What does the bootstrap method mean?
The Bootstrap method is an important estimation of statistical variability in non-parametric statistics. It is also a statistical method that can estimate the interval of statistical quantities, also known as the bootstrap method.
The core ideas and basic steps are as follows:
(1) Use repeated sampling technology to extract a certain number of samples (can be given by yourself, generally the same as the original sample) from the original sample. This The process allows for repeated sampling.
(2) Calculate the statistic T to be estimated based on the extracted samples.
(3) Repeat the above N times (generally greater than 1000) to obtain N statistics T.
(4) Calculate the sample variance of the above N statistics T to estimate the variance of the statistic T.
It should be said that Bootstrap is a popular statistical method in modern statistics and works well in small samples. Confidence intervals can be constructed through the estimation of variance, and its application scope is further extended.
Example of specific sampling method: If you want to know the number of fish in the pond, you can first extract N fish, mark them, and put them back into the pond.
Carry out repeated sampling, draw M times, and draw N fish each time. Examine the proportion of marked fish among the fish drawn each time, and calculate the statistics based on the proportion of M times.
Reasons for applying bootstrap: In fact, when performing analysis, the first thing to do is to determine the type of random variable, and then to determine what distribution the data of the random variable obeys. What distribution is crucial, because it directly determines whether it can be analyzed. For example: If you perform variance analysis, you must first require a normal distribution. If it is not a normal distribution, you must take remedial measures. This remedial measure is bootstrap. Bootstrap also has another use, because classic statistics is relatively perfect for central tendency, but it is not perfect for interval estimation of some other distribution parameters, such as median, quartiles, standard deviation, coefficient of variation, etc., so bootstrap is needed. This method. Bootstrap is similar to the classic statistical method. Generally, the parametric method is more efficient than the non-parametric method. However, the biggest drawback of the parametric method is that it requires a distribution model in advance. If the model does not conform to the model, the analysis results may be wrong, which is a white analysis.
If there are obvious layers in the data, stratified sampling can be used to improve analysis efficiency. spss defaults to the non-parametric bootstrap method and uses completely random sampling. Therefore, if stratified sampling is required, you cannot rely on the default and need to set it yourself. .
There is also special attention to be paid to how many observations are needed to be the most scientific and reasonable. The answer is 1,000. If it is less than this number, the calculation result will be inaccurate because the confidence interval is calculated according to the percentile method, so it cannot be too small. If there are more than 1000, the accuracy improvement is very limited in most cases, and system resources and computing time are wasted.
Operation steps in bootstrap's spss analysis: "Analysis" ~ "Compare Means" ~ "Means" ~ Select the independent variable and dependent variable ~ "Options" sub-dialog ~ "Cell Statistics" ~ bootstrap sub-dialog box ~ Execute bootstrap check box
One more point, if the dependent variable conforms to or approximately conforms to the normal distribution, the bootstrap method does not need to be used.
The above is the detailed content of What does bootstrap method mean?. For more information, please follow other related articles on the PHP Chinese website!

Hot AI Tools

Undresser.AI Undress
AI-powered app for creating realistic nude photos

AI Clothes Remover
Online AI tool for removing clothes from photos.

Undress AI Tool
Undress images for free

Clothoff.io
AI clothes remover

AI Hentai Generator
Generate AI Hentai for free.

Hot Article

Hot Tools

MinGW - Minimalist GNU for Windows
This project is in the process of being migrated to osdn.net/projects/mingw, you can continue to follow us there. MinGW: A native Windows port of the GNU Compiler Collection (GCC), freely distributable import libraries and header files for building native Windows applications; includes extensions to the MSVC runtime to support C99 functionality. All MinGW software can run on 64-bit Windows platforms.

DVWA
Damn Vulnerable Web App (DVWA) is a PHP/MySQL web application that is very vulnerable. Its main goals are to be an aid for security professionals to test their skills and tools in a legal environment, to help web developers better understand the process of securing web applications, and to help teachers/students teach/learn in a classroom environment Web application security. The goal of DVWA is to practice some of the most common web vulnerabilities through a simple and straightforward interface, with varying degrees of difficulty. Please note that this software

SecLists
SecLists is the ultimate security tester's companion. It is a collection of various types of lists that are frequently used during security assessments, all in one place. SecLists helps make security testing more efficient and productive by conveniently providing all the lists a security tester might need. List types include usernames, passwords, URLs, fuzzing payloads, sensitive data patterns, web shells, and more. The tester can simply pull this repository onto a new test machine and he will have access to every type of list he needs.
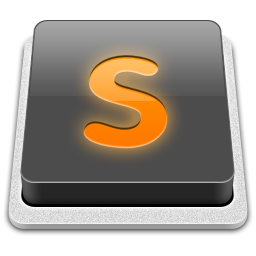
SublimeText3 Mac version
God-level code editing software (SublimeText3)

Notepad++7.3.1
Easy-to-use and free code editor