


Recently, a research team from the University of Zurich found that ChatGPT outperformed crowdsourcing workers on multiple NLP annotation tasks, with high consistency, and the cost of each annotation was only about US$0.003, which is 20 times cheaper than MTurk.
Currently, many natural language processing (NLP) applications require high-quality annotated data to support, especially when these data are used for tasks such as training classifiers or evaluating the performance of unsupervised models.
For example, artificial intelligence researchers often want to filter noisy social media data for relevance, assign text to different topic or conceptual categories, or measure its sentiment or stance.
Moreover, no matter what specific method (supervised, semi-supervised or unsupervised) is used for these tasks, labeled data is needed to establish a training set or gold standard.
However, in most cases, to complete high-quality data annotation work, it is still inseparable from crowdsourcing workers on the data annotation platform or well-trained annotators such as research assistants. You can do it manually.
Typically, trained annotators first create a relatively small gold standard data set, and then hire crowd workers to increase the amount of annotated data and perform repetitive work. Depending on the size and complexity, data annotation tasks can sometimes be very time-consuming and labor-intensive. Not only do they require a certain amount of labor costs, but the quality of data annotation cannot be guaranteed.
So, can machines help humans complete this basic task?
In the past, machines were not good at this kind of "slow work and careful work" tasks, but unexpectedly, the "data annotation" matter has been completed by ChatGPT, and it is better than Most people do better.
In a new study published today, a research team from the University of Zurich used a sample of 2,382 tweets to demonstrate that ChatGPT performs better on relevance, topic, and Outperforms crowdsourcing workers on multiple annotation tasks such as frame detection.
The related research paper is titled "ChatGPT Outperforms Crowd-Workers for Text-Annotation Tasks" and has been published on the preprint website arXiv.
Specifically, ChatGPT’s zero-shot accuracy exceeded crowdsourcing workers in four of the five tasks; it demonstrated intercoder consistency in all tasks In terms of agreement), ChatGPT not only surpasses crowdsourcing workers, but also surpasses trained annotators.
ChatGPT zero-sample text data annotation performance
It is worth mentioning that the cost of each annotation of ChatGPT is less than 0.003 US dollars, which is about 20 times cheaper than the data annotation platform.
The research team believes that while further research is needed to better understand how ChatGPT and other LLMs perform in a broader context, the findings suggest that they have the potential to change the way researchers annotate data. , greatly improving the efficiency of text classification and destroying some business models of data annotation platforms.
At least for now, these findings demonstrate the importance of delving deeper into the text annotation properties and capabilities of LLMs.
In the future, the research team will study the performance of ChatGPT in multiple languages, the performance of ChatGPT in multiple types of texts (social media, news media, legislation, speeches, etc.), and use Chain of Thoughts (CoT) Work continues on hints and other strategies to improve the performance of zero-shot inference.
It is worth mentioning that when the research team was conducting this work, OpenAI had not yet released GPT-4. What would be the result if GPT-4 was used to complete the data annotation task?
Reference:https://arxiv.org/abs/2303.15056
The above is the detailed content of It only costs $0.003 a time, which is 20 times cheaper than humans! ChatGPT puts data annotators in danger. For more information, please follow other related articles on the PHP Chinese website!
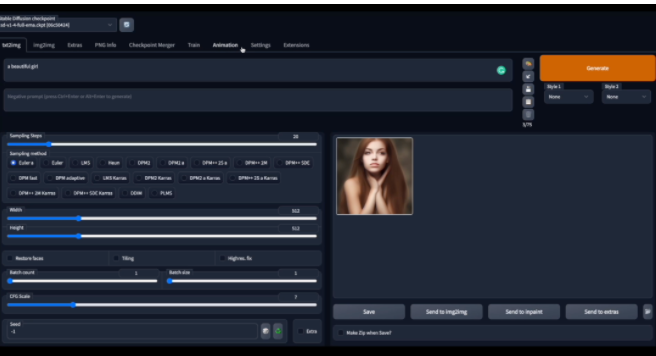
自从 ChatGPT、Stable Diffusion 发布以来,各种相关开源项目百花齐放,着实让人应接不暇。今天,着重挑选几个优质的开源项目分享给大家,对我们的日常工作、学习生活,都会有很大的帮助。
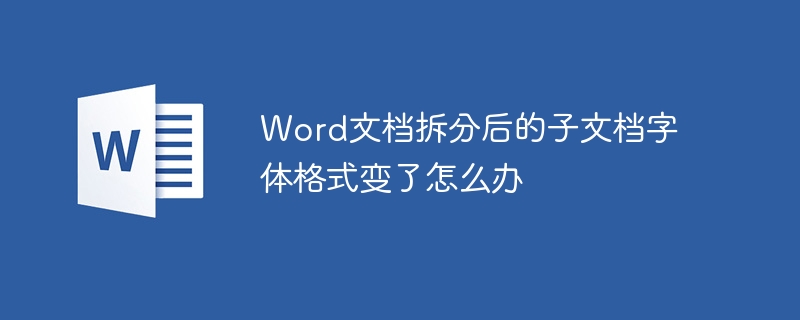
Word文档拆分后的子文档字体格式变了的解决办法:1、在大纲模式拆分文档前,先选中正文内容创建一个新的样式,给样式取一个与众不同的名字;2、选中第二段正文内容,通过选择相似文本的功能将剩余正文内容全部设置为新建样式格式;3、进入大纲模式进行文档拆分,操作完成后打开子文档,正文字体格式就是拆分前新建的样式内容。
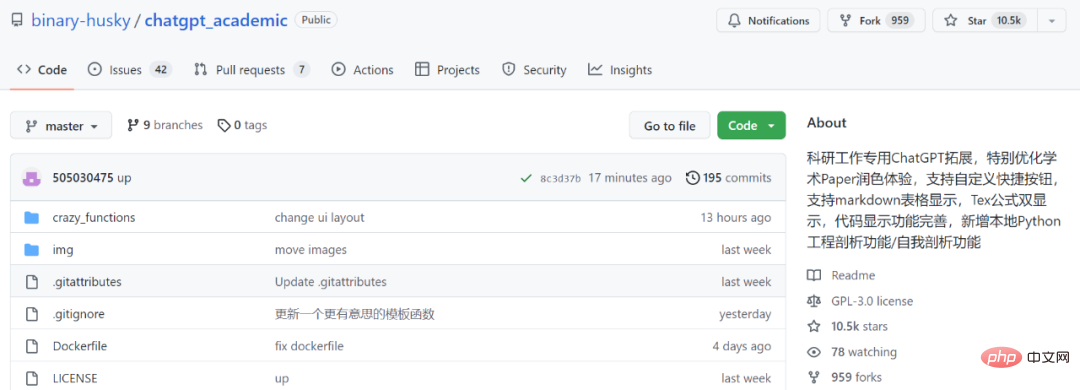
用 ChatGPT 辅助写论文这件事,越来越靠谱了。 ChatGPT 发布以来,各个领域的从业者都在探索 ChatGPT 的应用前景,挖掘它的潜力。其中,学术文本的理解与编辑是一种极具挑战性的应用场景,因为学术文本需要较高的专业性、严谨性等,有时还需要处理公式、代码、图谱等特殊的内容格式。现在,一个名为「ChatGPT 学术优化(chatgpt_academic)」的新项目在 GitHub 上爆火,上线几天就在 GitHub 上狂揽上万 Star。项目地址:https://github.com/
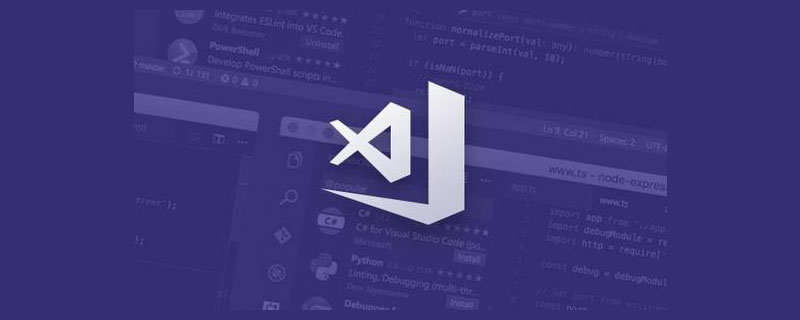
面对一夜爆火的 ChatGPT ,我最终也没抵得住诱惑,决定体验一下,不过这玩意要注册需要外国手机号以及科学上网,将许多人拦在门外,本篇博客将体验当下爆火的 ChatGPT 以及无需注册和科学上网,拿来即用的 ChatGPT 使用攻略,快来试试吧!
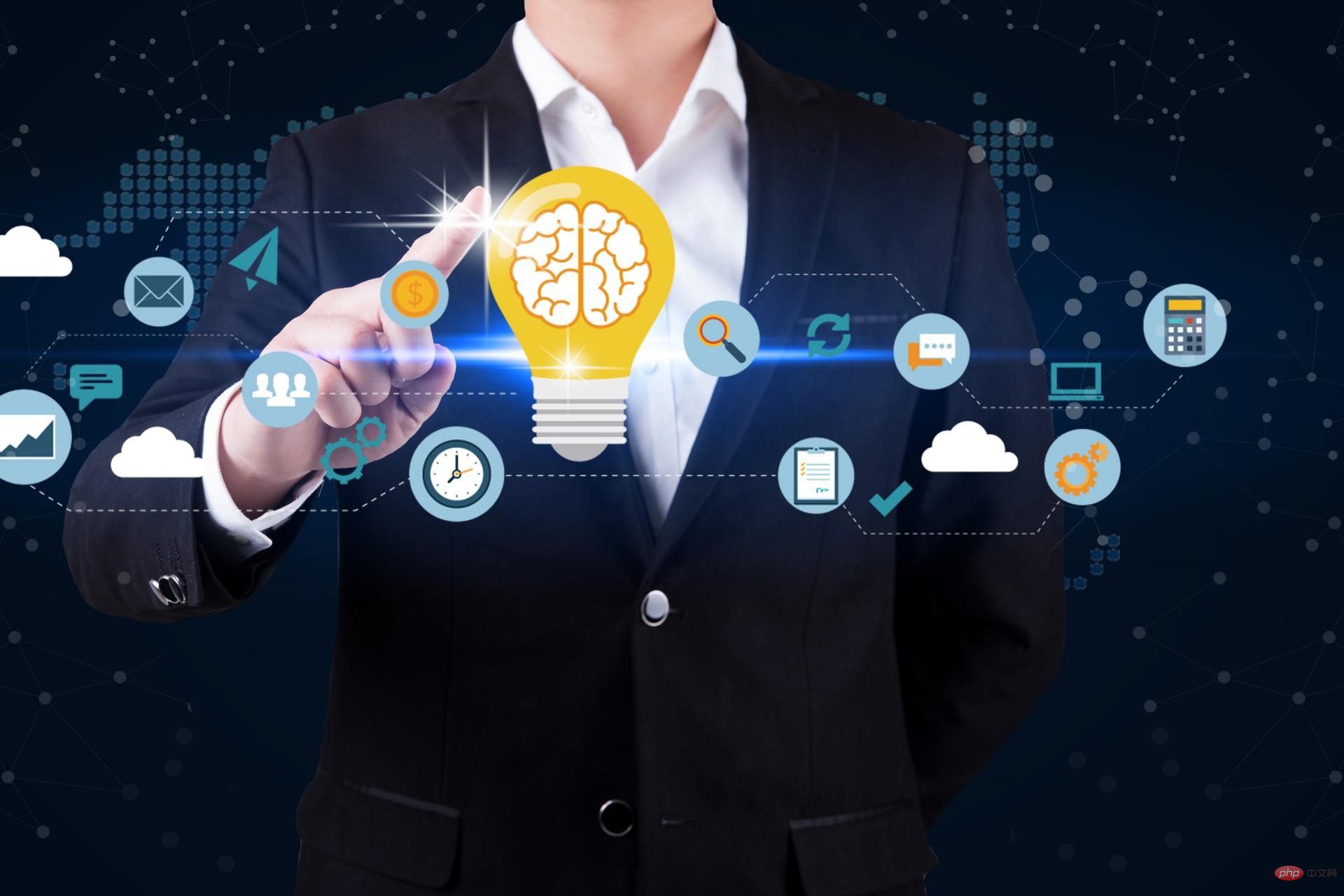
阅读论文可以说是我们的日常工作之一,论文的数量太多,我们如何快速阅读归纳呢?自从ChatGPT出现以后,有很多阅读论文的服务可以使用。其实使用ChatGPT API非常简单,我们只用30行python代码就可以在本地搭建一个自己的应用。 阅读论文可以说是我们的日常工作之一,论文的数量太多,我们如何快速阅读归纳呢?自从ChatGPT出现以后,有很多阅读论文的服务可以使用。其实使用ChatGPT API非常简单,我们只用30行python代码就可以在本地搭建一个自己的应用。使用 Python 和 C
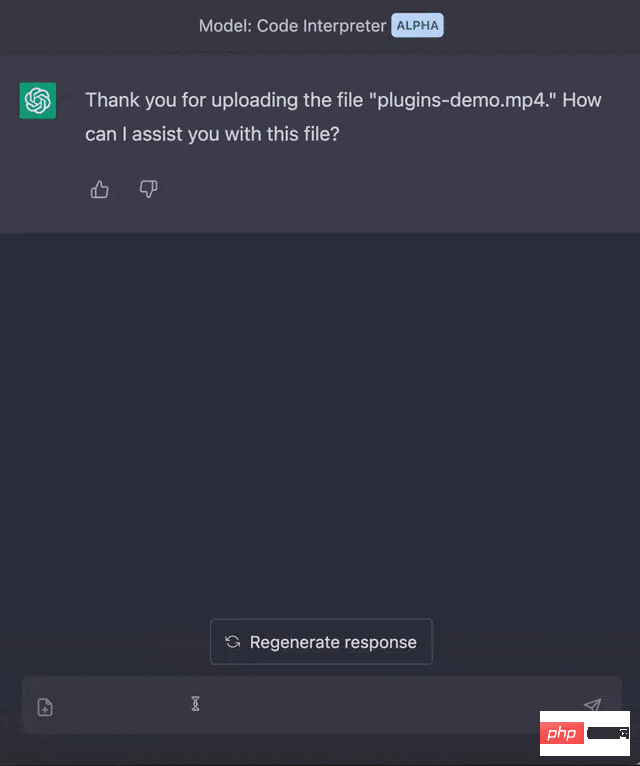
ChatGPT可以联网后,OpenAI还火速介绍了一款代码生成器,在这个插件的加持下,ChatGPT甚至可以自己生成机器学习模型了。 上周五,OpenAI刚刚宣布了惊爆的消息,ChatGPT可以联网,接入第三方插件了!而除了第三方插件,OpenAI也介绍了一款自家的插件「代码解释器」,并给出了几个特别的用例:解决定量和定性的数学问题;进行数据分析和可视化;快速转换文件格式。此外,Greg Brockman演示了ChatGPT还可以对上传视频文件进行处理。而一位叫Andrew Mayne的畅销作
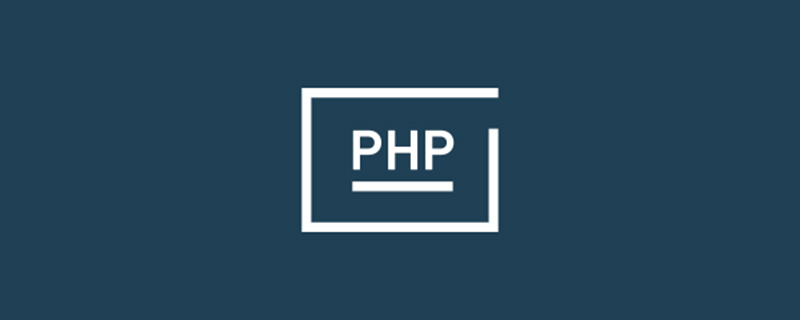
本篇文章给大家带来了关于php的相关知识,其中主要介绍了我是怎么用ChatGPT学习PHP中AOP的实现,感兴趣的朋友下面一起来看一下吧,希望对大家有帮助。


Hot AI Tools

Undresser.AI Undress
AI-powered app for creating realistic nude photos

AI Clothes Remover
Online AI tool for removing clothes from photos.

Undress AI Tool
Undress images for free

Clothoff.io
AI clothes remover

AI Hentai Generator
Generate AI Hentai for free.

Hot Article

Hot Tools

PhpStorm Mac version
The latest (2018.2.1) professional PHP integrated development tool

DVWA
Damn Vulnerable Web App (DVWA) is a PHP/MySQL web application that is very vulnerable. Its main goals are to be an aid for security professionals to test their skills and tools in a legal environment, to help web developers better understand the process of securing web applications, and to help teachers/students teach/learn in a classroom environment Web application security. The goal of DVWA is to practice some of the most common web vulnerabilities through a simple and straightforward interface, with varying degrees of difficulty. Please note that this software

SecLists
SecLists is the ultimate security tester's companion. It is a collection of various types of lists that are frequently used during security assessments, all in one place. SecLists helps make security testing more efficient and productive by conveniently providing all the lists a security tester might need. List types include usernames, passwords, URLs, fuzzing payloads, sensitive data patterns, web shells, and more. The tester can simply pull this repository onto a new test machine and he will have access to every type of list he needs.

Safe Exam Browser
Safe Exam Browser is a secure browser environment for taking online exams securely. This software turns any computer into a secure workstation. It controls access to any utility and prevents students from using unauthorized resources.

MinGW - Minimalist GNU for Windows
This project is in the process of being migrated to osdn.net/projects/mingw, you can continue to follow us there. MinGW: A native Windows port of the GNU Compiler Collection (GCC), freely distributable import libraries and header files for building native Windows applications; includes extensions to the MSVC runtime to support C99 functionality. All MinGW software can run on 64-bit Windows platforms.
