


This is the method in the latest paper published by Northeastern University and MIT: Reflexion.
This article is reprinted with the authorization of AI New Media Qubit (public account ID: QbitAI). Please contact the source for reprinting.
GPT-4 evolves again!
With a simple method, large language models such as GPT-4 can learn to self-reflect, and the performance can be directly improved by 30%.
Before this, large language models gave wrong answers. They often apologized without saying a word, and then emmmmmmm, they continued to make random guesses.
Now, it will no longer be like this. With the addition of new methods, GPT-4 will not only reflect on where it went wrong, but also give improvement strategies.
For example, it will automatically analyze why it is "stuck in a loop":
Or reflect on your own flawed search strategy:
This is the method in the latest paper published by Northeastern University and MIT: Reflexion.
Not only applies to GPT-4, but also to other large language models, allowing them to learn the unique human reflection ability.
The paper has been published on the preprint platform arxiv.
This directly made netizens say, "The speed of AI evolution has exceeded our ability to adapt, and we will be destroyed."
Some netizens even sent a "job warning" to developers:
The hourly wage for writing code in this way is cheaper than that of ordinary developers.
Use the binary reward mechanism to achieve reflection
As netizens said, the reflection ability given to GPT-4 by Reflexion is similar to the human thinking process:
can be summed up in two words: Feedback.
This feedback process can be divided into three major steps:
- 1. Evaluation: Test the accuracy of the currently generated answers
- 2. Generation of self-reflection: Error identification - implementation of correction
- 3. Implementation of an iterative feedback loop
In the first step of the evaluation process, first What you need to go through is the self-assessment of LLM (Large Language Model).
That is to say, LLM must first reflect on the answer itself when there is no external feedback.
How to conduct self-reflection?
The research team used a binary reward mechanism to assign values to the operations performed by LLM in the current state:
1 represents the generated result OK, 0 It means that the generated results are not very good.
The reason why binary is used instead of more descriptive reward mechanisms such as multi-valued or continuous output is related to the lack of external input.
To conduct self-reflection without external feedback, the answer must be restricted to binary states. Only in this way can the LLM be forced to make meaningful inferences.
After the self-evaluation is completed, if the output of the binary reward mechanism is 1, the self-reflection device will not be activated. If it is 0, the LLM will turn on the reflection mode.
During the reflection process, the model will trigger a heuristic function h (as shown below). Analogous to the human thinking process, h plays the same role as supervision.
#However, like human thinking, LLM also has limitations in the process of reflection, which can be reflected in the Ω and ε in the function.
Ω represents the number of times a continuous action is repeated. Generally, this value is set to 3. This means that if a step is repeated three times during the reflection process, it will jump directly to the next step.
And ε represents the maximum number of operations allowed to be performed during the reflection process.
Since there is supervision, correction must also be implemented. The function of the correction process is like this:
Among them, self-reflection Models are trained with "domain-specific failure trajectories and ideal reflection pairs" and do not allow access to domain-specific solutions to a given problem in the dataset.
In this way, LLM can come up with more "innovative" things in the process of reflection.
The performance increased by nearly 30% after reflection
Since LLMs such as GPT-4 can perform self-reflection, what is the specific effect?
The research team evaluated this approach on the ALFWorld and HotpotQA benchmarks.
In the HotpotQA test of 100 question and answer pairs, LLM using the Reflexion method showed huge advantages. After multiple rounds of reflection and repeated questions, the performance of LLM improved by nearly 30%.
Without using Reflexion, after repeated Q&A, there was no change in performance.
In HotpotQA’s 134 question-and-answer test, it can be seen that with the support of Reflexion, LLM’s accuracy reached 97% after multiple rounds of reflection.
In another blog, team members also showed the effect of their method on GPT-4. The scope of the test was writing code.
The results are also obvious. Using Reflexion, the programming ability of GPT-4 has been directly improved by 21%.
I already know how to "think" about GPT-4, how do you (huang) (le) read (ma)?
Paper address: https://arxiv.org/abs/2303.11366
The above is the detailed content of GPT-4 coding ability improved by 21%! MIT's new method allows LLM to learn to reflect, netizen: It's the same way as humans think. For more information, please follow other related articles on the PHP Chinese website!
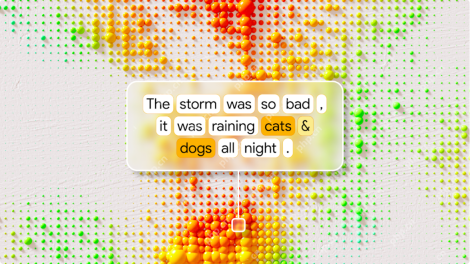
Exploring the Inner Workings of Language Models with Gemma Scope Understanding the complexities of AI language models is a significant challenge. Google's release of Gemma Scope, a comprehensive toolkit, offers researchers a powerful way to delve in
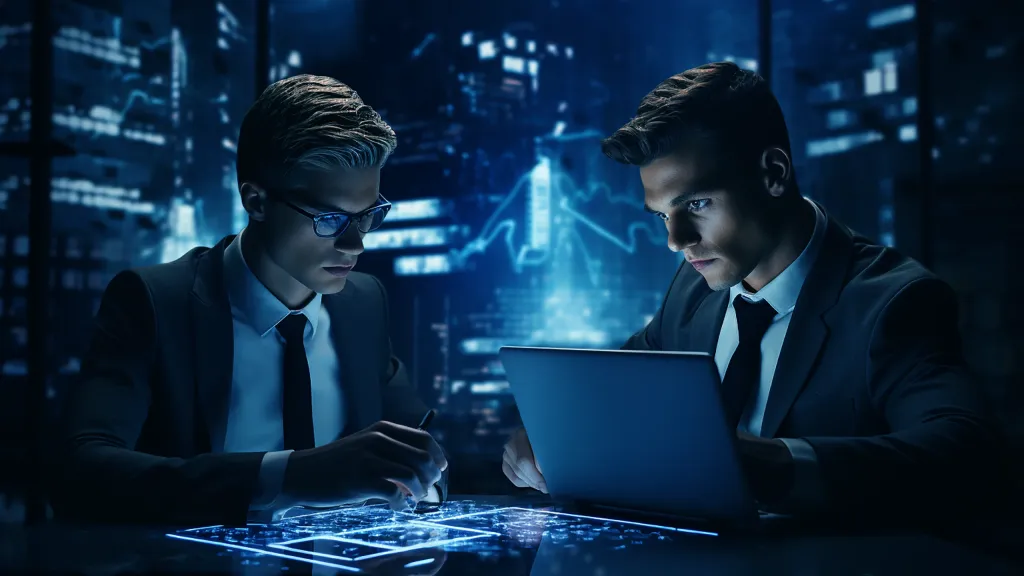
Unlocking Business Success: A Guide to Becoming a Business Intelligence Analyst Imagine transforming raw data into actionable insights that drive organizational growth. This is the power of a Business Intelligence (BI) Analyst – a crucial role in gu

SQL's ALTER TABLE Statement: Dynamically Adding Columns to Your Database In data management, SQL's adaptability is crucial. Need to adjust your database structure on the fly? The ALTER TABLE statement is your solution. This guide details adding colu
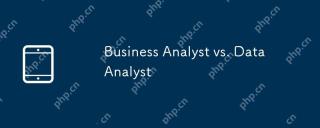
Introduction Imagine a bustling office where two professionals collaborate on a critical project. The business analyst focuses on the company's objectives, identifying areas for improvement, and ensuring strategic alignment with market trends. Simu
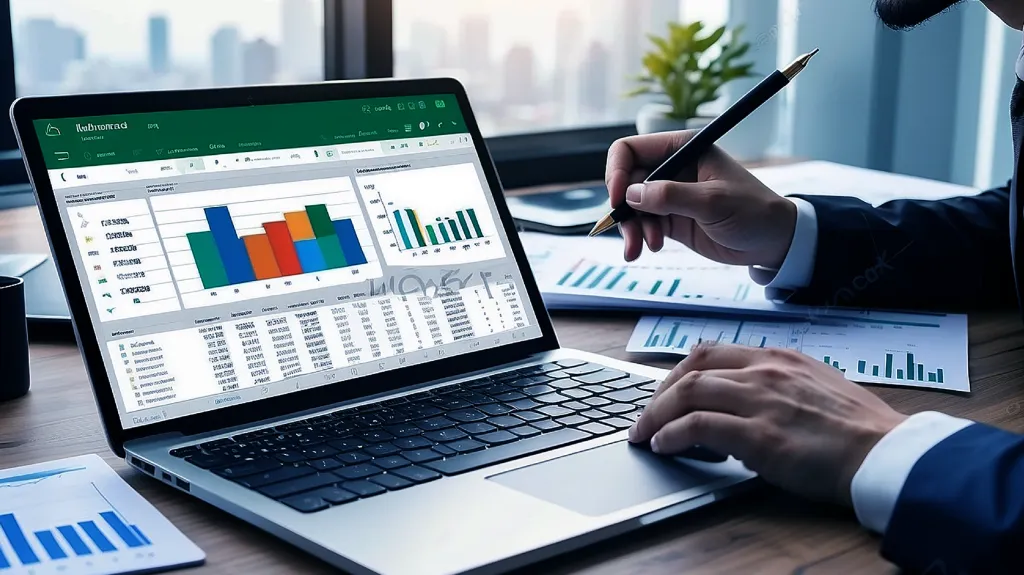
Excel data counting and analysis: detailed explanation of COUNT and COUNTA functions Accurate data counting and analysis are critical in Excel, especially when working with large data sets. Excel provides a variety of functions to achieve this, with the COUNT and COUNTA functions being key tools for counting the number of cells under different conditions. Although both functions are used to count cells, their design targets are targeted at different data types. Let's dig into the specific details of COUNT and COUNTA functions, highlight their unique features and differences, and learn how to apply them in data analysis. Overview of key points Understand COUNT and COU
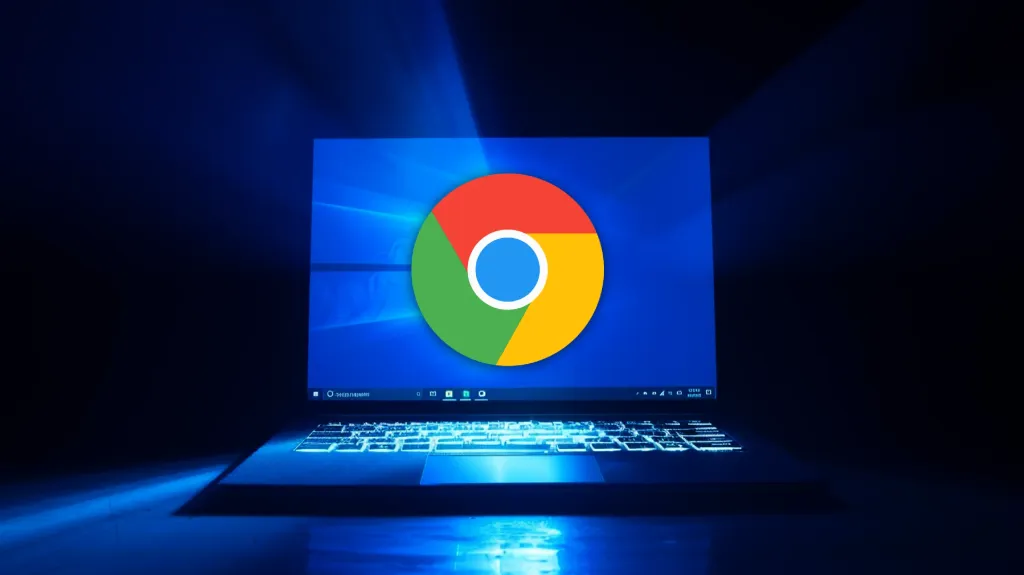
Google Chrome's AI Revolution: A Personalized and Efficient Browsing Experience Artificial Intelligence (AI) is rapidly transforming our daily lives, and Google Chrome is leading the charge in the web browsing arena. This article explores the exciti
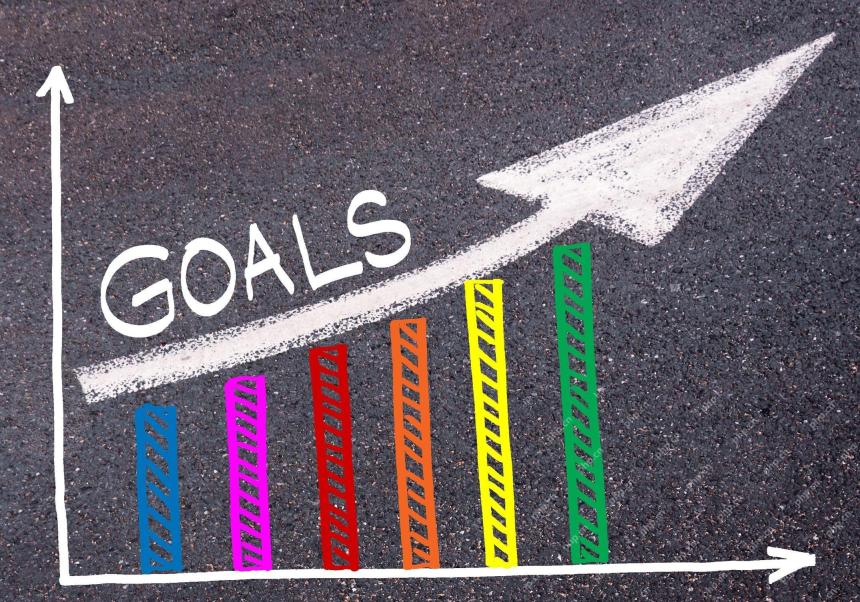
Reimagining Impact: The Quadruple Bottom Line For too long, the conversation has been dominated by a narrow view of AI’s impact, primarily focused on the bottom line of profit. However, a more holistic approach recognizes the interconnectedness of bu
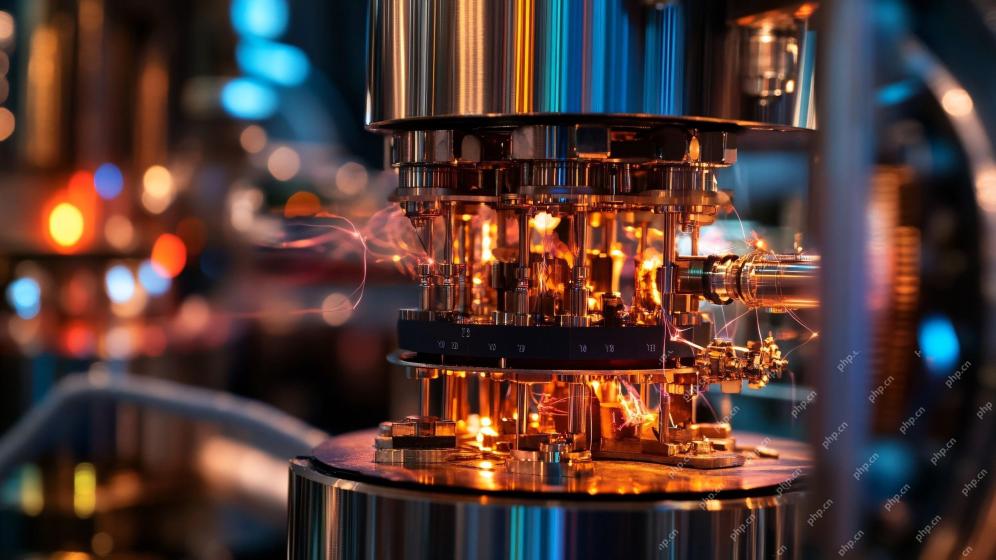
Things are moving steadily towards that point. The investment pouring into quantum service providers and startups shows that industry understands its significance. And a growing number of real-world use cases are emerging to demonstrate its value out


Hot AI Tools

Undresser.AI Undress
AI-powered app for creating realistic nude photos

AI Clothes Remover
Online AI tool for removing clothes from photos.

Undress AI Tool
Undress images for free

Clothoff.io
AI clothes remover

AI Hentai Generator
Generate AI Hentai for free.

Hot Article

Hot Tools

MinGW - Minimalist GNU for Windows
This project is in the process of being migrated to osdn.net/projects/mingw, you can continue to follow us there. MinGW: A native Windows port of the GNU Compiler Collection (GCC), freely distributable import libraries and header files for building native Windows applications; includes extensions to the MSVC runtime to support C99 functionality. All MinGW software can run on 64-bit Windows platforms.
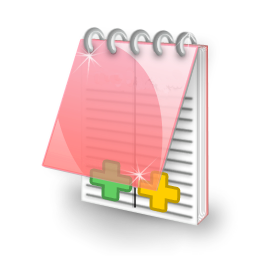
EditPlus Chinese cracked version
Small size, syntax highlighting, does not support code prompt function

SublimeText3 Chinese version
Chinese version, very easy to use

SublimeText3 Linux new version
SublimeText3 Linux latest version

Zend Studio 13.0.1
Powerful PHP integrated development environment