Artificial intelligence (AI) is being adopted by industries to harness the power of data and use it to make smarter decisions.
This article will explain how to identify opportunities for AI in machine vision applications.
Business Requirements for Artificial Intelligence Systems
Managing Expectations
AI approaches have specific use cases. After all, it is not a universal solution and cannot solve all problems. Some applications are better suited to traditional computer vision, some may require both, and some may require only artificial intelligence. AI systems are expensive—both in terms of cost and upfront resources required. Open source tools require significant development time, and external tools are often expensive. Additionally, a GPU is often required to achieve adequate performance on the system. Many manufacturers often don't have GPUs or equivalent processing power. Therefore, it is important to determine which applications are well suited for AI with strong business needs.
The importance of visual system settings
Before entering AI, it is recommended to have a solid foundation in visual system settings. However, this is less important for AI, which can often handle worse conditions than traditional systems. All the normal machine vision system rules apply here - good lighting, camera resolution, focal length, etc. If any of these factors aren't up to scratch, it's worth going back and addressing them before delving further into AI. Ensure robust vision system setup for best results.
Reference Human Performance
AI systems are most successful where human performance is strong. Once the system is set up, operators can easily identify/classify images by eye, thus determining whether they are suitable for AI. However, if human performance is insufficient, then the AI model is likely to perform poorly. Using human performance as a reference point for what an AI model can achieve, if an operator can only identify images 70% of the time correctly, it's unlikely that the AI will perform better than that. Therefore, if human performance is not good enough for an application, that performance issue should be addressed first and improved to an acceptable level. Once operators achieve expected performance, AI can be considered.
Time and Resources
Collecting images and training the model requires considerable effort. Often, collecting high-quality images is the hardest part because many manufacturers have very low defect levels. Without data, it can be difficult to train a model for defective parts. Training tools are helpful, providing pre-trained models that require fewer samples to train. Training is an iterative process spanning multiple steps to find the ideal parameters for the model to run. Optimizing a model often requires time and experimentation. Additionally, if new data appears in the field, the model will need to be trained and deployed again.
Artificial Intelligence Application Examples:
One example application of artificial intelligence in machine vision is for final assembly inspection, another is for printed circuit boards or PCB detection.
❶ Final assembly inspection:
Background
Final inspection of parts/products or components is usually performed by operators, or traditional machine vision system, or both. Teledyne cameras will be highlighted here as an example product. The final inspection might check for bent pins, scratches on the surface, proper placement of connectors, alignment of stickers, proper printing of text, distance between mechanisms, and more. Basically, any exceptions that occur during the build process need to be found. But then the list of criteria that needs to be looked up quickly becomes very long. Traditional rule-based systems struggle to handle all corner cases, and training new operators is difficult.
Why AI?
There are often too many rules to determine what a "pass" is. This makes it difficult for traditional machine vision systems to achieve good performance. The alternative is that manual inspection is time-consuming for many companies and difficult for new operators to make some ambiguous judgments. Traditional rule-based systems often do not have adequate performance, and manufacturers rely on operator judgment to help. There may be different lighting conditions, as well as high variations in defect location, shape, and texture. Often, a simple "good/bad" qualitative output is all that's needed. However, this can also be combined with traditional rule-based algorithms if desired.
benefit
With AI, setup is much easier. After collecting a large number of images to train a model, getting a system running usually requires far less development work than a rules-based system, especially using AI tools. With a suitable system, usually using a GPU, the check is much faster, on the order of milliseconds. If provided with good data, AI systems should also perform more reliably than humans and are a good way to standardize inspection procedures. The algorithm is typically trained on data provided by multiple operators, which can reduce human error. This helps mitigate human bias or fatigue that may arise from a single operator. In this example, AI can help manufacturers reduce out-of-box failures and improve inspection quality and throughput.
❷ PCB Inspection:
Background
PCB manufacturers need to inspect their circuit boards for any defects. It may be a bad solder joint, short circuit or other abnormality. AOI (Automated Optical Inspection) machines are usually used. However, since defects vary so much, it is difficult to handle all edge cases. And the performance of rule-based systems is not accurate enough, and manufacturers will ask operators to perform manual inspections, which is time-consuming and expensive.
Why AI?
It is difficult for traditional AOI systems to identify defects. It either overshoots or underperforms, causing a defective PCB to pass or a good PCB to fail. Similar to other situations, there are too many rules to determine a "good board". Depending on the application, AI can be used here to classify defects that vary widely in size and shape, such as short circuits, opens, faulty components, welding defects, etc.
Benefits
With artificial intelligence, manufacturers can improve the accuracy and quality of inspections. This helps reduce the number of defective PCBs passing inspection. It also saves the time and labor costs of any manually assisted inspections and increases throughput by automating tasks that take operators longer to complete.
The above is the detailed content of How to identify opportunities for AI in machine vision?. For more information, please follow other related articles on the PHP Chinese website!
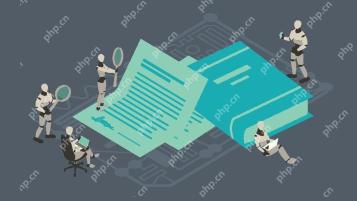
The legal tech revolution is gaining momentum, pushing legal professionals to actively embrace AI solutions. Passive resistance is no longer a viable option for those aiming to stay competitive. Why is Technology Adoption Crucial? Legal professional
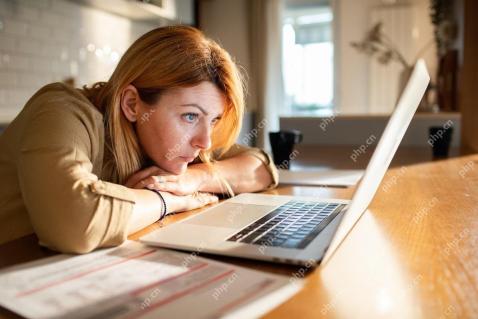
Many assume interactions with AI are anonymous, a stark contrast to human communication. However, AI actively profiles users during every chat. Every prompt, every word, is analyzed and categorized. Let's explore this critical aspect of the AI revo
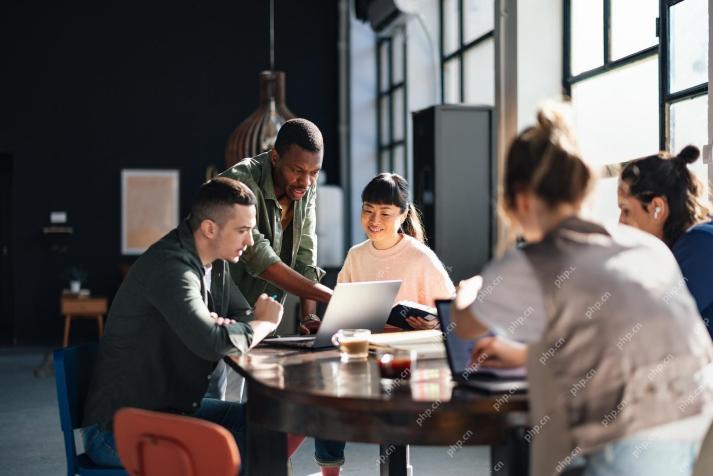
A successful artificial intelligence strategy cannot be separated from strong corporate culture support. As Peter Drucker said, business operations depend on people, and so does the success of artificial intelligence. For organizations that actively embrace artificial intelligence, building a corporate culture that adapts to AI is crucial, and it even determines the success or failure of AI strategies. West Monroe recently released a practical guide to building a thriving AI-friendly corporate culture, and here are some key points: 1. Clarify the success model of AI: First of all, we must have a clear vision of how AI can empower business. An ideal AI operation culture can achieve a natural integration of work processes between humans and AI systems. AI is good at certain tasks, while humans are good at creativity and judgment
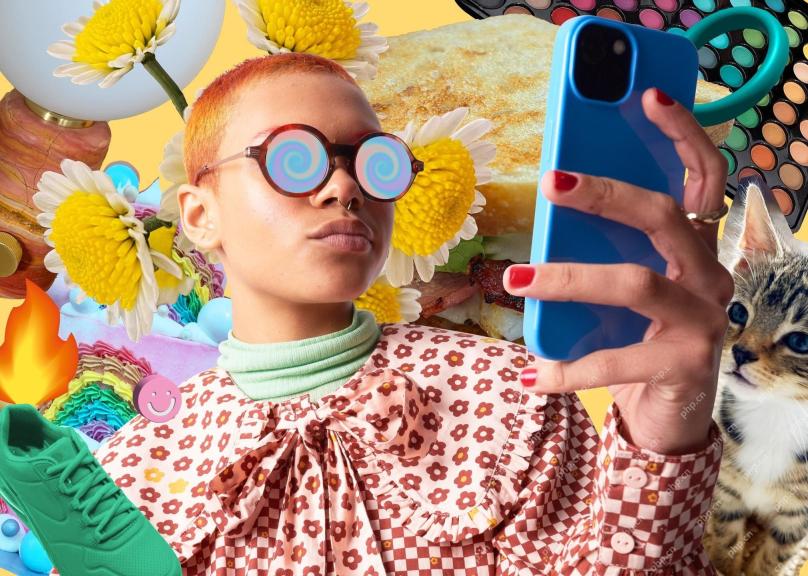
Meta upgrades AI assistant application, and the era of wearable AI is coming! The app, designed to compete with ChatGPT, offers standard AI features such as text, voice interaction, image generation and web search, but has now added geolocation capabilities for the first time. This means that Meta AI knows where you are and what you are viewing when answering your question. It uses your interests, location, profile and activity information to provide the latest situational information that was not possible before. The app also supports real-time translation, which completely changed the AI experience on Ray-Ban glasses and greatly improved its usefulness. The imposition of tariffs on foreign films is a naked exercise of power over the media and culture. If implemented, this will accelerate toward AI and virtual production
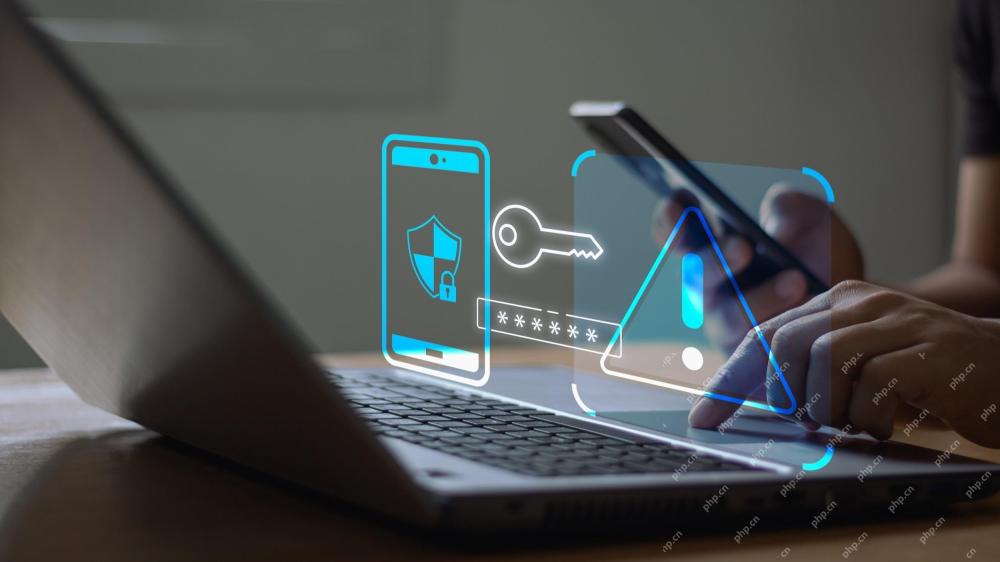
Artificial intelligence is revolutionizing the field of cybercrime, which forces us to learn new defensive skills. Cyber criminals are increasingly using powerful artificial intelligence technologies such as deep forgery and intelligent cyberattacks to fraud and destruction at an unprecedented scale. It is reported that 87% of global businesses have been targeted for AI cybercrime over the past year. So, how can we avoid becoming victims of this wave of smart crimes? Let’s explore how to identify risks and take protective measures at the individual and organizational level. How cybercriminals use artificial intelligence As technology advances, criminals are constantly looking for new ways to attack individuals, businesses and governments. The widespread use of artificial intelligence may be the latest aspect, but its potential harm is unprecedented. In particular, artificial intelligence
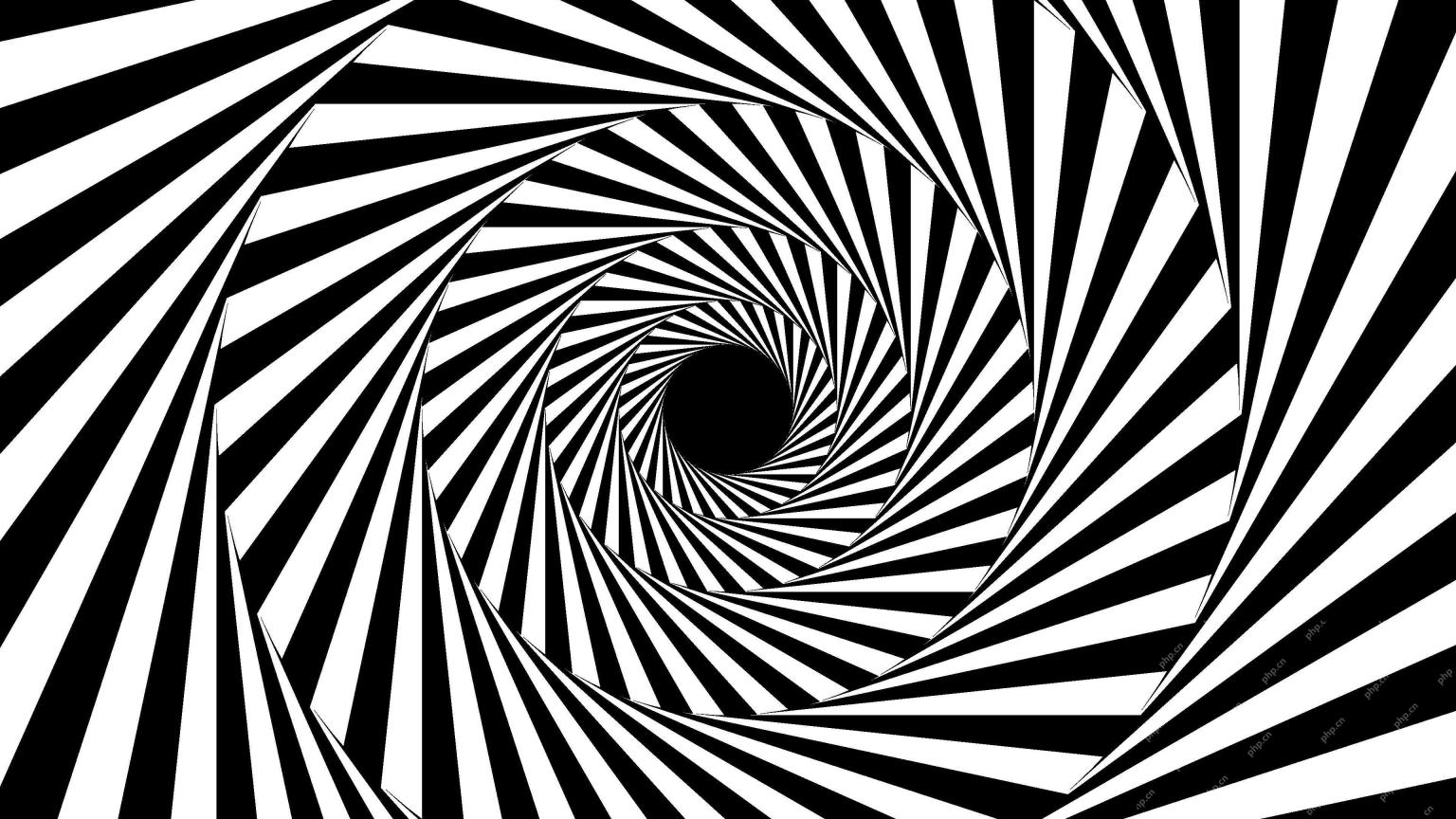
The intricate relationship between artificial intelligence (AI) and human intelligence (NI) is best understood as a feedback loop. Humans create AI, training it on data generated by human activity to enhance or replicate human capabilities. This AI
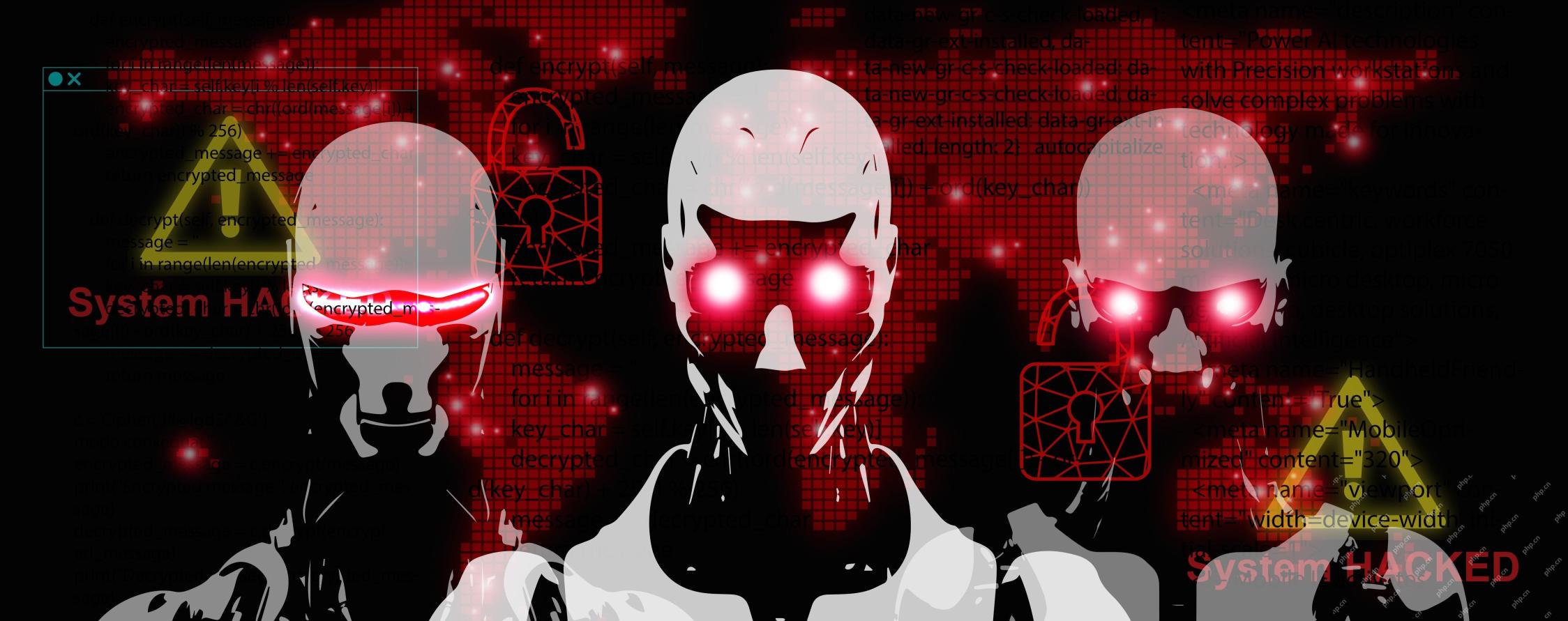
Anthropic's recent statement, highlighting the lack of understanding surrounding cutting-edge AI models, has sparked a heated debate among experts. Is this opacity a genuine technological crisis, or simply a temporary hurdle on the path to more soph
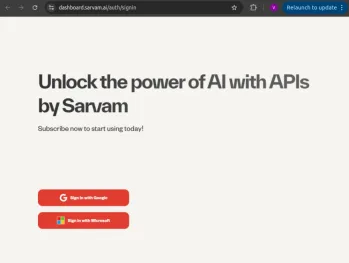
India is a diverse country with a rich tapestry of languages, making seamless communication across regions a persistent challenge. However, Sarvam’s Bulbul-V2 is helping to bridge this gap with its advanced text-to-speech (TTS) t


Hot AI Tools

Undresser.AI Undress
AI-powered app for creating realistic nude photos

AI Clothes Remover
Online AI tool for removing clothes from photos.

Undress AI Tool
Undress images for free

Clothoff.io
AI clothes remover

Video Face Swap
Swap faces in any video effortlessly with our completely free AI face swap tool!

Hot Article

Hot Tools

Safe Exam Browser
Safe Exam Browser is a secure browser environment for taking online exams securely. This software turns any computer into a secure workstation. It controls access to any utility and prevents students from using unauthorized resources.

SublimeText3 Linux new version
SublimeText3 Linux latest version

DVWA
Damn Vulnerable Web App (DVWA) is a PHP/MySQL web application that is very vulnerable. Its main goals are to be an aid for security professionals to test their skills and tools in a legal environment, to help web developers better understand the process of securing web applications, and to help teachers/students teach/learn in a classroom environment Web application security. The goal of DVWA is to practice some of the most common web vulnerabilities through a simple and straightforward interface, with varying degrees of difficulty. Please note that this software

SublimeText3 English version
Recommended: Win version, supports code prompts!
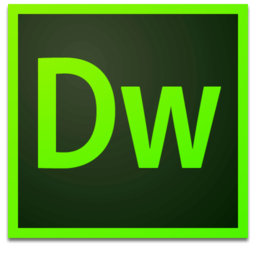
Dreamweaver Mac version
Visual web development tools
