


We have begun to experience the feeling of reaching the upper limit of silicon computing experience. In the next 10 years, there will be a serious computing power gap, and neither existing technology companies nor governments have been able to solve this problem.
Now, we are so accustomed to computing becoming cheaper and cheaper that we never doubt that one day we may not be able to afford it.
Now, Rodolfo Rosini, CEO of a startup, asks a question that shocks us: What if we are reaching the fundamental physical limits of the classical computing model, as if our economy relies on cheap computing? what to do?
The stagnation of large-scale computing
Now, due to a lack of technological innovation, the United States has reached a plateau.
Wright’s Law holds true in many industries—every time the manufacturing process is improved by about 20%, productivity will double.
In the field of technology, it manifests itself as Moore's Law.
In the 1960s, Intel co-founder Gordon Moore noticed that the number of transistors in integrated circuits seemed to have doubled year-on-year and proposed Moore's Law.
Since then, this law has become the basis of the contract between marketing and engineering, leveraging excess computing power and shrinking size to drive the construction of products in the computing stack.
The expectation was that computing power would improve exponentially over time with faster and cheaper processors.
However, the different forces that make up Moore’s Law have changed.
For decades, the driving force behind Moore’s Law was Dennard’s Law of Scaling. Transistor size and power consumption are simultaneously halved, doubling the amount of computation per unit of energy (the latter is also known as Koomey’s LawKoomey’s Law).
50 Years of Microprocessor Trend Data
In 2005, this scaling began to fail due to current leakage causing the chip to heat up, and the The problem is that the performance of chips with a single processing core has stagnated.
To maintain its computing growth trajectory, the chip industry has turned to multi-core architectures: multiple microprocessors "glued" together. While this may extend Moore's Law in terms of transistor density, it increases the complexity of the entire computing stack.
For certain types of computing tasks, such as machine learning or computer graphics, this brings performance improvements. But for many general-purpose computing tasks that are not well parallelized, multi-core architectures are powerless.
In short, the computing power for many tasks is no longer increasing exponentially.
Even in terms of the performance of multi-core supercomputers, judging from the TOP500 (ranking of the world's fastest supercomputers), there was an obvious turning point around 2010.
What are the impacts of this slowdown? The growing role computing plays in different industries shows that the impact is immediate and will only become more important as Moore's Law falters further.
To take two extreme examples: Increased computing power and reduced costs have led to a 49% increase in the productivity of oil exploration in the energy industry, and a 94% increase in protein folding predictions in the biotechnology industry.
This means that the impact of computing speed is not limited to the technology industry. Much of the economic growth of the past 50 years has been a second-order effect driven by Moore's Law. Without it, the world economy may stop growing.
Another prominent reason for the need for more computing power is the rise of artificial intelligence. Today, training a large language model (LLM) can cost millions of dollars and take weeks.
The future promised by machine learning cannot be realized without continued increase in number crunching and data expansion.
As the growing popularity of machine learning models in consumer technology heralds huge and potentially hyperbolic demands for computing in other industries, cheap processing is becoming the cornerstone of productivity.
The death of Moore's Law may bring about a great stagnation in computing. Compared with the multi-modal neural networks that may be required to achieve AGI, today's LLMs are still relatively small and easy to train. Future GPTs and their competitors will require particularly powerful high-performance computers to improve and even optimize.
Perhaps many people will feel doubtful. After all, the end of Moore's Law has been predicted many times. Why should it be now?
Historically, many of these predictions stem from engineering challenges. Human ingenuity has overcome these obstacles time and time again before.
The difference now is that instead of being faced with engineering and intelligence challenges, we are faced with constraints imposed by physics.
MIT Technology Review published an article on February 24 stating that we are not prepared for the end of Moore’s Law
Overheating causes inability to process
Computers work by processing information.
As they process information, some of it is discarded as the microprocessor merges computational branches or overwrites the registry. It's not free.
The laws of thermodynamics, which place strict limits on the efficiency of certain processes, apply to calculations just as they do to steam engines. This cost is called Landauer’s limit.
It is the tiny amount of heat emitted during each computational operation: approximately 10^-21 joules per bit.
Given that this heat is so small, the Landauer limit has long been considered negligible.
However, engineering capabilities have now evolved to the point where this energy scale can be achieved because the real-world limit is estimated to be 10-100 times larger than Landauer's bound due to other overheads such as current leakage. Chips have hundreds of billions of transistors that operate billions of times per second.
Add these numbers together, and perhaps Moore’s Law still has an order of magnitude left to grow before reaching the thermal barrier.
At that point, existing transistor architectures will be unable to further improve energy efficiency, and the heat generated will prevent transistors from being packed more tightly.
If we don’t figure this out, we won’t be able to see clearly how industry values will change.
Microprocessors will be constrained and industry will compete for lower rewards for marginal energy efficiency.
The chip size will expand. Look at Nvidia's 4000-series GPU card: despite using a higher-density process, it's about the size of a small dog and packs a whopping 650W of power.
This prompted NVIDIA CEO Jen-Hsun Huang to declare in late 2022 that "Moore's Law is dead"—a statement that, while mostly true, has been denied by other semiconductor companies.
The IEEE releases a semiconductor roadmap every year. The latest assessment is that 2D shrinkage will be completed in 2028, and 3D shrinkage should be fully launched in 2031.
3D scaling (where chips are stacked on top of each other) is already common, but in computer memory, not in microprocessors.
This is because the heat dissipation of the memory is much lower; however, heat dissipation is complex in 3D architecture, so active memory cooling becomes important.
Memory with 256 layers is on the horizon, and is expected to reach the 1,000-layer mark by 2030.
Back to microprocessors, multi-gate device architectures that are becoming commercial standards (such as FinFETs and Gates-all-round) will continue to follow Moore's Law in the coming years.
However, due to inherent thermal problems, true vertical scaling was impossible after the 1930s.
In fact, current chipsets carefully monitor which parts of the processor are active at any time to avoid overheating even on a single plane.
2030 Crisis?
A century ago, American poet Robert Frost once asked: Will the world end in frost or fire?
If the answer is fire, it almost heralds the end of computing.
Or, just accept the fact that electricity use will increase and scale up microprocessor manufacturing.
For this purpose, humans have consumed a large portion of the earth's energy.
Perhaps another option is to simply accept increased power use and scale up microprocessor manufacturing. We already use a large portion of the Earth's energy supply for this purpose.
In Ireland, just 70 data centers consume 14% of the country’s energy. By the 2030s, it is expected that 30-50% of globally produced electricity will be used for computing and cooling.
(Interestingly, after the blog post was published on March 19, the author deleted this prediction. His explanation was that this was based on the worst-case scenario in the Nature paper. The extrapolation of the situation, which has now been deleted for the sake of clarity and precision of the argument)
And the current scale of energy production will lead to a slight increase in the cost of Moore's Law scale in the future.
A series of one-time optimization measures at the design (energy efficiency) and implementation levels (replacing old designs still in use with the latest technology) will allow developing economies such as India to catch up with the global overall productive forces.
After the end of Moore's Law, mankind will run out of energy before the manufacturing of microprocessor chips reaches its limit, and the pace of decline in computing costs will stagnate.
Although quantum computing is touted as an effective way to surpass Moore's Law, there are too many unknowns in it. It is still decades away from commercial development, and it will not be useful for at least the next 20 to 30 years. .
Clearly, there will be a serious computing power gap in the next 10 years, and no existing technology companies, investors, or government agencies will be able to solve it.
The collision between Moore's Law and Landauer's limit has been going on for decades, and it can be said to be one of the most significant and critical events in the 2030s.
But now, it seems that not many people know about this matter.
The above is the detailed content of Humanity has reached the upper limit of silicon computing architecture! AI is expected to consume 50% of global electricity supply by 2030. For more information, please follow other related articles on the PHP Chinese website!
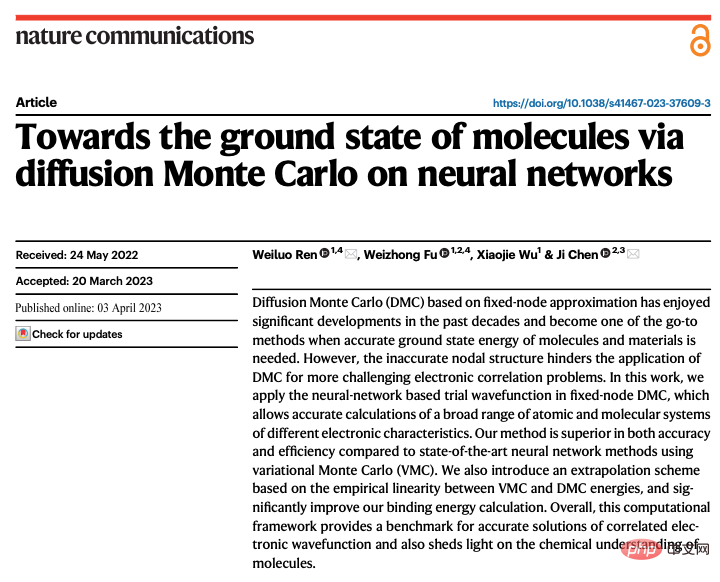
时隔四个月,ByteDanceResearch与北京大学物理学院陈基课题组又一合作工作登上国际顶级刊物NatureCommunications:论文《TowardsthegroundstateofmoleculesviadiffusionMonteCarloonneuralnetworks》将神经网络与扩散蒙特卡洛方法结合,大幅提升神经网络方法在量子化学相关任务上的计算精度、效率以及体系规模,成为最新SOTA。论文链接:https://www.nature.com
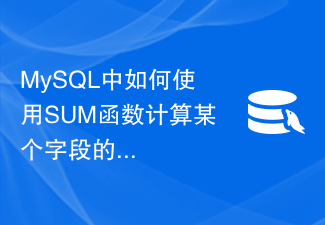
MySQL中如何使用SUM函数计算某个字段的总和在MySQL数据库中,SUM函数是一个非常有用的聚合函数,它可以用于计算某个字段的总和。本文将介绍如何在MySQL中使用SUM函数,并提供一些代码示例来帮助读者深入理解。首先,让我们看一个简单的示例。假设我们有一个名为"orders"的表,其中包含了顾客的订单信息。表结构如下:CREATETABLEorde
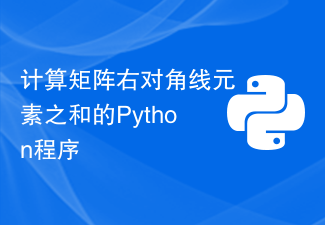
一种受欢迎的通用编程语言是Python。它被应用于各种行业,包括桌面应用程序、网页开发和机器学习。幸运的是,Python具有简单易懂的语法,适合初学者使用。在本文中,我们将使用Python来计算矩阵的右对角线之和。什么是矩阵?在数学中,我们使用一个矩形排列或矩阵,用于描述一个数学对象或其属性,它是一个包含数字、符号或表达式的矩形数组或表格,这些数字、符号或表达式按行和列排列。例如−234512367574因此,这是一个有3行4列的矩阵,表示为3*4矩阵。现在,矩阵中有两条对角线,即主对角线和次对
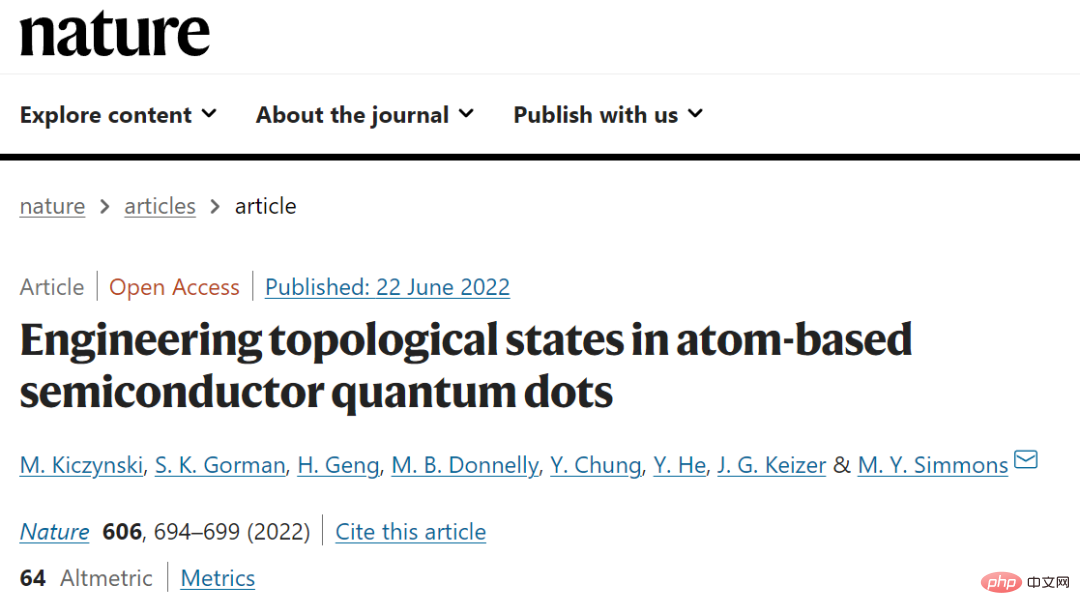
6 月 23 日,澳大利亚量子计算公司 SQC(Silicon Quantum Computing)宣布推出世界上第一个量子集成电路。这是一个包含经典计算机芯片上所有基本组件的电路,但体量是在量子尺度上。SQC 团队使用这种量子处理器准确地模拟了一个有机聚乙炔分子的量子态——最终证明了新量子系统建模技术的有效性。「这是一个重大突破,」SQC 创始人 Michelle Simmons 说道。由于原子之间可能存在大量相互作用,如今的经典计算机甚至难以模拟相对较小的分子。SQC 原子级电路技术的开发将
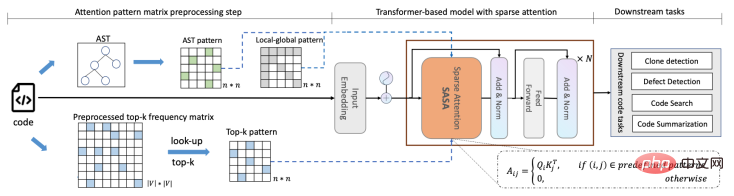
阿里云机器学习平台PAI与华东师范大学高明教授团队合作在SIGIR2022上发表了结构感知的稀疏注意力Transformer模型SASA,这是面向长代码序列的Transformer模型优化方法,致力于提升长代码场景下的效果和性能。由于self-attention模块的复杂度随序列长度呈次方增长,多数编程预训练语言模型(Programming-basedPretrainedLanguageModels,PPLM)采用序列截断的方式处理代码序列。SASA方法将self-attention的计算稀疏化
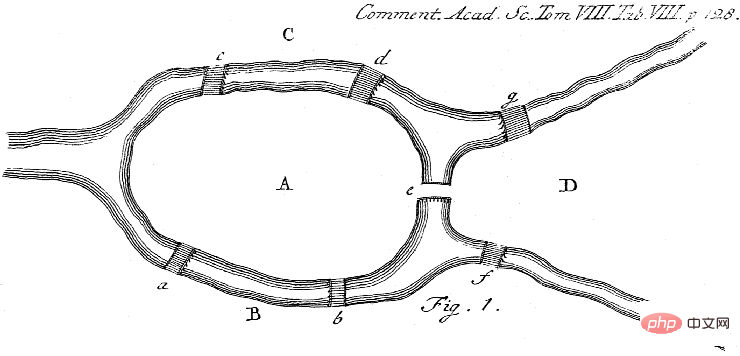
本文由Cristian Bodnar 和Fabrizio Frasca 合著,以 C. Bodnar 、F. Frasca 等人发表于2021 ICML《Weisfeiler and Lehman Go Topological: 信息传递简单网络》和2021 NeurIPS 《Weisfeiler and Lehman Go Cellular: CW 网络》论文为参考。本文仅是通过微分几何学和代数拓扑学的视角讨论图神经网络系列的部分内容。从计算机网络到大型强子对撞机中的粒子相互作用,图可以用来模
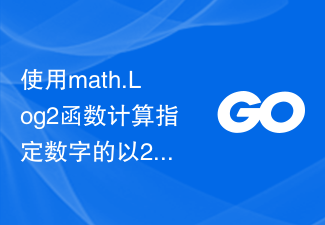
使用math.Log2函数计算指定数字的以2为底的对数在数学中,对数是一个重要的概念,它描述了一个数与另一个数(所谓的底)的指数关系。其中,以2为底的对数特别常见,并在计算机科学和信息技术领域中经常用到。在Python编程语言中,我们可以使用math库中的log2函数来计算一个数字的以2为底的对数。下面是一个简单的代码示例:importmathdef
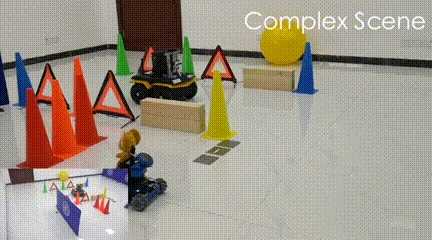
清华大学举办的一场机器人版猫捉老鼠游戏,登上了Science子刊封面。这里的汤姆猫有了新的名字:“天机猫”,它搭载了清华大学类脑芯片的最新研究成果——一款名为TianjicX的28nm神经形态计算芯片。它的任务是抓住一只随机奔跑的电子老鼠:在复杂的动态环境下,各种障碍被随机地、动态地放置在不同的位置,“天机猫”需要通过视觉识别、声音跟踪或两者结合的方式来追踪老鼠,然后在不与障碍物碰撞的情况下向老鼠移动,最终追上它。在此过程中,“天机猫”需要实现实时场景下的语音识别、声源定位、目标检测、避障和决


Hot AI Tools

Undresser.AI Undress
AI-powered app for creating realistic nude photos

AI Clothes Remover
Online AI tool for removing clothes from photos.

Undress AI Tool
Undress images for free

Clothoff.io
AI clothes remover

AI Hentai Generator
Generate AI Hentai for free.

Hot Article

Hot Tools

SAP NetWeaver Server Adapter for Eclipse
Integrate Eclipse with SAP NetWeaver application server.

SublimeText3 Linux new version
SublimeText3 Linux latest version

MinGW - Minimalist GNU for Windows
This project is in the process of being migrated to osdn.net/projects/mingw, you can continue to follow us there. MinGW: A native Windows port of the GNU Compiler Collection (GCC), freely distributable import libraries and header files for building native Windows applications; includes extensions to the MSVC runtime to support C99 functionality. All MinGW software can run on 64-bit Windows platforms.
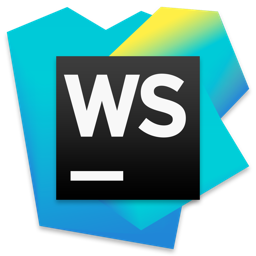
WebStorm Mac version
Useful JavaScript development tools
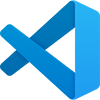
VSCode Windows 64-bit Download
A free and powerful IDE editor launched by Microsoft
