


View synthesis is a key problem at the intersection of computer vision and computer graphics. It refers to creating a new view of a scene from multiple pictures of the scene.
To accurately synthesize a new view of a scene, a model needs to capture multiple types of information from a small set of reference images, such as detailed 3D structure , materials and lighting, etc.
Since researchers proposed the Neural Radiation Field (NeRF) model in 2020, this issue has also received increasing attention, greatly promoting new views Synthetic performance.
One of the super big players is Google, which has also published many papers in the field of NeRF. This article will introduce two A paper published by Google at CVPR 2022 and ECCV 2022, describing the evolution of light field neural rendering model.
The first paper proposes a two-stage model based on Transformer to learn to combine reference pixel colors. First, the features along the epipolar lines are obtained, The features along the reference view are then obtained to generate the color of the target ray, greatly improving the accuracy of view reproduction.
Paper link: https://arxiv.org/pdf/2112.09687.pdf
ClassicLight Field RenderingCan accurately reproduce view-related effects such as reflection, refraction, and translucency, but requires dense view sampling of the scene. Methods based on geometric reconstruction only require sparse views, but cannot accurately simulate non-Lambertian effects, that is, non-ideal scattering.
The new model proposed in this article combines the advantages of these two directions and alleviates its limitations, by focusing on light By manipulating the four-dimensional representation of the field, the model can learn to accurately represent view-dependent effects. Scene geometry is implicitly learned from a sparse set of views by enforcing geometric constraints during training and inference.
The model outperforms state-of-the-art models on multiple forward and 360° datasets and has severe line-of-sight dependence There is greater leeway in scenes of sexual change.
Another paper solves the generalization problem of synthesizing unseen scenes by using Transformer sequences with canonicalized position encoding . After the model is trained on a set of scenes, it can be used to synthesize views of new scenes.
Paper link: https://arxiv.org/pdf/2207.10662.pdf
This article proposes a different paradigm that does not require depth features and NeRF-like volume rendering. This method can directly predict the color of target rays in new scenes by simply sampling a patch set from the scene.
First use epipolar geometry to extract patches along the epipolar lines of each reference view, and assign each patch Linearly projected into a one-dimensional feature vector, this set is then processed by a series of Transformers.
For position encoding, the researchers used a method similar to the light field representation methodto parameterize the rays. The difference is that the coordinates are normalized relative to the target ray, and also This makes the method independent of the reference frame and improves versatility.
The innovation of the model is that it performs image-based rendering, combining the color and characteristics of the reference image to render a new view, and it is purely It is based on Transformer and operates on image patch sets. And they utilize 4D light field representations for position encoding, helping to simulate view-related effects.
Final experimental results show that this method outperforms other methods in new view synthesis of unseen scenes, even when trained with much less data than The same is true for ##.
Light Field Neural RenderingThe input to the model includes a set of reference images, the corresponding camera parameters (focal length, position and spatial orientation), and the user's desired The coordinates of the color's target ray.
In order to generate a new image, we need to start with the camera parameters of the input image, first obtain the coordinates of the target ray (each one corresponds to a pixel), and for each coordinate Model query.
The researchers’ solution was to not fully process each reference image, but only to look at the areas that might affect the target pixels. These regions can be determined by epipolar geometry, mapping each target pixel to a line on each reference frame.
For the sake of safety, you need to select a small area around some points on the epipolar line to form a set of patches that will be actually processed by the model, and then apply the Transformer to this set of patches. Get the color of the target pixel.
Transformer is particularly useful in this case because the self-attention mechanism in it can naturally take the patch collection as input and the attention weight itself It can be used to predict the color of output pixels by combining reference view colors and features.
In light field neural rendering (LFNR), researchers use two Transformer sequences to map a collection of patches to target pixel colors.
The first Transformer aggregates information along each epipolar line, and the second Transformer aggregates information along each reference image.
This method can interpret the first Transformer as finding the potential correspondence of the target pixel on each reference frame, while the second Transformer is responsible for occlusion and line-of-sight dependence effects. reasoning, which is also a common difficulty with image-based rendering.
LFNR outperforms the sota model on the most popular view synthesis benchmarks (NeRF’s Blender and Real Forward-Facing scenes and NeX’s Shiny) The peak signal-to-noise ratio (PSNR) is improved by up to 5dB, which is equivalent to reducing the pixel-level error by 1.8 times.
LFNR can reproduce some of the more difficult line-of-sight-dependent effects in the NeX/Shiny dataset, such as rainbows and reflections on CDs, reflections, refractions and translucency on bottles.
Compared with previous methods such as NeX and NeRF, they are unable to reproduce line-of-sight-related effects, such as in the NeX/Shiny dataset Translucency and refractive index of test tubes in a laboratory scene.
But LFNR also has limitations.
The first Transformer folds information along each epipolar line independently for each reference image, which also means that the model can only decide what information to retain based on the output ray coordinates and patches of each reference image. , which works well in training on a single scene (like most neural rendering methods), but it cannot generalize to different scenes.
Generalizable models are important because they can be directly applied to new scenarios without retraining.
The researchers proposed a general patch-based neural rendering (GPNR) model to solve this shortcoming of LFNR.
By adding a Transformer to the model so that it runs before the other two Transformers and between the points of the same depth of all reference images exchange information between.
GPNR consists of a sequence of three Transformers that map a set of patches extracted along epipolar lines into pixel colors. Image patches are mapped to initial features through linear projection layers, and then these features are continuously refined and aggregated by the model to finally form features and colors.
For example, after the first Transformer extracts the patch sequence from "Park Bench", the new model can use "Flowers" that appear at corresponding depths in both views Such clues indicate a potential match.
Another key idea of this work is to normalize the position encoding according to the target ray, because we want to generalize in different scenarios, Quantities must be represented in a relative rather than an absolute frame of reference
To evaluate the model's generalization performance, the researchers trained GPNR on a set of scenarios and tested it on new scenarios .
GPNR improves by an average of 0.5-1.0 dB on several benchmarks (following IBRNet and MVSNeRF protocols), especially on the IBRNet benchmark, where GPNR improves using only 11% of the training scenarios. case, it exceeds the baseline model.
GPNR generated view details on maintained scenes in NeX/Shiny and LLFF without any fine-tuning. GPNR more accurately reproduces details on the blades and refraction through the lens than IBRNet.
The above is the detailed content of Just train once to generate new 3D scenes! The evolution history of Google's 'Light Field Neural Rendering”. For more information, please follow other related articles on the PHP Chinese website!
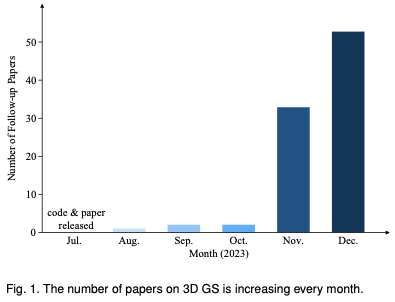
写在前面&笔者的个人理解三维Gaussiansplatting(3DGS)是近年来在显式辐射场和计算机图形学领域出现的一种变革性技术。这种创新方法的特点是使用了数百万个3D高斯,这与神经辐射场(NeRF)方法有很大的不同,后者主要使用隐式的基于坐标的模型将空间坐标映射到像素值。3DGS凭借其明确的场景表示和可微分的渲染算法,不仅保证了实时渲染能力,而且引入了前所未有的控制和场景编辑水平。这将3DGS定位为下一代3D重建和表示的潜在游戏规则改变者。为此我们首次系统地概述了3DGS领域的最新发展和关
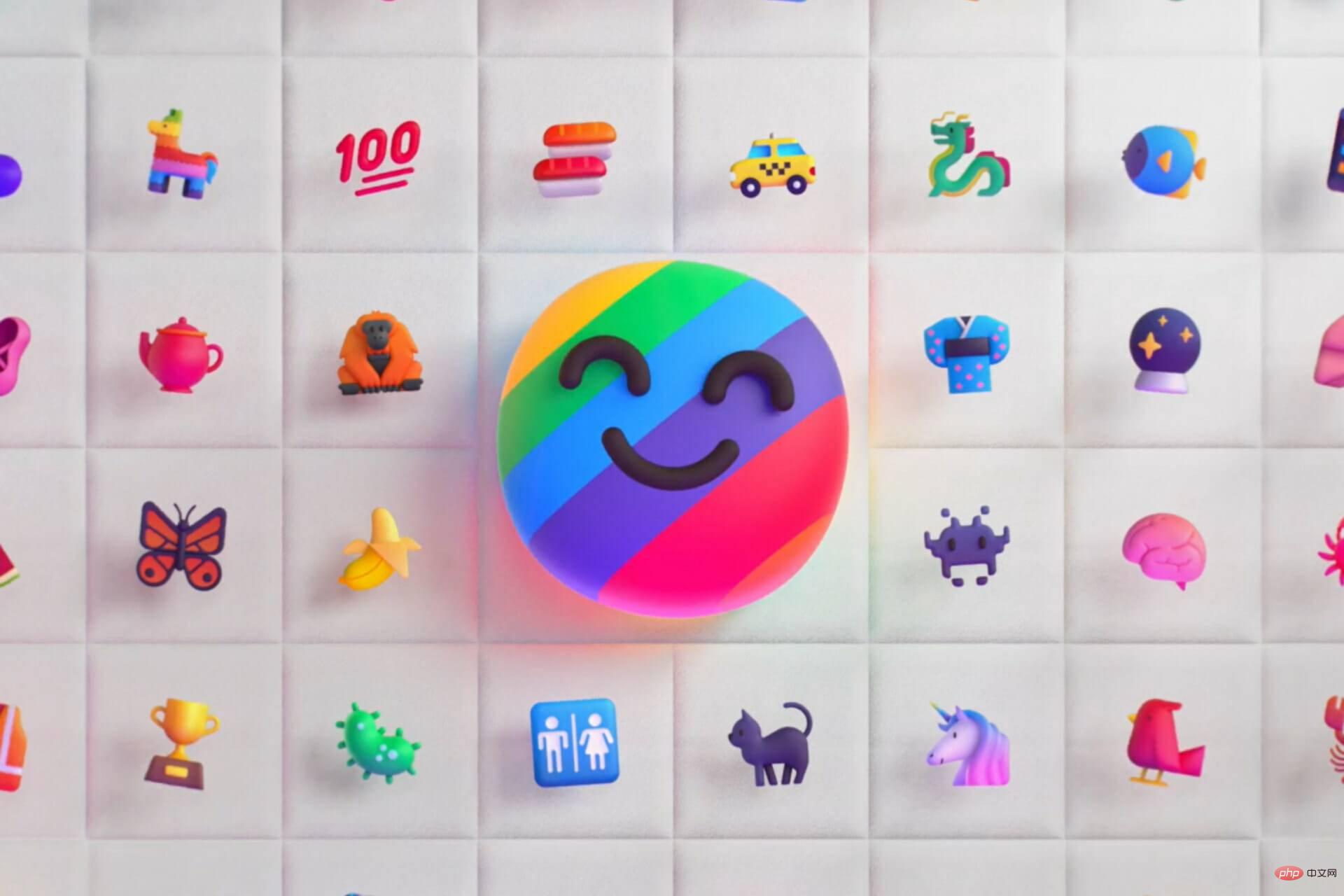
您一定记得,尤其是如果您是Teams用户,Microsoft在其以工作为重点的视频会议应用程序中添加了一批新的3DFluent表情符号。在微软去年宣布为Teams和Windows提供3D表情符号之后,该过程实际上已经为该平台更新了1800多个现有表情符号。这个宏伟的想法和为Teams推出的3DFluent表情符号更新首先是通过官方博客文章进行宣传的。最新的Teams更新为应用程序带来了FluentEmojis微软表示,更新后的1800表情符号将为我们每天
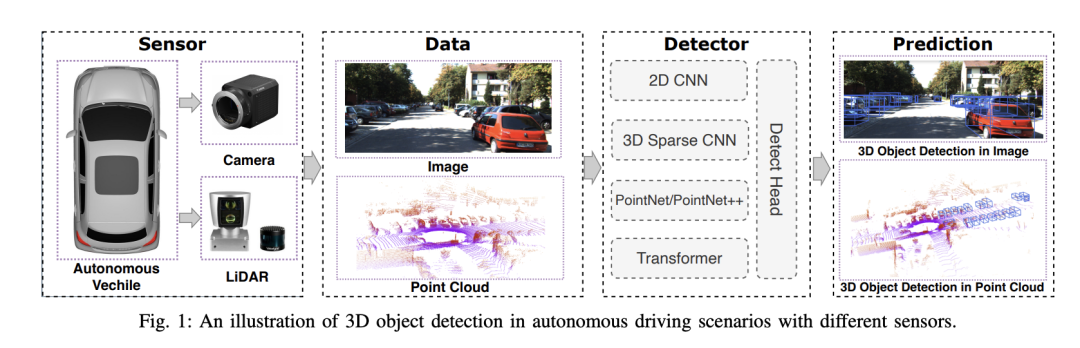
0.写在前面&&个人理解自动驾驶系统依赖于先进的感知、决策和控制技术,通过使用各种传感器(如相机、激光雷达、雷达等)来感知周围环境,并利用算法和模型进行实时分析和决策。这使得车辆能够识别道路标志、检测和跟踪其他车辆、预测行人行为等,从而安全地操作和适应复杂的交通环境.这项技术目前引起了广泛的关注,并认为是未来交通领域的重要发展领域之一。但是,让自动驾驶变得困难的是弄清楚如何让汽车了解周围发生的事情。这需要自动驾驶系统中的三维物体检测算法可以准确地感知和描述周围环境中的物体,包括它们的位置、
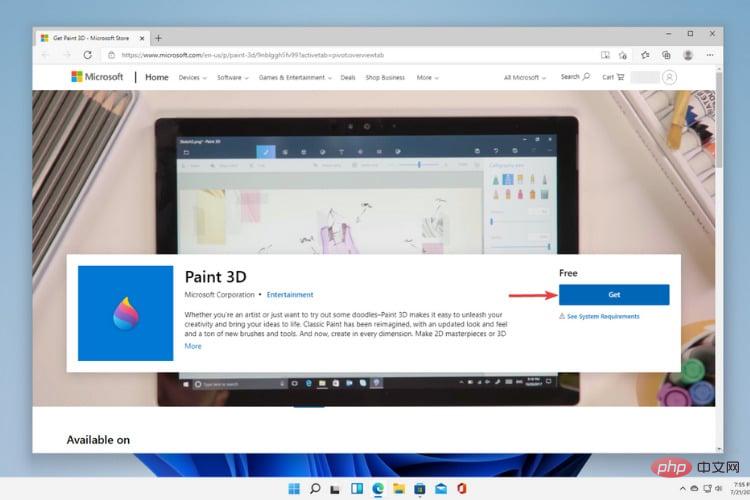
当八卦开始传播新的Windows11正在开发中时,每个微软用户都对新操作系统的外观以及它将带来什么感到好奇。经过猜测,Windows11就在这里。操作系统带有新的设计和功能更改。除了一些添加之外,它还带有功能弃用和删除。Windows11中不存在的功能之一是Paint3D。虽然它仍然提供经典的Paint,它对抽屉,涂鸦者和涂鸦者有好处,但它放弃了Paint3D,它提供了额外的功能,非常适合3D创作者。如果您正在寻找一些额外的功能,我们建议AutodeskMaya作为最好的3D设计软件。如
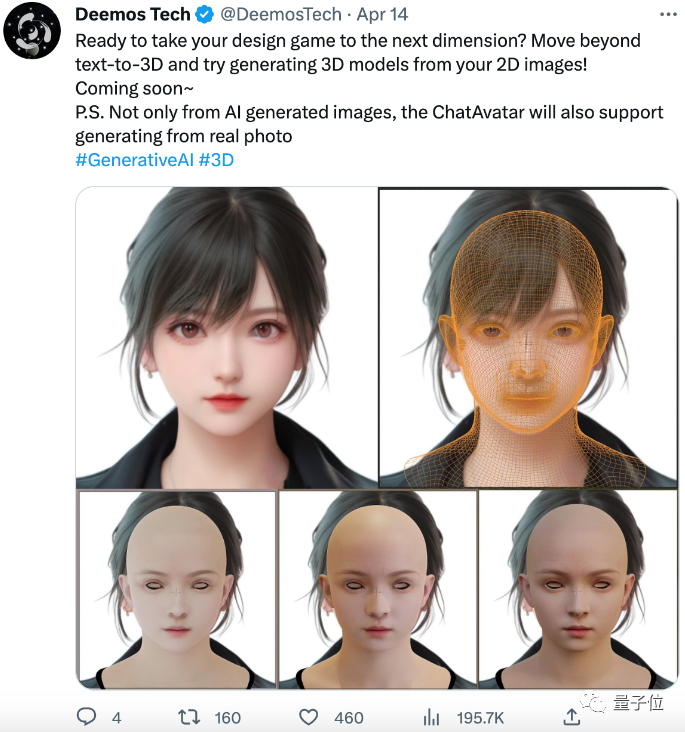
ChatGPT给AI行业注入一剂鸡血,一切曾经的不敢想,都成为如今的基操。正持续进击的Text-to-3D,就被视为继Diffusion(图像)和GPT(文字)后,AIGC领域的下一个前沿热点,得到了前所未有的关注度。这不,一款名为ChatAvatar的产品低调公测,火速收揽超70万浏览与关注,并登上抱抱脸周热门(Spacesoftheweek)。△ChatAvatar也将支持从AI生成的单视角/多视角原画生成3D风格化角色的Imageto3D技术,受到了广泛关注现行beta版本生成的3D模型,
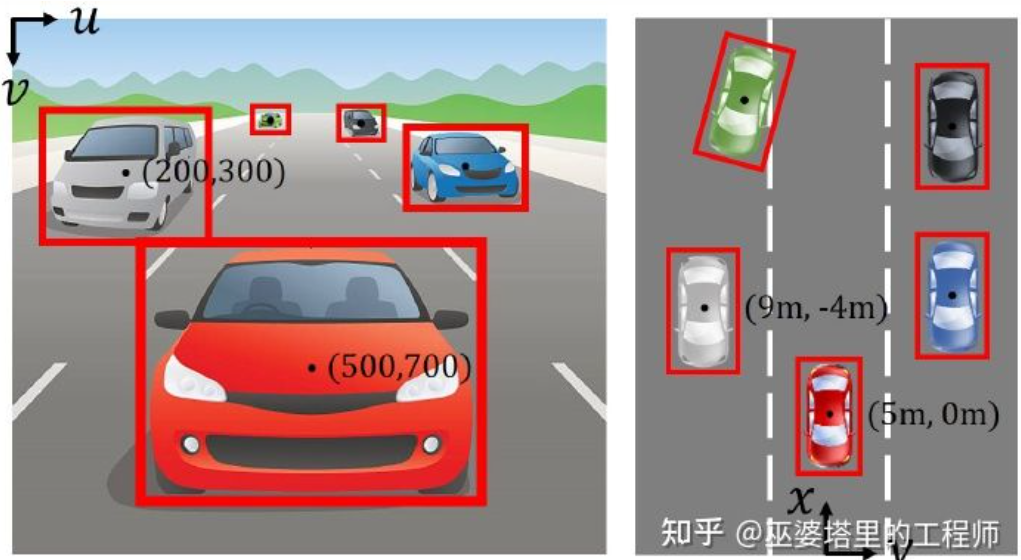
对于自动驾驶应用来说,最终还是需要对3D场景进行感知。道理很简单,车辆不能靠着一张图像上得到感知结果来行驶,就算是人类司机也不能对着一张图像来开车。因为物体的距离和场景的和深度信息在2D感知结果上是体现不出来的,而这些信息才是自动驾驶系统对周围环境作出正确判断的关键。一般来说,自动驾驶车辆的视觉传感器(比如摄像头)安装在车身上方或者车内后视镜上。无论哪个位置,摄像头所得到的都是真实世界在透视视图(PerspectiveView)下的投影(世界坐标系到图像坐标系)。这种视图与人类的视觉系统很类似,
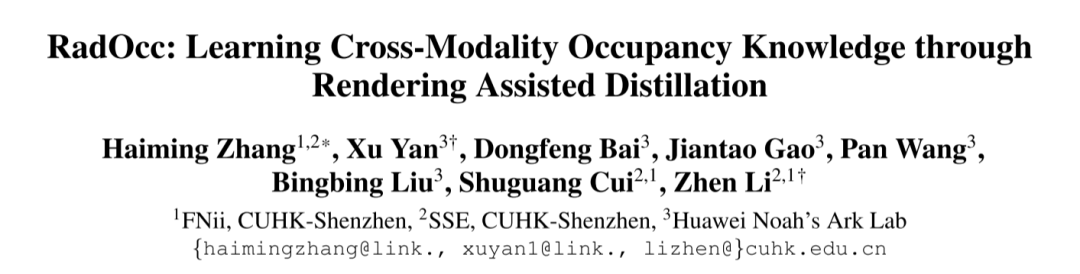
原标题:Radocc:LearningCross-ModalityOccupancyKnowledgethroughRenderingAssistedDistillation论文链接:https://arxiv.org/pdf/2312.11829.pdf作者单位:FNii,CUHK-ShenzhenSSE,CUHK-Shenzhen华为诺亚方舟实验室会议:AAAI2024论文思路:3D占用预测是一项新兴任务,旨在使用多视图图像估计3D场景的占用状态和语义。然而,由于缺乏几何先验,基于图像的场景
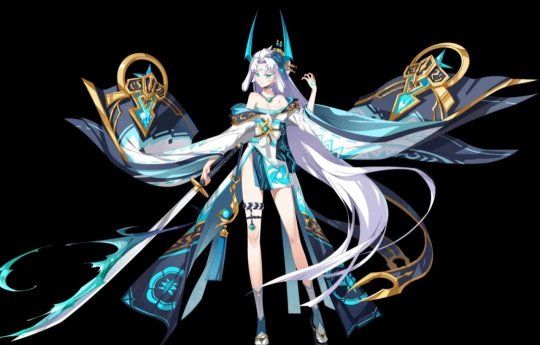
一些原神“奇怪”的关键词,在这两天很有关注度,明明搜索指数没啥变化,却不断有热议话题蹦窜。例如了龙王、钟离等“转变”立绘激增,虽在网络上疯传了一阵子,但是经过追溯发现这些是合理、常规的二创同人。如果单是这些,倒也翻不起多大的热度。按照一部分网友的说法,除了原神自身就有热度外,发现了一件格外醒目的事情:原神3d同人作者shirakami已经被捕。这引发了不小的热议。为什么被捕?关键词,原神3D动画。还是越过了线(就是你想的那种),再多就不能明说了。经过多方求证,以及新闻报道,确实有此事。自从去年发


Hot AI Tools

Undresser.AI Undress
AI-powered app for creating realistic nude photos

AI Clothes Remover
Online AI tool for removing clothes from photos.

Undress AI Tool
Undress images for free

Clothoff.io
AI clothes remover

AI Hentai Generator
Generate AI Hentai for free.

Hot Article

Hot Tools

PhpStorm Mac version
The latest (2018.2.1) professional PHP integrated development tool

DVWA
Damn Vulnerable Web App (DVWA) is a PHP/MySQL web application that is very vulnerable. Its main goals are to be an aid for security professionals to test their skills and tools in a legal environment, to help web developers better understand the process of securing web applications, and to help teachers/students teach/learn in a classroom environment Web application security. The goal of DVWA is to practice some of the most common web vulnerabilities through a simple and straightforward interface, with varying degrees of difficulty. Please note that this software

SecLists
SecLists is the ultimate security tester's companion. It is a collection of various types of lists that are frequently used during security assessments, all in one place. SecLists helps make security testing more efficient and productive by conveniently providing all the lists a security tester might need. List types include usernames, passwords, URLs, fuzzing payloads, sensitive data patterns, web shells, and more. The tester can simply pull this repository onto a new test machine and he will have access to every type of list he needs.

Safe Exam Browser
Safe Exam Browser is a secure browser environment for taking online exams securely. This software turns any computer into a secure workstation. It controls access to any utility and prevents students from using unauthorized resources.

MinGW - Minimalist GNU for Windows
This project is in the process of being migrated to osdn.net/projects/mingw, you can continue to follow us there. MinGW: A native Windows port of the GNU Compiler Collection (GCC), freely distributable import libraries and header files for building native Windows applications; includes extensions to the MSVC runtime to support C99 functionality. All MinGW software can run on 64-bit Windows platforms.
