


Hello, everyone.
I have shared with you fall recognition and fight recognition before. Today, we will take fall recognition as an example to see if we can completely leave it to ChatGPT.
Let ChatGPT do this. The most important thing is how to ask questions to ChatGPT. Throw all the questions directly to ChatGPT. For example, it is not advisable to write a fall detection code in Python. Instead, Like squeezing toothpaste, we guide ChatGPT step by step to get accurate answers, so that ChatGPT can truly improve our efficiency in solving problems.
The fall recognition case shared today has a clear idea of dialogue with ChatGPT and high code usability. According to the results returned by GPT, a fall recognition project can be developed, and there are some extended topics. Such as: Bi She’s improvement points.
The formal sharing begins below.
1. Role setting
To ask a question to ChatGPT, you must first give him a role setting. In the algorithm field, this is called prompt. Only by entering the role can you answer the question better. Therefore, the first sentence of my question is:
If you are an AI engineer, and I want to identify whether there is a fall in the video, what are the methods?
There is no need to let it go as soon as you come up. He writes the code directly, and first asks him what methods or ideas he has.
Let’s take a look at ChatGPT’s answer
gives 3 solutions, including traditional image methods and deep learning methods.
2. Idea guidance
Of course we are interested in deep learning, so we continue to ask about deep learning methods
What are the methods of using deep learning
ChatGPT answer
I have talked about it all from convolutional neural network to convolutional recurrent neural network.
It seems that the recurrent neural network RNN is simpler and more reliable. My next question is
I need the method of recurrent neural network (RNN)
ChatGPT answer
gives very detailed and very practical steps. I will briefly classify them into two steps:
The first step is to Video preprocessing, use a convolutional neural network (such as ResNet) to extract video action features (similar to wordembedding). The second step is to use the action features as the input of the lstm model, train the lstm recurrent neural network, classify the action features, and thereby identify falls. Inverted behavior
3. Write code
So far, ChatGPT has provided us with very clear ideas for solving problems and very strong executability.
So, at this time we can let ChatGPT write code.
According to the steps I divided above, I asked him to write the first step code at once, so my question is:
Write the video preprocessing code and use pytorch resnet Extracting video features
ChatGPT’s answer
好的,下面是通过 Pytorch 的 ResNet 提取视频特征的代码,以及视频预处理的示例代码: 视频预处理: import cv2 import numpy as np def preprocess_video(video_path, n_frames=16, resize=(224, 224)): # 读取视频 cap = cv2.VideoCapture(video_path) frames = [] while True: ret, frame = cap.read() if not ret: break # 强制转换为 RGB frame = cv2.cvtColor(frame, cv2.COLOR_BGR2RGB) # 图像缩放 frame = cv2.resize(frame, resize) # 图像增强等操作 # ... frames.append(frame) cap.release() # 取固定帧数 n_frames,超过后从中间开始截取 n_frames = min(len(frames), n_frames) start = (len(frames) - n_frames) // 2 end = start + n_frames # 截取帧数后堆叠成四维数组 frames = np.stack(frames[start:end], axis=0) # 转换为支持 Pytorch 模型的格式 (B, C, T, H, W) frames = np.transpose(frames, (0, 3, 1, 2)) return frames 提取视频特征: import torch import torchvision.models as models def extract_features(frames): # 加载 ResNet 模型 resnet = models.resnet50(pretrained=True) # 去掉最后的全连接层 resnet = torch.nn.Sequential(*list(resnet.children())[:-1]) # 将 ResNet 设置为评估模式 resnet.eval() # 将预处理后的视频序列转化为 Tensor,并调整为指定的数据类型和范围 frames = torch.from_numpy(frames).float() / 255.0 # 减去 ImageNet 数据集的均值和标准差 normalize = torch.nn.Normalize( mean=[0.485, 0.456, 0.406], std=[0.229, 0.224, 0.225] ) frames = normalize(frames) # 前向预测,输出 2048 维特征向量 with torch.no_grad(): features = resnet(frames) return features.reshape(-1, 2048) 在上面这两块代码中,preprocess_video() 函数将读取视频,并将其转换为指定大小的图像帧,返回处理后的数据;extract_features() 函数将接受上一步处理后的数据,并将其转化为 Pytorch 的 Tensor,使用训练好的 ResNet 模型对每一帧图像进行特征提取,并返回一个形状为 (B, 2048) 的 Tensor,其中 B 为帧数。
The picture is too long. In order for everyone to better understand ChatGPT’s answer, I directly posted the code text.
Defines two independent functions, which respectively complete the two small steps in the first step.
There is no problem with the code. First call the preprocess_video function to preprocess the video, and then call the extract_features convolution operation to embed a vector into a 2048-dimensional vector.
In the second step, I split it into two small steps, first building the lstm model, and then training
Based on the results produced above, use pytorch lstm to build the video classification model
ChatGPT’s answer
Write the model training code
ChatGPT’s answer
好的,下面是使用 Pytorch 训练视频分类模型的代码: import torch import torch.nn as nn import torch.optim as optim # 训练函数 def train(model, criterion, optimizer, dataloader, num_epochs=10): device = torch.device("cuda" if torch.cuda.is_available() else "cpu") model.to(device)# 将模型移动到 GPU 或 CPU 上 for epoch in range(num_epochs): running_loss = 0.0 correct_predictions = 0 total_predictions = 0 for inputs, labels in dataloader: inputs = inputs.to(device) labels = labels.to(device) optimizer.zero_grad() with torch.set_grad_enabled(True): outputs = model(inputs) _, preds = torch.max(outputs, 1) loss = criterion(outputs, labels) loss.backward() optimizer.step() running_loss += loss.item() * inputs.size(0) correct_predictions += torch.sum(preds == labels.data) total_predictions += labels.size(0) epoch_loss = running_loss / len(dataloader.dataset) epoch_acc = correct_predictions.double() / total_predictions print("Epoch {}: Loss {:.4f} Acc: {:.4f}".format(epoch + 1, epoch_loss, epoch_acc)) # 定义超参数 BATCH_SIZE = 4 NUM_EPOCHS = 20 # 定义数据集,假设已经准备好了训练数据集和测试数据集 train_dataset = ... test_dataset = ... # 定义数据加载器 train_dataloader = torch.utils.data.DataLoader( train_dataset, batch_size=BATCH_SIZE, shuffle=True ) test_dataloader = torch.utils.data.DataLoader( test_dataset, batch_size=BATCH_SIZE, shuffle=False ) # 定义模型 input_size = 2048 hidden_size = 512 num_layers = 1 num_classes = 2 model = VideoLSTM(input_size, hidden_size, num_layers, num_classes) # 定义损失函数和优化器 criterion = nn.CrossEntropyLoss() optimizer = optim.Adam(model.parameters()) # 训练模型 train(model, criterion, optimizer, train_dataloader, num_epochs=NUM_EPOCHS) 在代码中,首先定义了模型的超参数,如 BATCH_SIZE 和 NUM_EPOCHS。接着定义了训练数据集和测试数据集,并使用 Pytorch 的 DataLoader 将数据加载为 batch。然后定义了模型、损失函数和优化器。最后调用 train() 函数进行模型训练。 在训练过程中,每个 epoch 循环训练所有的视频帧,计算损失函数,计算准确率,并输出当前 epoch 的平均损失和准确率。训练完成后,可以将模型的参数保存到磁盘上,以便以后使用。
The usability of the code is very high. Except for the cost of code concatenation, each independent module basically does not need to be moved, and the development efficiency is very efficient.
You can also ask ChatGPT to find some data sets
You can also ask him to write the code for model inference
It can automatically help us string together the three parts of video preprocessing, feature extraction and inference to form a complete project.
4. Extension
At this point, we have completely completed the project using ChatGPT.
We can also talk about some extended topics, such as:
We can also ask ChatGPT to help us think of some points that can highlight academic value
Seeing this, it means that my article is of great significance to You have some help. Regardless of whether I join Planet or not, I am grateful for everyone's recognition and trust in me.
The above is the detailed content of Fall detection - completely developed with ChatGPT, share how to ask questions to ChatGPT correctly. For more information, please follow other related articles on the PHP Chinese website!
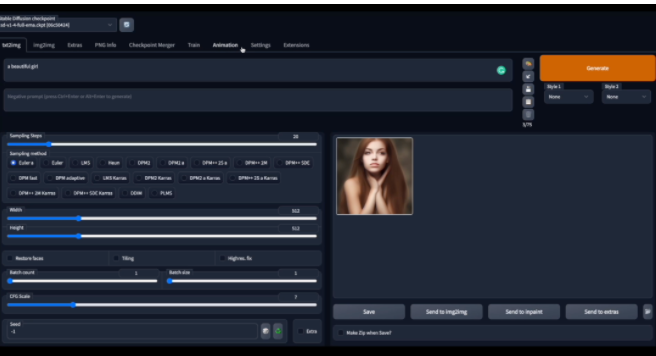
自从 ChatGPT、Stable Diffusion 发布以来,各种相关开源项目百花齐放,着实让人应接不暇。今天,着重挑选几个优质的开源项目分享给大家,对我们的日常工作、学习生活,都会有很大的帮助。
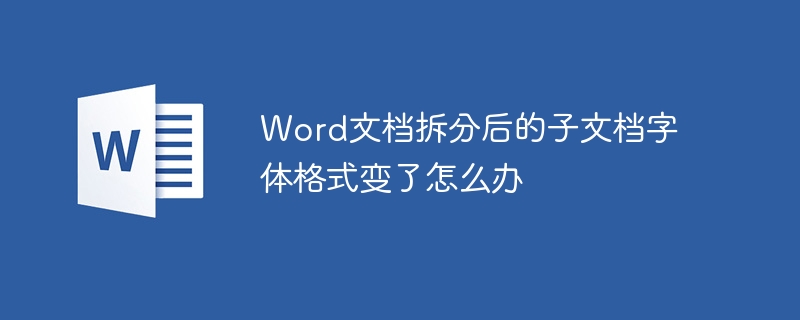
Word文档拆分后的子文档字体格式变了的解决办法:1、在大纲模式拆分文档前,先选中正文内容创建一个新的样式,给样式取一个与众不同的名字;2、选中第二段正文内容,通过选择相似文本的功能将剩余正文内容全部设置为新建样式格式;3、进入大纲模式进行文档拆分,操作完成后打开子文档,正文字体格式就是拆分前新建的样式内容。
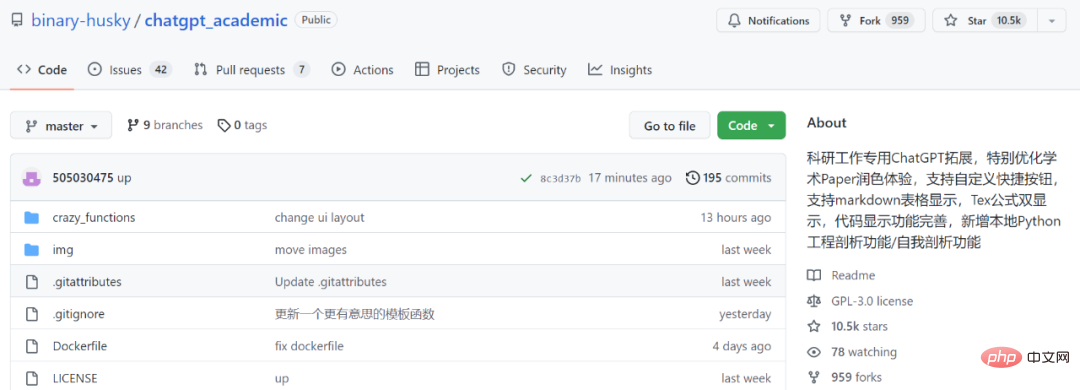
用 ChatGPT 辅助写论文这件事,越来越靠谱了。 ChatGPT 发布以来,各个领域的从业者都在探索 ChatGPT 的应用前景,挖掘它的潜力。其中,学术文本的理解与编辑是一种极具挑战性的应用场景,因为学术文本需要较高的专业性、严谨性等,有时还需要处理公式、代码、图谱等特殊的内容格式。现在,一个名为「ChatGPT 学术优化(chatgpt_academic)」的新项目在 GitHub 上爆火,上线几天就在 GitHub 上狂揽上万 Star。项目地址:https://github.com/
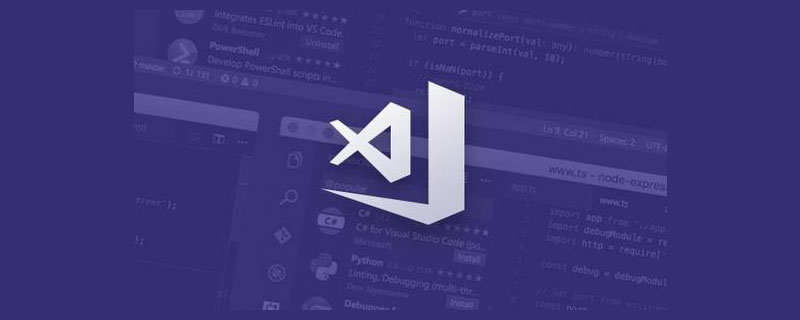
面对一夜爆火的 ChatGPT ,我最终也没抵得住诱惑,决定体验一下,不过这玩意要注册需要外国手机号以及科学上网,将许多人拦在门外,本篇博客将体验当下爆火的 ChatGPT 以及无需注册和科学上网,拿来即用的 ChatGPT 使用攻略,快来试试吧!
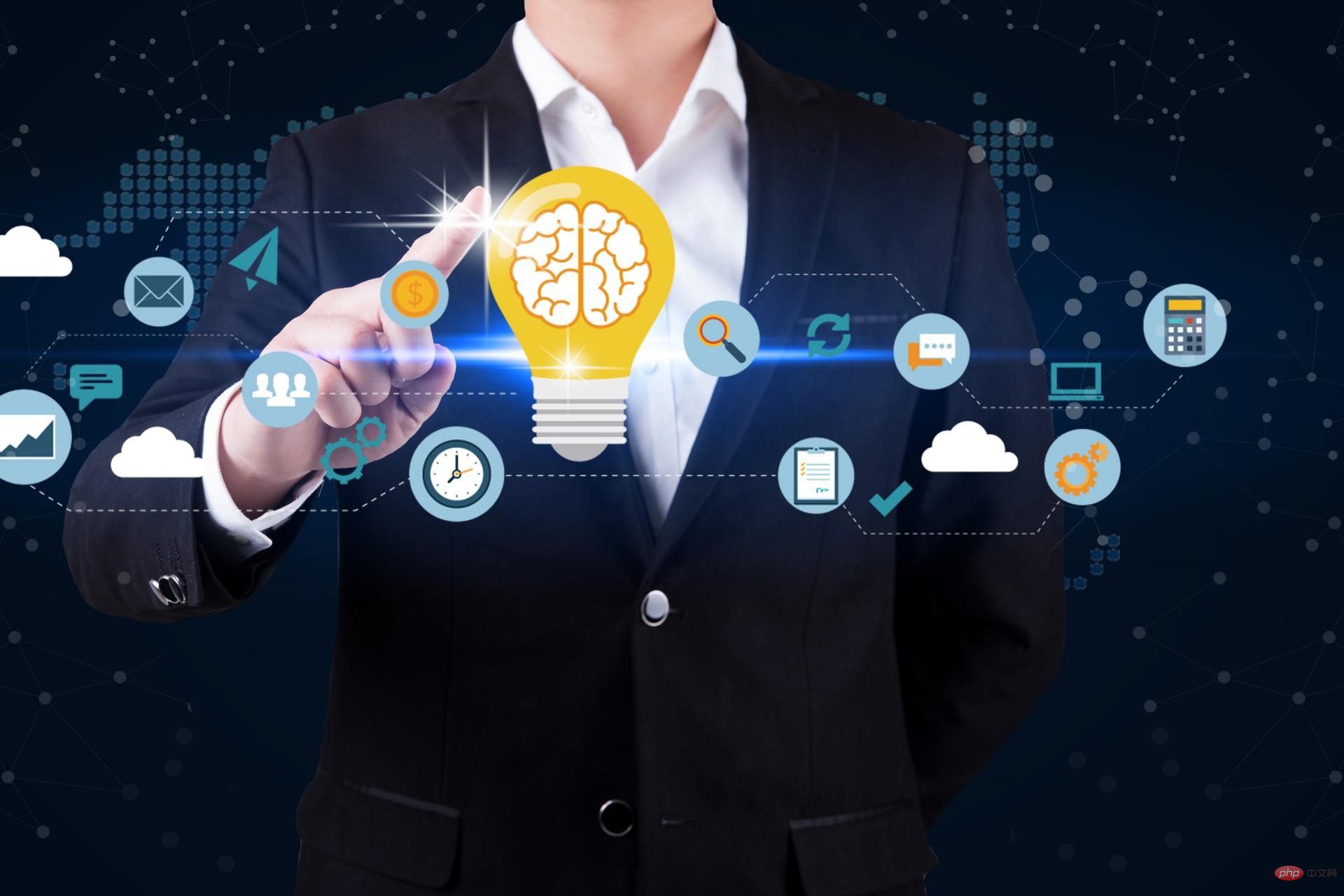
阅读论文可以说是我们的日常工作之一,论文的数量太多,我们如何快速阅读归纳呢?自从ChatGPT出现以后,有很多阅读论文的服务可以使用。其实使用ChatGPT API非常简单,我们只用30行python代码就可以在本地搭建一个自己的应用。 阅读论文可以说是我们的日常工作之一,论文的数量太多,我们如何快速阅读归纳呢?自从ChatGPT出现以后,有很多阅读论文的服务可以使用。其实使用ChatGPT API非常简单,我们只用30行python代码就可以在本地搭建一个自己的应用。使用 Python 和 C
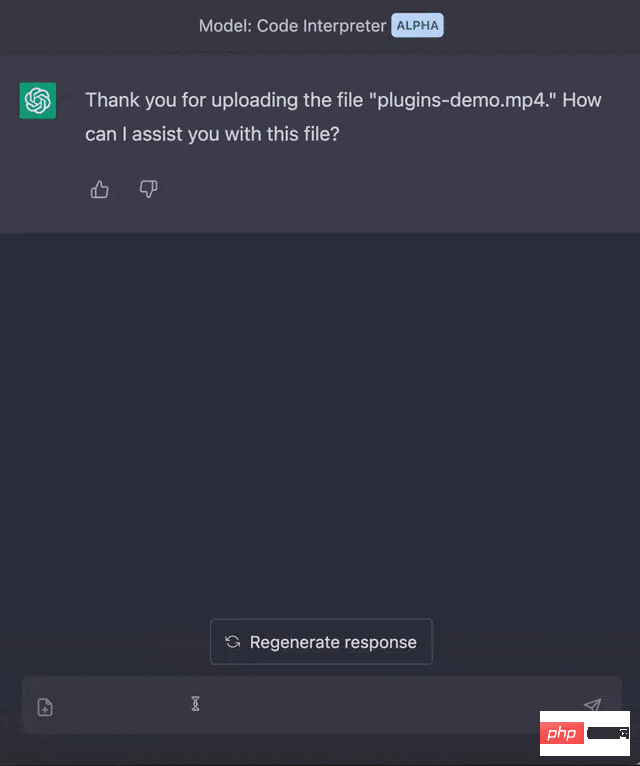
ChatGPT可以联网后,OpenAI还火速介绍了一款代码生成器,在这个插件的加持下,ChatGPT甚至可以自己生成机器学习模型了。 上周五,OpenAI刚刚宣布了惊爆的消息,ChatGPT可以联网,接入第三方插件了!而除了第三方插件,OpenAI也介绍了一款自家的插件「代码解释器」,并给出了几个特别的用例:解决定量和定性的数学问题;进行数据分析和可视化;快速转换文件格式。此外,Greg Brockman演示了ChatGPT还可以对上传视频文件进行处理。而一位叫Andrew Mayne的畅销作
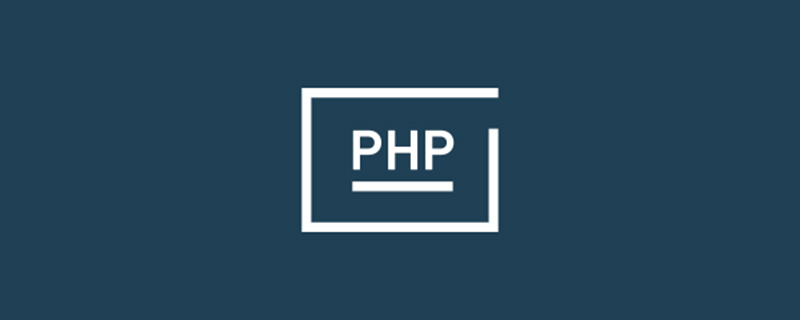
本篇文章给大家带来了关于php的相关知识,其中主要介绍了我是怎么用ChatGPT学习PHP中AOP的实现,感兴趣的朋友下面一起来看一下吧,希望对大家有帮助。


Hot AI Tools

Undresser.AI Undress
AI-powered app for creating realistic nude photos

AI Clothes Remover
Online AI tool for removing clothes from photos.

Undress AI Tool
Undress images for free

Clothoff.io
AI clothes remover

AI Hentai Generator
Generate AI Hentai for free.

Hot Article

Hot Tools

Safe Exam Browser
Safe Exam Browser is a secure browser environment for taking online exams securely. This software turns any computer into a secure workstation. It controls access to any utility and prevents students from using unauthorized resources.
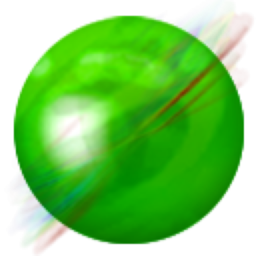
ZendStudio 13.5.1 Mac
Powerful PHP integrated development environment

SublimeText3 English version
Recommended: Win version, supports code prompts!

Zend Studio 13.0.1
Powerful PHP integrated development environment
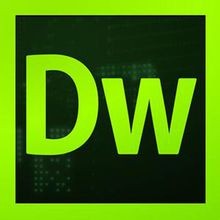
Dreamweaver CS6
Visual web development tools
