The eight major sorting algorithms are: 1. Direct insertion sort; 2. Hill sort; 3. Simple selection sort; 4. Heap sort; 5. Bubble sort; 6. Quick sort; 7. Merge sort; 8. Bucket sort/radix sort.
The operating environment of this tutorial: Windows 10 system, Dell G3 computer.
Sorting includes internal sorting and external sorting. Internal sorting is to sort data records in memory, while external sorting is because the sorted data is very large and cannot accommodate all the sorted records at one time. Access is required during the sorting process. External storage.
The eight major sorting we talk about here are internal sorting.
When n is large, a sorting method with a time complexity of O(nlog2n) should be used: quick sort, Heap sort or merge sort.
Quick sort: It is currently considered the best method among internal sorting based on comparison. When the keywords to be sorted are randomly distributed, the average time of quick sort is the shortest;
1. Insertion sort—Straight Insertion Sort (Straight Insertion Sort)
Basic idea :
Insert a record into the sorted ordered list, thereby obtaining a new ordered list with the number of records increased by 1. That is: first treat the first record of the sequence as an ordered subsequence, and then insert the second record one by one until the entire sequence is ordered.
Key points: Set up sentinels for temporary storage and judgment of array boundaries. Direct insertion sorting example:#If you encounter an element that is equal to the inserted element, then the inserted element will place the element you want to insert after the equal element. Therefore, the order of equal elements has not changed. The order from the original unordered sequence is the order after sorting.
Implementation of algorithm:
void print(int a[], int n ,int i){
cout<<i <<":";
for(int j= 0; j<8; j++){
cout<<a[j] <<" ";
}
cout<<endl;
}
void InsertSort(int a[], int n)
{
for(int i= 1; i<n; i++){
if(a[i] < a[i-1]){ //若第i个元素大于i-1元素,直接插入。小于的话,移动有序表后插入
int j= i-1;
int x = a[i]; //复制为哨兵,即存储待排序元素
a[i] = a[i-1]; //先后移一个元素
while(x < a[j]){ //查找在有序表的插入位置
a[j+1] = a[j];
j--; //元素后移
}
a[j+1] = x; //插入到正确位置
}
print(a,n,i); //打印每趟排序的结果
}
}
int main(){
int a[8] = {3,1,5,7,2,4,9,6};
InsertSort(a,8);
print(a,8,8);
}
Time complexity: O(n^2).
Other insertion sorting includes binary insertion sorting and 2-way insertion sorting.
2. Insertion sort—Hill sort (Shell`s Sort)
Hill sort was proposed by D.L. Shell in 1959 , which is a great improvement over direct sorting. Hill sorting is also called
Narrowing incremental sorting
Basic idea:
First put The entire record sequence to be sorted is divided into several subsequences for direct insertion sorting. When the records in the entire sequence are "basically in order", all records are then directly inserted and sorted.
Operation method:Select an incremental sequence t1, t2,...,tk, where ti>tj , tk=1;
- Sort the sequence k times according to the incremental sequence number k;
- Sort each time according to the corresponding The increment ti divides the column to be sorted into several subsequences of length m, and performs direct insertion sorting on each subtable. Only when the increment factor is 1, the entire sequence is processed as a table, and the length of the table is the length of the entire sequence.
- Example of Hill sorting: The sorting process of shell sorting
Assume that the file to be sorted has 10 records, and their keywords are: 49, 38, 65, 97, 76, 13, 27, 49, 55, 04.
The values of the incremental series are: 5, 3, 1We simply process the incremental sequence: incremental sequence d = {n/2,n/4, n/8...1} n is the number of numbers to be sorted
That is: first divide a group of records to be sorted into several groups of subsequences according to a certain increment d (n/2, n is the number to be sorted), and the subscripts of the records in each group differ by d. For each group, Perform direct insertion sort on all elements, then group them with a smaller increment (d/2), and perform direct insertion sort on each group. Continue to reduce the increment until it is 1, and finally use direct insertion sort to complete the sorting.void print(int a[], int n ,int i){ cout<<i <<":"; for(int j= 0; j<8; j++){ cout<<a[j] <<" "; } cout<<endl; } /** * 直接插入排序的一般形式 * * @param int dk 缩小增量,如果是直接插入排序,dk=1 * */ void ShellInsertSort(int a[], int n, int dk) { for(int i= dk; i<n; ++i){ if(a[i] < a[i-dk]){ //若第i个元素大于i-1元素,直接插入。小于的话,移动有序表后插入 int j = i-dk; int x = a[i]; //复制为哨兵,即存储待排序元素 a[i] = a[i-dk]; //首先后移一个元素 while(x < a[j]){ //查找在有序表的插入位置 a[j+dk] = a[j]; j -= dk; //元素后移 } a[j+dk] = x; //插入到正确位置 } print(a, n,i ); } } /** * 先按增量d(n/2,n为要排序数的个数进行希尔排序 * */ void shellSort(int a[], int n){ int dk = n/2; while( dk >= 1 ){ ShellInsertSort(a, n, dk); dk = dk/2; } } int main(){ int a[8] = {3,1,5,7,2,4,9,6}; //ShellInsertSort(a,8,1); //直接插入排序 shellSort(a,8); //希尔插入排序 print(a,8,8); }
希尔排序时效分析很难,关键码的比较次数与记录移动次数依赖于增量因子序列d的选取,特定情况下可以准确估算出关键码的比较次数和记录的移动次数。目前还没有人给出选取最好的增量因子序列的方法。增量因子序列可以有各种取法,有取奇数的,也有取质数的,但需要注意:增量因子中除1 外没有公因子,且最后一个增量因子必须为1。希尔排序方法是一个不稳定的排序方法。
3. 选择排序—简单选择排序(Simple Selection Sort)
基本思想:
在要排序的一组数中,选出最小(或者最大)的一个数与第1个位置的数交换;然后在剩下的数当中再找最小(或者最大)的与第2个位置的数交换,依次类推,直到第n-1个元素(倒数第二个数)和第n个元素(最后一个数)比较为止。
简单选择排序的示例:
操作方法:
第一趟,从n 个记录中找出关键码最小的记录与第一个记录交换;
第二趟,从第二个记录开始的n-1 个记录中再选出关键码最小的记录与第二个记录交换;
以此类推.....
第i 趟,则从第i 个记录开始的n-i+1 个记录中选出关键码最小的记录与第i 个记录交换,
直到整个序列按关键码有序。
算法实现:
void print(int a[], int n ,int i){ cout<<"第"<<i+1 <<"趟 : "; for(int j= 0; j<8; j++){ cout<<a[j] <<" "; } cout<<endl; } /** * 数组的最小值 * * @return int 数组的键值 */ int SelectMinKey(int a[], int n, int i) { int k = i; for(int j=i+1 ;j< n; ++j) { if(a[k] > a[j]) k = j; } return k; } /** * 选择排序 * */ void selectSort(int a[], int n){ int key, tmp; for(int i = 0; i< n; ++i) { key = SelectMinKey(a, n,i); //选择最小的元素 if(key != i){ tmp = a[i]; a[i] = a[key]; a[key] = tmp; //最小元素与第i位置元素互换 } print(a, n , i); } } int main(){ int a[8] = {3,1,5,7,2,4,9,6}; cout<<"初始值:"; for(int j= 0; j<8; j++){ cout<<a[j] <<" "; } cout<<endl<<endl; selectSort(a, 8); print(a,8,8); }
简单选择排序的改进——二元选择排序
简单选择排序,每趟循环只能确定一个元素排序后的定位。我们可以考虑改进为每趟循环确定两个元素(当前趟最大和最小记录)的位置,从而减少排序所需的循环次数。改进后对n个数据进行排序,最多只需进行[n/2]趟循环即可。具体实现如下:
/** 这是伪函数, 逻辑判断不严谨 void selectSort(int r[],int n) { int i ,j , min ,max, tmp; for (i=1 ;i <= n/2;i++) { // 做不超过n/2趟选择排序 min = i; max = i ; //分别记录最大和最小关键字记录位置 for (j= i+1; j<= n-i; j++) { if (r[j] > r[max]) { max = j ; continue ; } if (r[j]< r[min]) { min = j ; } } //该交换操作还可分情况讨论以提高效率 tmp = r[i-1]; r[i-1] = r[min]; r[min] = tmp; tmp = r[n-i]; r[n-i] = r[max]; r[max] = tmp; } } */ void selectSort(int a[],int len) { int i,j,min,max,tmp; for(i=0; i<len/2; i++){ // 做不超过n/2趟选择排序 min = max = i; for(j=i+1; j<=len-1-i; j++){ //分别记录最大和最小关键字记录位置 if(a[j] > a[max]){ max = j; continue; } if(a[j] < a[min]){ min = j; } } //该交换操作还可分情况讨论以提高效率 if(min != i){//当第一个为min值,不用交换 tmp=a[min]; a[min]=a[i]; a[i]=tmp; } if(min == len-1-i && max == i)//当第一个为max值,同时最后一个为min值,不再需要下面操作 continue; if(max == i)//当第一个为max值,则交换后min的位置为max值 max = min; if(max != len-1-i){//当最后一个为max值,不用交换 tmp=a[max]; a[max]=a[len-1-i]; a[len-1-i]=tmp; } print(a,len, i); } }
4. 选择排序—堆排序(Heap Sort)
堆排序是一种树形选择排序,是对直接选择排序的有效改进。
基本思想:
堆的定义如下:具有n个元素的序列(k1,k2,...,kn),当且仅当满足
时称之为堆。由堆的定义可以看出,堆顶元素(即第一个元素)必为最小项(小顶堆)。
若以一维数组存储一个堆,则堆对应一棵完全二叉树,且所有非叶结点的值均不大于(或不小于)其子女的值,根结点(堆顶元素)的值是最小(或最大)的。如:
(a)大顶堆序列:(96, 83,27,38,11,09)
(b) 小顶堆序列:(12,36,24,85,47,30,53,91)
初始时把要排序的n个数的序列看作是一棵顺序存储的二叉树(一维数组存储二叉树),调整它们的存储序,使之成为一个堆,将堆顶元素输出,得到n 个元素中最小(或最大)的元素,这时堆的根节点的数最小(或者最大)。然后对前面(n-1)个元素重新调整使之成为堆,输出堆顶元素,得到n 个元素中次小(或次大)的元素。依此类推,直到只有两个节点的堆,并对它们作交换,最后得到有n个节点的有序序列。称这个过程为堆排序。
因此,实现堆排序需解决两个问题:
1. 如何将n 个待排序的数建成堆;
2. 输出堆顶元素后,怎样调整剩余n-1 个元素,使其成为一个新堆。
首先讨论第二个问题:输出堆顶元素后,对剩余n-1元素重新建成堆的调整过程。
调整小顶堆的方法:
1)设有m 个元素的堆,输出堆顶元素后,剩下m-1 个元素。将堆底元素送入堆顶((最后一个元素与堆顶进行交换),堆被破坏,其原因仅是根结点不满足堆的性质。
2)将根结点与左、右子树中较小元素的进行交换。
3)若与左子树交换:如果左子树堆被破坏,即左子树的根结点不满足堆的性质,则重复方法 (2).
4)若与右子树交换,如果右子树堆被破坏,即右子树的根结点不满足堆的性质。则重复方法 (2).
5)继续对不满足堆性质的子树进行上述交换操作,直到叶子结点,堆被建成。
称这个自根结点到叶子结点的调整过程为筛选。如图:
再讨论对n 个元素初始建堆的过程。
建堆方法:对初始序列建堆的过程,就是一个反复进行筛选的过程。
1)n 个结点的完全二叉树,则最后一个结点是第个结点的子树。
2)筛选从第个结点为根的子树开始,该子树成为堆。
3)之后向前依次对各结点为根的子树进行筛选,使之成为堆,直到根结点。
如图建堆初始过程:无序序列:(49,38,65,97,76,13,27,49)
算法的实现:
从算法描述来看,堆排序需要两个过程,一是建立堆,二是堆顶与堆的最后一个元素交换位置。所以堆排序有两个函数组成。一是建堆的渗透函数,二是反复调用渗透函数实现排序的函数。
void print(int a[], int n){ for(int j= 0; j<n; j++){ cout<<a[j] <<" "; } cout<<endl; } /** * 已知H[s…m]除了H[s] 外均满足堆的定义 * 调整H[s],使其成为大顶堆.即将对第s个结点为根的子树筛选, * * @param H是待调整的堆数组 * @param s是待调整的数组元素的位置 * @param length是数组的长度 * */ void HeapAdjust(int H[],int s, int length) { int tmp = H[s]; int child = 2*s+1; //左孩子结点的位置。(i+1 为当前调整结点的右孩子结点的位置) while (child < length) { if(child+1 <length && H[child]<H[child+1]) { // 如果右孩子大于左孩子(找到比当前待调整结点大的孩子结点) ++child ; } if(H[s]<H[child]) { // 如果较大的子结点大于父结点 H[s] = H[child]; // 那么把较大的子结点往上移动,替换它的父结点 s = child; // 重新设置s ,即待调整的下一个结点的位置 child = 2*s+1; } else { // 如果当前待调整结点大于它的左右孩子,则不需要调整,直接退出 break; } H[s] = tmp; // 当前待调整的结点放到比其大的孩子结点位置上 } print(H,length); } /** * 初始堆进行调整 * 将H[0..length-1]建成堆 * 调整完之后第一个元素是序列的最小的元素 */ void BuildingHeap(int H[], int length) { //最后一个有孩子的节点的位置 i= (length -1) / 2 for (int i = (length -1) / 2 ; i >= 0; --i) HeapAdjust(H,i,length); } /** * 堆排序算法 */ void HeapSort(int H[],int length) { //初始堆 BuildingHeap(H, length); //从最后一个元素开始对序列进行调整 for (int i = length - 1; i > 0; --i) { //交换堆顶元素H[0]和堆中最后一个元素 int temp = H[i]; H[i] = H[0]; H[0] = temp; //每次交换堆顶元素和堆中最后一个元素之后,都要对堆进行调整 HeapAdjust(H,0,i); } } int main(){ int H[10] = {3,1,5,7,2,4,9,6,10,8}; cout<<"初始值:"; print(H,10); HeapSort(H,10); //selectSort(a, 8); cout<<"结果:"; print(H,10); }
分析:
设树深度为k,。从根到叶的筛选,元素比较次数至多2(k-1)次,交换记录至多k 次。所以,在建好堆后,排序过程中的筛选次数不超过下式:
而建堆时的比较次数不超过4n 次,因此堆排序最坏情况下,时间复杂度也为:O(nlogn )。
5. 交换排序—冒泡排序(Bubble Sort)
基本思想:
在要排序的一组数中,对当前还未排好序的范围内的全部数,自上而下对相邻的两个数依次进行比较和调整,让较大的数往下沉,较小的往上冒。即:每当两相邻的数比较后发现它们的排序与排序要求相反时,就将它们互换。
冒泡排序的示例:
算法的实现:
void bubbleSort(int a[], int n){ for(int i =0 ; i< n-1; ++i) { for(int j = 0; j < n-i-1; ++j) { if(a[j] > a[j+1]) { int tmp = a[j] ; a[j] = a[j+1] ; a[j+1] = tmp; } } } }
冒泡排序算法的改进
对冒泡排序常见的改进方法是加入一标志性变量exchange,用于标志某一趟排序过程中是否有数据交换,如果进行某一趟排序时并没有进行数据交换,则说明数据已经按要求排列好,可立即结束排序,避免不必要的比较过程。本文再提供以下两种改进算法:
1.设置一标志性变量pos,用于记录每趟排序中最后一次进行交换的位置。由于pos位置之后的记录均已交换到位,故在进行下一趟排序时只要扫描到pos位置即可。
改进后算法如下:
void Bubble_1 ( int r[], int n) { int i= n -1; //初始时,最后位置保持不变 while ( i> 0) { int pos= 0; //每趟开始时,无记录交换 for (int j= 0; j< i; j++) if (r[j]> r[j+1]) { pos= j; //记录交换的位置 int tmp = r[j]; r[j]=r[j+1];r[j+1]=tmp; } i= pos; //为下一趟排序作准备 } }
2.传统冒泡排序中每一趟排序操作只能找到一个最大值或最小值,我们考虑利用在每趟排序中进行正向和反向两遍冒泡的方法一次可以得到两个最终值(最大者和最小者) , 从而使排序趟数几乎减少了一半。
改进后的算法实现为:
void Bubble_2 ( int r[], int n){ int low = 0; int high= n -1; //设置变量的初始值 int tmp,j; while (low < high) { for (j= low; j< high; ++j) //正向冒泡,找到最大者 if (r[j]> r[j+1]) { tmp = r[j]; r[j]=r[j+1];r[j+1]=tmp; } --high; //修改high值, 前移一位 for ( j=high; j>low; --j) //反向冒泡,找到最小者 if (r[j]<r[j-1]) { tmp = r[j]; r[j]=r[j-1];r[j-1]=tmp; } ++low; //修改low值,后移一位 } }
6. 交换排序—快速排序(Quick Sort)
基本思想:
1)选择一个基准元素,通常选择第一个元素或者最后一个元素,
2)通过一趟排序讲待排序的记录分割成独立的两部分,其中一部分记录的元素值均比基准元素值小。另一部分记录的 元素值比基准值大。
3)此时基准元素在其排好序后的正确位置
4)然后分别对这两部分记录用同样的方法继续进行排序,直到整个序列有序。
快速排序的示例:
(a)一趟排序的过程:
(b)排序的全过程
算法的实现:
递归实现:
void print(int a[], int n){ for(int j= 0; j<n; j++){ cout<<a[j] <<" "; } cout<<endl; } void swap(int *a, int *b) { int tmp = *a; *a = *b; *b = tmp; } int partition(int a[], int low, int high) { int privotKey = a[low]; //基准元素 while(low < high){ //从表的两端交替地向中间扫描 while(low < high && a[high] >= privotKey) --high; //从high 所指位置向前搜索,至多到low+1 位置。将比基准元素小的交换到低端 swap(&a[low], &a[high]); while(low < high && a[low] <= privotKey ) ++low; swap(&a[low], &a[high]); } print(a,10); return low; } void quickSort(int a[], int low, int high){ if(low < high){ int privotLoc = partition(a, low, high); //将表一分为二 quickSort(a, low, privotLoc -1); //递归对低子表递归排序 quickSort(a, privotLoc + 1, high); //递归对高子表递归排序 } } int main(){ int a[10] = {3,1,5,7,2,4,9,6,10,8}; cout<<"初始值:"; print(a,10); quickSort(a,0,9); cout<<"结果:"; print(a,10); }
分析:
快速排序是通常被认为在同数量级(O(nlog2n))的排序方法中平均性能最好的。但若初始序列按关键码有序或基本有序时,快排序反而蜕化为冒泡排序。为改进之,通常以“三者取中法”来选取基准记录,即将排序区间的两个端点与中点三个记录关键码居中的调整为支点记录。快速排序是一个不稳定的排序方法。
快速排序的改进
在本改进算法中,只对长度大于k的子序列递归调用快速排序,让原序列基本有序,然后再对整个基本有序序列用插入排序算法排序。实践证明,改进后的算法时间复杂度有所降低,且当k取值为 8 左右时,改进算法的性能最佳。算法思想如下:
void print(int a[], int n){ for(int j= 0; j<n; j++){ cout<<a[j] <<" "; } cout<<endl; } void swap(int *a, int *b) { int tmp = *a; *a = *b; *b = tmp; } int partition(int a[], int low, int high) { int privotKey = a[low]; //基准元素 while(low < high){ //从表的两端交替地向中间扫描 while(low < high && a[high] >= privotKey) --high; //从high 所指位置向前搜索,至多到low+1 位置。将比基准元素小的交换到低端 swap(&a[low], &a[high]); while(low < high && a[low] <= privotKey ) ++low; swap(&a[low], &a[high]); } print(a,10); return low; } void qsort_improve(int r[ ],int low,int high, int k){ if( high -low > k ) { //长度大于k时递归, k为指定的数 int pivot = partition(r, low, high); // 调用的Partition算法保持不变 qsort_improve(r, low, pivot - 1,k); qsort_improve(r, pivot + 1, high,k); } } void quickSort(int r[], int n, int k){ qsort_improve(r,0,n,k);//先调用改进算法Qsort使之基本有序 //再用插入排序对基本有序序列排序 for(int i=1; i<=n;i ++){ int tmp = r[i]; int j=i-1; while(tmp < r[j]){ r[j+1]=r[j]; j=j-1; } r[j+1] = tmp; } } int main(){ int a[10] = {3,1,5,7,2,4,9,6,10,8}; cout<<"初始值:"; print(a,10); quickSort(a,9,4); cout<<"结果:"; print(a,10); }
7. 归并排序(Merge Sort)
基本思想:
归并(Merge)排序法是将两个(或两个以上)有序表合并成一个新的有序表,即把待排序序列分为若干个子序列,每个子序列是有序的。然后再把有序子序列合并为整体有序序列。
归并排序示例:
合并方法:
设r[i…n]由两个有序子表r[i…m]和r[m+1…n]组成,两个子表长度分别为n-i +1、n-m。
j=m+1;k=i;i=i; //置两个子表的起始下标及辅助数组的起始下标
若i>m 或j>n,转⑷ //其中一个子表已合并完,比较选取结束
//选取r[i]和r[j]较小的存入辅助数组rf
如果r[i]否则,rf[k]=r[j]; j++; k++; 转⑵ //将尚未处理完的子表中元素存入rf
如果i 如果j合并结束。
//将r[i…m]和r[m +1 …n]归并到辅助数组rf[i…n] void Merge(ElemType *r,ElemType *rf, int i, int m, int n) { int j,k; for(j=m+1,k=i; i<=m && j <=n ; ++k){ if(r[j] < r[i]) rf[k] = r[j++]; else rf[k] = r[i++]; } while(i <= m) rf[k++] = r[i++]; while(j <= n) rf[k++] = r[j++]; }
归并的迭代算法
1 个元素的表总是有序的。所以对n 个元素的待排序列,每个元素可看成1 个有序子表。对子表两两合并生成n/2个子表,所得子表除最后一个子表长度可能为1 外,其余子表长度均为2。再进行两两合并,直到生成n 个元素按关键码有序的表。
void print(int a[], int n){ for(int j= 0; j<n; j++){ cout<<a[j] <<" "; } cout<<endl; } //将r[i…m]和r[m +1 …n]归并到辅助数组rf[i…n] void Merge(ElemType *r,ElemType *rf, int i, int m, int n) { int j,k; for(j=m+1,k=i; i<=m && j <=n ; ++k){ if(r[j] < r[i]) rf[k] = r[j++]; else rf[k] = r[i++]; } while(i <= m) rf[k++] = r[i++]; while(j <= n) rf[k++] = r[j++]; print(rf,n+1); } void MergeSort(ElemType *r, ElemType *rf, int lenght) { int len = 1; ElemType *q = r ; ElemType *tmp ; while(len < lenght) { int s = len; len = 2 * s ; int i = 0; while(i+ len <lenght){ Merge(q, rf, i, i+ s-1, i+ len-1 ); //对等长的两个子表合并 i = i+ len; } if(i + s < lenght){ Merge(q, rf, i, i+ s -1, lenght -1); //对不等长的两个子表合并 } tmp = q; q = rf; rf = tmp; //交换q,rf,以保证下一趟归并时,仍从q 归并到rf } } int main(){ int a[10] = {3,1,5,7,2,4,9,6,10,8}; int b[10]; MergeSort(a, b, 10); print(b,10); cout<<"结果:"; print(a,10); }
两路归并的递归算法
void MSort(ElemType *r, ElemType *rf,int s, int t) { ElemType *rf2; if(s==t) r[s] = rf[s]; else { int m=(s+t)/2; /*平分*p 表*/ MSort(r, rf2, s, m); /*递归地将p[s…m]归并为有序的p2[s…m]*/ MSort(r, rf2, m+1, t); /*递归地将p[m+1…t]归并为有序的p2[m+1…t]*/ Merge(rf2, rf, s, m+1,t); /*将p2[s…m]和p2[m+1…t]归并到p1[s…t]*/ } } void MergeSort_recursive(ElemType *r, ElemType *rf, int n) { /*对顺序表*p 作归并排序*/ MSort(r, rf,0, n-1); }
8. 桶排序/基数排序(Radix Sort)
说基数排序之前,我们先说桶排序:
Basic idea: is to divide the array into a limited number of buckets. Each bucket is sorted individually (it is possible to use other sorting algorithms or continue to use bucket sorting in a recursive manner). Bucket sort is an inductive result of pigeonhole sort. Bucket sort uses linear time (Θ(n)) when the values within the array to be sorted are evenly distributed. But bucket sort is not a comparison sort, and it is not affected by the O(n log n) lower limit.
To put it simply, it means grouping the data into buckets, and then sorting the contents in each bucket.
For example, if you want to sort n integers A[1..n] in the range of [1..1000]
First, you can set the bucket to the range of 10, Specifically, let set B[1] store the integers of [1..10], set B[2] store the integers of (10..20],...set B[i] store ((i-1)* 10, i*10] is an integer, i = 1,2,..100. There are 100 buckets in total.
Then, scan A[1..n] from beginning to end, and put each A[i] is put into the corresponding bucket B[j]. Then sort the numbers in each of these 100 buckets. At this time, you can use bubbles, selection, or even quick sort. Generally speaking, any sorting method can be used. Yes.
Finally, output the numbers in each bucket in sequence, and output the numbers in each bucket from small to large, so as to get a sequence in which all the numbers are sorted.
Suppose there are n numbers and m buckets. If the numbers are evenly distributed, there will be an average of n/m numbers in each bucket. If
uses quick sorting for the numbers in each bucket, Then the complexity of the entire algorithm is O(n m * n/m*log(n/m)) = O(n nlogn - nlogm)
From the above formula, when When m is close to n, the bucket sorting complexity is close to O(n)
Of course, the above complexity calculation is based on the assumption that the input n numbers are evenly distributed. This assumption is very strong. In fact, The effect in application is not so good. If all the numbers fall in the same bucket, it will degenerate into general sorting.
The time complexity of most of the major sorting algorithms mentioned above is O(n2), there are also some sorting algorithms whose time complexity is O(nlogn). Bucket sorting can achieve O(n) time complexity. But the disadvantages of bucket sorting are:
1) First The space complexity is relatively high and requires a lot of extra overhead. Sorting has the space overhead of two arrays, one to store the array to be sorted, and the other is the so-called bucket. For example, if the value to be sorted is from 0 to m-1, then m is needed buckets, this bucket array must have at least m spaces.
2) Secondly, the elements to be sorted must be within a certain range, etc.
Bucket sort is a distribution sort. The special thing about allocation sorting is that there is no need to compare key codes, but the premise is that some specific conditions of the columns to be sorted must be known.
The basic idea of allocation sorting: To put it bluntly, it is to perform multiple bucket sorting.
The radix sorting process does not require comparing keywords, but implements sorting through the "allocation" and "collection" processes. Their time complexity can reach linear order: O(n).
Example:The 52 cards in the playing cards can be divided into two fields according to the suit and face value. The size relationship is: :
Color:
Plum Blossom Face Value:
2 If the playing cards are sorted in ascending order by suit and face value Sort and get the following sequence:
That is, two cards. If the suits are different, regardless of the face value, the card with the lower suit is smaller than the card with the higher suit. Only in the case of the same suit Next, the size relationship is determined by the size of the face value. This is multi-key sorting.
为得到排序结果,我们讨论两种排序方法。
方法1:先对花色排序,将其分为4 个组,即梅花组、方块组、红心组、黑心组。再对每个组分别按面值进行排序,最后,将4 个组连接起来即可。
方法2:先按13 个面值给出13 个编号组(2 号,3 号,...,A 号),将牌按面值依次放入对应的编号组,分成13 堆。再按花色给出4 个编号组(梅花、方块、红心、黑心),将2号组中牌取出分别放入对应花色组,再将3 号组中牌取出分别放入对应花色组,……,这样,4 个花色组中均按面值有序,然后,将4 个花色组依次连接起来即可。
设n 个元素的待排序列包含d 个关键码{k1,k2,…,kd},则称序列对关键码{k1,k2,…,kd}有序是指:对于序列中任两个记录r[i]和r[j](1≤i≤j≤n)都满足下列有序关系:
其中k1 称为最主位关键码,kd 称为最次位关键码 。
两种多关键码排序方法:
多关键码排序按照从最主位关键码到最次位关键码或从最次位到最主位关键码的顺序逐次排序,分两种方法:
最高位优先(Most Significant Digit first)法,简称MSD 法:
1)先按k1 排序分组,将序列分成若干子序列,同一组序列的记录中,关键码k1 相等。
2)再对各组按k2 排序分成子组,之后,对后面的关键码继续这样的排序分组,直到按最次位关键码kd 对各子组排序后。
3)再将各组连接起来,便得到一个有序序列。扑克牌按花色、面值排序中介绍的方法一即是MSD 法。
最低位优先(Least Significant Digit first)法,简称LSD 法:
1) 先从kd 开始排序,再对kd-1进行排序,依次重复,直到按k1排序分组分成最小的子序列后。
2) 最后将各个子序列连接起来,便可得到一个有序的序列, 扑克牌按花色、面值排序中介绍的方法二即是LSD 法。
基于LSD方法的链式基数排序的基本思想
“多关键字排序”的思想实现“单关键字排序”。对数字型或字符型的单关键字,可以看作由多个数位或多个字符构成的多关键字,此时可以采用“分配-收集”的方法进行排序,这一过程称作基数排序法,其中每个数字或字符可能的取值个数称为基数。比如,扑克牌的花色基数为4,面值基数为13。在整理扑克牌时,既可以先按花色整理,也可以先按面值整理。按花色整理时,先按红、黑、方、花的顺序分成4摞(分配),再按此顺序再叠放在一起(收集),然后按面值的顺序分成13摞(分配),再按此顺序叠放在一起(收集),如此进行二次分配和收集即可将扑克牌排列有序。
基数排序:
是按照低位先排序,然后收集;再按照高位排序,然后再收集;依次类推,直到最高位。有时候有些属性是有优先级顺序的,先按低优先级排序,再按高优先级排序。最后的次序就是高优先级高的在前,高优先级相同的低优先级高的在前。基数排序基于分别排序,分别收集,所以是稳定的。
算法实现:
Void RadixSort(Node L[],length,maxradix) { int m,n,k,lsp; k=1;m=1; int temp[10][length-1]; Empty(temp); //清空临时空间 while(k<maxradix) //遍历所有关键字 { for(int i=0;i<length;i++) //分配过程 { if(L[i]<m) Temp[0][n]=L[i]; else Lsp=(L[i]/m)%10; //确定关键字 Temp[lsp][n]=L[i]; n++; } CollectElement(L,Temp); //收集 n=0; m=m*10; k++; } }
总结
各种排序的稳定性,时间复杂度和空间复杂度总结:
我们比较时间复杂度函数的情况:
The growth of the time complexity function O(n)
So sort records with larger n. The general choice is a sorting method with a time complexity of O(nlog2n).
In terms of time complexity:
(1) Square order (O(n2)) sorting
Various types of simple sorting: Direct insertion, direct selection and bubble sort;
(2) Linear logarithmic order (O(nlog2n)) sorting
Quick sort, heap sort and merge sort;
(3)O(n1 §) ) sort, § is a constant between 0 and 1.
Hill sorting
(4) Linear order (O(n)) sorting
Cardinal sorting, in addition to bucket and box sorting.
Note:
When the original table is ordered or basically ordered, direct insertion sort and bubble sort will greatly reduce the number of comparisons and moving records, and the time complexity can be reduced to O (n);
Quick sorting is the opposite. When the original table is basically ordered, it will degenerate into bubble sorting, and the time complexity increases to O(n2);
Is the original table Ordered, has little impact on the time complexity of simple selection sort, heap sort, merge sort and radix sort.
Stability:
Stability of sorting algorithm:If in the sequence to be sorted, there are multiple records with the same keyword , after sorting, the relative order of these records remains unchanged, then the algorithm is said to be stable; if after sorting, the records If the relative order of them changes, the algorithm is said to be unstable.
Benefits of stability: If the sorting algorithm is stable, then sort from one key, and then sort from another key, the result of sorting by the first key can be the second key Used for sorting. Radix sorting is like this. Sort by low bit first, then sort by high bit. The order of elements with the same low bit will not change when the high bit is the same. In addition, if the sorting algorithm is stable, redundant comparisons can be avoided;
Stable sorting algorithm: bubble sort, insertion sort, merge sort and radix Sorting
is not a stable sorting algorithm: selection sort, quick sort, Hill sort, heap sort
Selection sorting algorithm guidelines:
#Each sorting algorithm has its own advantages and disadvantages. Therefore, in practice, it is necessary to choose appropriately according to different situations, and even multiple methods can be combined.
The basis for choosing a sorting algorithm
There are many factors that affect sorting. An algorithm with a low average time complexity is not necessarily the optimal one. Conversely, sometimes an algorithm with a high average time complexity may be more suitable for some special cases. At the same time, when selecting an algorithm, its readability must also be considered to facilitate software maintenance. Generally speaking, the following four factors need to be considered:
1. The size of the number n of records to be sorted;
2. The size of the data volume of the record itself, that is, the size of other information in the record except keywords;
3. The structure and distribution of keywords;
4. Requirements for sorting stability.
Suppose the number of elements to be sorted is n.
1) When n is larger, the time complexity should be O( nlog2n) sorting method: quick sort, heap sort or merge sort.
Quick sort: It is currently considered the best method among comparison-based internal sorting. When the keywords to be sorted are randomly distributed, the average time of quick sort is the shortest;
Heap sort: If If the memory space allows and requires stability,
Merge sort: It has a certain amount of data movement, so we may combine it with insertion sort to first obtain a sequence of a certain length and then merge it, which will improve efficiency has seen an increase.
2) When n is large, memory space allows, and stability is required => Merge sort
3) When n is small, Direct insertion or direct selection sorting can be used.
Direct insertion sort: When the elements are distributed in order, direct insertion sort will greatly reduce the number of comparisons and the number of moving records.
Direct selection sorting: The elements are distributed in order. If stability is not required, choose direct selection sorting
5) Generally do not use or do not use traditional bubbling directly Sort.
6)Radix sort
It is a stable sorting algorithm, but it has certain limitations:
1. Keywords can be decomposed.
2. The recorded keywords have fewer digits, and it would be better if they are denser
3. If it is a number, it is best to be unsigned, otherwise it will increase the corresponding mapping complexity. You can first add or remove it. Sort separately.
For more programming-related knowledge, please visit: Introduction to Programming! !
The above is the detailed content of What are the eight sorting algorithms?. For more information, please follow other related articles on the PHP Chinese website!

Hot AI Tools

Undresser.AI Undress
AI-powered app for creating realistic nude photos

AI Clothes Remover
Online AI tool for removing clothes from photos.

Undress AI Tool
Undress images for free

Clothoff.io
AI clothes remover

AI Hentai Generator
Generate AI Hentai for free.

Hot Article

Hot Tools

Zend Studio 13.0.1
Powerful PHP integrated development environment

DVWA
Damn Vulnerable Web App (DVWA) is a PHP/MySQL web application that is very vulnerable. Its main goals are to be an aid for security professionals to test their skills and tools in a legal environment, to help web developers better understand the process of securing web applications, and to help teachers/students teach/learn in a classroom environment Web application security. The goal of DVWA is to practice some of the most common web vulnerabilities through a simple and straightforward interface, with varying degrees of difficulty. Please note that this software
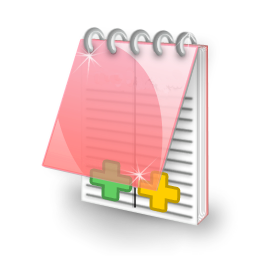
EditPlus Chinese cracked version
Small size, syntax highlighting, does not support code prompt function
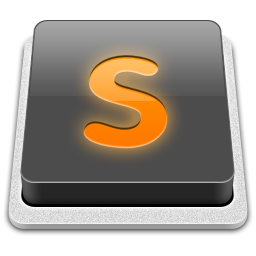
SublimeText3 Mac version
God-level code editing software (SublimeText3)

Safe Exam Browser
Safe Exam Browser is a secure browser environment for taking online exams securely. This software turns any computer into a secure workstation. It controls access to any utility and prevents students from using unauthorized resources.