This article brings you relevant knowledge about mysql, mainly introducing the elegant solution to the mysql deep paging problem. This article will discuss how to optimize deep paging when the mysql table has a large amount of data. Pagination problem, and attached is the pseudo code of a recent case of optimizing slow SQL problem. I hope it will be helpful to everyone.
Recommended learning: mysql video tutorial
In the daily demand development process, I believe everyone will be familiar with limit, but using limit, when the offset (offset) is very large, you will find that the query efficiency is getting slower and slower. When the limit is 2000 at the beginning, it may take 200ms to query the required data. However, when the limit is 4000 offset 100000, you will find that its query efficiency already requires about 1S. If it is larger, it will only get worse and worse. slow.
Summary
This article will discuss how to optimize the deep paging problem when the mysql table has a large amount of data, and attach the pseudo code of a recent case of optimizing the slow sql problem.
1. Limit deep paging problem description
Let’s take a look at the table structure first (just give an example, the table structure is incomplete and useless fields will not be displayed)
CREATE TABLE `p2p_detail_record` ( `id` varchar(32) COLLATE utf8mb4_bin NOT NULL DEFAULT '' COMMENT '主键', `batch_num` int NOT NULL DEFAULT '0' COMMENT '上报数量', `uptime` bigint NOT NULL DEFAULT '0' COMMENT '上报时间', `uuid` varchar(64) COLLATE utf8mb4_bin NOT NULL DEFAULT '' COMMENT '会议id', `start_time_stamp` bigint NOT NULL DEFAULT '0' COMMENT '开始时间', `answer_time_stamp` bigint NOT NULL DEFAULT '0' COMMENT '应答时间', `end_time_stamp` bigint NOT NULL DEFAULT '0' COMMENT '结束时间', `duration` int NOT NULL DEFAULT '0' COMMENT '持续时间', PRIMARY KEY (`id`), KEY `idx_uuid` (`uuid`), KEY `idx_start_time_stamp` (`start_time_stamp`) //索引, ) ENGINE=InnoDB DEFAULT CHARSET=utf8mb4 COLLATE=utf8mb4_bin COMMENT='p2p通话记录详情表';
Suppose the deep paging SQL we want to query looks like this
select * from p2p_detail_record ppdr where ppdr .start_time_stamp >1656666798000 limit 0,2000
The query efficiency is 94ms. Is it very fast? So if we limit 100000 or 2000, the query efficiency is 1.5S, which is already very slow. What if there are more?
Clustered index: Leaf nodes store the entire row of data.
Non-clustered index: The leaf node stores the primary key value corresponding to the entire row of data.
The process of using non-clustered index query
- Find the corresponding leaf node through the non-clustered index tree , get the value of the primary key.
- Then get the value of the primary key and return to the
- clustered index tree to find the corresponding entire row of data. (The whole process is called table return)
limit 100000,10 will scan 100010 rows, while
limit 0,10 will only scan 10 rows. Here we need to return to the table 100010 times, and a lot of time is spent on returning the table.
Core idea of the solution: Can we know in advance which primary key ID to start from, so as to reduce the number of table returns?
Common solutionsThrough subqueries Optimizationselect *
from p2p_detail_record ppdr
where id >= (select id from p2p_detail_record ppdr2 where ppdr2 .start_time_stamp >1656666798000 limit 100000,1)
limit 2000
The same query result is also the 2000th item starting from 10W. The query efficiency is 200ms, which is much faster.
Tag recording method: In fact, mark which one was queried last time, and check again next time When the time comes, start scanning from this bar down. Similar to the effect of bookmarks
select * from p2p_detail_record ppdr where ppdr.id > 'bb9d67ee6eac4cab9909bad7c98f54d4' order by id limit 2000 备注:bb9d67ee6eac4cab9909bad7c98f54d4是上次查询结果的最后一条IDIf you use the tag recording method, the performance will be good because the
id index is hit. But this method has several
disadvantages.
- 1. It can only be queried on consecutive pages, not across pages.
- 2. A field similar to
- continuous auto-increment is needed (orber by id can be used).
- Using
- through subquery optimization
Advantages: You can query across pages, and you can check the data on whichever page you want to check.
Disadvantages: is not as efficient as tag recording method. Reason: For example, after you need to check 100,000 pieces of data, you also need to query the 1000th piece of data corresponding to the non-clustered index first, and then get the ID starting from the 100,000th piece for query.
- Use
- tag recording method
Advantages: The query efficiency is very stable and very fast.
shortcoming:
- 不跨页查询,
- 需要一种类似连续自增的字段
关于第二点的说明: 该点一般都好解决,可使用任意不重复的字段进行排序即可。若使用可能重复的字段进行排序的字段,由于mysql对于相同值的字段排序是无序,导致如果正好在分页时,上下页中可能存在相同的数据。
实战案例
需求: 需要查询查询某一时间段的数据量,假设有几十万的数据量需要查询出来,进行某些操作。
需求分析 1、分批查询(分页查询),设计深分页问题,导致效率较慢。
CREATE TABLE `p2p_detail_record` ( `id` varchar(32) COLLATE utf8mb4_bin NOT NULL DEFAULT '' COMMENT '主键', `batch_num` int NOT NULL DEFAULT '0' COMMENT '上报数量', `uptime` bigint NOT NULL DEFAULT '0' COMMENT '上报时间', `uuid` varchar(64) COLLATE utf8mb4_bin NOT NULL DEFAULT '' COMMENT '会议id', `start_time_stamp` bigint NOT NULL DEFAULT '0' COMMENT '开始时间', `answer_time_stamp` bigint NOT NULL DEFAULT '0' COMMENT '应答时间', `end_time_stamp` bigint NOT NULL DEFAULT '0' COMMENT '结束时间', `duration` int NOT NULL DEFAULT '0' COMMENT '持续时间', PRIMARY KEY (`id`), KEY `idx_uuid` (`uuid`), KEY `idx_start_time_stamp` (`start_time_stamp`) //索引, ) ENGINE=InnoDB DEFAULT CHARSET=utf8mb4 COLLATE=utf8mb4_bin COMMENT='p2p通话记录详情表';
伪代码实现:
//最小ID String lastId = null; //一页的条数 Integer pageSize = 2000; List<P2pRecordVo> list ; do{ list = listP2pRecordByPage(lastId,pageSize); //标签记录法,记录上次查询过的Id lastId = list.get(list.size()-1).getId(); //获取上一次查询数据最后的ID,用于记录 //对数据的操作逻辑 XXXXX(); }while(isNotEmpty(list)); <select id ="listP2pRecordByPage"> select * from p2p_detail_record ppdr where 1=1 <if test = "lastId != null"> and ppdr.id > #{lastId} </if> order by id asc limit #{pageSize} </select>
这里有个小优化点: 可能有的人会先对所有数据排序一遍,拿到最小ID,但是这样对所有数据排序,然后去min(id),耗时也蛮长的,其实第一次查询,可不带lastId进行查询,查询结果也是一样。速度更快。
总结
1、当业务需要从表中查出大数据量时,而又项目架构没上ES时,可考虑使用标签记录法的方式,对查询效率进行优化。
2、从需求上也应该尽可能避免,在大数据量的情况下,分页查询最后一页的功能。或者限制成只能一页一页往后划的场景。
推荐学习:mysql视频教程
The above is the detailed content of How to solve mysql deep paging problem. For more information, please follow other related articles on the PHP Chinese website!
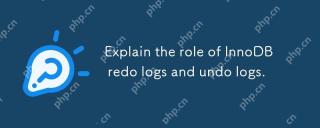
InnoDB uses redologs and undologs to ensure data consistency and reliability. 1.redologs record data page modification to ensure crash recovery and transaction persistence. 2.undologs records the original data value and supports transaction rollback and MVCC.
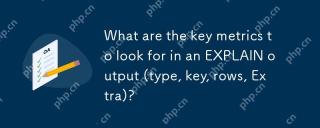
Key metrics for EXPLAIN commands include type, key, rows, and Extra. 1) The type reflects the access type of the query. The higher the value, the higher the efficiency, such as const is better than ALL. 2) The key displays the index used, and NULL indicates no index. 3) rows estimates the number of scanned rows, affecting query performance. 4) Extra provides additional information, such as Usingfilesort prompts that it needs to be optimized.
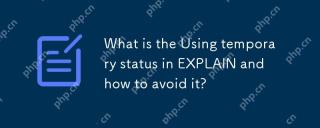
Usingtemporary indicates that the need to create temporary tables in MySQL queries, which are commonly found in ORDERBY using DISTINCT, GROUPBY, or non-indexed columns. You can avoid the occurrence of indexes and rewrite queries and improve query performance. Specifically, when Usingtemporary appears in EXPLAIN output, it means that MySQL needs to create temporary tables to handle queries. This usually occurs when: 1) deduplication or grouping when using DISTINCT or GROUPBY; 2) sort when ORDERBY contains non-index columns; 3) use complex subquery or join operations. Optimization methods include: 1) ORDERBY and GROUPB
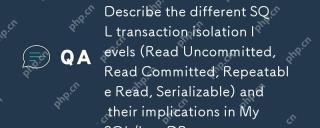
MySQL/InnoDB supports four transaction isolation levels: ReadUncommitted, ReadCommitted, RepeatableRead and Serializable. 1.ReadUncommitted allows reading of uncommitted data, which may cause dirty reading. 2. ReadCommitted avoids dirty reading, but non-repeatable reading may occur. 3.RepeatableRead is the default level, avoiding dirty reading and non-repeatable reading, but phantom reading may occur. 4. Serializable avoids all concurrency problems but reduces concurrency. Choosing the appropriate isolation level requires balancing data consistency and performance requirements.
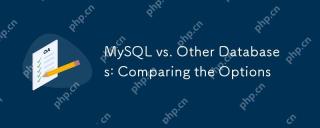
MySQL is suitable for web applications and content management systems and is popular for its open source, high performance and ease of use. 1) Compared with PostgreSQL, MySQL performs better in simple queries and high concurrent read operations. 2) Compared with Oracle, MySQL is more popular among small and medium-sized enterprises because of its open source and low cost. 3) Compared with Microsoft SQL Server, MySQL is more suitable for cross-platform applications. 4) Unlike MongoDB, MySQL is more suitable for structured data and transaction processing.
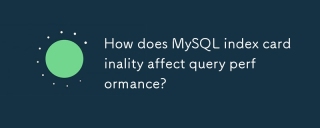
MySQL index cardinality has a significant impact on query performance: 1. High cardinality index can more effectively narrow the data range and improve query efficiency; 2. Low cardinality index may lead to full table scanning and reduce query performance; 3. In joint index, high cardinality sequences should be placed in front to optimize query.
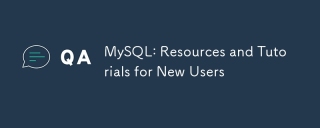
The MySQL learning path includes basic knowledge, core concepts, usage examples, and optimization techniques. 1) Understand basic concepts such as tables, rows, columns, and SQL queries. 2) Learn the definition, working principles and advantages of MySQL. 3) Master basic CRUD operations and advanced usage, such as indexes and stored procedures. 4) Familiar with common error debugging and performance optimization suggestions, such as rational use of indexes and optimization queries. Through these steps, you will have a full grasp of the use and optimization of MySQL.
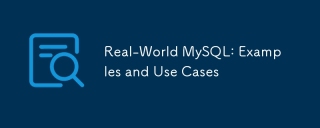
MySQL's real-world applications include basic database design and complex query optimization. 1) Basic usage: used to store and manage user data, such as inserting, querying, updating and deleting user information. 2) Advanced usage: Handle complex business logic, such as order and inventory management of e-commerce platforms. 3) Performance optimization: Improve performance by rationally using indexes, partition tables and query caches.


Hot AI Tools

Undresser.AI Undress
AI-powered app for creating realistic nude photos

AI Clothes Remover
Online AI tool for removing clothes from photos.

Undress AI Tool
Undress images for free

Clothoff.io
AI clothes remover

AI Hentai Generator
Generate AI Hentai for free.

Hot Article

Hot Tools

Zend Studio 13.0.1
Powerful PHP integrated development environment

DVWA
Damn Vulnerable Web App (DVWA) is a PHP/MySQL web application that is very vulnerable. Its main goals are to be an aid for security professionals to test their skills and tools in a legal environment, to help web developers better understand the process of securing web applications, and to help teachers/students teach/learn in a classroom environment Web application security. The goal of DVWA is to practice some of the most common web vulnerabilities through a simple and straightforward interface, with varying degrees of difficulty. Please note that this software
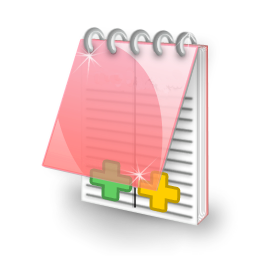
EditPlus Chinese cracked version
Small size, syntax highlighting, does not support code prompt function
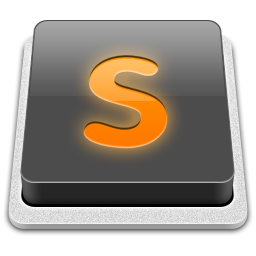
SublimeText3 Mac version
God-level code editing software (SublimeText3)

Safe Exam Browser
Safe Exam Browser is a secure browser environment for taking online exams securely. This software turns any computer into a secure workstation. It controls access to any utility and prevents students from using unauthorized resources.