


Recently, I have taken over more and more things. The work of release and operation and maintenance is quite mechanical, and the frequency is quite high, which leads to a waste of time but has many advantages. Fix bugs, test, submit the repository (2 minutes), ssh to the test environment for pull deployment (2 minutes), rsync to online machines A, B, C, D, E (1 minute), ssh to ABCDE5 respectively Each machine is restarted one by one (8-10 minutes) = 13-15 minutes. The frustrating thing is that each operation is the same and the command is the same. The terrible thing is that on multiple machines, it is difficult to do it with one script on this machine. The main time was wasted on ssh, typing commands, and writing them into scripts, which can be executed with one click. It took two minutes to look at the execution results until I discovered that fabric can solidify commands for automated deployment or multi-machine operations. Into a script is very similar to some operation and maintenance tools. The main reason for using it is that it is simple, easy to use and easy to use. Of course, you can also combine various shell commands. The difference between ancient artifacts and modern weapons
Environment Configuration
Install the corresponding package on the local machine and the target machine (note, both must be present)
sudo easy_install fabric
It is currently version 1.6 (or use pip install, the same )
After the installation is completed, you can check whether the installation is successful
[ken@~$] which fab /usr/local/bin/fab
After the installation is completed, you can browse the official documentation
Then, you can get started
hello world
First perform simple operations on this machine and have a preliminary understanding. The source of the example is from the official website
Create a new py script: fabfile.py
def hello(): print("Hello world!")
Command line execution:
[ken@~/tmp/fab$] fab hello Hello world!
Done.
Note that fabfile does not need to be used as the file name here, but the file needs to be specified when executing[ken@~/tmp/fab$] mv fabfile.py test.py fabfile.py -> test.py [ken@~/tmp/fab$] fab hello Fatal error: Couldn't find any fabfiles! Remember that -f can be used to specify fabfile path, and use -h for help. Aborting. [ken@~/tmp/fab$] fab -f test.py hello Hello world!
Done.With parameters:
Modify the fabfile.py script :
def hello(name, value): print("%s = %s!" % (name, value))
Execute
[ken@~/tmp/fab$] fab hello:name=age,value=20 age = 20! Done. [ken@~/tmp/fab$] fab hello:age,20 age = 20!
Done.
Perform native operationSimple local operation
from fabric.api import local def lsfab(): local('cd ~/tmp/fab') local('ls')
Result:
[ken@~/tmp/fab$] pwd;ls /Users/ken/tmp/fab fabfile.py fabfile.pyc test.py test.pyc [ken@~/tmp/fab$] fab -f test.py lsfab [localhost] local: cd ~/tmp/fab [localhost] local: ls fabfile.py fabfile.pyc test.py test.pyc
Done.
Actual combat begins:
Assume that you have to submit a configuration file settings.py to the repository every day (conflicts are not considered here)
If it is a manual operation:
cd /home/project/test/conf/ git add settings.py git commit -m 'daily update settings.py' git pull origin git push origin
In other words, you have to type these commands manually once a day. The so-called daily job is a mechanized job that needs to be repeated every day. Let us see how to use fabric to achieve one-click completion: (It is actually practical Shell scripts can be done directly, but the advantage of fab is not here. Here we mainly prepare for local + remote operations. After all, writing one script for operations in two places is easy to maintain)
from fabric.api import local def setting_ci(): local("cd /home/project/test/conf/") local("git add settings.py") #后面你懂的,懒得敲了…..
Mix and match to integrate remote operations Operation
At this time, assume that you want to go to the project directory corresponding to machine A's /home/ken/project to update the configuration file#!/usr/bin/env python # encoding: utf-8 from fabric.api import local,cd,run env.hosts=['user@ip:port',] #ssh要用到的参数 env.password = 'pwd' def setting_ci(): local('echo "add and commit settings in local"') #刚才的操作换到这里,你懂的 def update_setting_remote(): print "remote update" with cd('~/temp'): #cd用于进入某个目录 run('ls -l | wc -l') #远程操作用run def update(): setting_ci() update_setting_remote()
Then execute:
[ken@~/tmp/fab$] fab -f deploy.py update [user@ip:port] Executing task 'update' [localhost] local: echo "add and commit settings in local" add and commit settings in local remote update [user@ip:port] run: ls -l | wc -l [user@ip:port] out: 12 [user@ip:port] out:
Done.
Note that if env.password is not declared, an interaction requiring password input will pop up when executing to the corresponding machine
Multiple server mashup
#!/usr/bin/env python # encoding: utf-8 from fabric.api import * #操作一致的服务器可以放在一组,同一组的执行同一套操作 env.roledefs = { 'testserver': ['user1@host1:port1',], 'realserver': ['user2@host2:port2', ] } #env.password = '这里不要用这种配置了,不可能要求密码都一致的,明文编写也不合适。打通所有ssh就行了' @roles('testserver') def task1(): run('ls -l | wc -l') @roles('realserver') def task2(): run('ls ~/temp/ | wc -l') def dotask(): execute(task1) execute(task2)
Result:
[ken@~/tmp/fab$] fab -f mult.py dotask [user1@host1:port1] Executing task 'task1' [user1@host1:port1] run: ls -l | wc -l [user1@host1:port1] out: 9 [user1@host1:port1] out: [user2@host2:port2] Executing task 'task2' [user2@host2:port2] run: ls ~/temp/ | wc -l [user2@host2:port2] out: 11 [user2@host2:port2] out:
Done.
Extension1. Color
You can print the color, which is more eye-catching and convenient when viewing the operation result information
from fabric.colors import * def show(): print green('success') print red('fail') print yellow('yellow') #fab -f color.py show
2. Errors and exceptions
About error handling
By default, a set of commands will not continue to execute after the previous command fails to execute
After failure Different processing can also be performed. The document
is not used at the moment. Please read it if you use it later
3. Password management
See the document
A better way to manage passwords. I’m a bit unsophisticated and haven’t gotten through it. The main reason is that the server list changes frequently. My solution is:
1. Host, user, port, password configuration list, all written in A file
or directly into the script, of course this is more...
env.hosts = [ 'host1', 'host2' ] env.passwords = { 'host1': "pwdofhost1", 'host2': "pwdofhost2", }
or
env.roledefs = { 'testserver': ['host1', 'host2'], 'realserver': ['host3', ] } env.passwords = { 'host1': "pwdofhost1", 'host2': "pwdofhost2", 'host3': "pwdofhost3", }
2. Parse into map nesting based on key , put it in deploy
In addition, the command can also be solidified into a cmds list...
For more python fabric implementation examples of remote operation and deployment related articles, please pay attention to the PHP Chinese website!
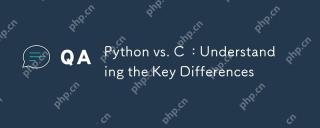
Python and C each have their own advantages, and the choice should be based on project requirements. 1) Python is suitable for rapid development and data processing due to its concise syntax and dynamic typing. 2)C is suitable for high performance and system programming due to its static typing and manual memory management.
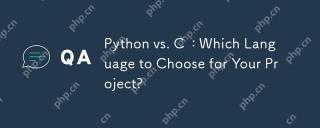
Choosing Python or C depends on project requirements: 1) If you need rapid development, data processing and prototype design, choose Python; 2) If you need high performance, low latency and close hardware control, choose C.
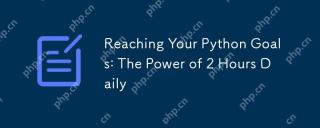
By investing 2 hours of Python learning every day, you can effectively improve your programming skills. 1. Learn new knowledge: read documents or watch tutorials. 2. Practice: Write code and complete exercises. 3. Review: Consolidate the content you have learned. 4. Project practice: Apply what you have learned in actual projects. Such a structured learning plan can help you systematically master Python and achieve career goals.
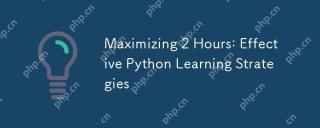
Methods to learn Python efficiently within two hours include: 1. Review the basic knowledge and ensure that you are familiar with Python installation and basic syntax; 2. Understand the core concepts of Python, such as variables, lists, functions, etc.; 3. Master basic and advanced usage by using examples; 4. Learn common errors and debugging techniques; 5. Apply performance optimization and best practices, such as using list comprehensions and following the PEP8 style guide.
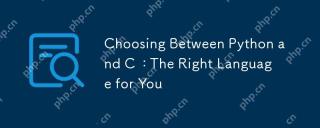
Python is suitable for beginners and data science, and C is suitable for system programming and game development. 1. Python is simple and easy to use, suitable for data science and web development. 2.C provides high performance and control, suitable for game development and system programming. The choice should be based on project needs and personal interests.
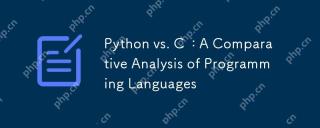
Python is more suitable for data science and rapid development, while C is more suitable for high performance and system programming. 1. Python syntax is concise and easy to learn, suitable for data processing and scientific computing. 2.C has complex syntax but excellent performance and is often used in game development and system programming.
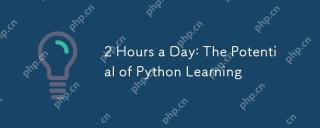
It is feasible to invest two hours a day to learn Python. 1. Learn new knowledge: Learn new concepts in one hour, such as lists and dictionaries. 2. Practice and exercises: Use one hour to perform programming exercises, such as writing small programs. Through reasonable planning and perseverance, you can master the core concepts of Python in a short time.
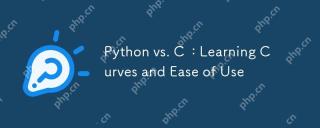
Python is easier to learn and use, while C is more powerful but complex. 1. Python syntax is concise and suitable for beginners. Dynamic typing and automatic memory management make it easy to use, but may cause runtime errors. 2.C provides low-level control and advanced features, suitable for high-performance applications, but has a high learning threshold and requires manual memory and type safety management.


Hot AI Tools

Undresser.AI Undress
AI-powered app for creating realistic nude photos

AI Clothes Remover
Online AI tool for removing clothes from photos.

Undress AI Tool
Undress images for free

Clothoff.io
AI clothes remover

Video Face Swap
Swap faces in any video effortlessly with our completely free AI face swap tool!

Hot Article

Hot Tools

MantisBT
Mantis is an easy-to-deploy web-based defect tracking tool designed to aid in product defect tracking. It requires PHP, MySQL and a web server. Check out our demo and hosting services.

SAP NetWeaver Server Adapter for Eclipse
Integrate Eclipse with SAP NetWeaver application server.

MinGW - Minimalist GNU for Windows
This project is in the process of being migrated to osdn.net/projects/mingw, you can continue to follow us there. MinGW: A native Windows port of the GNU Compiler Collection (GCC), freely distributable import libraries and header files for building native Windows applications; includes extensions to the MSVC runtime to support C99 functionality. All MinGW software can run on 64-bit Windows platforms.

PhpStorm Mac version
The latest (2018.2.1) professional PHP integrated development tool
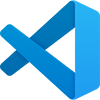
VSCode Windows 64-bit Download
A free and powerful IDE editor launched by Microsoft