Detailed explanation of serializing objects and storing them in HDFS in Spark
This article mainly introduces the relevant information of object serialization stored in hdfs in Spark in java. Friends in need can refer to
Spark in java. Object serialization and storage to HDFS
Abstract: Spark applications often encounter such a requirement: JAVA objects need to be serialized and stored in HDFS, especially using MLlib calculations Some of the models that come out are stored in hdfs so that the models can be used repeatedly. The following example demonstrates reading data from Hbase in the Spark environment, generating a word2vec model, and storing it in hdfs.
No more nonsense, just post the code. spark1.4 + hbase0.98
import org.apache.spark.storage.StorageLevel import scala.collection.JavaConverters._ import java.io.File import java.io.FileInputStream import java.io.FileOutputStream import java.io.ObjectInputStream import java.io.ObjectOutputStream import java.net.URI import java.util.Date import org.ansj.library.UserDefineLibrary import org.ansj.splitWord.analysis.NlpAnalysis import org.ansj.splitWord.analysis.ToAnalysis import org.apache.hadoop.fs.FSDataInputStream import org.apache.hadoop.fs.FSDataOutputStream import org.apache.hadoop.fs.FileSystem import org.apache.hadoop.fs.FileUtil import org.apache.hadoop.fs.Path import org.apache.hadoop.hbase.client._ import org.apache.hadoop.hbase.{HBaseConfiguration, HTableDescriptor, TableName} import org.apache.hadoop.hbase.filter.FilterList import org.apache.hadoop.hbase.filter.PageFilter import org.apache.hadoop.hbase.filter.RegexStringComparator import org.apache.hadoop.hbase.filter.SingleColumnValueFilter import org.apache.hadoop.hbase.filter.CompareFilter.CompareOp import org.apache.hadoop.hbase.mapreduce.TableInputFormat import org.apache.hadoop.hbase.protobuf.ProtobufUtil import org.apache.hadoop.hbase.util.{Base64, Bytes} import com.feheadline.fespark.db.Neo4jManager import com.feheadline.fespark.util.Env import org.apache.spark.SparkConf import org.apache.spark.SparkContext import org.apache.spark.rdd._ import org.apache.spark.mllib.feature.{Word2Vec, Word2VecModel} import scala.math.log import scala.io.Source object Word2VecDemo { def convertScanToString(scan: Scan) = { val proto = ProtobufUtil.toScan(scan) Base64.encodeBytes(proto.toByteArray) } def main(args: Array[String]): Unit = { val sparkConf = new SparkConf().setAppName("Word2Vec Demo") sparkConf.set("spark.serializer", "org.apache.spark.serializer.KryoSerializer") sparkConf.set("spark.kryoserializer.buffer", "256m") sparkConf.set("spark.kryoserializer.buffer.max","2046m") sparkConf.set("spark.akka.frameSize", "500") sparkConf.set("spark.rpc.askTimeout", "30") val sc = new SparkContext(sparkConf) val hbaseConf = HBaseConfiguration.create() hbaseConf.set("hbase.zookeeper.quorum", "myzookeeper") hbaseConf.set(TableInputFormat.INPUT_TABLE, "crawled") val scan = new Scan() val filterList:FilterList = new FilterList(FilterList.Operator.MUST_PASS_ALL) val comp:RegexStringComparator = new RegexStringComparator(""".{1500,}""") val articleFilter:SingleColumnValueFilter = new SingleColumnValueFilter( "data".getBytes, "article".getBytes, CompareOp.EQUAL, comp ) filterList.addFilter(articleFilter) filterList.addFilter(new PageFilter(100)) scan.setFilter(filterList) scan.setCaching(50) scan.setCacheBlocks(false) hbaseConf.set(TableInputFormat.SCAN,convertScanToString(scan)) val crawledRDD = sc.newAPIHadoopRDD( hbaseConf, classOf[TableInputFormat], classOf[org.apache.hadoop.hbase.io.ImmutableBytesWritable], classOf[org.apache.hadoop.hbase.client.Result] ) val articlesRDD = crawledRDD.filter{ case (_,result) => { val content = Bytes.toString(result.getValue("data".getBytes,"article".getBytes)) content != null } } val wordsInDoc = articlesRDD.map{ case (_,result) => { val content = Bytes.toString(result.getValue("data".getBytes,"article".getBytes)) if(content!=null)ToAnalysis.parse(content).asScala.map(_.getName).toSeq else Seq("") } } val fitleredWordsInDoc = wordsInDoc.filter(_.nonEmpty) val word2vec = new Word2Vec() val model = word2vec.fit(fitleredWordsInDoc) //---------------------------------------重点看这里------------------------------------------------------------- //将上面的模型存储到hdfs val hadoopConf = sc.hadoopConfiguration hadoopConf.set("fs.defaultFS", "hdfs://myhadoop:9000/") val fileSystem = FileSystem.get(hadoopConf) val path = new Path("/user/hadoop/data/mllib/word2vec-object") val oos = new ObjectOutputStream(new FSDataOutputStream(fileSystem.create(path))) oos.writeObject(model) oos.close //这里示例另外一个程序直接从hdfs读取序列化对象使用模型 val ois = new ObjectInputStream(new FSDataInputStream(fileSystem.open(path))) val sample_model = ois.readObject.asInstanceOf[Word2VecModel] /* * //你还可以将序列化文件从hdfs放到本地, scala程序使用模型 * import java.io._ * import org.apache.spark.mllib.feature.{Word2Vec, Word2VecModel} * val ois = new ObjectInputStream(new FileInputStream("/home/cherokee/tmp/word2vec-object")) * val sample_model = ois.readObject.asInstanceOf[Word2VecModel] * ois.close */ //-------------------------------------------------------------------------------------------------------------- } }
The above is the detailed content of Detailed explanation of serializing objects and storing them in HDFS in Spark. For more information, please follow other related articles on the PHP Chinese website!
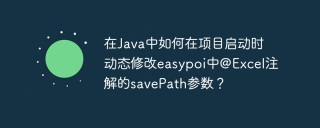
How to dynamically configure the parameters of entity class annotations in Java During the development process, we often encounter the need to dynamically configure the annotation parameters according to different environments...
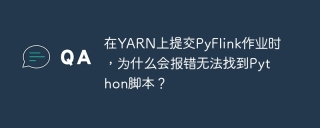
Analysis of the reason why Python script cannot be found when submitting a PyFlink job on YARN When you try to submit a PyFlink job through YARN, you may encounter...
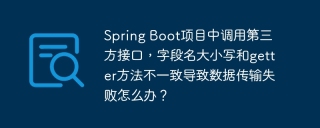
The difficulties encountered when calling third-party interfaces to transmit data in SpringBoot project will be used for a Spring...
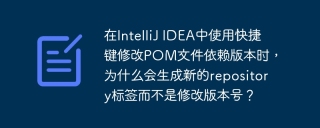
In IntelliJ...
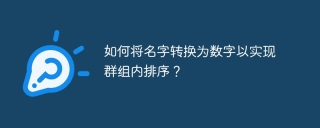
How to convert names to numbers to implement sorting within groups? When sorting users in groups, it is often necessary to convert the user's name into numbers so that it can be different...
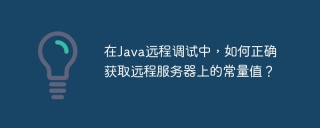
Questions and Answers about constant acquisition in Java Remote Debugging When using Java for remote debugging, many developers may encounter some difficult phenomena. It...
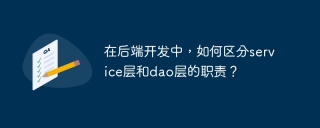
Discussing the hierarchical architecture in back-end development. In back-end development, hierarchical architecture is a common design pattern, usually including controller, service and dao three layers...


Hot AI Tools

Undresser.AI Undress
AI-powered app for creating realistic nude photos

AI Clothes Remover
Online AI tool for removing clothes from photos.

Undress AI Tool
Undress images for free

Clothoff.io
AI clothes remover

AI Hentai Generator
Generate AI Hentai for free.

Hot Article

Hot Tools

SecLists
SecLists is the ultimate security tester's companion. It is a collection of various types of lists that are frequently used during security assessments, all in one place. SecLists helps make security testing more efficient and productive by conveniently providing all the lists a security tester might need. List types include usernames, passwords, URLs, fuzzing payloads, sensitive data patterns, web shells, and more. The tester can simply pull this repository onto a new test machine and he will have access to every type of list he needs.
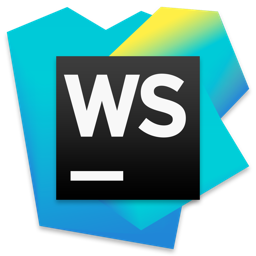
WebStorm Mac version
Useful JavaScript development tools
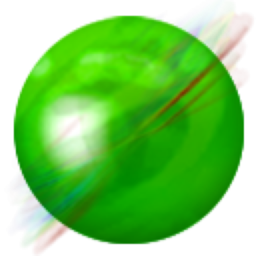
ZendStudio 13.5.1 Mac
Powerful PHP integrated development environment

Safe Exam Browser
Safe Exam Browser is a secure browser environment for taking online exams securely. This software turns any computer into a secure workstation. It controls access to any utility and prevents students from using unauthorized resources.

MinGW - Minimalist GNU for Windows
This project is in the process of being migrated to osdn.net/projects/mingw, you can continue to follow us there. MinGW: A native Windows port of the GNU Compiler Collection (GCC), freely distributable import libraries and header files for building native Windows applications; includes extensions to the MSVC runtime to support C99 functionality. All MinGW software can run on 64-bit Windows platforms.