Introduction
Large Language Models (LLMs) are revolutionizing natural language processing, but their immense size and computational demands limit deployment. Quantization, a technique to shrink models and lower computational costs, is a crucial solution. This paper comprehensively explores LLM quantization, examining various methods, their effects on performance, and practical applications across diverse fields. We also discuss challenges and future research directions.
Overview
This paper analyzes:
- How quantization reduces the computational burden of LLMs without significant performance loss.
- The challenges posed by the size and resource needs of advanced LLMs.
- Quantization as a method to discretize continuous values, simplifying LLMs.
- Different quantization methods (post-training and quantization-aware training), and their performance impact.
- The potential of quantized LLMs in edge computing, mobile apps, and autonomous systems.
- Trade-offs, hardware considerations, and the need for ongoing research to improve LLM quantization.
Table of contents
- The Rise of Large Language Models
- LLM Quantization: An In-Depth Look
- Understanding Quantization
- Quantization Methods
- Visualizations
- Quantization's Effect on Model Performance
- Key Metrics
- Applications of Quantized LLMs
- Challenges in LLM Quantization
- Frequently Asked Questions
The Rise of Large Language Models
LLMs represent a major advancement in natural language processing, powering innovative applications. However, their size and computational intensity make deployment on resource-limited devices difficult. Quantization, a technique to reduce model complexity while preserving functionality, offers a promising solution to this problem. This paper provides a thorough examination of LLM quantization, covering its theoretical foundations, practical implementation, and real-world uses. We analyze different quantization methods, their impact on performance, and deployment challenges to provide a complete understanding.
LLM Quantization: An In-Depth Look
Understanding Quantization
Quantization maps continuous values to discrete representations, usually with lower bit-widths. In LLMs, this means reducing the precision of weights and activations from floating-point to lower-bit integers or fixed-point formats. This results in smaller models, faster inference, and reduced memory usage.
Quantization Methods
-
Post-training Quantization:
- Uniform quantization: Maps floating-point values to a fixed number of quantization levels. This is straightforward but can introduce errors, especially with skewed data.
- Dynamic quantization: Adapts quantization parameters during inference based on input statistics. This can improve accuracy but adds computational overhead.
- Weight clustering: Groups weights into clusters, representing each with a central value. This reduces the number of unique weights, saving memory and potentially improving computation.
- Quantization-Aware Training (QAT): Integrates quantization into training, improving performance. Techniques include simulated quantization, the straight-through estimator (STE), and differentiable quantization.
Visualizations
Uniform Quantization:
Illustrates mapping floating-point values to discrete levels.
Dynamic Quantization:
Shows how the quantization range adjusts based on input values.
Weight Clustering:
Depicts grouping weights into clusters represented by central values.
Quantization-Aware Training:
Illustrates the integration of quantization into the training process.
Quantization's Effect on Model Performance
Quantization inevitably reduces performance. The extent of this depends on:
- Model Architecture: Deeper, wider models are more robust to quantization.
- Dataset Size and Complexity: Larger, more complex datasets mitigate performance loss.
- Quantization Bitwidth: Lower bitwidths lead to greater performance drops.
- Quantization Method: The chosen method significantly impacts performance.
Key Metrics
Performance is assessed using:
- Accuracy: Measures performance on a given task (e.g., classification accuracy, BLEU score).
- Model Size: Quantifies the reduction in model size.
- Inference Speed: Evaluates speed improvements from quantization.
- Energy Consumption: Measures the power efficiency of the quantized model.
Applications of Quantized LLMs
Quantized LLMs are transforming various applications:
- Edge Computing: Deploying LLMs on resource-constrained devices for real-time applications.
- Mobile Applications: Improving the performance and efficiency of mobile apps.
- Internet of Things (IoT): Enabling intelligent capabilities on IoT devices.
- Autonomous Systems: Reducing computational costs for real-time decision-making.
- Natural Language Understanding (NLU): Accelerating NLU tasks across various domains.
(Python code snippet for autonomous systems omitted for brevity, but the description remains.)
Challenges in LLM Quantization
Despite its potential, LLM quantization faces challenges:
- Performance-Accuracy Trade-off: Balancing model size reduction with performance degradation.
- Hardware Acceleration: Developing specialized hardware for efficient quantization operations.
- Task-Specific Quantization: Tailoring techniques for different tasks and domains.
Future Research:
- Developing novel quantization methods with minimal performance loss.
- Exploring hardware-software co-design for optimized quantization.
- Investigating the impact of quantization on different LLM architectures.
- Quantifying the environmental benefits of LLM quantization.
Conclusion
LLM quantization is crucial for deploying large language models on resource-limited platforms. By carefully selecting quantization methods, evaluation metrics, and application requirements, practitioners can effectively utilize this technique to achieve optimal performance and efficiency. Continued research promises further advancements, unlocking new possibilities for AI applications.
Frequently Asked Questions
Q1. What is LLM Quantization? It reduces the precision of model weights and activations to lower-bit formats, resulting in smaller, faster, and more memory-efficient models.
Q2. What are the main quantization methods? Post-Training Quantization (uniform and dynamic) and Quantization-Aware Training (QAT).
Q3. What challenges does LLM Quantization face? Balancing performance and accuracy, the need for specialized hardware, and the development of task-specific quantization techniques.
Q4. How does quantization affect model performance? It can degrade performance, but the impact varies depending on model architecture, dataset complexity, and the bitwidth used.
The above is the detailed content of A Comprehensive Guide on LLM Quantization and Use Cases. For more information, please follow other related articles on the PHP Chinese website!
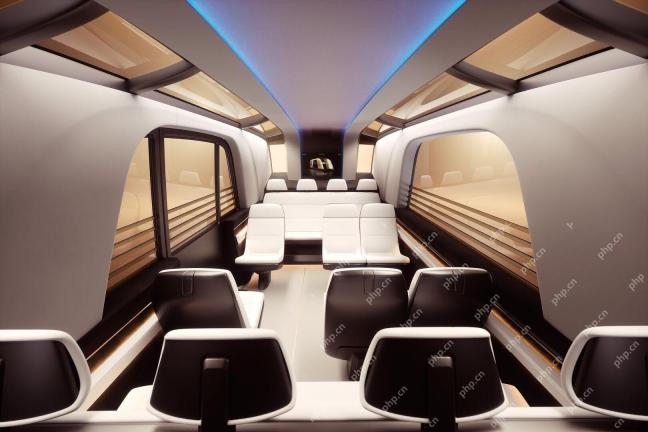
Since 2008, I've championed the shared-ride van—initially dubbed the "robotjitney," later the "vansit"—as the future of urban transportation. I foresee these vehicles as the 21st century's next-generation transit solution, surpas
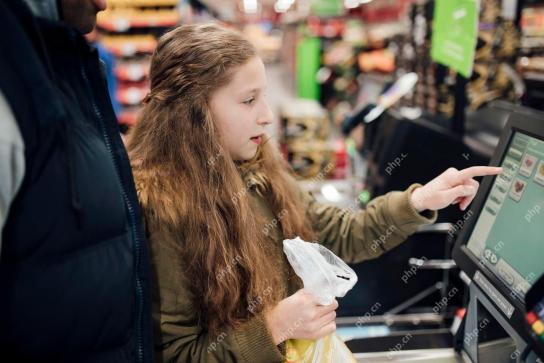
Revolutionizing the Checkout Experience Sam's Club's innovative "Just Go" system builds on its existing AI-powered "Scan & Go" technology, allowing members to scan purchases via the Sam's Club app during their shopping trip.
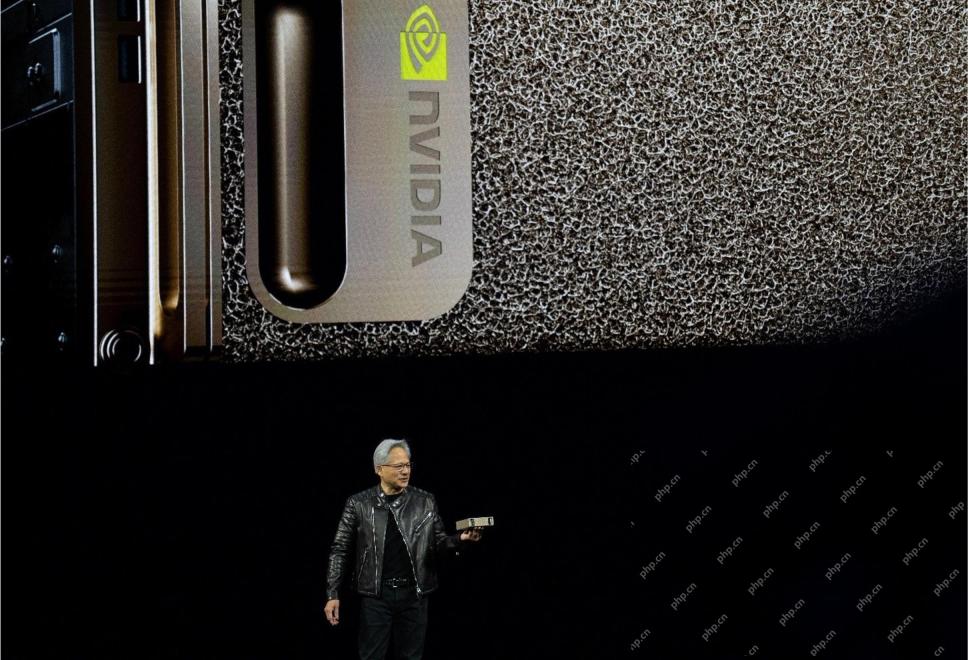
Nvidia's Enhanced Predictability and New Product Lineup at GTC 2025 Nvidia, a key player in AI infrastructure, is focusing on increased predictability for its clients. This involves consistent product delivery, meeting performance expectations, and
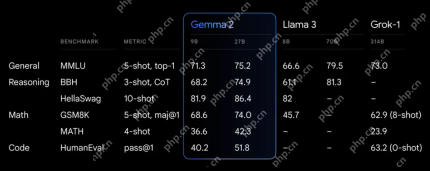
Google's Gemma 2: A Powerful, Efficient Language Model Google's Gemma family of language models, celebrated for efficiency and performance, has expanded with the arrival of Gemma 2. This latest release comprises two models: a 27-billion parameter ver
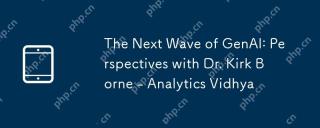
This Leading with Data episode features Dr. Kirk Borne, a leading data scientist, astrophysicist, and TEDx speaker. A renowned expert in big data, AI, and machine learning, Dr. Borne offers invaluable insights into the current state and future traje
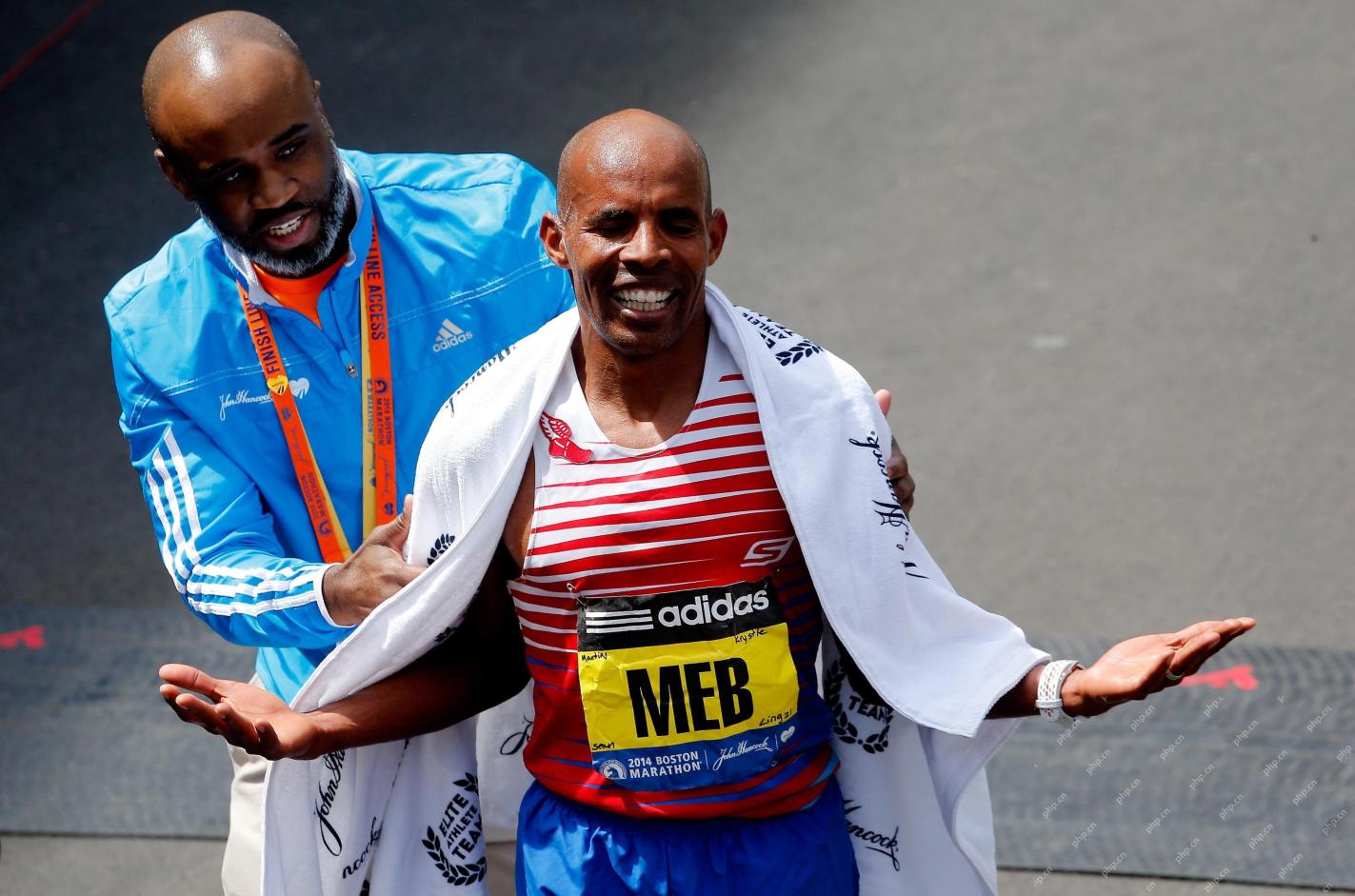
There were some very insightful perspectives in this speech—background information about engineering that showed us why artificial intelligence is so good at supporting people’s physical exercise. I will outline a core idea from each contributor’s perspective to demonstrate three design aspects that are an important part of our exploration of the application of artificial intelligence in sports. Edge devices and raw personal data This idea about artificial intelligence actually contains two components—one related to where we place large language models and the other is related to the differences between our human language and the language that our vital signs “express” when measured in real time. Alexander Amini knows a lot about running and tennis, but he still
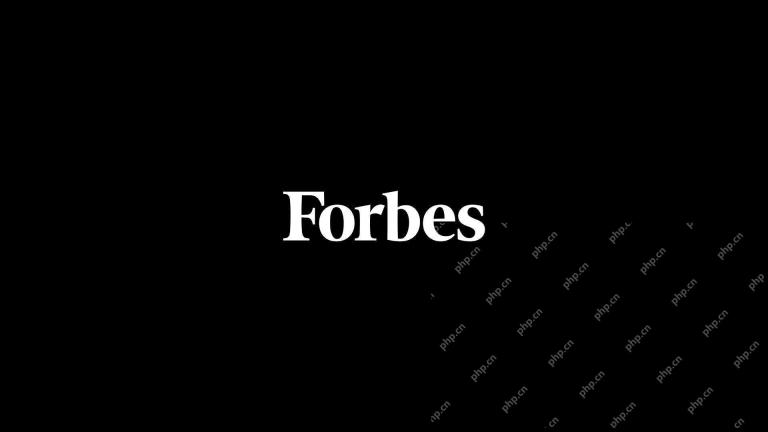
Caterpillar's Chief Information Officer and Senior Vice President of IT, Jamie Engstrom, leads a global team of over 2,200 IT professionals across 28 countries. With 26 years at Caterpillar, including four and a half years in her current role, Engst
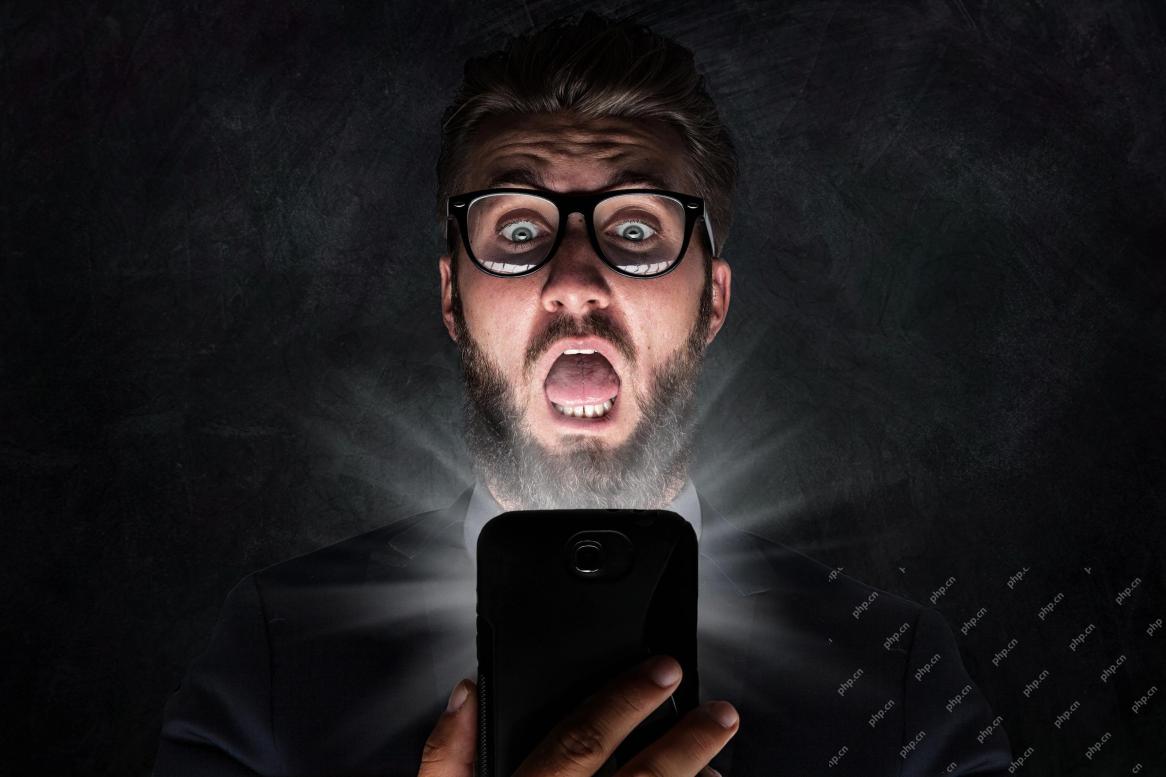
Google Photos' New Ultra HDR Tool: A Quick Guide Enhance your photos with Google Photos' new Ultra HDR tool, transforming standard images into vibrant, high-dynamic-range masterpieces. Ideal for social media, this tool boosts the impact of any photo,


Hot AI Tools

Undresser.AI Undress
AI-powered app for creating realistic nude photos

AI Clothes Remover
Online AI tool for removing clothes from photos.

Undress AI Tool
Undress images for free

Clothoff.io
AI clothes remover

Video Face Swap
Swap faces in any video effortlessly with our completely free AI face swap tool!

Hot Article

Hot Tools

Atom editor mac version download
The most popular open source editor

SublimeText3 Linux new version
SublimeText3 Linux latest version

mPDF
mPDF is a PHP library that can generate PDF files from UTF-8 encoded HTML. The original author, Ian Back, wrote mPDF to output PDF files "on the fly" from his website and handle different languages. It is slower than original scripts like HTML2FPDF and produces larger files when using Unicode fonts, but supports CSS styles etc. and has a lot of enhancements. Supports almost all languages, including RTL (Arabic and Hebrew) and CJK (Chinese, Japanese and Korean). Supports nested block-level elements (such as P, DIV),

Zend Studio 13.0.1
Powerful PHP integrated development environment

SecLists
SecLists is the ultimate security tester's companion. It is a collection of various types of lists that are frequently used during security assessments, all in one place. SecLists helps make security testing more efficient and productive by conveniently providing all the lists a security tester might need. List types include usernames, passwords, URLs, fuzzing payloads, sensitive data patterns, web shells, and more. The tester can simply pull this repository onto a new test machine and he will have access to every type of list he needs.