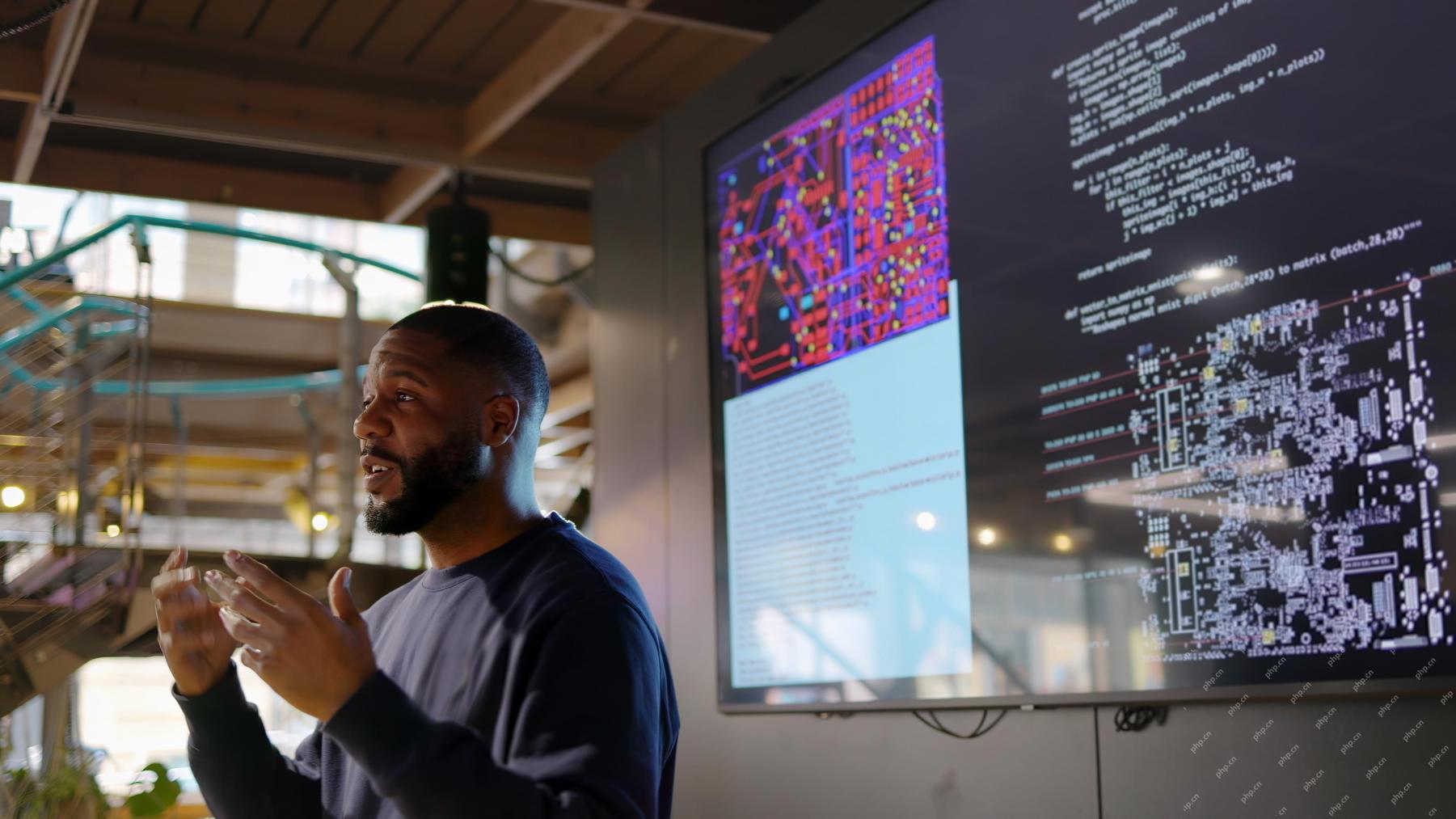
First of all, it’s apparent that this is happening quickly. Various companies are talking about the proportions of their code that are currently written by AI, and these are increasing at a rapid clip. There’s a lot of job displacement already around
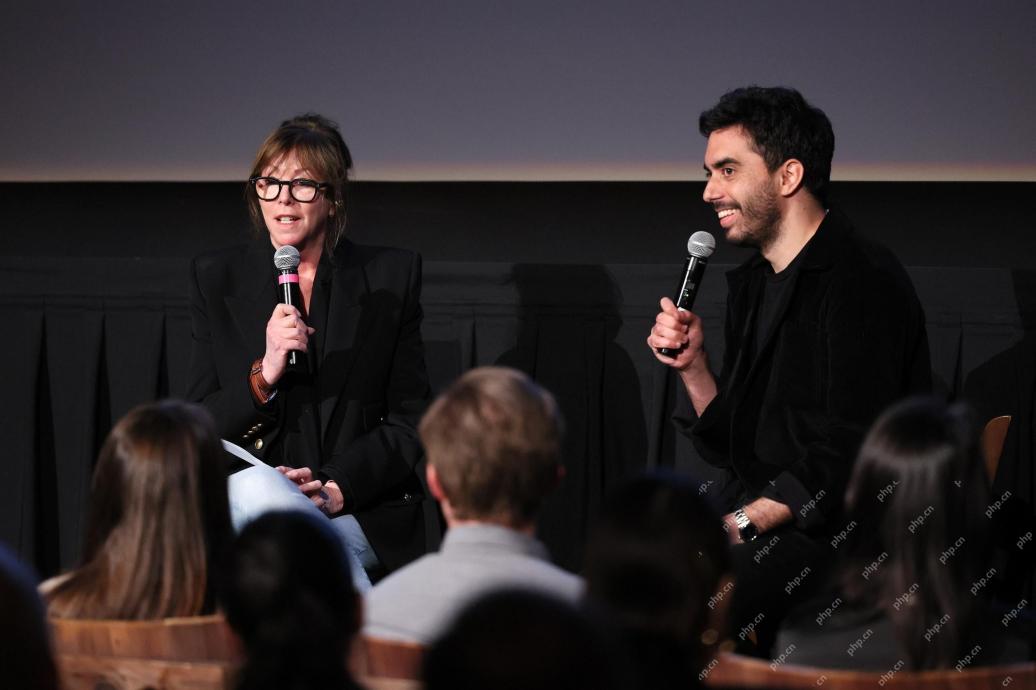
The film industry, alongside all creative sectors, from digital marketing to social media, stands at a technological crossroad. As artificial intelligence begins to reshape every aspect of visual storytelling and change the landscape of entertainment
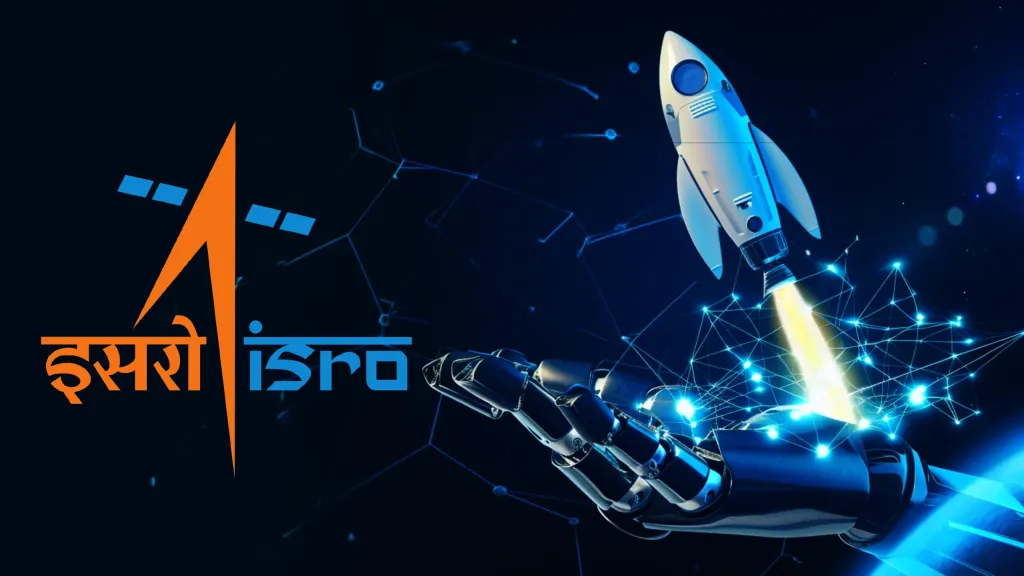
ISRO's Free AI/ML Online Course: A Gateway to Geospatial Technology Innovation The Indian Space Research Organisation (ISRO), through its Indian Institute of Remote Sensing (IIRS), is offering a fantastic opportunity for students and professionals to
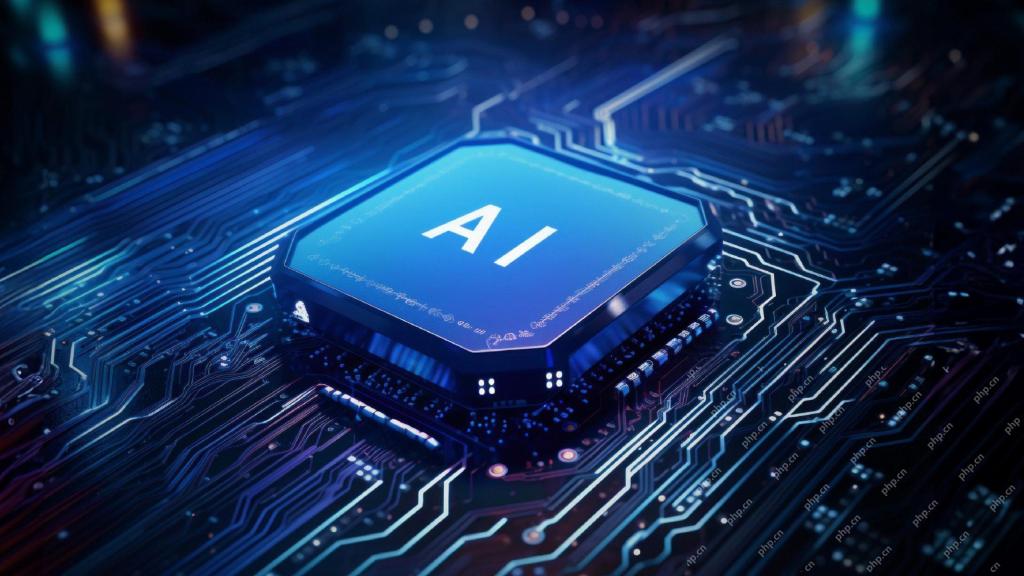
Local Search Algorithms: A Comprehensive Guide Planning a large-scale event requires efficient workload distribution. When traditional approaches fail, local search algorithms offer a powerful solution. This article explores hill climbing and simul
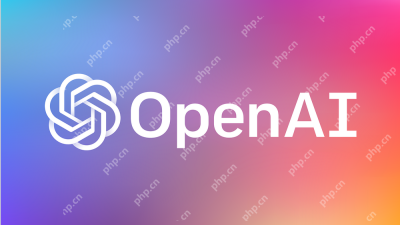
The release includes three distinct models, GPT-4.1, GPT-4.1 mini and GPT-4.1 nano, signaling a move toward task-specific optimizations within the large language model landscape. These models are not immediately replacing user-facing interfaces like
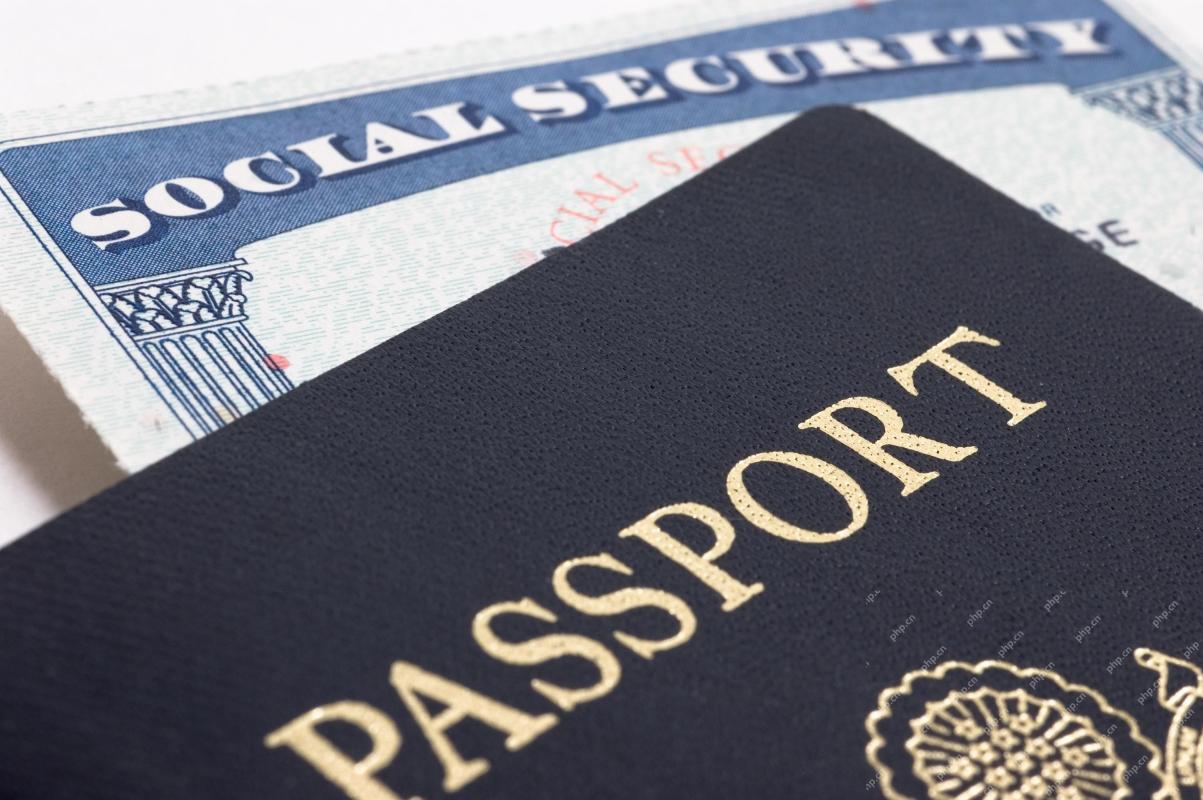
Chip giant Nvidia said on Monday it will start manufacturing AI supercomputers— machines that can process copious amounts of data and run complex algorithms— entirely within the U.S. for the first time. The announcement comes after President Trump si


Hot AI Tools

Undresser.AI Undress
AI-powered app for creating realistic nude photos

AI Clothes Remover
Online AI tool for removing clothes from photos.

Undress AI Tool
Undress images for free

Clothoff.io
AI clothes remover

AI Hentai Generator
Generate AI Hentai for free.

Hot Article

Hot Tools

Atom editor mac version download
The most popular open source editor

MinGW - Minimalist GNU for Windows
This project is in the process of being migrated to osdn.net/projects/mingw, you can continue to follow us there. MinGW: A native Windows port of the GNU Compiler Collection (GCC), freely distributable import libraries and header files for building native Windows applications; includes extensions to the MSVC runtime to support C99 functionality. All MinGW software can run on 64-bit Windows platforms.
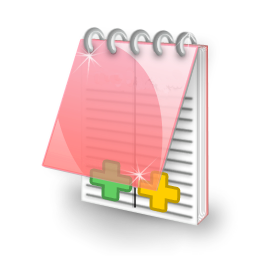
EditPlus Chinese cracked version
Small size, syntax highlighting, does not support code prompt function
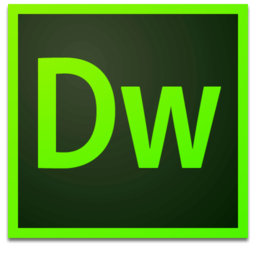
Dreamweaver Mac version
Visual web development tools

Notepad++7.3.1
Easy-to-use and free code editor