


What is KL Divergence that Revolutionized Machine Learning? - Analytics Vidhya
Kullback-Leibler (KL) Divergence: A Deep Dive into Relative Entropy
Few mathematical concepts have as profoundly impacted modern machine learning and artificial intelligence as Kullback-Leibler (KL) divergence. This powerful metric, also known as relative entropy or information gain, is now essential across numerous fields, from statistical inference to the cutting edge of deep learning. This article explores KL divergence, its origins, applications, and its crucial role in the age of big data and AI.
Key Takeaways
- KL divergence quantifies the difference between two probability distributions.
- It's a game-changer in machine learning and information theory, requiring only two probability distributions for calculation.
- It measures the extra information needed to encode data from one distribution using the code optimized for another.
- KL divergence is vital in training advanced generative models like diffusion models, optimizing noise distribution, and improving text-to-image generation.
- Its strong theoretical foundation, flexibility, scalability, and interpretability make it invaluable for complex models.
Table of Contents
- Introduction to KL Divergence
- KL Divergence: Essential Components and Transformative Influence
- Understanding KL Divergence: A Step-by-Step Guide
- KL Divergence in Diffusion Models: A Revolutionary Application
- Advantages of KL Divergence
- Real-World Applications of KL Divergence
- Frequently Asked Questions
Introduction to KL Divergence
KL divergence measures the disparity between two probability distributions. Consider two models predicting the same event – their differences can be quantified using KL divergence.
For discrete probability distributions P and Q, the KL divergence from Q to P is:
This formula, while initially complex, is intuitively understood as the average extra information needed to encode data from P using a code optimized for Q.
KL Divergence: Essential Components and Transformative Influence
Calculating KL divergence requires:
- Two probability distributions defined over the same event space.
- A logarithmic function (base 2 or natural logarithm).
With these simple inputs, KL divergence has revolutionized various fields:
- Machine Learning: Crucial in variational inference and generative models (e.g., VAEs), measuring how well a model approximates the true data distribution.
- Information Theory: Provides a fundamental measure of information content and compression efficiency.
- Statistical Inference: Essential in hypothesis testing and model selection.
- Natural Language Processing: Used in topic modeling and language model evaluation.
- Reinforcement Learning: Aids in policy optimization and exploration strategies.
Understanding KL Divergence: A Step-by-Step Guide
Let's dissect KL divergence:
- Probability Comparison: We compare the probability of each event under distributions P and Q.
- Ratio Calculation: We compute the ratio P(x)/Q(x), showing how much more (or less) likely each event is under P compared to Q.
- Logarithmic Transformation: The logarithm of this ratio ensures non-negativity and that the divergence is zero only when P and Q are identical.
- Weighting: We weight the log ratio by P(x), emphasizing events more likely under P.
- Summation: Finally, we sum the weighted log ratios across all events.
The result is a single value representing the difference between P and Q. Note that KL divergence is asymmetric: DKL(P || Q) ≠ DKL(Q || P). This asymmetry is a key feature, indicating the direction of the difference.
KL Divergence in Diffusion Models: A Revolutionary Application
Diffusion models, like DALL-E 2 and Stable Diffusion, are a prime example of KL divergence's power. They generate remarkably realistic images from text descriptions.
KL divergence's role in diffusion models includes:
- Training: Measures the difference between the true and estimated noise distributions at each step, enabling the model to effectively reverse the diffusion process.
- Variational Lower Bound: Often used in the training objective, ensuring generated samples closely match the data distribution.
- Latent Space Regularization: Helps regularize the latent space, ensuring well-behaved representations.
- Model Comparison: Used to compare different diffusion model architectures.
- Conditional Generation: In text-to-image models, it measures how well generated images match text descriptions.
Advantages of KL Divergence
KL divergence's strengths include:
- Strong Theoretical Basis: Grounded in information theory, offering interpretability in terms of information bits.
- Flexibility: Applicable to both discrete and continuous distributions.
- Scalability: Effective in high-dimensional spaces, suitable for complex machine learning models.
- Mathematical Properties: Satisfies non-negativity and convexity, beneficial for optimization.
- Interpretability: Its asymmetry is intuitively understood in terms of encoding and compression.
Real-World Applications of KL Divergence
KL divergence's impact extends to various applications:
- Recommendation Systems: Used to measure how well models predict user preferences.
- Image Generation: Essential in training AI image generation models.
- Language Models: Plays a role in training chatbots and other language models.
- Climate Modeling: Used to compare and assess the reliability of climate models.
- Financial Risk Assessment: Utilized in risk models for market prediction.
Conclusion
KL divergence is a powerful tool that extends beyond pure mathematics, impacting machine learning, market predictions, and more. Its importance in our data-driven world is undeniable. As AI and data analysis advance, KL divergence's role will only become more significant.
Frequently Asked Questions
Q1. What does “KL” stand for? A: Kullback-Leibler, named after Solomon Kullback and Richard Leibler.
Q2. Is KL divergence a distance metric? A: No, its asymmetry prevents it from being a true distance metric.
Q3. Can KL divergence be negative? A: No, it's always non-negative.
Q4. How is KL divergence used in machine learning? A: In model selection, variational inference, and evaluating generative models.
Q5. What’s the difference between KL divergence and cross-entropy? A: Minimizing cross-entropy is equivalent to minimizing KL divergence plus the entropy of the true distribution.
The above is the detailed content of What is KL Divergence that Revolutionized Machine Learning? - Analytics Vidhya. For more information, please follow other related articles on the PHP Chinese website!
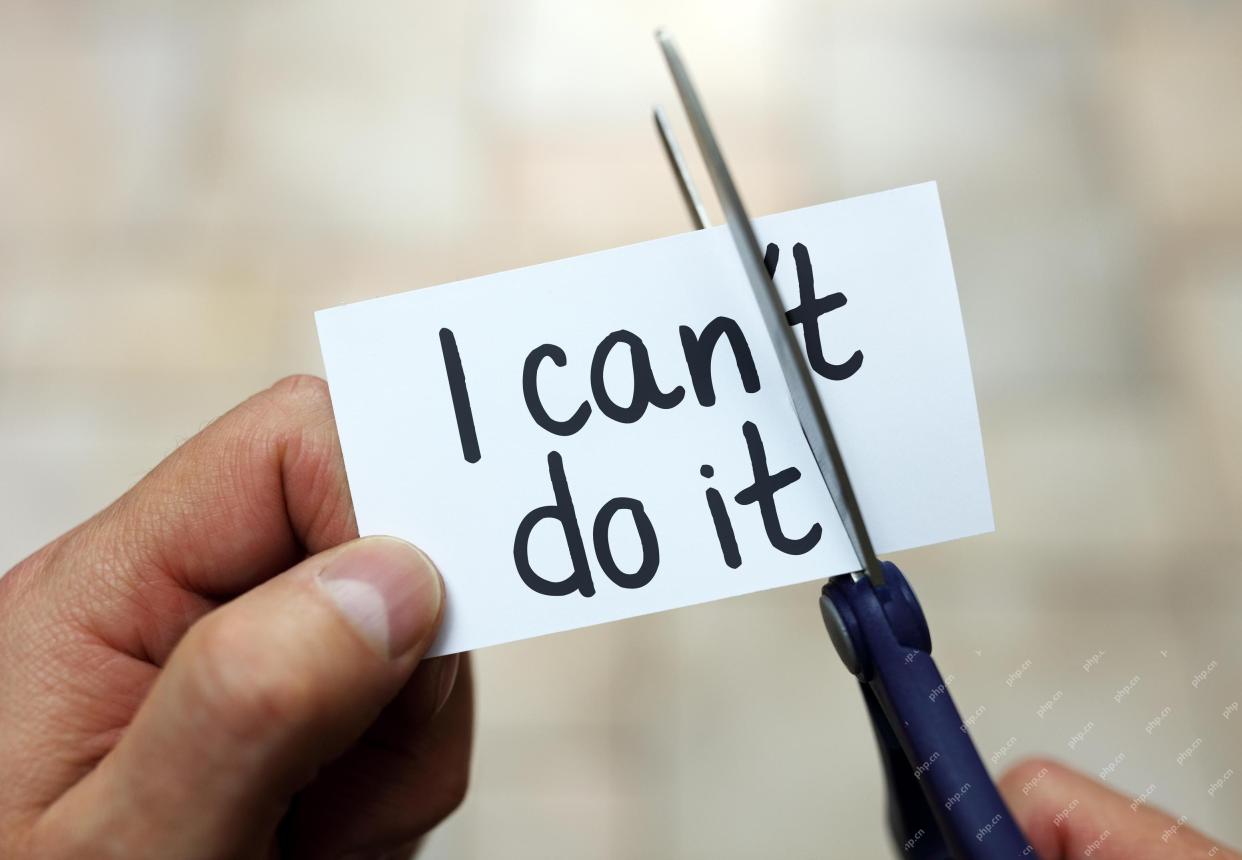
This article explores the growing concern of "AI agency decay"—the gradual decline in our ability to think and decide independently. This is especially crucial for business leaders navigating the increasingly automated world while retainin
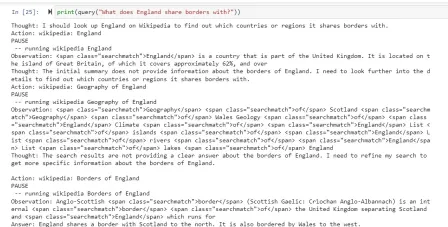
Ever wondered how AI agents like Siri and Alexa work? These intelligent systems are becoming more important in our daily lives. This article introduces the ReAct pattern, a method that enhances AI agents by combining reasoning an
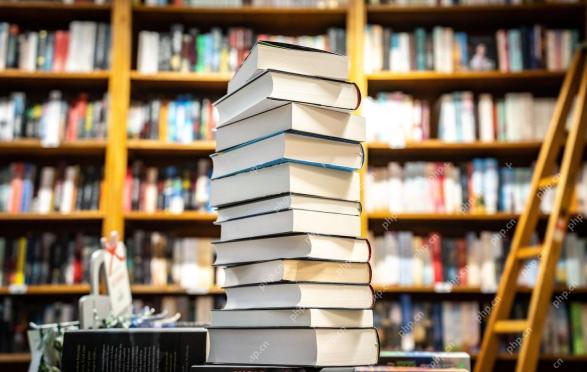
"I think AI tools are changing the learning opportunities for college students. We believe in developing students in core courses, but more and more people also want to get a perspective of computational and statistical thinking," said University of Chicago President Paul Alivisatos in an interview with Deloitte Nitin Mittal at the Davos Forum in January. He believes that people will have to become creators and co-creators of AI, which means that learning and other aspects need to adapt to some major changes. Digital intelligence and critical thinking Professor Alexa Joubin of George Washington University described artificial intelligence as a “heuristic tool” in the humanities and explores how it changes
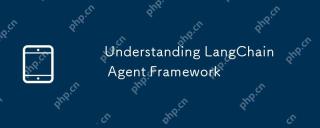
LangChain is a powerful toolkit for building sophisticated AI applications. Its agent architecture is particularly noteworthy, allowing developers to create intelligent systems capable of independent reasoning, decision-making, and action. This expl
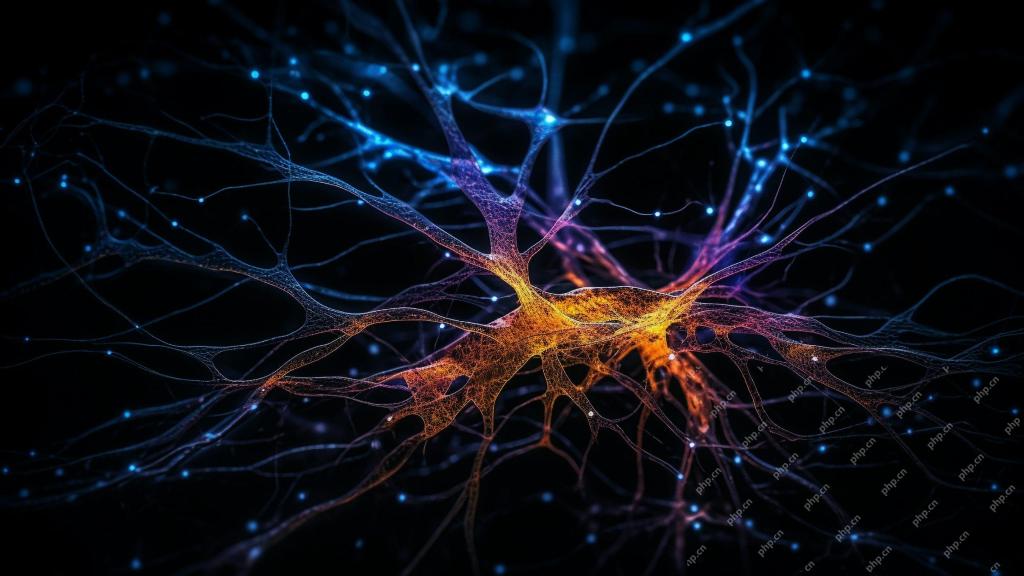
Radial Basis Function Neural Networks (RBFNNs): A Comprehensive Guide Radial Basis Function Neural Networks (RBFNNs) are a powerful type of neural network architecture that leverages radial basis functions for activation. Their unique structure make
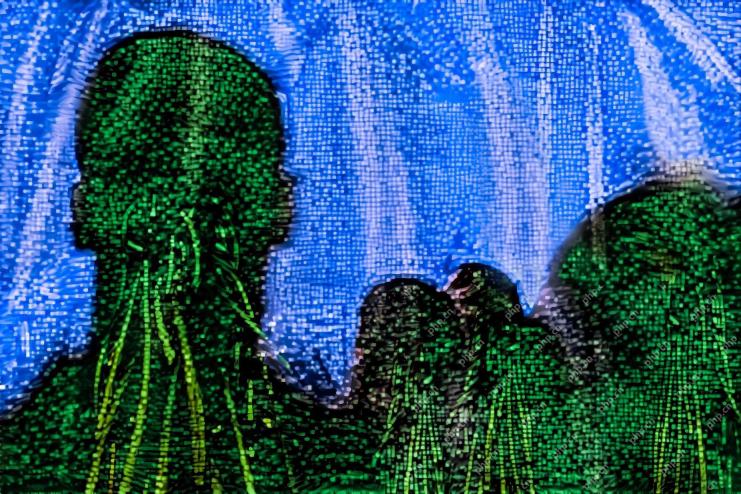
Brain-computer interfaces (BCIs) directly link the brain to external devices, translating brain impulses into actions without physical movement. This technology utilizes implanted sensors to capture brain signals, converting them into digital comman
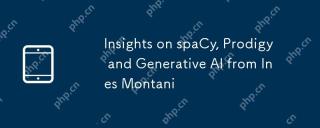
This "Leading with Data" episode features Ines Montani, co-founder and CEO of Explosion AI, and co-developer of spaCy and Prodigy. Ines offers expert insights into the evolution of these tools, Explosion's unique business model, and the tr
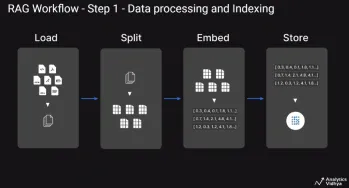
This article explores Retrieval Augmented Generation (RAG) systems and how AI agents can enhance their capabilities. Traditional RAG systems, while useful for leveraging custom enterprise data, suffer from limitations such as a lack of real-time dat


Hot AI Tools

Undresser.AI Undress
AI-powered app for creating realistic nude photos

AI Clothes Remover
Online AI tool for removing clothes from photos.

Undress AI Tool
Undress images for free

Clothoff.io
AI clothes remover

Video Face Swap
Swap faces in any video effortlessly with our completely free AI face swap tool!

Hot Article

Hot Tools

MantisBT
Mantis is an easy-to-deploy web-based defect tracking tool designed to aid in product defect tracking. It requires PHP, MySQL and a web server. Check out our demo and hosting services.

PhpStorm Mac version
The latest (2018.2.1) professional PHP integrated development tool

MinGW - Minimalist GNU for Windows
This project is in the process of being migrated to osdn.net/projects/mingw, you can continue to follow us there. MinGW: A native Windows port of the GNU Compiler Collection (GCC), freely distributable import libraries and header files for building native Windows applications; includes extensions to the MSVC runtime to support C99 functionality. All MinGW software can run on 64-bit Windows platforms.

mPDF
mPDF is a PHP library that can generate PDF files from UTF-8 encoded HTML. The original author, Ian Back, wrote mPDF to output PDF files "on the fly" from his website and handle different languages. It is slower than original scripts like HTML2FPDF and produces larger files when using Unicode fonts, but supports CSS styles etc. and has a lot of enhancements. Supports almost all languages, including RTL (Arabic and Hebrew) and CJK (Chinese, Japanese and Korean). Supports nested block-level elements (such as P, DIV),
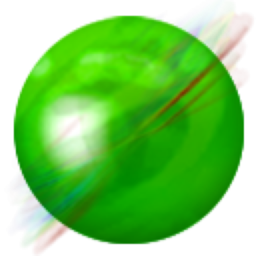
ZendStudio 13.5.1 Mac
Powerful PHP integrated development environment