Interpreting CRISP-ML(Q): Machine Learning Lifecycle Process
Translator | Bugatti
Reviewer | Sun Shujuan
Currently, there are no standard practices for building and managing machine learning (ML) applications. Machine learning projects are poorly organized, lack repeatability, and tend to fail outright in the long run. Therefore, we need a process to help us maintain quality, sustainability, robustness, and cost management throughout the machine learning lifecycle.
Figure 1. Machine Learning Development Lifecycle Process
Cross-industry standard process for developing machine learning applications using quality assurance methods (CRISP-ML(Q )) is an upgraded version of CRISP-DM to ensure the quality of machine learning products.
CRISP-ML (Q) has six separate phases:
1. Business and data understanding
2. Data preparation
3. Model Engineering
4. Model Evaluation
5. Model Deployment
6. Monitoring and Maintenance
These stages require continuous iteration and exploration to build better s solution. Even if there is order in the framework, the output of a later stage can determine whether we need to re-examine the previous stage.
Figure 2. Quality assurance at each stage
Quality assurance methods are introduced into each stage of the framework. This approach has requirements and constraints, such as performance metrics, data quality requirements, and robustness. It helps reduce risks that impact the success of machine learning applications. It can be achieved by continuously monitoring and maintaining the entire system.
For example: In e-commerce companies, data and concept drift will lead to model degradation; if we do not deploy a system to monitor these changes, the company will suffer losses, that is, lose customers.
Business and Data Understanding
At the beginning of the development process, we need to determine the project scope, success criteria, and feasibility of the ML application. After that, we started the data collection and quality verification process. The process is long and challenging.
Scope: What we hope to achieve by using the machine learning process. Is it to retain customers or reduce operating costs through automation?
Success Criteria: We must define clear and measurable business, machine learning (statistical indicators) and economic (KPI) success indicators.
Feasibility: We need to ensure data availability, suitability for machine learning applications, legal constraints, robustness, scalability, interpretability, and resource requirements.
Data Collection: By collecting data, versioning it for reproducibility and ensuring a continuous flow of real and generated data.
Data Quality Verification: Ensure quality by maintaining data descriptions, requirements and validations.
To ensure quality and reproducibility, we need to record the statistical properties of the data and the data generation process.
Data preparation
The second stage is very simple. We will prepare the data for the modeling phase. This includes data selection, data cleaning, feature engineering, data enhancement and normalization.
1. We start with feature selection, data selection, and handling of imbalanced classes through oversampling or undersampling.
2. Then, focus on reducing noise and handling missing values. For quality assurance purposes, we will add data unit tests to reduce erroneous values.
3. Depending on the model, we perform feature engineering and data augmentation such as one-hot encoding and clustering.
4. Normalize and extend data. This reduces the risk of biased features.
To ensure reproducibility, we created data modeling, transformation, and feature engineering pipelines.
Model Engineering
The constraints and requirements of the business and data understanding phases will determine the modeling phase. We need to understand the business problems and how we will develop machine learning models to solve them. We will focus on model selection, optimization and training, ensuring model performance metrics, robustness, scalability, interpretability, and optimizing storage and computing resources.
1. Research on model architecture and similar business problems.
2. Define model performance indicators.
3. Model selection.
4. Understand domain knowledge by integrating experts.
5. Model training.
6. Model compression and integration.
To ensure quality and reproducibility, we will store and version control model metadata, such as model architecture, training and validation data, hyperparameters, and environment descriptions.
Finally, we will track ML experiments and create ML pipelines to create repeatable training processes.
Model Evaluation
This is the stage where we test and ensure the model is ready for deployment.
- We will test the model performance on the test data set.
- Evaluate the robustness of the model by providing random or fake data.
- Enhance the interpretability of the model to meet regulatory requirements.
- Automatically or with domain experts, compare results to initial success metrics.
Every step of the evaluation phase is documented for quality assurance.
Model Deployment
Model deployment is the stage where we integrate machine learning models into existing systems. The model can be deployed on servers, browsers, software and edge devices. Predictions from the model are available in BI dashboards, APIs, web applications and plug-ins.
Model deployment process:
- Define hardware inference.
- Model evaluation in production environment.
- Ensure user acceptance and usability.
- Provide backup plans to minimize losses.
- Deployment strategy.
Monitoring and Maintenance
Models in production environments require continuous monitoring and maintenance. We will monitor model timeliness, hardware performance, and software performance.
Continuous monitoring is the first part of the process; if performance drops below a threshold, a decision is made automatically to retrain the model on new data. Furthermore, the maintenance part is not limited to model retraining. It requires decision-making mechanisms, acquiring new data, updating software and hardware, and improving ML processes based on business use cases.
In short, it is continuous integration, training and deployment of ML models.
Conclusion
Training and validating models is a small part of ML applications. Turning an initial idea into reality requires several processes. In this article we introduce CRISP-ML(Q) and how it focuses on risk assessment and quality assurance.
We first define the business goals, collect and clean data, build the model, verify the model with a test data set, and then deploy it to the production environment.
The key components of this framework are ongoing monitoring and maintenance. We will monitor data and software and hardware metrics to determine whether to retrain the model or upgrade the system.
If you are new to machine learning operations and want to learn more, read the free MLOps course reviewed by DataTalks.Club. You'll gain hands-on experience in all six phases, understanding the practical implementation of CRISP-ML.
Original title: Making Sense of CRISP-ML(Q): The Machine Learning Lifecycle Process, Author: Abid Ali Awan
The above is the detailed content of Interpreting CRISP-ML(Q): Machine Learning Lifecycle Process. For more information, please follow other related articles on the PHP Chinese website!
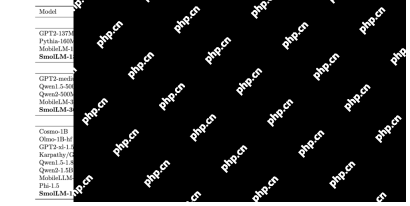
Harness the Power of On-Device AI: Building a Personal Chatbot CLI In the recent past, the concept of a personal AI assistant seemed like science fiction. Imagine Alex, a tech enthusiast, dreaming of a smart, local AI companion—one that doesn't rely
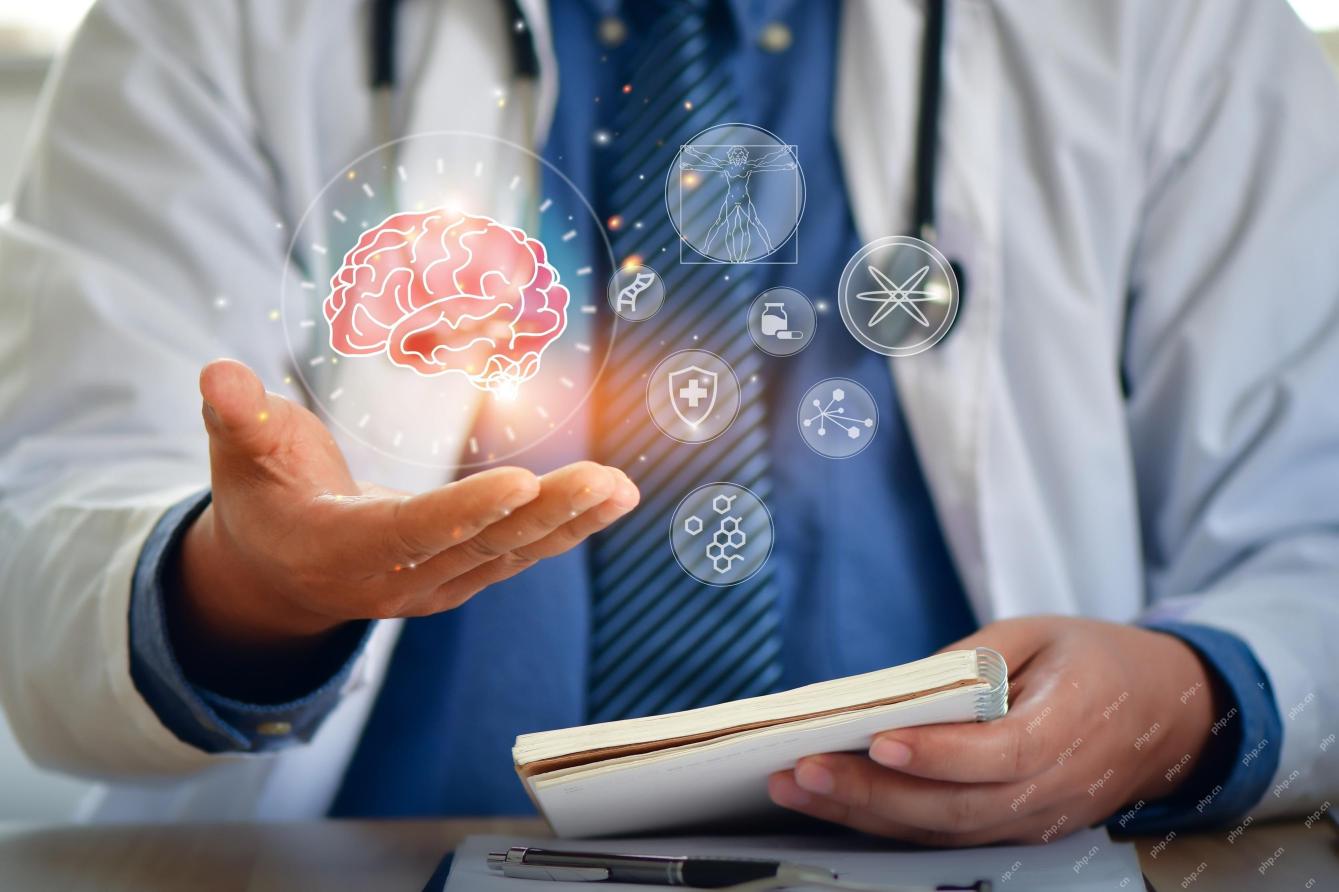
Their inaugural launch of AI4MH took place on April 15, 2025, and luminary Dr. Tom Insel, M.D., famed psychiatrist and neuroscientist, served as the kick-off speaker. Dr. Insel is renowned for his outstanding work in mental health research and techno
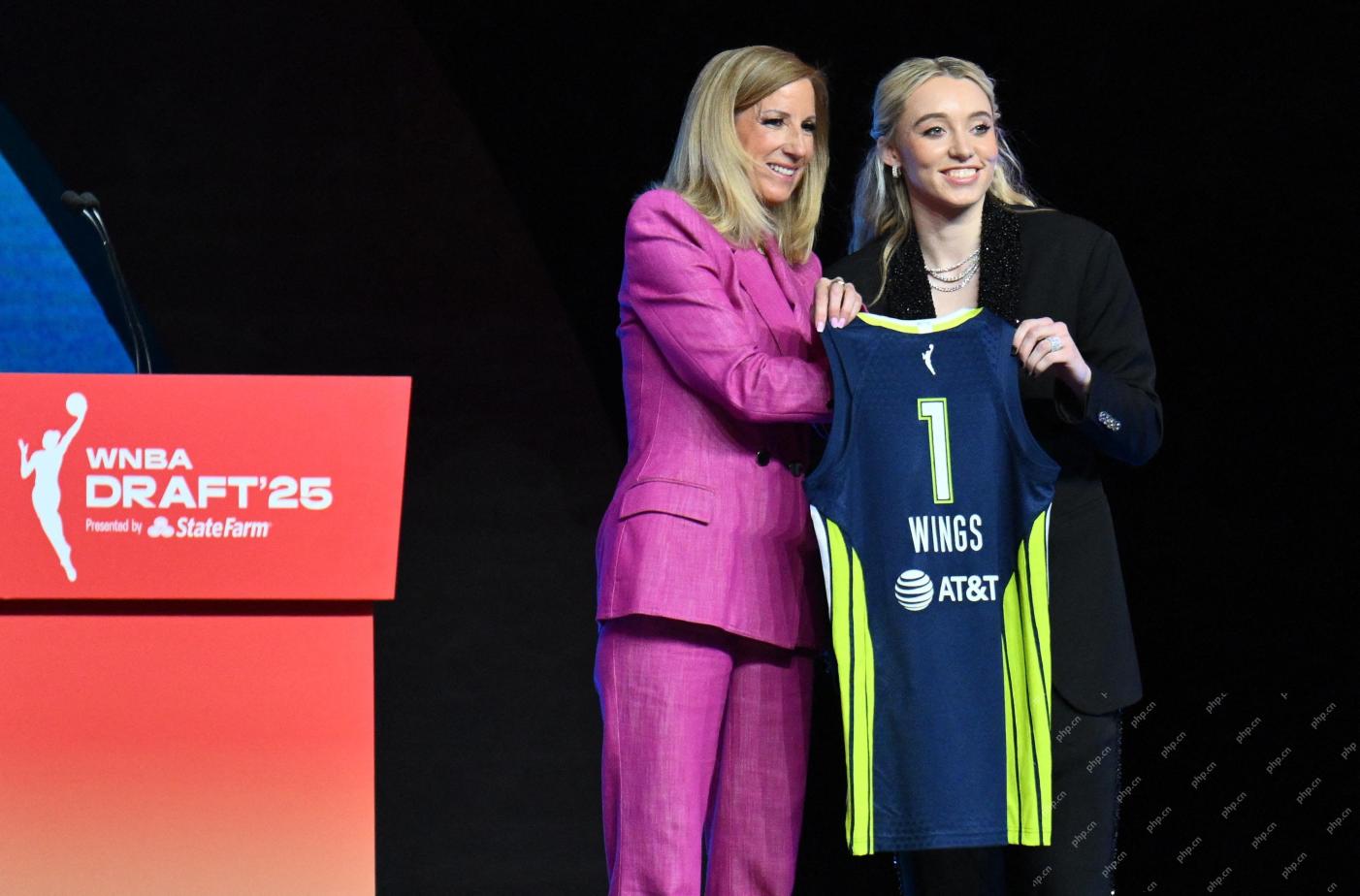
"We want to ensure that the WNBA remains a space where everyone, players, fans and corporate partners, feel safe, valued and empowered," Engelbert stated, addressing what has become one of women's sports' most damaging challenges. The anno
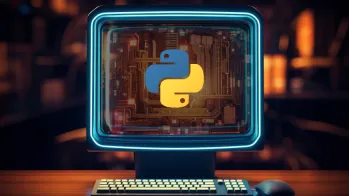
Introduction Python excels as a programming language, particularly in data science and generative AI. Efficient data manipulation (storage, management, and access) is crucial when dealing with large datasets. We've previously covered numbers and st
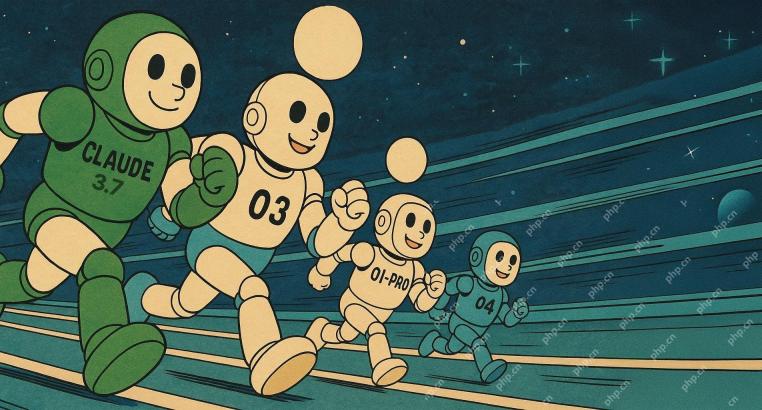
Before diving in, an important caveat: AI performance is non-deterministic and highly use-case specific. In simpler terms, Your Mileage May Vary. Don't take this (or any other) article as the final word—instead, test these models on your own scenario
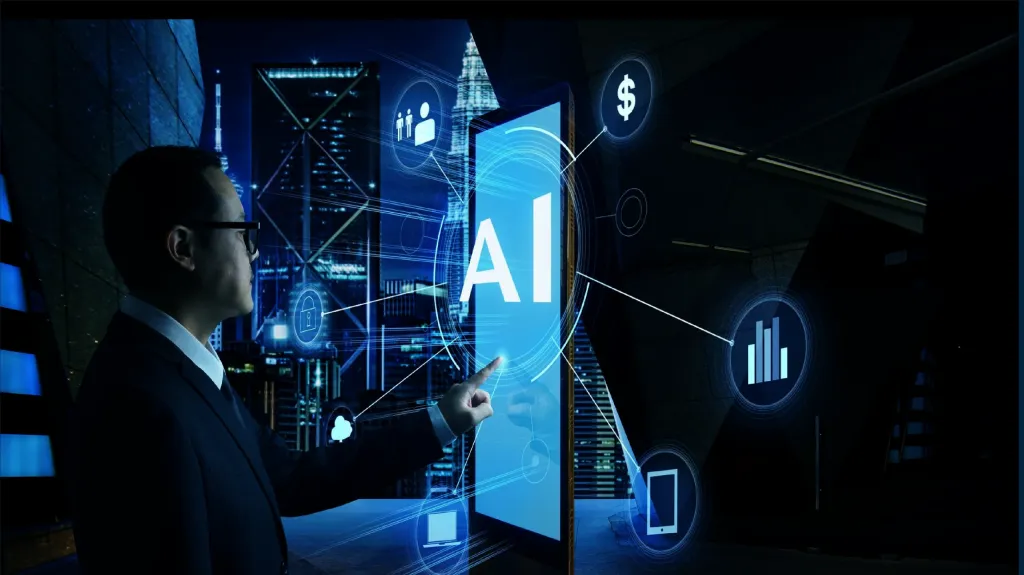
Building a Standout AI/ML Portfolio: A Guide for Beginners and Professionals Creating a compelling portfolio is crucial for securing roles in artificial intelligence (AI) and machine learning (ML). This guide provides advice for building a portfolio
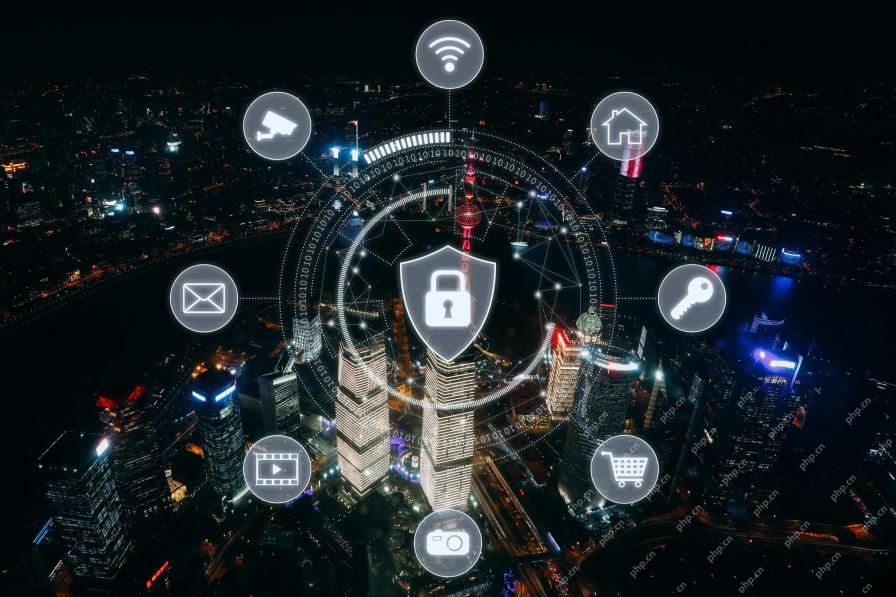
The result? Burnout, inefficiency, and a widening gap between detection and action. None of this should come as a shock to anyone who works in cybersecurity. The promise of agentic AI has emerged as a potential turning point, though. This new class
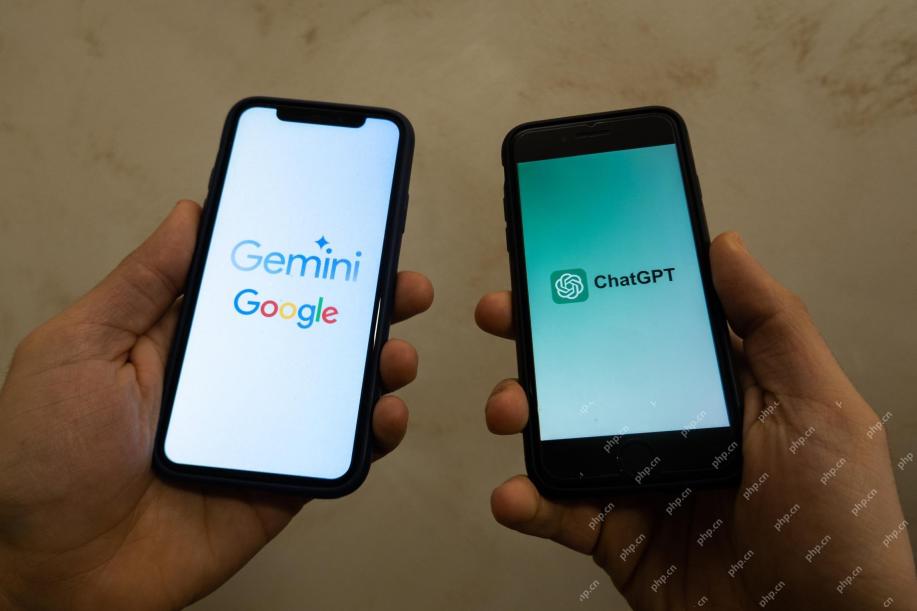
Immediate Impact versus Long-Term Partnership? Two weeks ago OpenAI stepped forward with a powerful short-term offer, granting U.S. and Canadian college students free access to ChatGPT Plus through the end of May 2025. This tool includes GPT‑4o, an a


Hot AI Tools

Undresser.AI Undress
AI-powered app for creating realistic nude photos

AI Clothes Remover
Online AI tool for removing clothes from photos.

Undress AI Tool
Undress images for free

Clothoff.io
AI clothes remover

AI Hentai Generator
Generate AI Hentai for free.

Hot Article

Hot Tools

MinGW - Minimalist GNU for Windows
This project is in the process of being migrated to osdn.net/projects/mingw, you can continue to follow us there. MinGW: A native Windows port of the GNU Compiler Collection (GCC), freely distributable import libraries and header files for building native Windows applications; includes extensions to the MSVC runtime to support C99 functionality. All MinGW software can run on 64-bit Windows platforms.

DVWA
Damn Vulnerable Web App (DVWA) is a PHP/MySQL web application that is very vulnerable. Its main goals are to be an aid for security professionals to test their skills and tools in a legal environment, to help web developers better understand the process of securing web applications, and to help teachers/students teach/learn in a classroom environment Web application security. The goal of DVWA is to practice some of the most common web vulnerabilities through a simple and straightforward interface, with varying degrees of difficulty. Please note that this software

SecLists
SecLists is the ultimate security tester's companion. It is a collection of various types of lists that are frequently used during security assessments, all in one place. SecLists helps make security testing more efficient and productive by conveniently providing all the lists a security tester might need. List types include usernames, passwords, URLs, fuzzing payloads, sensitive data patterns, web shells, and more. The tester can simply pull this repository onto a new test machine and he will have access to every type of list he needs.
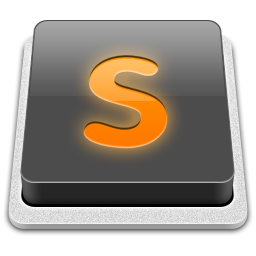
SublimeText3 Mac version
God-level code editing software (SublimeText3)

Notepad++7.3.1
Easy-to-use and free code editor