Detailed explanation of common sorting codes in python
This article mainly introduces the basic tutorial of Python algorithm in detail, which has certain reference value. Interested friends can refer to it
Foreword: The Tencent written test received 10,000 points in the first two days Critical attack, I feel like I wasted two days to go to Niuke.com to do the questions... This blog introduces several simple/common sorting algorithms, which is sorted out.
Time complexity
(1) Time frequencyThe time it takes to execute an algorithm cannot be calculated theoretically, You have to run the test on your machine to know. But it is impossible and unnecessary for us to test every algorithm on the computer. We only need to know which algorithm takes more time and which algorithm takes less time. And the time an algorithm takes is proportional to the number of executions of statements in the algorithm. Whichever algorithm has more statements is executed, it takes more time. The number of statement executions in an algorithm is called statement frequency or time frequency. Denote it as T(n).
(2) Time complexity In the time frequency just mentioned, n is called the scale of the problem. When n continues to change, the time frequency T(n) will also keep changing. But sometimes we want to know what pattern it shows when it changes. To this end, we introduce the concept of time complexity. Generally speaking, the number of times the basic operations in the algorithm are repeated is a function of the problem size n, represented by T(n). If there is an auxiliary function f(n), makes it so that when n approaches infinity , the limit value of T(n)/f(n) is a constant that is not equal to zero, then f(n) is said to be a function of the same order of magnitude as T(n). Denoted as T(n)=O(f(n)), O(f(n)) is called the asymptotic time complexity of the algorithm, or time complexity for short.
Exponential time
refers to the calculation time m(n) required to solve a problem, depending on the size of the input data And it grows exponentially (that is, the amount of input data grows linearly, and the time it takes will grow exponentially)
for (i=1; i<=n; i++) x++; for (i=1; i<=n; i++) for (j=1; j<=n; j++) x++;
The time complexity of the first for loop is Ο(n), the time complexity of the second for loop is Ο(n2), then the time complexity of the entire algorithm is Ο(n+n2)=Ο(n2).
Constant time
If the upper bound of an algorithm has nothing to do with the input size, it is said to have constant time, recorded as time. An example is accessing a single element in an array, since accessing it requires only one instruction. However, finding the smallest element in an unordered array is not, as this requires looping through all elements to find the smallest value. This is a linear time operation, or time. But if the number of elements is known in advance and the number is assumed to remain constant, the operation can also be said to have constant time.
Logarithmic time
If the algorithm’s T(n) =O(logn), then It is said to have logarithmic time
Common algorithms withlogarithmic time include binary tree related operations and binary search.
The logarithmic time algorithm is very efficient because every time an input is added, the additional calculation time required becomes smaller.
Recursively chop a string in half and output is a simple example of this category of functions. It takes O(log n) time because we cut the string in half before each output. This means that if we want to increase the number of outputs, we need to double the string length.
Linear time
If the time complexity of an algorithm is O(n), the algorithm is said to have linear time, or O(n) time. Informally, this means that for sufficiently large inputs, the running time increases linearly with the size of the input. For example, a program that calculates the sum of all elements of a list takes time proportional to the length of the list.
1. Bubble Algorithm
Basic idea:
In a group of numbers to be sorted, the current pair has not been sorted yet All the numbers within the sequence range are compared and adjusted from top to bottom, so that the larger number sinks and the smaller number rises. That is: whenever two adjacent numbers are compared and it is found that their ordering is opposite to the ordering requirement, they are swapped.
Example of bubble sort:
Algorithm implementation:
def bubble(array): for i in range(len(array)-1): for j in range(len(array)-1-i): if array[j] > array[j+1]: # 如果前一个大于后一个,则交换 temp = array[j] array[j] = array[j+1] array[j+1] = temp if __name__ == "__main__": array = [265, 494, 302, 160, 370, 219, 247, 287, 354, 405, 469, 82, 345, 319, 83, 258, 497, 423, 291, 304] print("------->排序前<-------") print(array) bubble(array) print("------->排序后<-------") print(array)
Output:
------->Before sorting[265, 494, 302, 160, 370, 219, 247, 287, 354, 405, 469, 82, 345, 319, 83, 258, 497, 423, 291, 304]
------->After sorting[82, 83, 160, 219, 247, 258, 265, 287, 291, 302, 304, 319, 345, 354, 370, 405, 423, 469, 494, 497]
explain:
以随机产生的五个数为例: li=[354,405,469,82,345]
冒泡排序是怎么实现的?
首先先来个大循环,每次循环找出最大的数,放在列表的最后面。在上面的例子中,第一次找出最大数469,将469放在最后一个,此时我们知道
列表最后一个肯定是最大的,故还需要再比较前面4个数,找出4个数中最大的数405,放在列表倒数第二个......
5个数进行排序,需要多少次的大循环?? 当然是4次啦!同理,若有n个数,需n-1次大循环。
现在你会问我: 第一次找出最大数469,将469放在最后一个??怎么实现的??
嗯,(在大循环里)用一个小循环进行两数比较,首先354与405比较,若前者较大,需要交换数;反之不用交换。
当469与82比较时,需交换,故列表倒数第二个为469;469与345比较,需交换,此时最大数469位于列表最后一个啦!
难点来了,小循环需要多少次??
进行两数比较,从列表头比较至列表尾,此时需len(array)-1次!! 但是,嗯,举个例子吧: 当大循环i为3时,说明此时列表的最后3个数已经排好序了,不必进行两数比较,故小循环需len(array)-1-3. 即len(array)-1-i
冒泡排序复杂度:
时间复杂度: 最好情况O(n), 最坏情况O(n^2), 平均情况O(n^2)
空间复杂度: O(1)
稳定性: 稳定
简单选择排序的示例:
二、选择排序
The selection sort works as follows: you look through the entire array for the smallest element, once you find it you swap it (the smallest element) with the first element of the array. Then you look for the smallest element in the remaining array (an array without the first element) and swap it with the second element. Then you look for the smallest element in the remaining array (an array without first and second elements) and swap it with the third element, and so on. Here is an example
基本思想:
在要排序的一组数中,选出最小(或者最大)的一个数与第1个位置的数交换;然后在剩下的数当中再找最小(或者最大)的与第2个位置的数交换,依次类推,直到第n-1个元素(倒数第二个数)和第n个元素(最后一个数)比较为止。
简单选择排序的示例:
算法实现:
def select_sort(array): for i in range(len(array)-1): # 找出最小的数放与array[i]交换 for j in range(i+1, len(array)): if array[i] > array[j]: temp = array[i] array[i] = array[j] array[j] = temp if __name__ == "__main__": array = [265, 494, 302, 160, 370, 219, 247, 287, 354, 405, 469, 82, 345, 319, 83, 258, 497, 423, 291, 304] print(array) select_sort(array) print(array)
选择排序复杂度:
时间复杂度: 最好情况O(n^2), 最坏情况O(n^2), 平均情况O(n^2)
空间复杂度: O(1)
稳定性: 不稳定
举个例子:序列5 8 5 2 9, 我们知道第一趟选择第1个元素5会与2进行交换,那么原序列中两个5的相对先后顺序也就被破坏了。
排序效果:
三、直接插入排序
插入排序(Insertion Sort)的基本思想是:将列表分为2部分,左边为排序好的部分,右边为未排序的部分,循环整个列表,每次将一个待排序的记录,按其关键字大小插入到前面已经排好序的子序列中的适当位置,直到全部记录插入完成为止。
插入排序非常类似于整扑克牌。
在开始摸牌时,左手是空的,牌面朝下放在桌上。接着,一次从桌上摸起一张牌,并将它插入到左手一把牌中的正确位置上。为了找到这张牌的正确位置,要将它与手中已有的牌从右到左地进行比较。无论什么时候,左手中的牌都是排好序的。
也许你没有意识到,但其实你的思考过程是这样的:现在抓到一张7,把它和手里的牌从右到左依次比较,7比10小,应该再往左插,7比5大,好,就插这里。为什么比较了10和5就可以确定7的位置?为什么不用再比较左边的4和2呢?因为这里有一个重要的前提:手里的牌已经是排好序的。现在我插了7之后,手里的牌仍然是排好序的,下次再抓到的牌还可以用这个方法插入。编程对一个数组进行插入排序也是同样道理,但和插入扑克牌有一点不同,不可能在两个相邻的存储单元之间再插入一个单元,因此要将插入点之后的数据依次往后移动一个单元。
设监视哨是我大一在书上有看过,大家忽视上图的监视哨。
算法实现:
import time def insertion_sort(array): for i in range(1, len(array)): # 对第i个元素进行插入,i前面是已经排序好的元素 position = i # 要插入数的下标 current_val = array[position] # 把当前值存下来 # 如果前一个数大于要插入数,则将前一个数往后移,比如5,8,12,7;要将7插入,先把7保存下来,比较12与7,将12往后移 while position > 0 and current_val < array[position-1]: array[position] = array[position-1] position -= 1 else: # 当position为0或前一个数比待插入还小时 array[position] = current_val if __name__ == "__main__": array = [92, 77, 67, 8, 6, 84, 55, 85, 43, 67] print(array) time_start = time.time() insertion_sort(array) time_end = time.time() print("time: %s" % (time_end-time_start)) print(array)
输出:
[92, 77, 67, 8, 6, 84, 55, 85, 43, 67]
time: 0.0
[6, 8, 43, 55, 67, 67, 77, 84, 85, 92]
如果碰见一个和插入元素相等的,那么插入元素把想插入的元素放在相等元素的后面。所以,相等元素的前后顺序没有改变,从原无序序列出去的顺序就是排好序后的顺序,所以插入排序是稳定的。
直接插入排序复杂度:
时间复杂度: 最好情况O(n), 最坏情况O(n^2), 平均情况O(n^2)
空间复杂度: O(1)
稳定性: 稳定
个人感觉直接插入排序算法难度是选择/冒泡算法是两倍……
四、快速排序
快速排序示例:
算法实现:
def quick_sort(array, left, right): ''' :param array: :param left: 列表的第一个索引 :param right: 列表最后一个元素的索引 :return: ''' if left >= right: return low = left high = right key = array[low] # 第一个值,即基准元素 while low < high: # 只要左右未遇见 while low < high and array[high] > key: # 找到列表右边比key大的值 为止 high -= 1 # 此时直接 把key跟 比它大的array[high]进行交换 array[low] = array[high] array[high] = key while low < high and array[low] <= key: # 找到key左边比key大的值,这里为何是<=而不是<呢?你要思考。。。 low += 1 # 找到了左边比k大的值 ,把array[high](此时应该刚存成了key) 跟这个比key大的array[low]进行调换 array[high] = array[low] array[low] = key quick_sort(array, left, low-1) # 最后用同样的方式对分出来的左边的小组进行同上的做法 quick_sort(array,low+1, right) # 用同样的方式对分出来的右边的小组进行同上的做法 if __name__ == '__main__': array = [8,4,1, 14, 6, 2, 3, 9,5, 13, 7,1, 8,10, 12] print("-------排序前-------") print(array) quick_sort(array, 0, len(array)-1) print("-------排序后-------") print(array)
输出:
-------排序前-------
[8, 4, 1, 14, 6, 2, 3, 9, 5, 13, 7, 1, 8, 10, 12]
-------排序后-------
[1, 1, 2, 3, 4, 5, 6, 7, 8, 8, 9, 10, 12, 13, 14]
22行那里如果不加=号,当排序64,77,64是会死循环,此时key=64, 最后的64与开始的64交换,开始的64与本最后的64交换…… 无穷无尽
直接插入排序复杂度:
时间复杂度: 最好情况O(nlogn), 最坏情况O(n^2), 平均情况O(nlogn)
下面空间复杂度是看别人博客的,我也不大懂了……改天再研究下。
最优的情况下空间复杂度为:O(logn);每一次都平分数组的情况
最差的情况下空间复杂度为:O( n );退化为冒泡排序的情况
稳定性:不稳定
快速排序效果:
The above is the detailed content of Detailed explanation of common sorting codes in python. For more information, please follow other related articles on the PHP Chinese website!
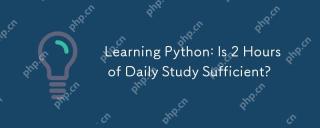
Is it enough to learn Python for two hours a day? It depends on your goals and learning methods. 1) Develop a clear learning plan, 2) Select appropriate learning resources and methods, 3) Practice and review and consolidate hands-on practice and review and consolidate, and you can gradually master the basic knowledge and advanced functions of Python during this period.
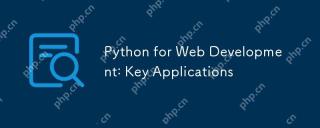
Key applications of Python in web development include the use of Django and Flask frameworks, API development, data analysis and visualization, machine learning and AI, and performance optimization. 1. Django and Flask framework: Django is suitable for rapid development of complex applications, and Flask is suitable for small or highly customized projects. 2. API development: Use Flask or DjangoRESTFramework to build RESTfulAPI. 3. Data analysis and visualization: Use Python to process data and display it through the web interface. 4. Machine Learning and AI: Python is used to build intelligent web applications. 5. Performance optimization: optimized through asynchronous programming, caching and code
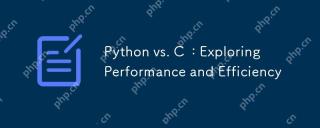
Python is better than C in development efficiency, but C is higher in execution performance. 1. Python's concise syntax and rich libraries improve development efficiency. 2.C's compilation-type characteristics and hardware control improve execution performance. When making a choice, you need to weigh the development speed and execution efficiency based on project needs.
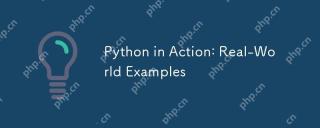
Python's real-world applications include data analytics, web development, artificial intelligence and automation. 1) In data analysis, Python uses Pandas and Matplotlib to process and visualize data. 2) In web development, Django and Flask frameworks simplify the creation of web applications. 3) In the field of artificial intelligence, TensorFlow and PyTorch are used to build and train models. 4) In terms of automation, Python scripts can be used for tasks such as copying files.
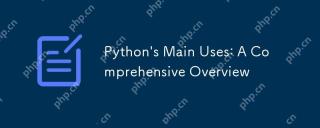
Python is widely used in data science, web development and automation scripting fields. 1) In data science, Python simplifies data processing and analysis through libraries such as NumPy and Pandas. 2) In web development, the Django and Flask frameworks enable developers to quickly build applications. 3) In automated scripts, Python's simplicity and standard library make it ideal.
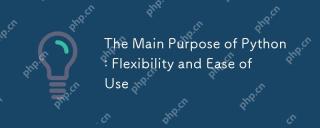
Python's flexibility is reflected in multi-paradigm support and dynamic type systems, while ease of use comes from a simple syntax and rich standard library. 1. Flexibility: Supports object-oriented, functional and procedural programming, and dynamic type systems improve development efficiency. 2. Ease of use: The grammar is close to natural language, the standard library covers a wide range of functions, and simplifies the development process.
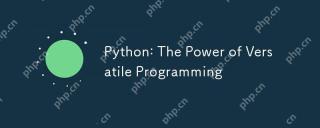
Python is highly favored for its simplicity and power, suitable for all needs from beginners to advanced developers. Its versatility is reflected in: 1) Easy to learn and use, simple syntax; 2) Rich libraries and frameworks, such as NumPy, Pandas, etc.; 3) Cross-platform support, which can be run on a variety of operating systems; 4) Suitable for scripting and automation tasks to improve work efficiency.
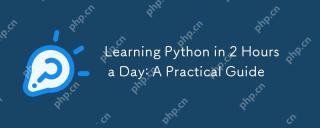
Yes, learn Python in two hours a day. 1. Develop a reasonable study plan, 2. Select the right learning resources, 3. Consolidate the knowledge learned through practice. These steps can help you master Python in a short time.


Hot AI Tools

Undresser.AI Undress
AI-powered app for creating realistic nude photos

AI Clothes Remover
Online AI tool for removing clothes from photos.

Undress AI Tool
Undress images for free

Clothoff.io
AI clothes remover

AI Hentai Generator
Generate AI Hentai for free.

Hot Article

Hot Tools

MinGW - Minimalist GNU for Windows
This project is in the process of being migrated to osdn.net/projects/mingw, you can continue to follow us there. MinGW: A native Windows port of the GNU Compiler Collection (GCC), freely distributable import libraries and header files for building native Windows applications; includes extensions to the MSVC runtime to support C99 functionality. All MinGW software can run on 64-bit Windows platforms.
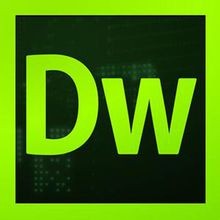
Dreamweaver CS6
Visual web development tools
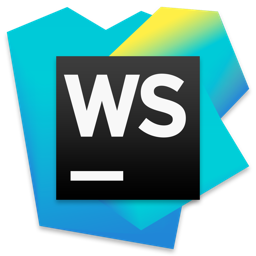
WebStorm Mac version
Useful JavaScript development tools
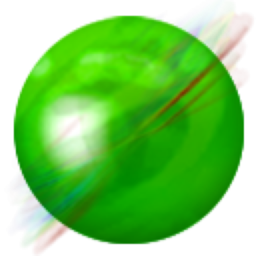
ZendStudio 13.5.1 Mac
Powerful PHP integrated development environment

Notepad++7.3.1
Easy-to-use and free code editor