Predictive Maintenance for Jet Engines: A Machine Learning Approach
Imagine a future where jet engine failures are predicted before they occur, saving millions and potentially lives. This research uses NASA's jet engine simulation data to explore a novel predictive maintenance method. We'll demonstrate how machine learning analyzes sensor data (temperature, pressure, etc.) to assess engine health, showcasing AI's potential to revolutionize maintenance and improve safety. This involves data preparation, feature selection, and sophisticated algorithms like Random Forest and Neural Networks.
Key Learning Points:
- Forecasting equipment failures using AI and machine learning.
- Preparing and processing complex sensor data for analysis.
- Practical application of Random Forest and Neural Networks for predictive modeling.
- Feature selection and engineering to boost model accuracy.
- Improving safety and operational efficiency through predictive maintenance.
(This article is part of the Data Science Blogathon.)
Table of Contents:
- Dataset Overview
- Business Understanding
- Data Exploration
- Data Preprocessing
- Modeling and Evaluation
- Frequently Asked Questions
Dataset Overview
NASA's publicly available jet engine simulation dataset contains sensor readings from engine operation until failure. We'll analyze these patterns to classify engine health (normal or failed). This project utilizes the CRISP-DM methodology for a structured data mining process.
Business Understanding
This section outlines the project's context, challenges, and goals.
Importance of Failure Prediction: Jet engines are critical in aerospace, powering aircraft and generating thrust. Predictive maintenance prevents catastrophic failures, enhancing safety. Engine performance is monitored via sensors measuring temperature, pressure, vibration, and other parameters. This project analyzes sensor data to predict engine health proactively.
Problem: Unforeseen engine failures pose significant risks.
Objective: Classify engine health (normal/failure) based on sensor data.
Data Exploration
This stage involves initial data examination.
Dataset Details: The project uses the train_FD001.txt
file from the CMAPSS Jet Engine Simulated Data, containing 26 columns and 20,631 data points.
Feature Description:
Parameter | Symbol | Description | Unit |
---|---|---|---|
Engine | – | – | – |
Cycle | – | – | t |
Setting 1 | – | Altitude | ft |
Setting 2 | – | Mach Number | M |
Setting 3 | – | Sea-level Temperature | °F |
Sensor 1 | T2 | Total temperature at fan inlet | °R |
Sensor 2 | T24 | Total temperature at LPC outlet | °R |
... | ... | ... | ... |
Raw Data Inspection: Initial data inspection reveals unnamed columns and NaN values, requiring cleaning during preprocessing.
Data Preprocessing
This stage focuses on data cleaning and preparation for modeling.
Handling NaN values and renaming columns: NaN values are removed, and columns are renamed for clarity.
Statistical Summary: Descriptive statistics are calculated to identify potential issues like constant-value columns (removed to improve efficiency).
Constant Value Removal: A custom function identifies and removes columns with constant values.
Target Variable Creation: A 'status' column is created (0=normal, 1=failure) using a threshold (20 cycles remaining) to indicate impending failure.
Feature Correlation (Heatmap): A heatmap visualizes feature correlations with the target variable, using a threshold of 0.2 to identify relevant features.
Feature Selection: Features with a correlation value below the threshold are removed.
Class Imbalance and SMOTE: The dataset shows class imbalance (more normal than failure instances). SMOTE (Synthetic Minority Oversampling Technique) is used to oversample the minority class, balancing the dataset for training.
Data Splitting and Scaling: The data is split into training (80%) and testing (20%) sets. Z-score standardization is applied to the training data to scale features.
Modeling and Evaluation
This section details model building, training, and evaluation.
Random Forest Model: A Random Forest Classifier is trained on the preprocessed data, and predictions are made on the test set. The model's performance is evaluated using accuracy, precision, recall, F1-score, and a confusion matrix.
Artificial Neural Network (ANN) Model: An ANN model is built using TensorFlow/Keras, trained, and evaluated using similar metrics as the Random Forest model.
Conclusion
This research demonstrates the effectiveness of machine learning in predictive maintenance for jet engines. Random Forest and ANN models accurately predict potential failures, improving safety and efficiency. The results highlight the importance of data preprocessing and feature selection for accurate predictions. This work sets a precedent for applying predictive analytics in various industries. (Full code available on GitHub).
Key Takeaways:
- Predictive maintenance is crucial for jet engine reliability and safety.
- Machine learning models effectively forecast engine failures.
- Data preparation and feature selection are vital for model accuracy.
- NASA's data provides valuable resources for aviation predictive maintenance.
- This approach is applicable across various industries.
Frequently Asked Questions:
- Q1. What is predictive maintenance for jet engines? A. It uses data analysis to predict potential failures, enabling proactive maintenance.
- Q2. Why is it important? A. It improves safety, reduces downtime, and lowers costs.
- Q3. What models are used? A. Random Forest, Neural Networks, and others.
- Q4. How does NASA contribute? A. NASA provides valuable simulation data for model development.
(Note: Images used are not owned by the model and are used at the author's discretion.)
The above is the detailed content of CMAPSS Jet Engine Failure Classification Based On Sensor Data. For more information, please follow other related articles on the PHP Chinese website!
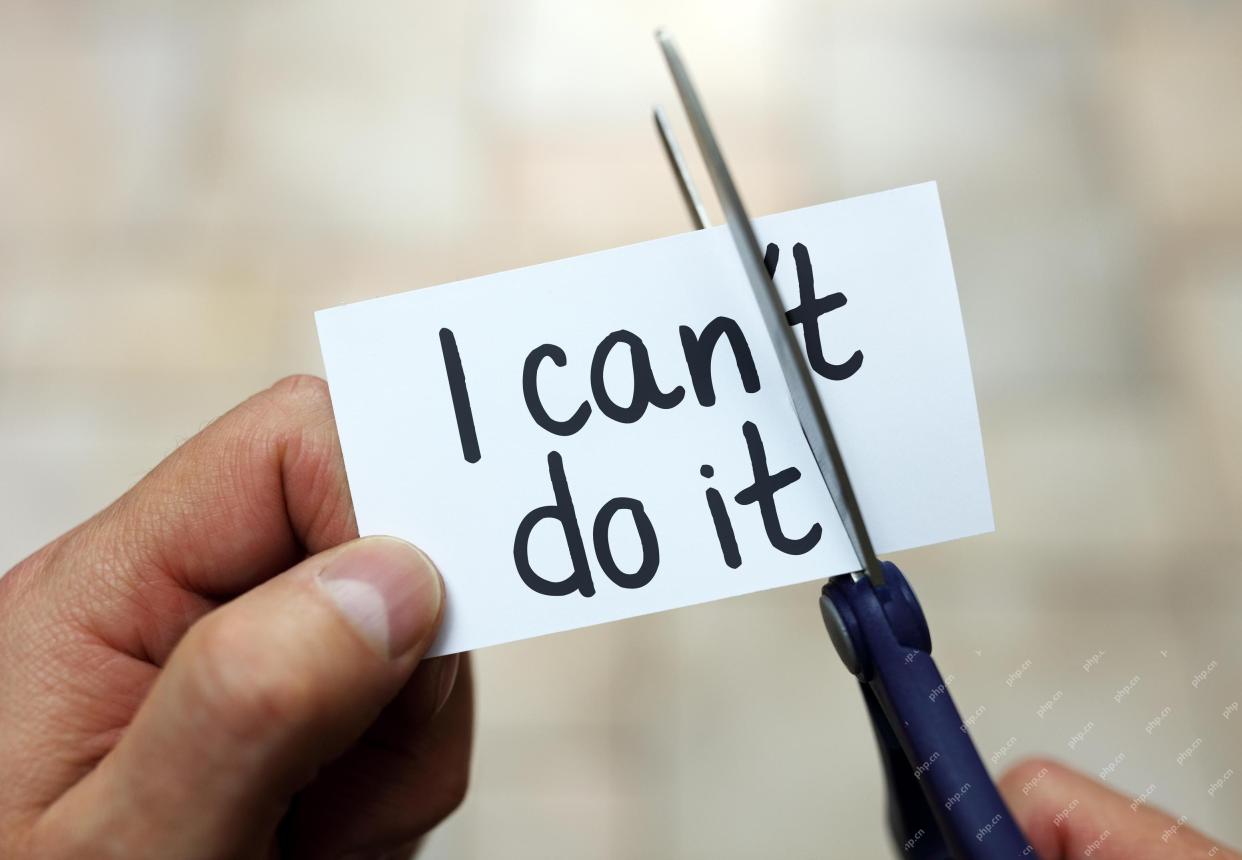
This article explores the growing concern of "AI agency decay"—the gradual decline in our ability to think and decide independently. This is especially crucial for business leaders navigating the increasingly automated world while retainin
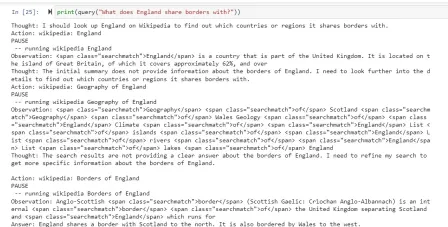
Ever wondered how AI agents like Siri and Alexa work? These intelligent systems are becoming more important in our daily lives. This article introduces the ReAct pattern, a method that enhances AI agents by combining reasoning an
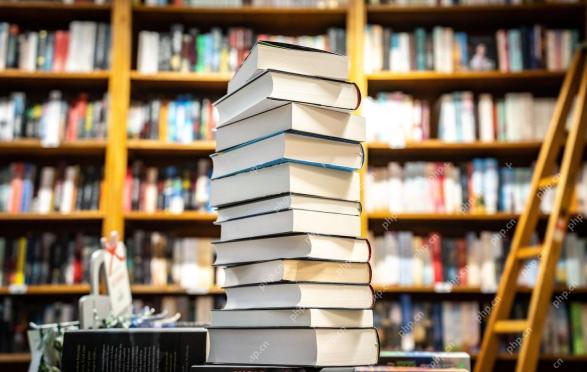
"I think AI tools are changing the learning opportunities for college students. We believe in developing students in core courses, but more and more people also want to get a perspective of computational and statistical thinking," said University of Chicago President Paul Alivisatos in an interview with Deloitte Nitin Mittal at the Davos Forum in January. He believes that people will have to become creators and co-creators of AI, which means that learning and other aspects need to adapt to some major changes. Digital intelligence and critical thinking Professor Alexa Joubin of George Washington University described artificial intelligence as a “heuristic tool” in the humanities and explores how it changes
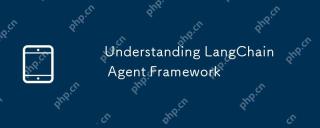
LangChain is a powerful toolkit for building sophisticated AI applications. Its agent architecture is particularly noteworthy, allowing developers to create intelligent systems capable of independent reasoning, decision-making, and action. This expl
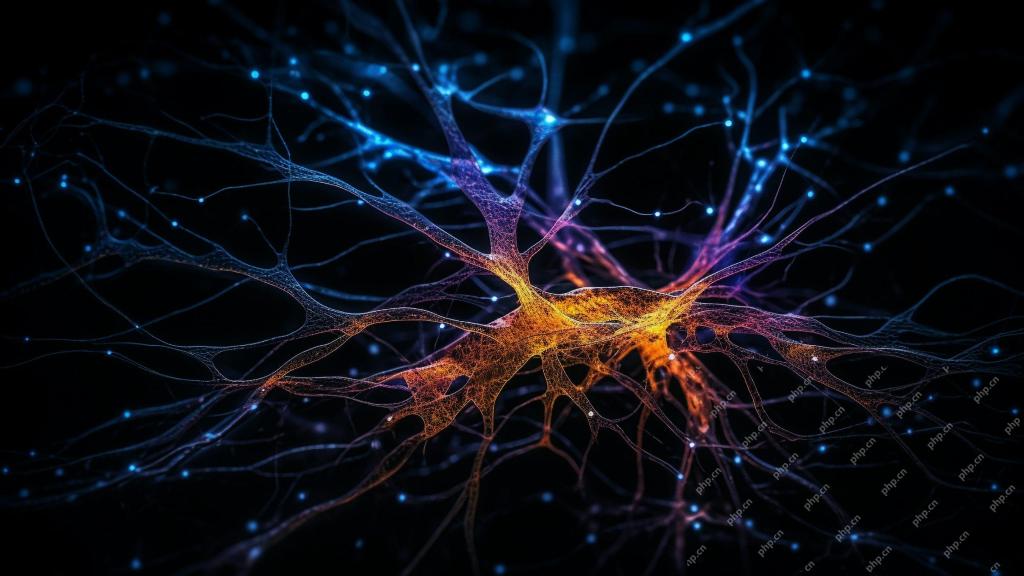
Radial Basis Function Neural Networks (RBFNNs): A Comprehensive Guide Radial Basis Function Neural Networks (RBFNNs) are a powerful type of neural network architecture that leverages radial basis functions for activation. Their unique structure make
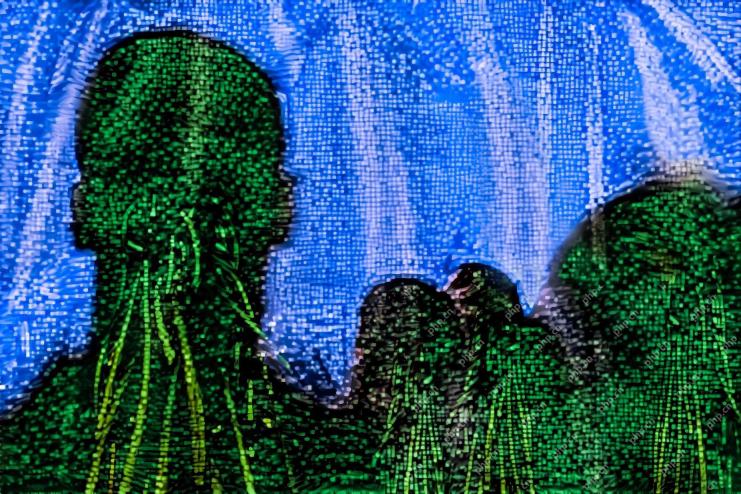
Brain-computer interfaces (BCIs) directly link the brain to external devices, translating brain impulses into actions without physical movement. This technology utilizes implanted sensors to capture brain signals, converting them into digital comman
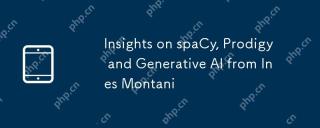
This "Leading with Data" episode features Ines Montani, co-founder and CEO of Explosion AI, and co-developer of spaCy and Prodigy. Ines offers expert insights into the evolution of these tools, Explosion's unique business model, and the tr
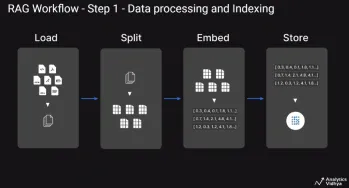
This article explores Retrieval Augmented Generation (RAG) systems and how AI agents can enhance their capabilities. Traditional RAG systems, while useful for leveraging custom enterprise data, suffer from limitations such as a lack of real-time dat


Hot AI Tools

Undresser.AI Undress
AI-powered app for creating realistic nude photos

AI Clothes Remover
Online AI tool for removing clothes from photos.

Undress AI Tool
Undress images for free

Clothoff.io
AI clothes remover

Video Face Swap
Swap faces in any video effortlessly with our completely free AI face swap tool!

Hot Article

Hot Tools

SecLists
SecLists is the ultimate security tester's companion. It is a collection of various types of lists that are frequently used during security assessments, all in one place. SecLists helps make security testing more efficient and productive by conveniently providing all the lists a security tester might need. List types include usernames, passwords, URLs, fuzzing payloads, sensitive data patterns, web shells, and more. The tester can simply pull this repository onto a new test machine and he will have access to every type of list he needs.
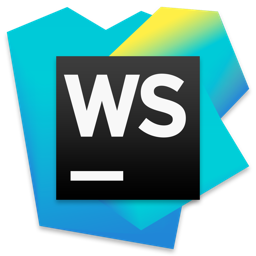
WebStorm Mac version
Useful JavaScript development tools

Atom editor mac version download
The most popular open source editor
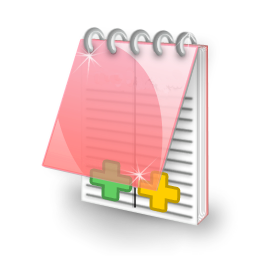
EditPlus Chinese cracked version
Small size, syntax highlighting, does not support code prompt function

DVWA
Damn Vulnerable Web App (DVWA) is a PHP/MySQL web application that is very vulnerable. Its main goals are to be an aid for security professionals to test their skills and tools in a legal environment, to help web developers better understand the process of securing web applications, and to help teachers/students teach/learn in a classroom environment Web application security. The goal of DVWA is to practice some of the most common web vulnerabilities through a simple and straightforward interface, with varying degrees of difficulty. Please note that this software