


Currently, this paper has been accepted by CVPR2023.
GPT-4, which can read images, is shockingly released! But you have to queue up to use it. . .
Why not try this first~
Add a small model, you can make large language models such as ChatGPT and GPT-3 that can only understand text easilyRead pictures, all kinds of tricky details can be dealt with easily.
And train this small modelIt can be done with a single card (an RTX 3090).
As for the effect, just look at the picture.
For example, input a picture of a "music scene" to the trained GPT-3 and ask it: What activities are being held at the scene?
Without any hesitation, GPT-3 gave the answer to Concert.
To make it more difficult, give GPT-3 a photo of Jiang Zi and let it identify what type of material the curtain in the photo is.
GPT-3: lace.
Bingo! (It seems that there is something on it)
This method is the latest result of a team from Hangzhou University of Electronic Science and Technology and Hefei University of Technology: Prophet, which they had already developed half a year ago Get to work on this.
The first author of the paper is Shao Zhenwei, a graduate student of Hangzhou Dianzi University. He was diagnosed with "progressive spinal muscular atrophy" when he was 1 year old. He regretted not passing Zhejiang University during the college entrance examination and chose Hangzhou Dianzi University, which is close to home. .
This paper has been accepted by CVPR2023.
Reaching the new SOTA in cross-modal tasks
Without further ado, let’s look directly at the reading of GPT-3 with the support of Prophet’s method. Figure ability.
Let’s first take a look at its test results on the data set.
The research team tested Prophet on two visual question and answer data sets based on external knowledge, OK-VQA and A-OKVQA, and both created new SOTA.
More specifically, on the OK-VQA data set, compared with Deepmind’s large model Flamingo with 80B parameters, Prophet reached Achieved an accuracy rate of 61.1%, successfully defeating Flamingo (57.8%).
And in terms of required computing power resources, Prophet also "beats" Flamingo.
Flamingo-80B needs to be trained on 1536 TPUv4 graphics cards for 15 days, while Prophet only requires one RTX-3090 graphics card to train the VQA model 4 days, then call the OpenAI API a certain number of times.
In fact, there have been methods similar to Prophet to help GPT-3 handle cross-modal tasks before, such as PICa, and later KAT and REVIVE.
However, they may not be satisfactory in handling some details.
Give a chestnut, let them read the picture below together, and then answer the question: What kind of fruit will the tree in the picture bear?
The only information that PICa, KAT and REVIVE extracted from the picture was: a group of people walking in the square, completely ignoring that there was a coconut tree behind. The final answer can only be guessed.
This situation will not happen with Prophet. It solves the problem of insufficient image information extracted by the above method and further stimulates the potential of GPT-3.
So how did Prophet do it?
Small model Big model
Effectively extract information and accurately answer questions. Prophet relies on its unique two-stage framework to be able to do this.
The division of labor between these two stages is also clear:
- The first stage: Give some enlightening answers based on the questions;
- The second stage: These answers will narrow the scope, giving GPT-3 sufficient space to realize its potential.
First, in the first stage, the research team trained an improved MCAN model (a VQA model) against a specific external knowledge VQA data set.
After training the model, extract two heuristic answers from it: answer candidates and answer-aware examples.
Among them, Answer candidates are sorted based on the confidence level output by the model classification layer, and the top10 are selected.
Answer perception example refers to using the features before the model classification layer as the potential answer features of the sample, the most similar labeled sample in this feature space.
The next step is the second stage, which is relatively simple and crude.
Organize the "inspiring answers" obtained in the previous step into prompts, and then input the prompts to GPT-3 to complete visual question and answer questions under certain prompts.
However, although some answer hints have been given in the previous step, this does not mean that GPT-3 will be limited to these answers.
If the confidence of the answer given by the prompt is too low or the correct answer is not among those prompts, it is entirely possible for GPT-3 to generate a new answer.
Research Team
Of course, in addition to the research results, the team behind this study also has to be mentioned.
First authorShao Zhenwei was diagnosed with "progressive spinal muscular atrophy" when he was 1 year old. It is a first-level physical disability and has no ability to take care of itself. Life and study require the full care of the mother. .
However, despite his physical limitations, Shao Zhenwei’s thirst for knowledge has not weakened.
In the 2017 college entrance examination, he scored a high score of 644 points and was admitted to the computer major of Hangzhou University of Electronic Science and Technology with the first place.
During this period, he also won honors such as the 2018 Chinese College Student Self-improvement Star, the 2020 National Scholarship, and the 2021 Zhejiang Province Outstanding Graduate.
During his undergraduate period, Shao Zhenwei had already started to conduct scientific research activities with Professor Yu Zhou.
In 2021, Shao Zhenwei had a chance encounter with Zhejiang University when he was preparing for postgraduate promotion, so he stayed at the school and joined Professor Yu Zhou’s research group to pursue a master’s degree. Currently, he is in the second year of graduate school, and his research direction is cross-modal learning.
Professor Yu Zhou is the second author and corresponding author of this research paper. He is the youngest professor at the School of Computer Science of Hangzhou Dianping University and a member of the “Complex System Modeling and Simulation” Laboratory of the Ministry of Education. deputy director.
For a long time, Yu Zhou has specialized in the multimodal intelligence direction, and has led the research team to win the championship and runner-up in the international visual question answering challenge VQA Challenge many times.
Most members of the research team are in Hangzhou Electronics Media Intelligence Laboratory (MIL).
The laboratory is headed by Professor Yu Jun, a National Distinguished Talent. In recent years, the laboratory has published a series of high-level journal conference papers (TPAMI, IJCV, CVPR, etc.) focusing on multi-modal learning, and has won many IEEE journal awards. Best paper award at the conference.
The laboratory has hosted more than 20 national projects such as the National Key R&D Plan and the National Natural Science Foundation of China. It has won the first prize of Zhejiang Province Natural Science Award and the second prize of Educational Natural Science Award.
The above is the detailed content of The guy from Hangzhou Electronics is the first to get the GPT image reading function. A single card can realize the new SOTA. The code has been open source. For more information, please follow other related articles on the PHP Chinese website!
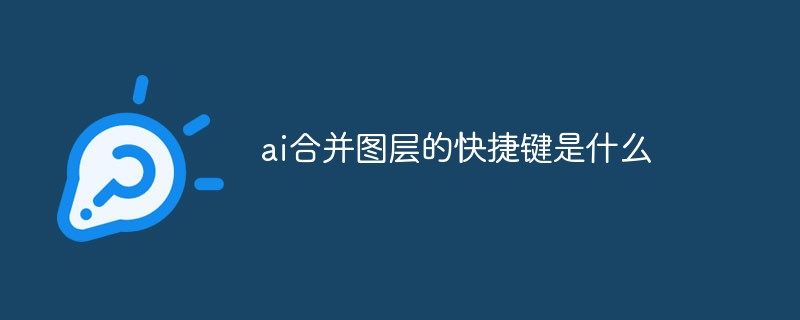
ai合并图层的快捷键是“Ctrl+Shift+E”,它的作用是把目前所有处在显示状态的图层合并,在隐藏状态的图层则不作变动。也可以选中要合并的图层,在菜单栏中依次点击“窗口”-“路径查找器”,点击“合并”按钮。
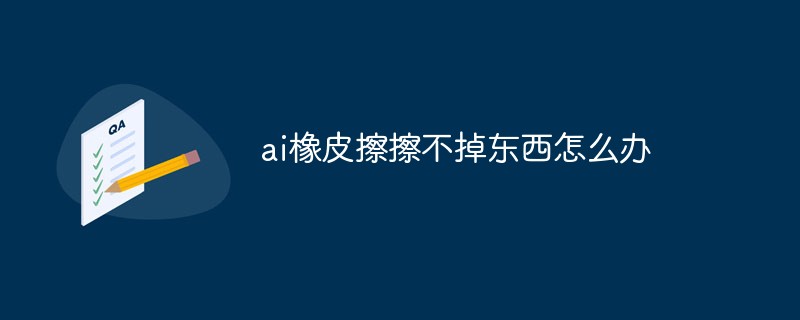
ai橡皮擦擦不掉东西是因为AI是矢量图软件,用橡皮擦不能擦位图的,其解决办法就是用蒙板工具以及钢笔勾好路径再建立蒙板即可实现擦掉东西。
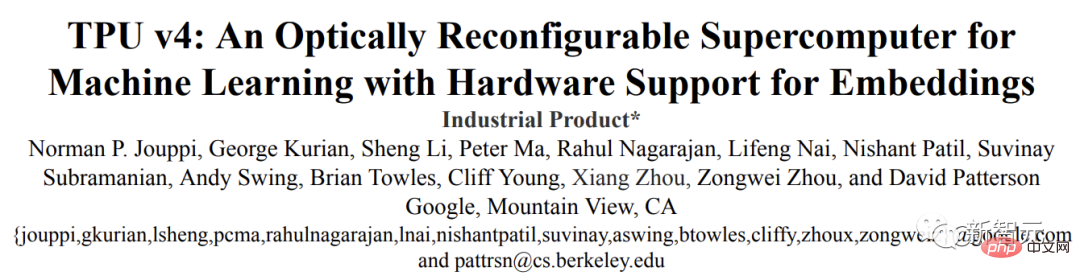
虽然谷歌早在2020年,就在自家的数据中心上部署了当时最强的AI芯片——TPU v4。但直到今年的4月4日,谷歌才首次公布了这台AI超算的技术细节。论文地址:https://arxiv.org/abs/2304.01433相比于TPU v3,TPU v4的性能要高出2.1倍,而在整合4096个芯片之后,超算的性能更是提升了10倍。另外,谷歌还声称,自家芯片要比英伟达A100更快、更节能。与A100对打,速度快1.7倍论文中,谷歌表示,对于规模相当的系统,TPU v4可以提供比英伟达A100强1.
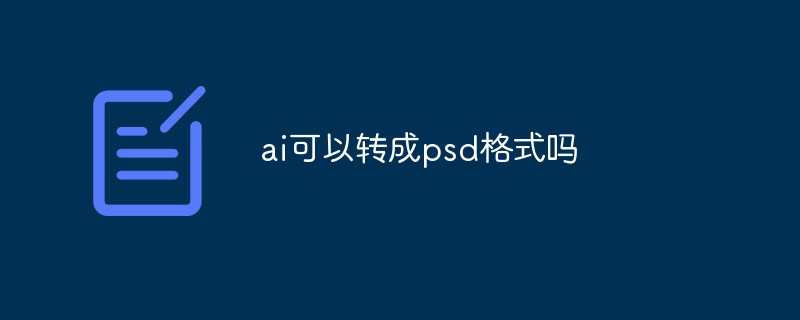
ai可以转成psd格式。转换方法:1、打开Adobe Illustrator软件,依次点击顶部菜单栏的“文件”-“打开”,选择所需的ai文件;2、点击右侧功能面板中的“图层”,点击三杠图标,在弹出的选项中选择“释放到图层(顺序)”;3、依次点击顶部菜单栏的“文件”-“导出”-“导出为”;4、在弹出的“导出”对话框中,将“保存类型”设置为“PSD格式”,点击“导出”即可;
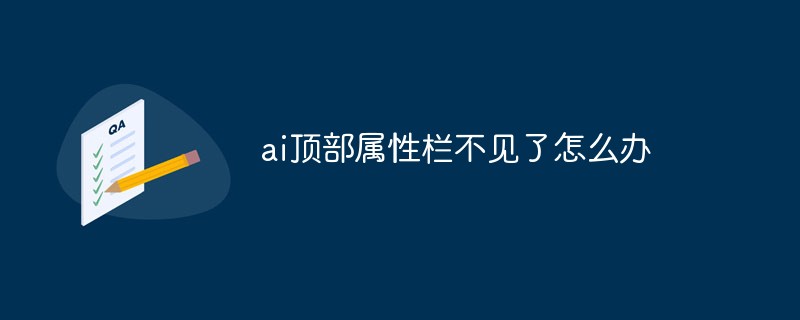
ai顶部属性栏不见了的解决办法:1、开启Ai新建画布,进入绘图页面;2、在Ai顶部菜单栏中点击“窗口”;3、在系统弹出的窗口菜单页面中点击“控制”,然后开启“控制”窗口即可显示出属性栏。
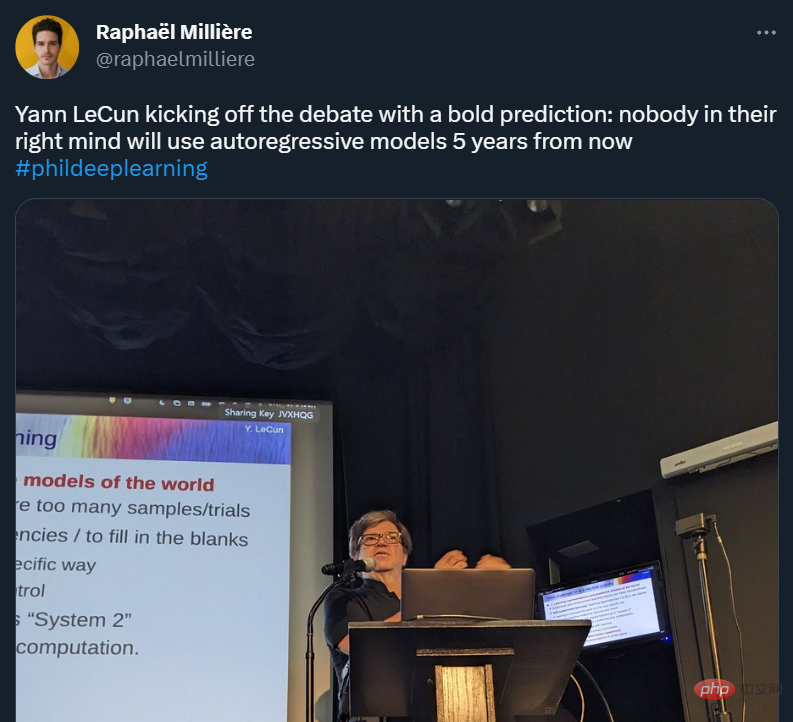
Yann LeCun 这个观点的确有些大胆。 「从现在起 5 年内,没有哪个头脑正常的人会使用自回归模型。」最近,图灵奖得主 Yann LeCun 给一场辩论做了个特别的开场。而他口中的自回归,正是当前爆红的 GPT 家族模型所依赖的学习范式。当然,被 Yann LeCun 指出问题的不只是自回归模型。在他看来,当前整个的机器学习领域都面临巨大挑战。这场辩论的主题为「Do large language models need sensory grounding for meaning and u
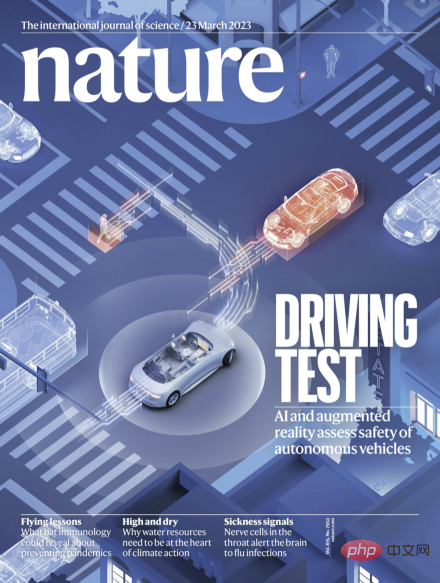
引入密集强化学习,用 AI 验证 AI。 自动驾驶汽车 (AV) 技术的快速发展,使得我们正处于交通革命的风口浪尖,其规模是自一个世纪前汽车问世以来从未见过的。自动驾驶技术具有显着提高交通安全性、机动性和可持续性的潜力,因此引起了工业界、政府机构、专业组织和学术机构的共同关注。过去 20 年里,自动驾驶汽车的发展取得了长足的进步,尤其是随着深度学习的出现更是如此。到 2015 年,开始有公司宣布他们将在 2020 之前量产 AV。不过到目前为止,并且没有 level 4 级别的 AV 可以在市场
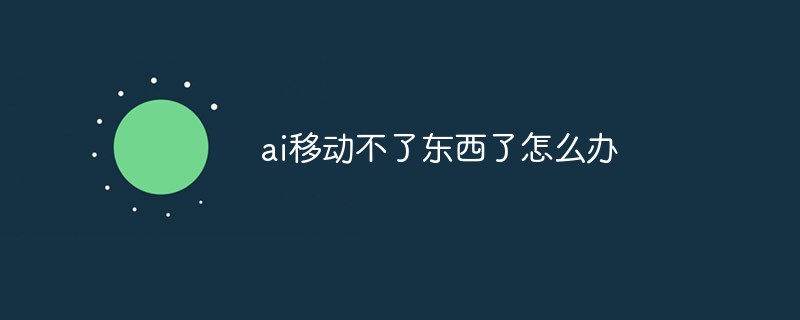
ai移动不了东西的解决办法:1、打开ai软件,打开空白文档;2、选择矩形工具,在文档中绘制矩形;3、点击选择工具,移动文档中的矩形;4、点击图层按钮,弹出图层面板对话框,解锁图层;5、点击选择工具,移动矩形即可。


Hot AI Tools

Undresser.AI Undress
AI-powered app for creating realistic nude photos

AI Clothes Remover
Online AI tool for removing clothes from photos.

Undress AI Tool
Undress images for free

Clothoff.io
AI clothes remover

AI Hentai Generator
Generate AI Hentai for free.

Hot Article

Hot Tools
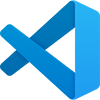
VSCode Windows 64-bit Download
A free and powerful IDE editor launched by Microsoft
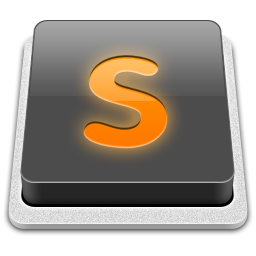
SublimeText3 Mac version
God-level code editing software (SublimeText3)

Zend Studio 13.0.1
Powerful PHP integrated development environment

mPDF
mPDF is a PHP library that can generate PDF files from UTF-8 encoded HTML. The original author, Ian Back, wrote mPDF to output PDF files "on the fly" from his website and handle different languages. It is slower than original scripts like HTML2FPDF and produces larger files when using Unicode fonts, but supports CSS styles etc. and has a lot of enhancements. Supports almost all languages, including RTL (Arabic and Hebrew) and CJK (Chinese, Japanese and Korean). Supports nested block-level elements (such as P, DIV),

SAP NetWeaver Server Adapter for Eclipse
Integrate Eclipse with SAP NetWeaver application server.
