Transformer unifies voxel-based representations for 3D object detection
arXiv paper "Unifying Voxel-based Representation with Transformer for 3D Object Detection", June 22, Chinese University of Hong Kong, University of Hong Kong, Megvii Technology (in memory of Dr. Sun Jian) and Simou Technology, etc.
This paper proposes a unified multi-modal 3-D target detection framework called UVTR. This method aims to unify multi-modal representations of voxel space and enable accurate and robust single-modal or cross-modal 3-D detection. To this end, modality-specific spaces are first designed to represent different inputs to the voxel feature space. Preserve voxel space without height compression, alleviate semantic ambiguity and enable spatial interaction. Based on this unified approach, cross-modal interaction is proposed to fully utilize the inherent characteristics of different sensors, including knowledge transfer and modal fusion. In this way, geometry-aware expressions of point clouds and context-rich features in images can be well exploited, resulting in better performance and robustness.
The transformer decoder is used to efficiently sample features from a unified space with learnable locations, which facilitates object-level interactions. Generally speaking, UVTR represents an early attempt to represent different modalities in a unified framework, outperforming previous work on single-modal and multi-modal inputs, achieving leading performance on the nuScenes test set, lidar, camera and The NDS of multi-modal output are 69.7%, 55.1% and 71.1% respectively.
Code:https://github.com/dvlab-research/UVTR.
As shown in the figure:
#In the representation unification process, it can be roughly divided into the representation of input-level flow and feature-level flow. For the first approach, multimodal data are aligned at the beginning of the network. In particular, the pseudo point cloud in (a) is converted from the predicted depth-assisted image, while the range view image in (b) is projected from the point cloud. Due to depth inaccuracies in pseudo point clouds and 3-D geometric collapse in range view images, the spatial structure of the data is destroyed, leading to poor results. For feature-level methods, the typical method is to convert image features into frustum and then compress them into BEV space, as shown in Figure (c). However, due to its ray-like trajectory, the height information (height) compression at each position aggregates the features of various targets, thus introducing semantic ambiguity. At the same time, its implicit approach is difficult to support explicit feature interaction in 3-D space and limits further knowledge transfer. Therefore, a more unified representation is needed to bridge the modal gaps and facilitate multifaceted interactions.
The framework proposed in this article unifies voxel-based representation and transformer. In particular, feature representation and interaction of images and point clouds in voxel-based explicit space. For images, the voxel space is constructed by sampling features from the image plane according to the predicted depth and geometric constraints, as shown in Figure (d). For point clouds, accurate locations naturally allow features to be associated with voxels. Then, a voxel encoder is introduced for spatial interaction to establish the relationship between adjacent features. In this way, cross-modal interactions proceed naturally with features in each voxel space. For target-level interactions, a deformable transformer is used as a decoder to sample target query-specific features at each position (x, y, z) in the unified voxel space, as shown in Figure (d). At the same time, the introduction of 3-D query positions effectively alleviates the semantic ambiguity caused by height information (height) compression in the BEV space.
As shown in the figure is the UVTR architecture of multi-modal input: given a single frame or multi-frame image and point cloud, it is first processed in a single backbone and converted into modality-specific spatial VI and VP, where view transformation is used for images. In voxel encoders, features interact spatially, and knowledge transfer is easy to support during training. Depending on the settings, select single-modal or multi-modal features via the modal switch. Finally, features are sampled from the unified spatial VU with learnable locations and predicted using the transformer decoder.
The picture shows the details of the view transformation:
The picture shows the details of the knowledge migration:
The experimental results are as follows:
The above is the detailed content of Transformer unifies voxel-based representations for 3D object detection. For more information, please follow other related articles on the PHP Chinese website!
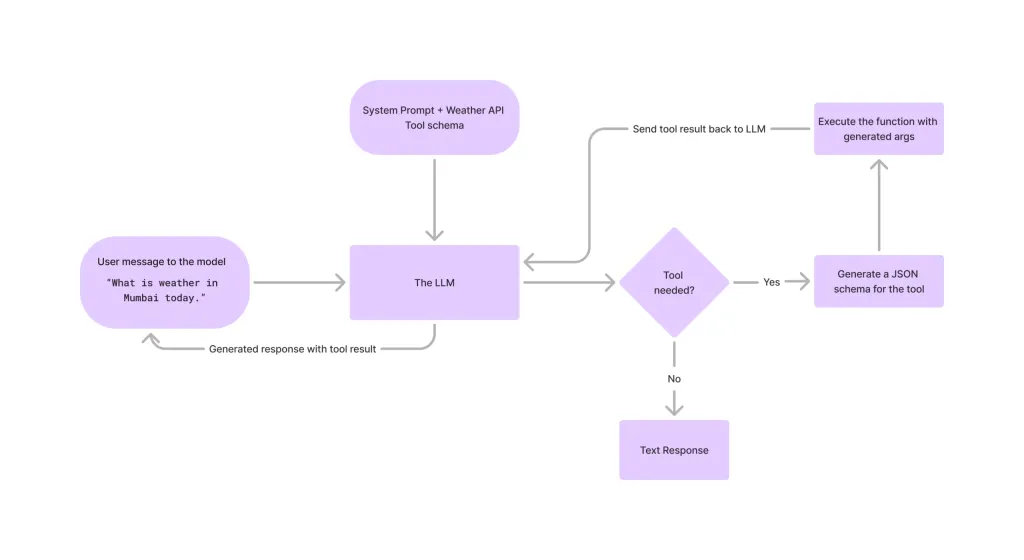
Large language models (LLMs) have surged in popularity, with the tool-calling feature dramatically expanding their capabilities beyond simple text generation. Now, LLMs can handle complex automation tasks such as dynamic UI creation and autonomous a
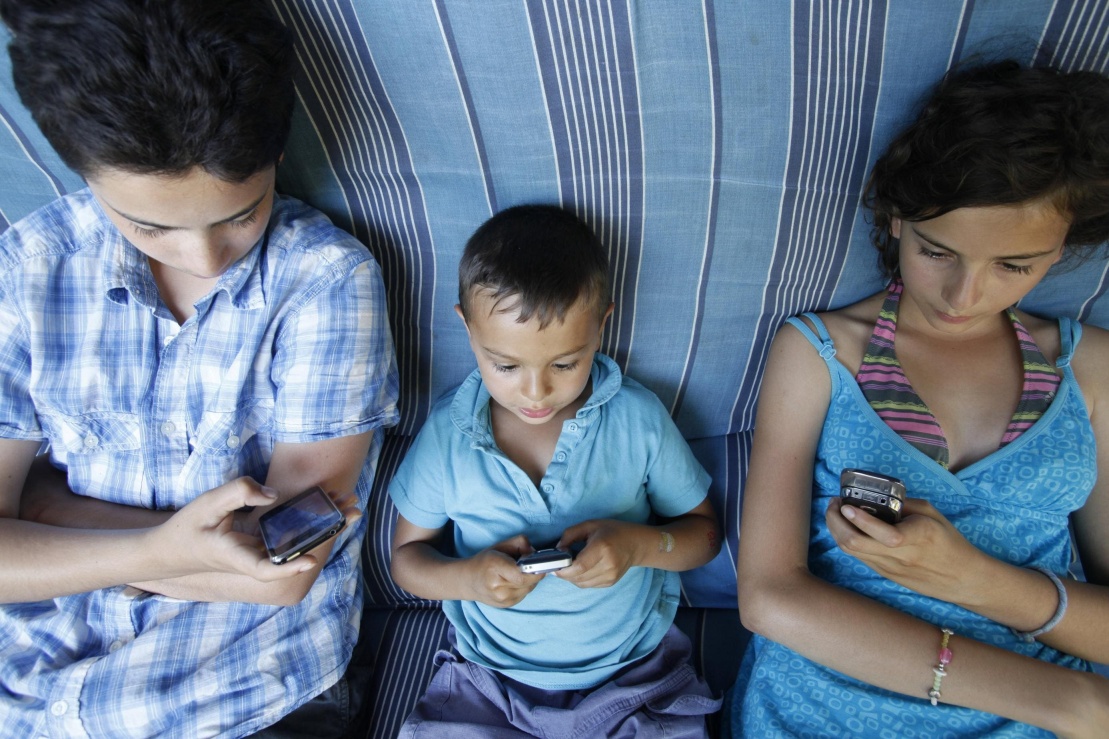
Can a video game ease anxiety, build focus, or support a child with ADHD? As healthcare challenges surge globally — especially among youth — innovators are turning to an unlikely tool: video games. Now one of the world’s largest entertainment indus
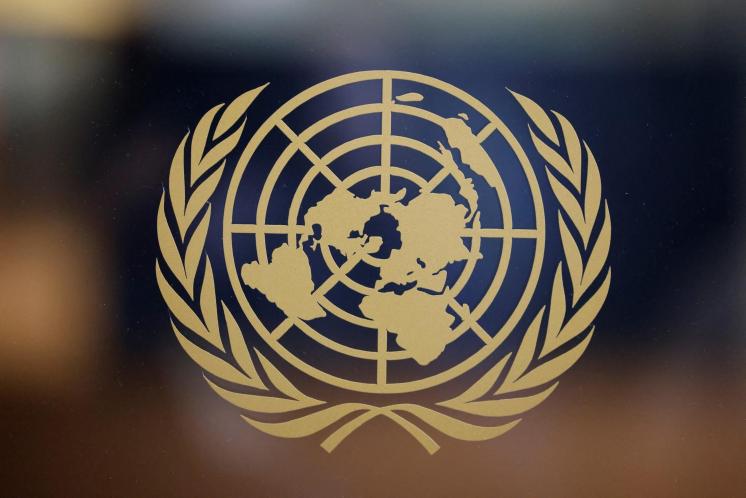
“History has shown that while technological progress drives economic growth, it does not on its own ensure equitable income distribution or promote inclusive human development,” writes Rebeca Grynspan, Secretary-General of UNCTAD, in the preamble.
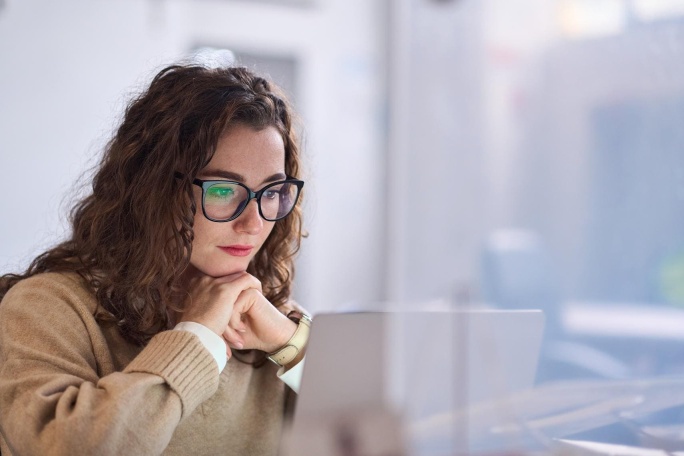
Easy-peasy, use generative AI as your negotiation tutor and sparring partner. Let’s talk about it. This analysis of an innovative AI breakthrough is part of my ongoing Forbes column coverage on the latest in AI, including identifying and explaining
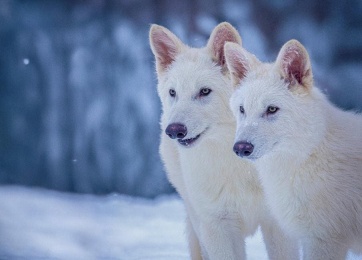
The TED2025 Conference, held in Vancouver, wrapped its 36th edition yesterday, April 11. It featured 80 speakers from more than 60 countries, including Sam Altman, Eric Schmidt, and Palmer Luckey. TED’s theme, “humanity reimagined,” was tailor made
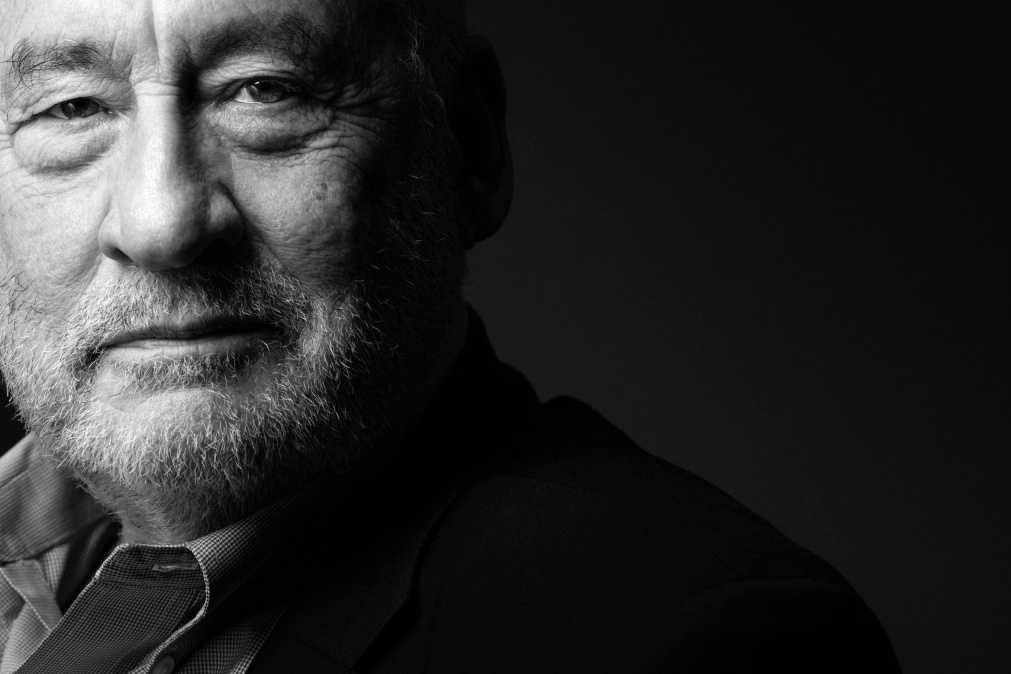
Joseph Stiglitz is renowned economist and recipient of the Nobel Prize in Economics in 2001. Stiglitz posits that AI can worsen existing inequalities and consolidated power in the hands of a few dominant corporations, ultimately undermining economic
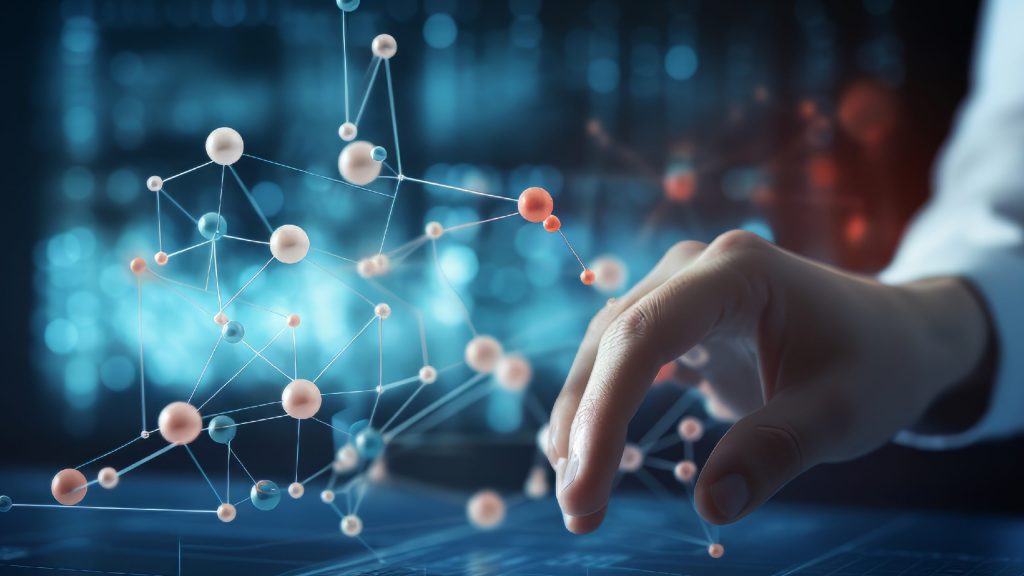
Graph Databases: Revolutionizing Data Management Through Relationships As data expands and its characteristics evolve across various fields, graph databases are emerging as transformative solutions for managing interconnected data. Unlike traditional
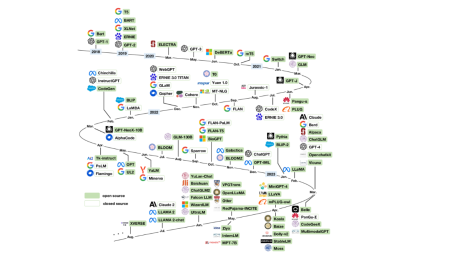
Large Language Model (LLM) Routing: Optimizing Performance Through Intelligent Task Distribution The rapidly evolving landscape of LLMs presents a diverse range of models, each with unique strengths and weaknesses. Some excel at creative content gen


Hot AI Tools

Undresser.AI Undress
AI-powered app for creating realistic nude photos

AI Clothes Remover
Online AI tool for removing clothes from photos.

Undress AI Tool
Undress images for free

Clothoff.io
AI clothes remover

AI Hentai Generator
Generate AI Hentai for free.

Hot Article

Hot Tools
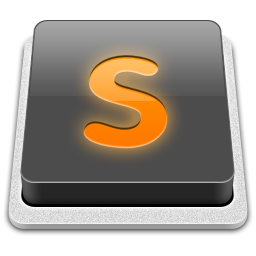
SublimeText3 Mac version
God-level code editing software (SublimeText3)

Safe Exam Browser
Safe Exam Browser is a secure browser environment for taking online exams securely. This software turns any computer into a secure workstation. It controls access to any utility and prevents students from using unauthorized resources.

MantisBT
Mantis is an easy-to-deploy web-based defect tracking tool designed to aid in product defect tracking. It requires PHP, MySQL and a web server. Check out our demo and hosting services.

SecLists
SecLists is the ultimate security tester's companion. It is a collection of various types of lists that are frequently used during security assessments, all in one place. SecLists helps make security testing more efficient and productive by conveniently providing all the lists a security tester might need. List types include usernames, passwords, URLs, fuzzing payloads, sensitive data patterns, web shells, and more. The tester can simply pull this repository onto a new test machine and he will have access to every type of list he needs.
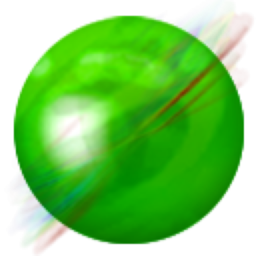
ZendStudio 13.5.1 Mac
Powerful PHP integrated development environment