


When GPT-4 reflected on its mistake: performance increased by nearly 30%, and programming ability increased by 21%
GPT-4’s way of thinking is becoming more and more human-like.
When humans make mistakes, they will reflect on their behavior to avoid making mistakes again. If large language models such as GPT-4 also have the ability to reflect, the performance will be improved by how much.
It is well known that large language models (LLMs) have demonstrated unprecedented performance on a variety of tasks. However, these SOTA methods usually require model fine-tuning, policy optimization and other operations on the defined state space. Due to the lack of high-quality training data and well-defined state space, it is still difficult to implement an optimized model. Furthermore, models do not yet possess certain qualities inherent to the human decision-making process, particularly the ability to learn from mistakes.
But now, in a recent paper, researchers from Northeastern University, MIT and other institutions proposed Reflexion, which gives the agent the ability to dynamically remember and self-reflect.
In order to verify the effectiveness of the method, this study evaluated the agent's ability to complete decision-making tasks in the AlfWorld environment, and to complete knowledge-intensive, search-based question and answer tasks in the HotPotQA environment. The mission success rates were 97% and 51% respectively.
Paper address: https://arxiv.org/pdf/2303.11366.pdf
Project address: https://github.com/GammaTauAI/ reflexion-human-eval
As shown in the figure below, in the AlfWorld environment, various items are placed in the room, and the agent is required to give a reasoning plan to get a certain object. The upper part of the figure below is due to The agent failed due to inefficient planning. After reflection, the agent realizes the error, corrects the reasoning trajectory, and gives a concise trajectory method (lower part of the figure).
Model Rethinking Flawed Search Strategies:
This paper shows that you can do this by requiring GPT-4 Reflect on "Why were you wrong?" and generate a new prompt for yourself, taking the reason for the error into account until the result is correct, thereby improving the performance of GPT-4 by an astonishing 30%.
Netizens can’t help but sigh: The development speed of artificial intelligence has exceeded our ability to adapt.
Method Introduction
The overall architecture of the Reflexion agent is shown in Figure 1 below, where Reflexion utilizes ReAct (Yao et al., 2023). In the first trial, the agent is given a task from the environment that constitutes the initial query, and then the agent performs a sequence of actions generated by the LLM and receives observations and rewards from the environment. For environments that offer descriptive or continuous rewards, the study limits the output to simple binary success states to ensure applicability.
After each action a_t, the agent will calculate a heuristic function h, as shown in the figure below
This heuristic function is designed to detect information illusion (i.e. false or wrong information) or inefficiency of the agent, and "tell" the agent when it needs to reflect (reflexion), where t is the time step, s_t is the current state, Ω represents the number of repeated action cycles, ε represents the maximum total number of executed actions, [a_o, o_0 . . . , a_(t−1), o_(t−1)] represents the trajectory history. repeat is a simple function that determines the number of times a loop of repeated actions produces the same result.
If function h tells the agent that reflection is needed, the agent queries the LLM to reflect its current task, trajectory history, and last reward, and then the agent resets the environment and tries again on subsequent trials. If function h does not tell the agent that reflection is needed, then the agent adds a_t and o_t to its trajectory history and queries the LLM for the next action.
If heuristic h recommends reflection at time step t, the agent will reflect based on its current state s_t, last reward r_t, previous actions and observations [a_0, o_0, . . . , a_t, o_t], and the agent's existing work storage mem, start a reflection process.
The purpose of reflection is to help the agent correct "illusions" and inefficiencies through repeated trials. The model used for reflection is an LLM that uses specific failure trajectories and ideal reflection examples to prompt.
The agent will iteratively carry out the above reflection process. In the experiments, the study set the number of reflections stored in the agent's memory to a maximum of 3 times in order to avoid queries exceeding the limits of the LLM. The run will terminate in the following situations:
- The maximum number of trials is exceeded;
- Failure to improve performance between two consecutive trials;
- Complete the task.
Experiments and results
AlfWorld provides six different tasks and more than 3,000 environments. These tasks require the agent to understand the target task, formulate a sequential plan for subtasks, and perform Perform operations in a given environment.
The study tested the agent in 134 AlfWorld environments, with tasks including finding hidden objects (for example, finding a fruit knife in a drawer), moving objects (for example, moving a knife to a cutting board), and using other object to manipulate another object (for example, refrigerating tomatoes in a refrigerator).
Without reflection, the accuracy of the agent was 63%, and Reflexion was added for comparison. The results showed that the agent was able to handle 97% of the environment in 12 trials and failed to solve only 4 out of 134 tasks.
The next experiment was conducted in HotPotQA, which is a data set based on Wikipedia and contains 113k question and answer pairs. It is mainly used to challenge the agent to parse content. and the ability to reason.
On HotpotQA's 100 question-answer pair tests, the study compared the base agent and the Reflexion-based agent until they failed to improve accuracy over successive trials. The results show that the performance of the basic agent has not improved. In the first trial, the accuracy of the basic agent was 34% and the accuracy of the Reflexion agent was 32%. However, after 7 trials, the performance of the Reflexion agent improved significantly. The improvement is close to 30%, which is much better than the basic agent.
Similarly, when testing the model's ability to write code, GPT-4 with Reflexion is also significantly better than regular GPT-4:
The above is the detailed content of When GPT-4 reflected on its mistake: performance increased by nearly 30%, and programming ability increased by 21%. For more information, please follow other related articles on the PHP Chinese website!
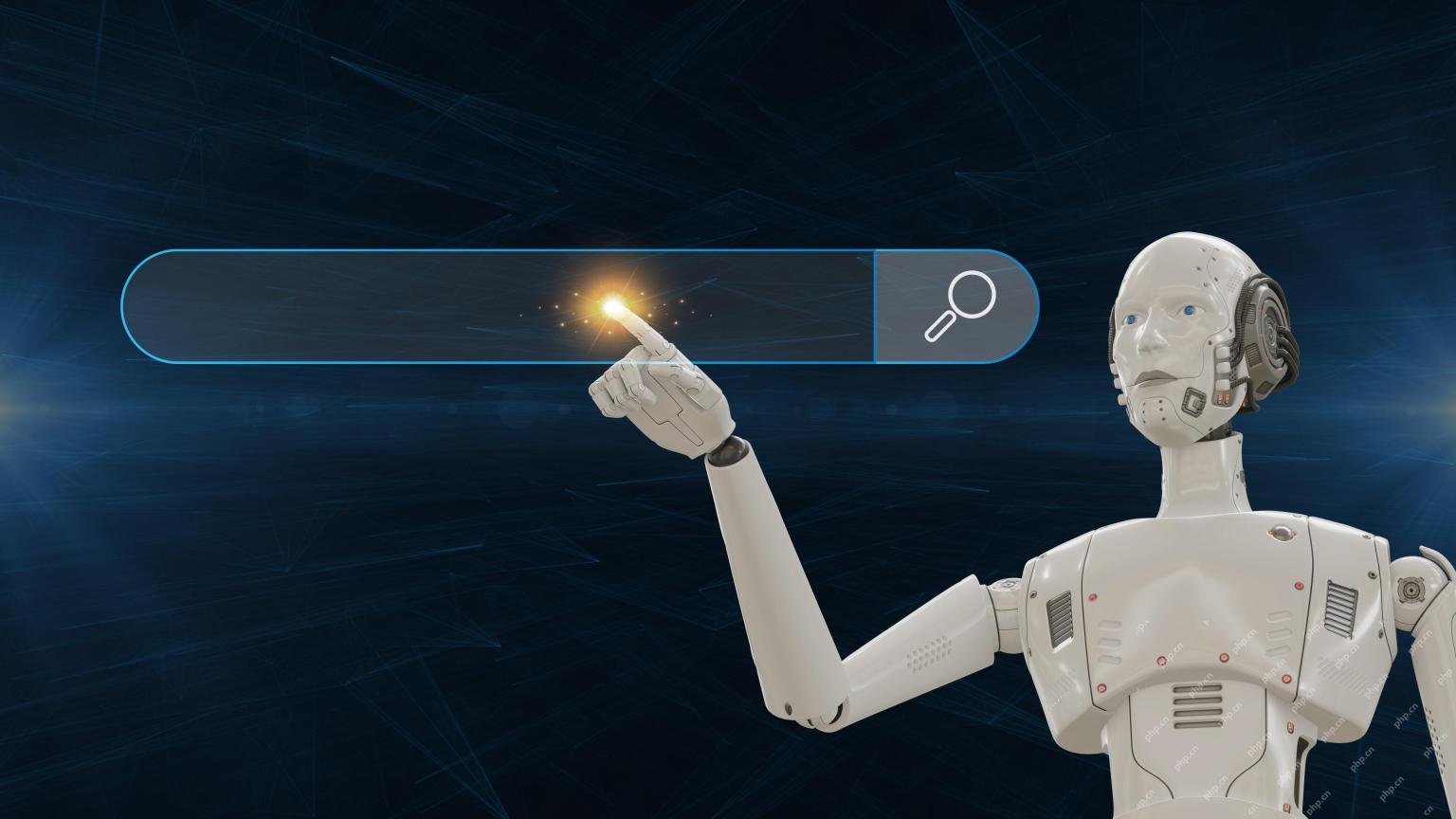
Recent research has shown that AI Overviews can cause a whopping 15-64% decline in organic traffic, based on industry and search type. This radical change is causing marketers to reconsider their whole strategy regarding digital visibility. The New
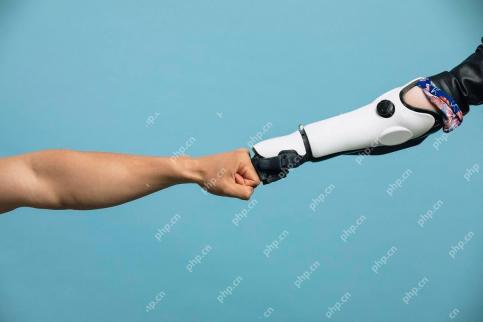
A recent report from Elon University’s Imagining The Digital Future Center surveyed nearly 300 global technology experts. The resulting report, ‘Being Human in 2035’, concluded that most are concerned that the deepening adoption of AI systems over t
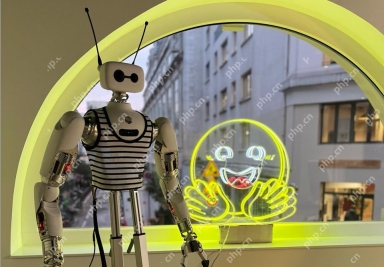
“Super happy to announce that we are acquiring Pollen Robotics to bring open-source robots to the world,” Hugging Face said on X. “Since Remi Cadene joined us from Tesla, we’ve become the most widely used software platform for open robotics thanks to
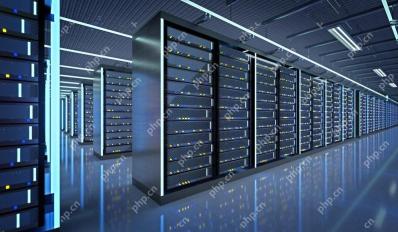
And before that happens, societies have to look more closely at the issue. First of all, we have to define human content, and bring a broadness to that category of information. You have creative works like songs, and poems and pieces of visual art.
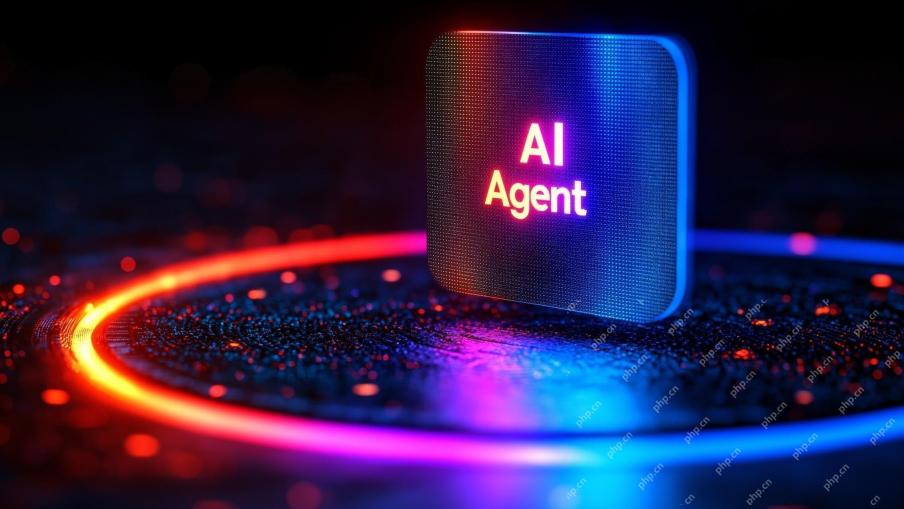
This will change a lot of things as we become able to delegate more and more tasks to machines. By connecting with external applications, agents can take care of shopping, scheduling, managing travel, and many of our day-to-day interactions with digi
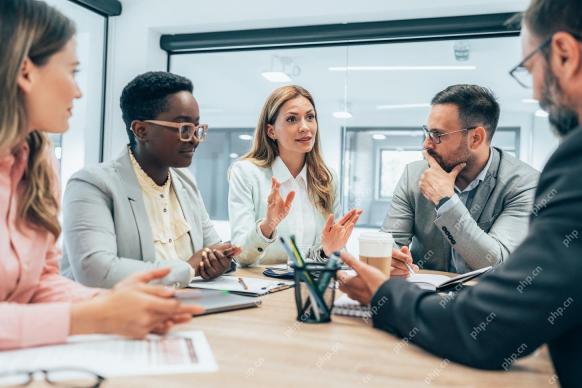
Let’s talk about it. This analysis of an innovative AI breakthrough is part of my ongoing Forbes column coverage on the latest in AI, including identifying and explaining various impactful AI complexities (see the link here). EU Makes Bold AI Procl
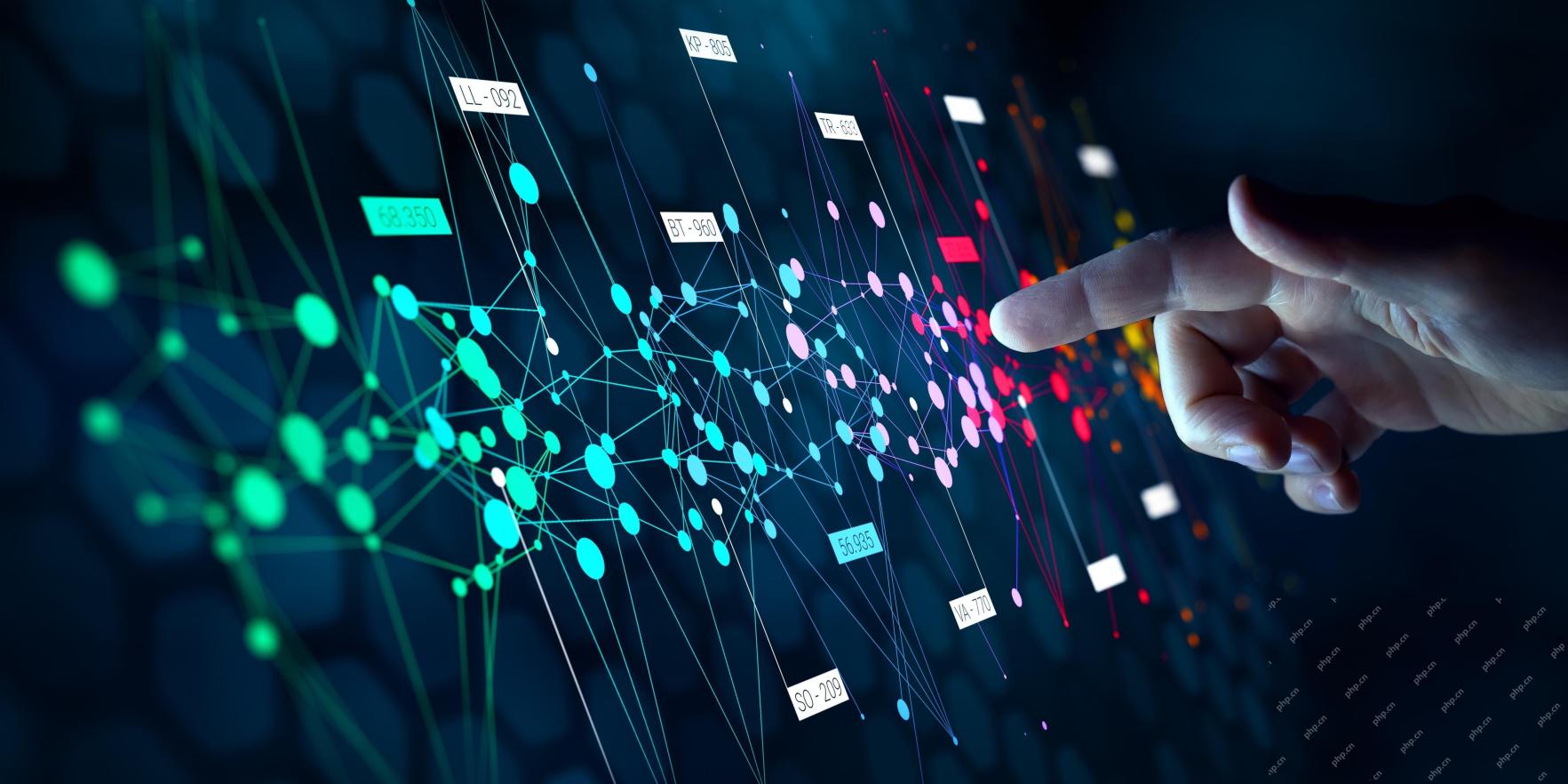
But that’s changing—thanks in large part to a fundamental shift in how we interpret and respond to risk. The Cloud Visibility Gap Is a Threat Vector in Itself Hybrid and multi-cloud environments have become the new normal. Organizations run workloa
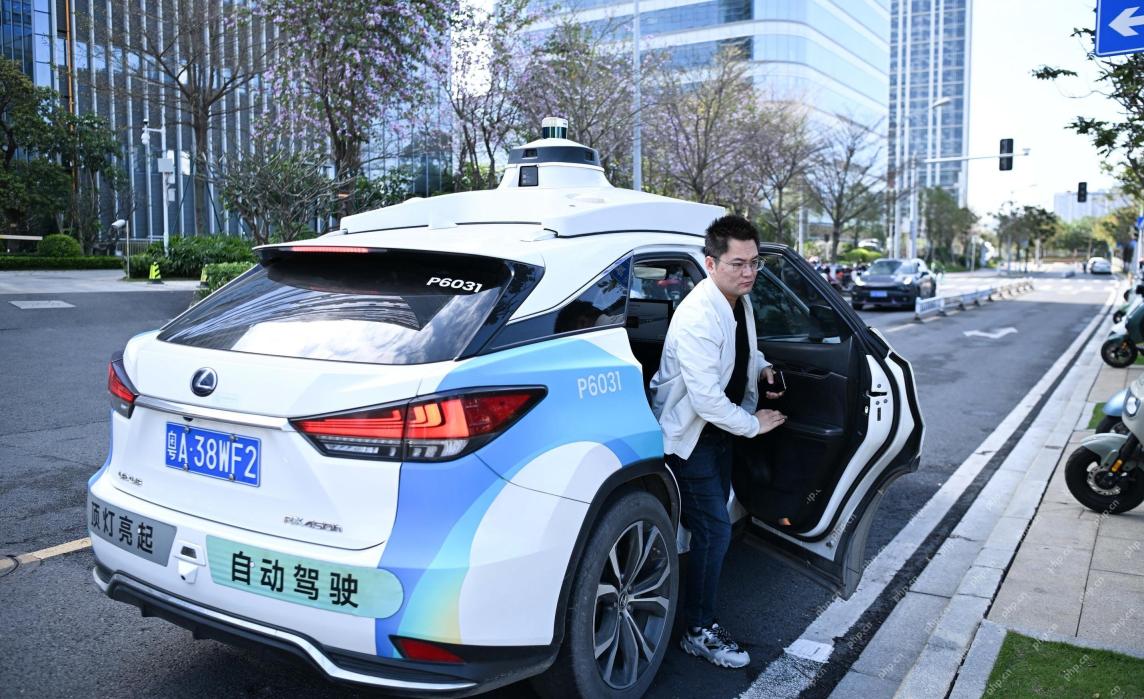
A recent session at last week’s Ride AI conference in Los Angeles revealed some details about the different regulatory regime in China, and featured a report from a Chinese-American Youtuber who has taken on a mission to ride in the different vehicle


Hot AI Tools

Undresser.AI Undress
AI-powered app for creating realistic nude photos

AI Clothes Remover
Online AI tool for removing clothes from photos.

Undress AI Tool
Undress images for free

Clothoff.io
AI clothes remover

AI Hentai Generator
Generate AI Hentai for free.

Hot Article

Hot Tools

SublimeText3 Chinese version
Chinese version, very easy to use

SAP NetWeaver Server Adapter for Eclipse
Integrate Eclipse with SAP NetWeaver application server.
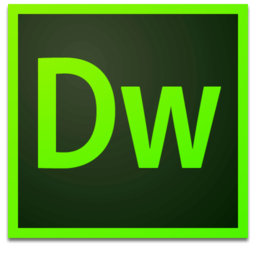
Dreamweaver Mac version
Visual web development tools

Safe Exam Browser
Safe Exam Browser is a secure browser environment for taking online exams securely. This software turns any computer into a secure workstation. It controls access to any utility and prevents students from using unauthorized resources.

MinGW - Minimalist GNU for Windows
This project is in the process of being migrated to osdn.net/projects/mingw, you can continue to follow us there. MinGW: A native Windows port of the GNU Compiler Collection (GCC), freely distributable import libraries and header files for building native Windows applications; includes extensions to the MSVC runtime to support C99 functionality. All MinGW software can run on 64-bit Windows platforms.