


Log
Yes, it’s a log. The importance of keeping adequate logs in your application cannot be overemphasized. You should log the important stuff. If your logs are good enough, you can find the problem just by looking at the logs. That saves you a lot of time.
If you have been using print statements randomly in your code, stop immediately. Use logging.debug instead. You can continue to reuse them in the future, or disable them all, etc.
Tracking
Sometimes a better approach is to see which statements were executed. You can use some IDE's debugger to step through, but you need to know exactly which statements you are looking for, otherwise the whole process will proceed very slowly.
The trace module in the standard library can print all executed statements in the modules included in it during runtime. (Like making a project report)
python -mtrace –trace script.py
This will produce a lot of output (every line executed will be printed, you may want to use grep to filter those modules that interest you).
For example :
python -mtrace –trace script.py | egrep '^(mod1.py|mod2.py)'
Debugger
The following is a basic introduction that everyone should know now:
import pdb pdb.set_trace() # 开启pdb提示
or
try: (一段抛出异常的代码) except: import pdb pdb.pm() # 或者 pdb.post_mortem() 或者(输入 c 开始执行脚本)
python-mpdb script.py
In the input-calculation-output loop (Note: REPL, abbreviation of READ-EVAL-PRINT-LOOP) environment, the following operations can be performed:
c or continue
q or quit
l or list, display the current step frame The source code
w or where, retrace the calling process
d or down, go back one frame (note: equivalent to rollback)
u or up, go forward one frame
(Enter), repeat the previous instruction
Almost all the remaining instructions (except for a few other commands) are parsed as Python code on the current step frame.
If you feel that the challenge is not enough, you can try smiley - it can show you the variables and you can use it to remotely trace the program.
Better debugger
Direct replacement for pdb:
ipdb(easy_install ipdb) – similar to ipython (with autocomplete, display colors, etc.)
pudb(easy_install pudb) – based on curses (similar to graphical interface interface) , especially suitable for browsing source code
Remote debugger
Installation method:
sudo apt-get install winpdb
Replace the previous pdb.set_trace() with the following method:
import rpdb2 rpdb2.start_embedded_debugger("secretpassword")
Now run winpdb, File-Association
Don’t like Winpdb? You can also wrap PDB directly to run on top of TCP!
Do this:
import loggging class Rdb(pdb.Pdb): """ This will run pdb as a ephemeral telnet service. Once you connect no one else can connect. On construction this object will block execution till a client has connected. Based on https://github.com/tamentis/rpdb I think ... To use this:: Rdb(4444).set_trace() Then run: telnet 127.0.0.1 4444 """ def __init__(self, port=0): self.old_stdout = sys.stdout self.old_stdin = sys.stdin self.listen_socket = socket.socket(socket.AF_INET, socket.SOCK_STREAM) self.listen_socket.bind(('0.0.0.0', port)) if not port: logging.critical("PDB remote session open on: %s", self.listen_socket.getsockname()) print >> sys.__stderr__, "PDB remote session open on:", self.listen_socket.getsockname() sys.stderr.flush() self.listen_socket.listen(1) self.connected_socket, address = self.listen_socket.accept() self.handle = self.connected_socket.makefile('rw') pdb.Pdb.__init__(self, completekey='tab', stdin=self.handle, stdout=self.handle) sys.stdout = sys.stdin = self.handle def do_continue(self, arg): sys.stdout = self.old_stdout sys.stdin = self.old_stdin self.handle.close() self.connected_socket.close() self.listen_socket.close() self.set_continue() return 1 do_c = do_cont = do_continue def set_trace(): """ Opens a remote PDB on first available port. """ rdb = Rdb() rdb.set_trace()
Just want a REPL environment? How about trying IPython?
If you don't need a complete debugger, just start an IPython with:
import IPython IPython.embed()
Standard linux tools
I'm often surprised at how underutilized they are . You can use these tools to solve a wide range of problems: from performance problems (too many system calls, memory allocations, etc.) to deadlocks, network problems, disk problems, and more.
The most useful one is strace, which is the most direct. You only need to run sudo strace -p 12345 or strace -f command (-f means tracking the child process coming out of the fork at the same time), and that's it. The output will typically be quite large, so you may want to redirect it to a file for more analysis (just add &> to the filename).
Then there is ltrace, which is somewhat similar to strace. The difference is that it outputs library function calls. The parameters are roughly the same.
There is also lsof used to point out the meaning of the handle values you see in ltrace/strace. For example:
lsof -p 12345
Better tracking
Easy to use and can do a lot - everyone should install htop!
sudo apt-get install htop
sudo htop
Now find the processes you want and enter:
s - represents the system call process (similar to strace)
L - represents the library call process (similar to ltrace)
l - stands for lsof
Monitoring
There is no good continuous server monitoring, but if you have ever encountered some weird situations, such as why everything is running so slowly, what are those system resources going to? . . When you want to understand these problems but have nowhere to start, you don't have to use tools like iotop, iftop, htop, iostat, vmstat, just use dstat! It can do most of the things we mentioned before, and maybe even better!
It will continuously display data to you in a compact, code-highlighted way (different from iostat, vmstat), and you can often see past data (different from iftop, iostop, htop).
Just run:
dstat --cpu --io --mem --net --load --fs --vm --disk-util --disk-tps --freespace --swap --top- io --top-bio-adv
很可能有一种更简短的方式来写上面这条命令,
这是一个相当复杂而又强大的工具,但是这里我只提到了一些基本的内容(安装以及基础的命令)
sudo apt-get install gdb python-dbg
zcat /usr/share/doc/python2.7/gdbinit.gz > ~/.gdbinit
用python2.7-dbg 运行程序:
sudo gdb -p 12345
现在使用:
bt - 堆栈跟踪(C 级别)
pystack - python 堆栈跟踪,不幸的是你需要有~/.gdbinit 并且使用python-dbg
c - 继续
发生段错误?用faulthandler !
python 3.3版本以后新增的一个很棒的功能,可以向后移植到python2.x版本。只需要运行下面的语句,你就可以大抵知道什么原因引起来段错误。
import faulthandler
faulthandler.enable()
内存泄露
嗯,这种情况下有很多的工具可以使用,其中有一些专门针对WSGI的程序比如Dozer,但是我最喜欢的当然是objgraph。使用简单方便,让人惊讶!
它没有集成WSGI或者其他,所以你需要自己去发现运行代码的方法,像下面这样:
import objgraph
objs = objgraph.by_type("Request")[:15]
objgraph.show_backrefs(objs, max_depth=20, highlight=lambda v: v in objs,
filename="/tmp/graph.png")
Graph written to /tmp/objgraph-zbdM4z.dot (107 nodes)
Image generated as /tmp/graph.png
你会得到像这样一张图(注意:它非常大)。你也可以得到一张点输出。
内存使用
有时你想少用些内存。更少的内存分配常常可以使程序执行的更快,更好,用户希望内存合适好用)
有许多可用的工具,但在我看来最好用的是pytracemalloc。与其他工具相比,它开销非常小(不需要依赖于严重影响速度的sys.settrace)而且输出非常详尽。但安装起来比较痛苦,你需要重新编译python,但有了apt,做起来也非常容易。
只需要运行这些命令然后去吃顿午餐或者干点别的:
apt-get source python2.7
cd python2.7-*
wget? https://github.com/wyplay/pytracemalloc/raw/master/python2.7_track_free_list.patch
patch -p1
debuild -us -uc
cd ..
sudo dpkg -i python2.7-minimal_2.7*.deb python2.7-dev_*.deb
接着安装pytracemalloc (注意如果你在一个virtualenv虚拟环境下操作,你需要在重新安装python后再次重建 – 只需要运行 virtualenv myenv)
pip install pytracemalloc
现在像下面这样在代码里包装你的应用程序
import tracemalloc, time tracemalloc.enable() top = tracemalloc.DisplayTop( 5000, # log the top 5000 locations file=open('/tmp/memory-profile-%s' % time.time(), "w") ) top.show_lineno = True try: # code that needs to be traced finally: top.display()
输出会像这样:
2013-05-31 18:05:07: Top 5000 allocations per file and line
#1: .../site-packages/billiard/_connection.py:198: size=1288 KiB, count=70 (+0),
average=18 KiB
#2: .../site-packages/billiard/_connection.py:199: size=1288 KiB, count=70 (+0),
average=18 KiB
#3: .../python2.7/importlib/__init__.py:37: size=459 KiB, count=5958 (+0),
average=78 B
#4: .../site-packages/amqp/transport.py:232: size=217 KiB, count=6960 (+0),
average=32 B
#5: .../site-packages/amqp/transport.py:231: size=206 KiB, count=8798 (+0),
average=24 B
#6: .../site-packages/amqp/serialization.py:210: size=199 KiB, count=822 (+0),
average=248 B
#7: .../lib/python2.7/socket.py:224: size=179 KiB, count=5947 (+0), average=30
B
#8: .../celery/utils/term.py:89: size=172 KiB, count=1953 (+0), average=90 B
#9: .../site-packages/kombu/connection.py:281: size=153 KiB, count=2400 (+0),
average=65 B
#10: .../site-packages/amqp/serialization.py:462: size=147 KiB, count=4704
(+0), average=32 B
…
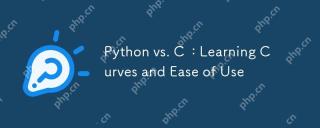
Python is easier to learn and use, while C is more powerful but complex. 1. Python syntax is concise and suitable for beginners. Dynamic typing and automatic memory management make it easy to use, but may cause runtime errors. 2.C provides low-level control and advanced features, suitable for high-performance applications, but has a high learning threshold and requires manual memory and type safety management.
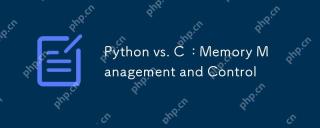
Python and C have significant differences in memory management and control. 1. Python uses automatic memory management, based on reference counting and garbage collection, simplifying the work of programmers. 2.C requires manual management of memory, providing more control but increasing complexity and error risk. Which language to choose should be based on project requirements and team technology stack.
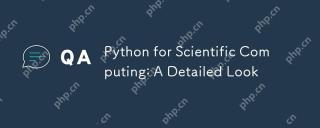
Python's applications in scientific computing include data analysis, machine learning, numerical simulation and visualization. 1.Numpy provides efficient multi-dimensional arrays and mathematical functions. 2. SciPy extends Numpy functionality and provides optimization and linear algebra tools. 3. Pandas is used for data processing and analysis. 4.Matplotlib is used to generate various graphs and visual results.
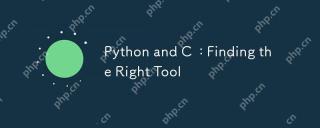
Whether to choose Python or C depends on project requirements: 1) Python is suitable for rapid development, data science, and scripting because of its concise syntax and rich libraries; 2) C is suitable for scenarios that require high performance and underlying control, such as system programming and game development, because of its compilation and manual memory management.
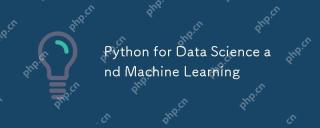
Python is widely used in data science and machine learning, mainly relying on its simplicity and a powerful library ecosystem. 1) Pandas is used for data processing and analysis, 2) Numpy provides efficient numerical calculations, and 3) Scikit-learn is used for machine learning model construction and optimization, these libraries make Python an ideal tool for data science and machine learning.
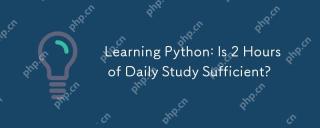
Is it enough to learn Python for two hours a day? It depends on your goals and learning methods. 1) Develop a clear learning plan, 2) Select appropriate learning resources and methods, 3) Practice and review and consolidate hands-on practice and review and consolidate, and you can gradually master the basic knowledge and advanced functions of Python during this period.
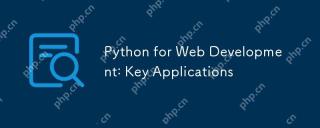
Key applications of Python in web development include the use of Django and Flask frameworks, API development, data analysis and visualization, machine learning and AI, and performance optimization. 1. Django and Flask framework: Django is suitable for rapid development of complex applications, and Flask is suitable for small or highly customized projects. 2. API development: Use Flask or DjangoRESTFramework to build RESTfulAPI. 3. Data analysis and visualization: Use Python to process data and display it through the web interface. 4. Machine Learning and AI: Python is used to build intelligent web applications. 5. Performance optimization: optimized through asynchronous programming, caching and code
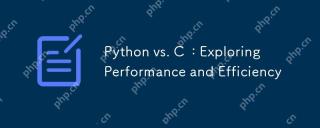
Python is better than C in development efficiency, but C is higher in execution performance. 1. Python's concise syntax and rich libraries improve development efficiency. 2.C's compilation-type characteristics and hardware control improve execution performance. When making a choice, you need to weigh the development speed and execution efficiency based on project needs.


Hot AI Tools

Undresser.AI Undress
AI-powered app for creating realistic nude photos

AI Clothes Remover
Online AI tool for removing clothes from photos.

Undress AI Tool
Undress images for free

Clothoff.io
AI clothes remover

AI Hentai Generator
Generate AI Hentai for free.

Hot Article

Hot Tools

Notepad++7.3.1
Easy-to-use and free code editor
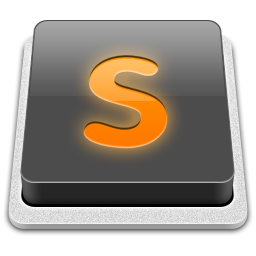
SublimeText3 Mac version
God-level code editing software (SublimeText3)
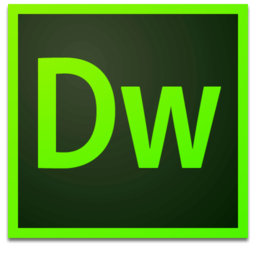
Dreamweaver Mac version
Visual web development tools
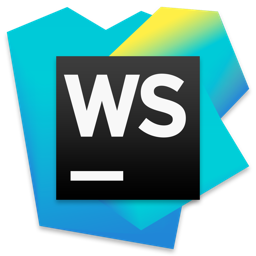
WebStorm Mac version
Useful JavaScript development tools

Zend Studio 13.0.1
Powerful PHP integrated development environment