How to conduct deep learning in PyTorch under CentOS
Using PyTorch for deep learning on CentOS system requires step-by-step operation:
1. PyTorch installation
You can choose Anaconda or pip to install PyTorch.
A. Anaconda installation
Download Anaconda: Download the Anaconda3 installation package for CentOS system from the official Anaconda website . Follow the installation wizard to complete the installation.
-
Create a virtual environment: Open the terminal, create a virtual environment named
pytorch
and activate:conda create -n pytorch python=3.8 conda activated pytorch
-
Install PyTorch: In the activated
pytorch
environment, use conda to install PyTorch. If you need GPU acceleration, make sure you have CUDA and cuDNN installed and select the corresponding PyTorch version. The following command installs PyTorch containing CUDA 11.8 support:conda install pytorch torchvision torchaudio cudatoolkit=11.8 -c pytorch
-
Verify installation: Start the Python interactive environment, run the following code to verify that PyTorch is installed successfully, and check GPU availability:
import torch print(torch.__version__) print(torch.cuda.is_available())
B. pip installation
-
Install pip: If your system does not have pip installed, please install it first:
sudo yum install python3-pip
-
Install PyTorch: Use pip to install PyTorch and use Tsinghua University mirror source to speed up downloading:
pip install torch torchvision torchaudio -f https://pypi.tuna.tsinghua.edu.cn/simple
-
Verify installation: Same as Anaconda method, run the following code to verify installation:
import torch print(torch.__version__) print(torch.cuda.is_available())
2. Deep learning practice
Here is a simple MNIST handwritten numeric recognition example that demonstrates how to use PyTorch for deep learning:
-
Import library:
import torch import torch.nn as nn import torch.optim as optim import torchvision import torchvision.transforms as transforms
-
Defining the model: This is a simple convolutional neural network (CNN):
class SimpleCNN(nn.Module): def __init__(self): super(SimpleCNN, self).__init__() self.conv1 = nn.Conv2d(1, 32, kernel_size=3, padding=1) self.pool = nn.MaxPool2d(2, 2) self.fc1 = nn.Linear(32 * 14 * 14, 10) #Adjust the input dimension of the fully connected layer def forward(self, x): x = self.pool(torch.relu(self.conv1(x))) x = torch.flatten(x, 1) # Flatten x = self.fc1(x) Return x
-
Prepare the data: Download the MNIST dataset and preprocess it:
transform = transforms.Compose([transforms.ToTensor(), transforms.Normalize((0.1307,), (0.3081,))]) train_dataset = torchvision.datasets.MNIST(root='./data', train=True, download=True, transform=transform) test_dataset = torchvision.datasets.MNIST(root='./data', train=False, download=True, transform=transform) train_loader = torch.utils.data.DataLoader(train_dataset, batch_size=64, shuffle=True) test_loader = torch.utils.data.DataLoader(test_dataset, batch_size=1000, shuffle=False)
-
Initialize the model, loss function, and optimizer:
model = SimpleCNN() criteria = nn.CrossEntropyLoss() optimizer = optim.Adam(model.parameters(), lr=0.001) # Use Adam Optimizer
-
Training the model:
epochs = 2 for epoch in range(epochs): running_loss = 0.0 for i, data in enumerate(train_loader, 0): inputs, labels = data optimizer.zero_grad() outputs = model(inputs) loss = criteria(outputs, labels) loss.backward() optimizer.step() running_loss = loss.item() if i % 100 == 99: print(f'[{epoch 1}, {i 1}] loss: {running_loss / 100:.3f}') running_loss = 0.0 print('Finished Training')
-
Model evaluation:
correct = 0 total = 0 with torch.no_grad(): for data in test_loader: images, labels = data outputs = model(images) _, predicted = torch.max(outputs.data, 1) total = labels.size(0) correct = (predicted == labels).sum().item() print(f'Accuracy: {100 * correct / total}%')
This example provides a basic framework. You can modify the model structure, dataset, and hyperparameters according to your needs. Remember to create the ./data
directory before running. This example uses the Adam optimizer and generally converges faster than SGD. The input size of the fully connected layer is also adjusted to suit the output after the pooling layer.
The above is the detailed content of How to conduct deep learning in PyTorch under CentOS. For more information, please follow other related articles on the PHP Chinese website!

CentOSStream is a cutting-edge version of RHEL, providing an open platform for users to experience the new RHEL functions in advance. 1.CentOSStream is the upstream development and testing environment of RHEL, connecting RHEL and Fedora. 2. Through rolling releases, users can continuously receive updates, but they need to pay attention to stability. 3. The basic usage is similar to traditional CentOS and needs to be updated frequently; advanced usage can be used to develop new functions. 4. Frequently asked questions include package compatibility and configuration file changes, and requires debugging using dnf and diff. 5. Performance optimization suggestions include regular cleaning of the system, optimizing update policies and monitoring system performance.

The reason for the end of CentOS is RedHat's business strategy adjustment, community-business balance and market competition. Specifically manifested as: 1. RedHat accelerates the RHEL development cycle through CentOSStream and attracts more users to participate in the RHEL ecosystem. 2. RedHat needs to find a balance between supporting open source communities and promoting commercial products, and CentOSStream can better convert community contributions into RHEL improvements. 3. Faced with fierce competition in the Linux market, RedHat needs new strategies to maintain its leading position in the enterprise-level market.

RedHat shut down CentOS8.x and launches CentOSStream because it hopes to provide a platform closer to the RHEL development cycle through the latter. 1. CentOSStream, as the upstream development platform of RHEL, adopts a rolling release mode. 2. This transformation aims to enable the community to get exposure to new RHEL features earlier and provide feedback to accelerate the RHEL development cycle. 3. Users need to adapt to changing systems and reevaluate system requirements and migration strategies.

CentOS stands out among enterprise Linux distributions because of its stability, security, community support and enterprise application advantages. 1. Stability: The update cycle is long and the software package has been strictly tested. 2. Security: Inherit the security features of RHEL, update and announce in a timely manner. 3. Community support: a huge community and detailed documentation to respond to problems quickly. 4. Enterprise applications: Support container technologies such as Docker, suitable for modern application deployment.

Alternatives to CentOS include AlmaLinux, RockyLinux, and OracleLinux. 1.AlmaLinux provides RHEL compatibility and community-driven development. 2. RockyLinux emphasizes enterprise-level support and long-term maintenance. 3. OracleLinux provides Oracle-specific optimization and support. These alternatives have similar stability and compatibility to CentOS, and are suitable for users with different needs.

CentOS is suitable for enterprise and server environments due to its stability and long life cycle. 1.CentOS provides up to 10 years of support, suitable for scenarios that require stable operation. 2.Ubuntu is suitable for environments that require quick updates and user-friendly. 3.Debian is suitable for developers who need pure and free software. 4.Fedora is suitable for users who like to try the latest technologies.

Alternatives to CentOS include AlmaLinux, RockyLinux, and OracleLinux. 1.AlmaLinux and RockyLinux rebuild RHEL 1:1, providing high stability and compatibility, suitable for enterprise environments. 2. OracleLinux provides high performance through UEK, suitable for users who are familiar with the Oracle technology stack. 3. When choosing, stability, community support and package management should be considered.

CentOS alternatives include RockyLinux, AlmaLinux, and OracleLinux. 1. RockyLinux and AlmaLinux provide stable distributions compatible with RHEL, suitable for users who need long-term support. 2. CentOSStream is suitable for users who focus on new features and development cycles. 3. OracleLinux is suitable for users who need enterprise-level support.


Hot AI Tools

Undresser.AI Undress
AI-powered app for creating realistic nude photos

AI Clothes Remover
Online AI tool for removing clothes from photos.

Undress AI Tool
Undress images for free

Clothoff.io
AI clothes remover

Video Face Swap
Swap faces in any video effortlessly with our completely free AI face swap tool!

Hot Article

Hot Tools
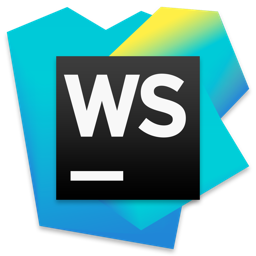
WebStorm Mac version
Useful JavaScript development tools

Notepad++7.3.1
Easy-to-use and free code editor

DVWA
Damn Vulnerable Web App (DVWA) is a PHP/MySQL web application that is very vulnerable. Its main goals are to be an aid for security professionals to test their skills and tools in a legal environment, to help web developers better understand the process of securing web applications, and to help teachers/students teach/learn in a classroom environment Web application security. The goal of DVWA is to practice some of the most common web vulnerabilities through a simple and straightforward interface, with varying degrees of difficulty. Please note that this software

MinGW - Minimalist GNU for Windows
This project is in the process of being migrated to osdn.net/projects/mingw, you can continue to follow us there. MinGW: A native Windows port of the GNU Compiler Collection (GCC), freely distributable import libraries and header files for building native Windows applications; includes extensions to the MSVC runtime to support C99 functionality. All MinGW software can run on 64-bit Windows platforms.

SublimeText3 Chinese version
Chinese version, very easy to use
