


Foreword
In the last article, we explained the virtual edge of the image. This article starts with smoothing (that is, blurring) processing.
Basic Principles
Here is a direct reference to the relevant content of OpenCV 2.4 C smoothing processing and OpenCV 2.4 C edge gradient calculation:
Smoothing, also called blurring, is a simple and frequently used image processing method.
A filter
is required for smoothing. The most commonly used filter is the linear filter, the output pixel value of the linear filtering process (for example:) is the weighted average of the input pixel value (for example:
):
, which is just a weighting coefficient.
is called the kernel
This involves an operation called "convolution", so what is convolution?
Convolution is an operation performed between each image block and a certain operator (kernel).
Nuclear? !
nbsp;dsds
The kernel is a fixed-size numerical array. The array has an anchor point
, which is usually located in the center of the array.
But how does this work?
If you want to get the convolution value at a specific position of the image, you can calculate it with the following method:
Place the anchor point of the kernel on the pixel at that specific position, and at the same time, other values in the kernel coincide with each pixel in the neighborhood of the pixel; multiply each value in the kernel by the corresponding pixel value, and add the products Add; place the result on the pixel corresponding to the anchor point; repeat the above process for all pixels in the image.
The above process is represented by the formula as follows:
What about convolution on the edge of the image?
Before calculating the convolution, you need to create virtual pixels by copying the boundary of the source image, so that there are enough pixels at the edge to calculate the convolution. This is why the previous article required a virtual edge function.
Mean Smoothing
Mean smoothing is actually a convolution operation in which the kernel elements are all 1, and then divided by the size of the kernel. The mathematical expression is:
Let’s implement the mean smoothing function blur:
function blur(__src, __size1, __size2, __borderType, __dst){
if(__src.type && __src.type == "CV_RGBA"){
var height = __src.row,
width = __src. col,
dst = __dst || new Mat(height, width, CV_RGBA),
dstData = dst.data;
var size1 = __size1 || 3,
size2 = __size2 || size1,
size = size1 * size2;
if(size1 % 2 !== 1 || size2 % 2 !== 1){
console.error("size must be an odd number");
return __src;
}
var startX = Math.floor(size1 / 2),
startY = Math.floor(size2 / 2);
var withBorderMat = copyMakeBorder(__src, startY, startX , 0, 0, __borderType),
mData = withBorderMat.data,
mWidth = withBorderMat.col;
var newValue, nowX, offsetY, offsetI;
var i, j, c , y, x;
for(i = height; i--;){
offsetI = i * width;
for(j = width; j--;){
for(c = 3; c--;){
newValue = 0;
for(y = size2; y--;){
offsetY = (y i) * mWidth * 4;
for(x = size1; offsetI) * 4 c] = newValue / size;
}
dstData[(j offsetI) * 4 3] = mData[offsetY startY * mWidth * 4 (j startX) * 4 3];
}
}
}else{
console.error("Type not supported. ");
}
return dst;
}
Where size1 and size2 are the horizontal and vertical sizes of the core respectively, and must be positive odd numbers.
Gaussian smoothing
The most useful filter (although not the fastest). Gaussian filtering is to convolve each pixel of the input array with a Gaussian kernel
and treat the convolution sum as the output pixel value.Referring to the one-dimensional Gaussian function, we can see that it is a function that is large in the middle and small on both sides.
So the weighting number of the Gaussian filter is large in the middle and small around it.
The two-dimensional Gaussian function is:
where




Here we refer to the implementation of OpenCV, but there should be room for optimization because the separation filter has not been used yet.
First we make a getGaussianKernel to return a one-dimensional array of Gaussian filters.
function getGaussianKernel(__n, __sigma){
var SMALL_GAUSSIAN_SIZE = 7,
smallGaussianTab = [[1],
[0.25, 0.5, 0.25],
[0.0625, 0.25, 0.375, 0.25, 0.0625],
[0.03125, 0.10937 5,0.21875, 0.28125, 0.21875, 0.109375, 0.03125]
];
var fixedKernel = __n & 2 == 1 && __n & gt; 1] : 0;
var sigmaX = __sigma > 0 ? __sigma : ((__n - 1) * 0.5 - 1) * 0.3 0.8,
scale2X = -0.5 / (sigmaX * sigmaX),
sum = 0;
var i, x, t, kernel = [];
for(i = 0; i x = i - (__n - 1) * 0.5;
t = fixedKernel ? fixedKernel[i] : Math.exp(scale2X * x * x);
kernel[i] = t;
sum = t;
}
sum = 1 / sum;
for(i = __n; i--;){
kernel[i] *= sum;
}
return kernel;
};
Then through two one-dimensional arrays, a complete Gaussian kernel can be calculated, and then using the loop method used in blur, The Gaussian smoothed matrix can be calculated.
function GaussianBlur(__src, __size1, __size2, __sigma1, __sigma2, __borderType, __dst){
if(__src.type && __src.type == "CV_RGBA"){
var height = __src.row,
width = __src.col,
dst = __dst || new Mat(height, width, CV_RGBA),
dstData = dst.data;
var sigma1 = __sigma1 || 0,
sigma2 = __sigma2 || __sigma1;
var size1 = __size1 || Math.round(sigma1 * 6 1) | 1,
size2 = __size2 || Math.round(sigma2 * 6 1) | 1,
size = size1 * size2;
if(size1 % 2 !== 1 || size2 % 2 !== 1){
console.error("size必须是奇数。");
return __src;
}
var startX = Math.floor(size1 / 2),
startY = Math.floor(size2 / 2);
var withBorderMat = copyMakeBorder(__src, startY, startX, 0, 0, __borderType),
mData = withBorderMat.data,
mWidth = withBorderMat.col;
var kernel1 = getGaussianKernel(size1, sigma1),
kernel2,
kernel = new Array(size1 * size2);
if(size1 === size2 && sigma1 === sigma2)
kernel2 = kernel1;
else
kernel2 = getGaussianKernel(size2, sigma2);
var i, j, c, y, x;
for(i = kernel2.length; i--;){
for(j = kernel1.length; j--;){
kernel[i * size1 j] = kernel2[i] * kernel1[j];
}
}
var newValue, nowX, offsetY, offsetI;
for(i = height; i--;){
offsetI = i * width;
for(j = width; j--;){
for(c = 3; c--;){
newValue = 0;
for(y = size2; y--;){
offsetY = (y i) * mWidth * 4;
for(x = size1; x--;){
nowX = (x j) * 4 c;
newValue = (mData[offsetY nowX] * kernel[y * size1 x]);
}
}
dstData[(j offsetI) * 4 c] = newValue;
}
dstData[(j offsetI) * 4 3] = mData[offsetY startY * mWidth * 4 (j startX) * 4 3];
}
}
}else{
console.error("不支持的类型");
}
return dst;
}
中值平滑
中值滤波将图像的每个像素用邻域 (以当前像素为中心的正方形区域)像素的
中值代替 。依然使用blur里面用到的循环,只要得到核中的所有值,再通过sort排序便可以得到中值,然后锚点由该值替代。
function medianBlur(__src, __size1, __size2, __borderType, __dst){
if(__src.type && __src.type == "CV_RGBA"){
var height = __src.row,
width = __src.col,
dst = __dst || new Mat(height, width, CV_RGBA),
dstData = dst.data;
var size1 = __size1 || 3,
size2 = __size2 || size1,
size = size1 * size2;
if(size1 % 2 !== 1 || size2 % 2 !== 1){
console.error("size必须是奇数");
return __src;
}
var startX = Math.floor(size1 / 2),
startY = Math.floor(size2 / 2);
var withBorderMat = copyMakeBorder(__src, startY, startX, 0, 0, __borderType),
mData = withBorderMat.data,
mWidth = withBorderMat.col;
var newValue = [], nowX, offsetY, offsetI;
var i, j, c, y, x;
for(i = height; i--;){
offsetI = i * width;
for(j = width; j--;){
for(c = 3; c--;){
for(y = size2; y--;){
offsetY = (y i) * mWidth * 4;
for(x = size1; x--;){
nowX = (x j) * 4 c;
newValue[y * size1 x] = mData[offsetY nowX];
}
}
newValue.sort();
dstData[(j offsetI) * 4 c] = newValue[Math.round(size / 2)];
}
dstData[(j offsetI) * 4 3] = mData[offsetY startY * mWidth * 4 (j startX) * 4 3];
}
}
}else{
console.error("类型不支持");
}
return dst;
};
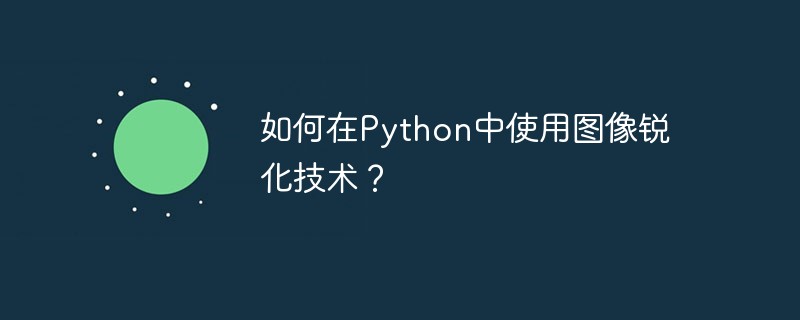
图像锐化是一种常用的图像处理技术,它能够使图片变得更加清晰和细节明显。在Python中,我们可以使用一些常见的图像处理库来实现图像锐化功能。本文将介绍如何使用Python中的Pillow库、OpenCV库和Scikit-Image库进行图像锐化。使用Pillow库进行图像锐化Pillow库是Python中常用的图像处理库,其提供了PIL(PythonIma
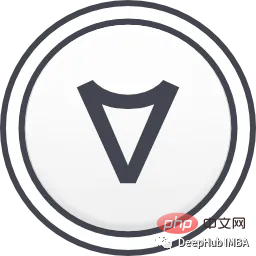
图像处理已经成为我们日常生活中不可或缺的一部分,涉及到社交媒体和医学成像等各个领域。通过数码相机或卫星照片和医学扫描等其他来源获得的图像可能需要预处理以消除或增强噪声。频域滤波是一种可行的解决方案,它可以在增强图像锐化的同时消除噪声。快速傅里叶变换(FFT)是一种将图像从空间域变换到频率域的数学技术,是图像处理中进行频率变换的关键工具。通过利用图像的频域表示,我们可以根据图像的频率内容有效地分析图像,从而简化滤波程序的应用以消除噪声。本文将讨论图像从FFT到逆FFT的频率变换所涉及的各个阶段,并
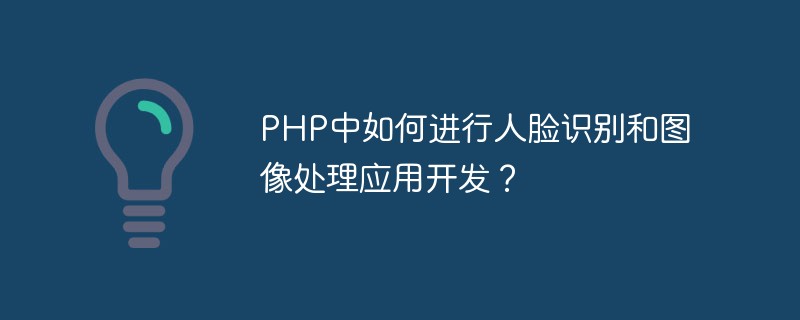
在当今数字化时代,图像处理技术已成为了一种必备的技能,而人脸识别技术则被广泛应用于各行各业。其中,PHP作为一门广泛应用于web开发的脚本语言,其在人脸识别和图像处理应用开发方面的技术初步成熟,而其开发工具和框架也在不断发展。本文将给大家介绍PHP中如何进行图像处理和人脸识别技术的应用开发。I.图像处理应用开发GD库GD库是PHP中非常重要的一个图像处理工
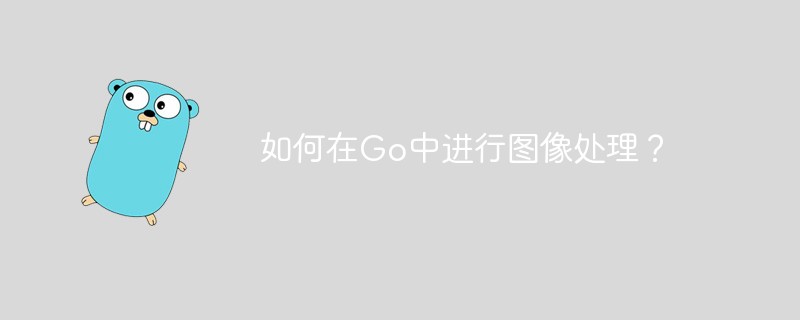
作为一门高效的编程语言,Go在图像处理领域也有着不错的表现。虽然Go本身的标准库中没有提供专门的图像处理相关的API,但是有一些优秀的第三方库可以供我们使用,比如GoCV、ImageMagick和GraphicsMagick等。本文将重点介绍使用GoCV进行图像处理的方法。GoCV是一个高度依赖于OpenCV的Go语言绑定库,其
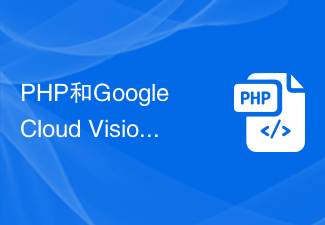
PHP是一种广泛使用的开放源代码的服务器端编程语言。它在网站开发二维图形处理和图片渲染技术方面广受欢迎。要实现有关图像和视觉数据的处理,我们可以使用GoogleCloudVisionAPI以及PHP。GoogleCloudVisionAPI是一个灵活的计算机视觉API,它可以帮助开发者更轻松地构建各种机器视觉应用程序。它支持图像标记、面部识别、文
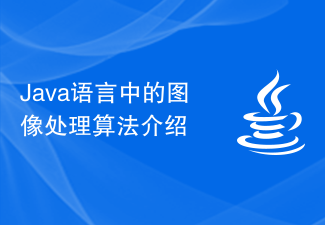
Java语言中的图像处理算法介绍随着数字化时代的到来,图像处理已经成为了计算机科学中的一个重要分支。在计算机中,图像是以数字形式存储的,而图像处理则是通过对这些数字进行一系列的算法运算,改变图像的质量和外观。Java语言作为一种跨平台的编程语言,其丰富的图像处理库和强大的算法支持,使得它成为了很多开发者的首选。本文将介绍Java语言中常用的图像处理算法,以及
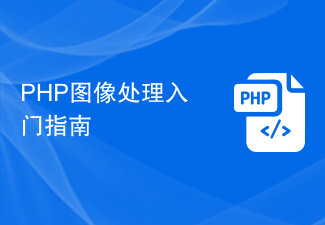
PHP是一种非常流行的服务器端编程语言,它被广泛用于Web开发。在Web开发中,图像处理是一个非常常见的需求,而在PHP中实现图像处理也是很简单的。本文将简要介绍PHP图像处理的入门指南。一、环境要求要使用PHP图像处理,首先需要PHPGD库的支持。该库提供了将图像写入文件或输出到浏览器的功能、裁剪和缩放图像、添加文字、以及使图像变为灰度或反转等功能。因此
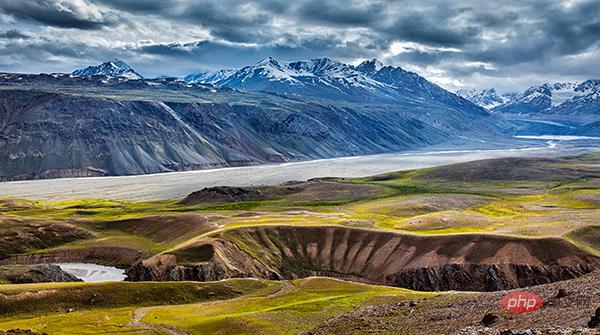
当今世界充满了各种数据,而图像是其中高的重要组成部分。然而,若想其有所应用,我们需要对这些图像进行处理。图像处理是分析和操纵数字图像的过程,旨在提高其质量或从中提取一些信息,然后将其用于某些方面。图像处理中的常见任务包括显示图像,基本操作(如裁剪、翻转、旋转等),图像分割,分类和特征提取,图像恢复和图像识别等。Python之成为图像处理任务的最佳选择,是因为这一科学编程语言日益普及,并且其自身免费提供许多最先进的图像处理工具。让我们看一下用于图像处理任务的一些常用Python库。1、scikit


Hot AI Tools

Undresser.AI Undress
AI-powered app for creating realistic nude photos

AI Clothes Remover
Online AI tool for removing clothes from photos.

Undress AI Tool
Undress images for free

Clothoff.io
AI clothes remover

AI Hentai Generator
Generate AI Hentai for free.

Hot Article

Hot Tools
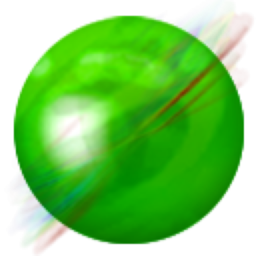
ZendStudio 13.5.1 Mac
Powerful PHP integrated development environment

mPDF
mPDF is a PHP library that can generate PDF files from UTF-8 encoded HTML. The original author, Ian Back, wrote mPDF to output PDF files "on the fly" from his website and handle different languages. It is slower than original scripts like HTML2FPDF and produces larger files when using Unicode fonts, but supports CSS styles etc. and has a lot of enhancements. Supports almost all languages, including RTL (Arabic and Hebrew) and CJK (Chinese, Japanese and Korean). Supports nested block-level elements (such as P, DIV),

SecLists
SecLists is the ultimate security tester's companion. It is a collection of various types of lists that are frequently used during security assessments, all in one place. SecLists helps make security testing more efficient and productive by conveniently providing all the lists a security tester might need. List types include usernames, passwords, URLs, fuzzing payloads, sensitive data patterns, web shells, and more. The tester can simply pull this repository onto a new test machine and he will have access to every type of list he needs.
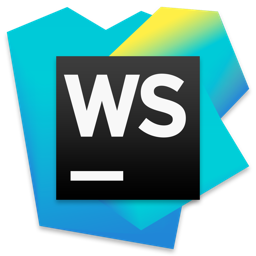
WebStorm Mac version
Useful JavaScript development tools

DVWA
Damn Vulnerable Web App (DVWA) is a PHP/MySQL web application that is very vulnerable. Its main goals are to be an aid for security professionals to test their skills and tools in a legal environment, to help web developers better understand the process of securing web applications, and to help teachers/students teach/learn in a classroom environment Web application security. The goal of DVWA is to practice some of the most common web vulnerabilities through a simple and straightforward interface, with varying degrees of difficulty. Please note that this software
